mirror of
https://github.com/google/benchmark.git
synced 2025-02-14 05:10:13 +08:00
The first problem you have to solve yourself. The second one can be aided. The benchmark library can compute some statistics over the repetitions, which helps with grasping the results somewhat. But that is only for the one set of results. It does not really help to compare the two benchmark results, which is the interesting bit. Thankfully, there are these bundled `tools/compare.py` and `tools/compare_bench.py` scripts. They can provide a diff between two benchmarking results. Yay! Except not really, it's just a diff, while it is very informative and better than nothing, it does not really help answer The Question - am i just looking at the noise? It's like not having these per-benchmark statistics... Roughly, we can formulate the question as: > Are these two benchmarks the same? > Did my change actually change anything, or is the difference below the noise level? Well, this really sounds like a [null hypothesis](https://en.wikipedia.org/wiki/Null_hypothesis), does it not? So maybe we can use statistics here, and solve all our problems? lol, no, it won't solve all the problems. But maybe it will act as a tool, to better understand the output, just like the usual statistics on the repetitions... I'm making an assumption here that most of the people care about the change of average value, not the standard deviation. Thus i believe we can use T-Test, be it either [Student's t-test](https://en.wikipedia.org/wiki/Student%27s_t-test), or [Welch's t-test](https://en.wikipedia.org/wiki/Welch%27s_t-test). **EDIT**: however, after @dominichamon review, it was decided that it is better to use more robust [Mann–Whitney U test](https://en.wikipedia.org/wiki/Mann–Whitney_U_test) I'm using [scipy.stats.mannwhitneyu](https://docs.scipy.org/doc/scipy/reference/generated/scipy.stats.mannwhitneyu.html#scipy.stats.mannwhitneyu). There are two new user-facing knobs: ``` $ ./compare.py --help usage: compare.py [-h] [-u] [--alpha UTEST_ALPHA] {benchmarks,filters,benchmarksfiltered} ... versatile benchmark output compare tool <...> optional arguments: -h, --help show this help message and exit -u, --utest Do a two-tailed Mann-Whitney U test with the null hypothesis that it is equally likely that a randomly selected value from one sample will be less than or greater than a randomly selected value from a second sample. WARNING: requires **LARGE** (9 or more) number of repetitions to be meaningful! --alpha UTEST_ALPHA significance level alpha. if the calculated p-value is below this value, then the result is said to be statistically significant and the null hypothesis is rejected. (default: 0.0500) ``` Example output: 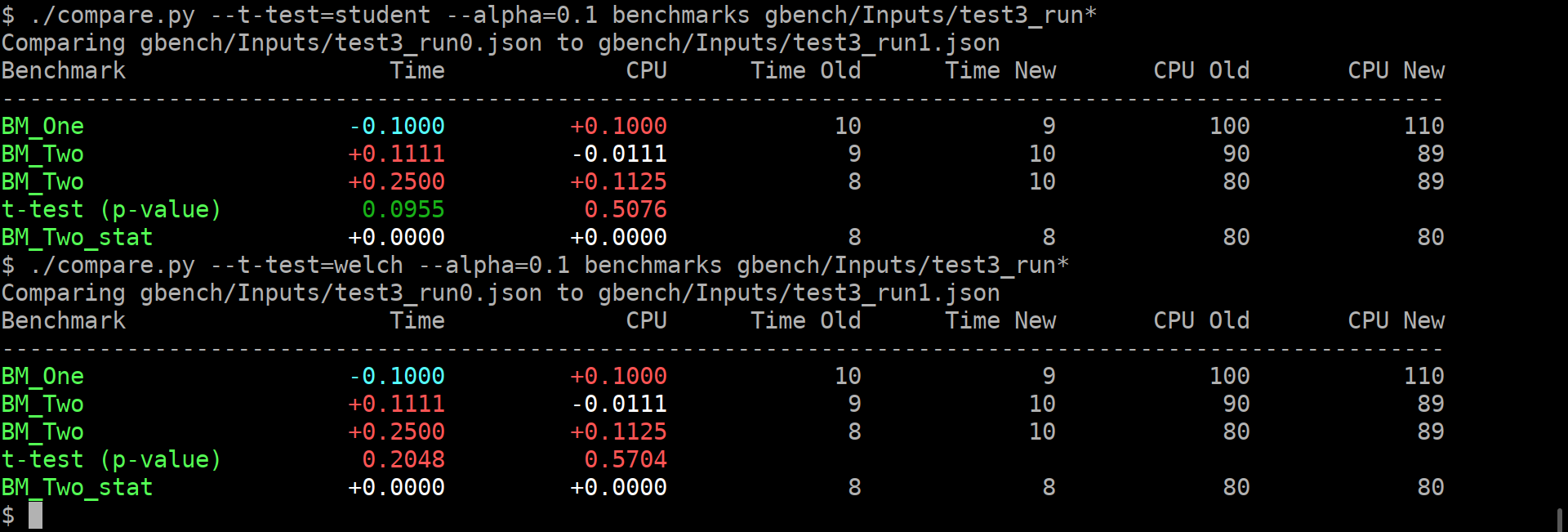 As you can guess, the alpha does affect anything but the coloring of the computed p-values. If it is green, then the change in the average values is statistically-significant. I'm detecting the repetitions by matching name. This way, no changes to the json are _needed_. Caveats: * This won't work if the json is not in the same order as outputted by the benchmark, or if the parsing does not retain the ordering. * This won't work if after the grouped repetitions there isn't at least one row with different name (e.g. statistic). Since there isn't a knob to disable printing of statistics (only the other way around), i'm not too worried about this. * **The results will be wrong if the repetition count is different between the two benchmarks being compared.** * Even though i have added (hopefully full) test coverage, the code of these python tools is staring to look a bit jumbled. * So far i have added this only to the `tools/compare.py`. Should i add it to `tools/compare_bench.py` too? Or should we deduplicate them (by removing the latter one)? |
||
---|---|---|
.. | ||
gbench | ||
compare_bench.py | ||
compare.py | ||
strip_asm.py |