mirror of
https://github.com/Vonng/ddia.git
synced 2025-01-05 15:30:06 +08:00
add en-us intro,summary,references
This commit is contained in:
parent
1c734bfbd1
commit
a5f4852a23
50
en-us/README.md
Normal file
50
en-us/README.md
Normal file
@ -0,0 +1,50 @@
|
||||
# Designing Data-Intensive Applications
|
||||
|
||||
—— **The Big Ideas Behind Reliable, Scalable, and Maintainable Systems**
|
||||
|
||||
[Martin Kleppmann](https://martin.kleppmann.com)
|
||||
|
||||
> The en-us version only includes **intro**, **summary**, **references** of all chapters to protect the intellectual property of author and publisher.
|
||||
|
||||
|
||||
--------
|
||||
|
||||
*Technology is a powerful force in our society. Data, software, and communication can*
|
||||
|
||||
*be used for bad: to entrench unfair power structures, to undermine human rights, and to protect vested interests. But they can also be used for good: to make underrepresented people’s voices heard, to create opportunities for everyone, and to avert disasters. This book is dedicated to everyone working toward the good.*
|
||||
|
||||
---------
|
||||
|
||||
*Computing is pop culture. [...] Pop culture holds a disdain for history. Pop culture is all about identity and feeling like you’re participating. It has nothing to do with cooperation, the past or the future—it’s living in the present. I think the same is true of most people who write code for money. They have no idea where [their culture came from].*
|
||||
|
||||
— [Alan Kay](http://www.drdobbs.com/architecture-and-design/interview-with-alan-kay/240003442), in interview with *Dr Dobb’s Journal* (2012)
|
||||
|
||||
---------
|
||||
|
||||
|
||||
## Table of Contents
|
||||
|
||||
### [Preface](preface.md)
|
||||
|
||||
### [Part I: Foundations of Data Systems](part-i.md)
|
||||
- [1. Reliable, Scalable, and Maintainable Applications](ch1.md)
|
||||
- [2. Data Models and Query Languages](ch2.md)
|
||||
- [3. Storage and Retrieval](ch3.md)
|
||||
- [4. Encoding and Evolution](ch4.md)
|
||||
|
||||
### [Part II: Distributed Data](part-ii.md)
|
||||
- [5. Replication](ch5.md)
|
||||
- [6. Partitioning](ch6.md)
|
||||
- [7. Transactions](ch7.md)
|
||||
- [8. The Trouble with Distributed Systems](ch8.md)
|
||||
- [9. Consistency and Consensus](ch9.md)
|
||||
|
||||
### [Part III: Derived Data](part-iii.md)
|
||||
- [10. Batch Processing](ch10.md)
|
||||
- [11. Stream Processing](ch11.md)
|
||||
- [12. The Future of Data Systems](ch12.md)
|
||||
|
||||
### [Glossary](glossary.md)
|
||||
|
||||
### [Colophon](colophon.md)
|
||||
|
21
en-us/SUMMARY.md
Normal file
21
en-us/SUMMARY.md
Normal file
@ -0,0 +1,21 @@
|
||||
# Summary
|
||||
|
||||
* [Preface](preface.md)
|
||||
* [Part I: Foundations of Data Systems](part-i.md)
|
||||
- [1. Reliable, Scalable, and Maintainable Applications](ch1.md)
|
||||
- [2. Data Models and Query Languages](ch2.md)
|
||||
- [3. Storage and Retrieval](ch3.md)
|
||||
- [4. Encoding and Evolution](ch4.md)
|
||||
* [Part II: Distributed Data](part-ii.md)
|
||||
- [5. Replication](ch5.md)
|
||||
- [6. Partitioning](ch6.md)
|
||||
- [7. Transactions](ch7.md)
|
||||
- [8. The Trouble with Distributed Systems](ch8.md)
|
||||
- [9. Consistency and Consensus](ch9.md)
|
||||
* [Part III: Derived Data](part-iii.md)
|
||||
- [10. Batch Processing](ch10.md)
|
||||
- [11. Stream Processing](ch11.md)
|
||||
- [12. The Future of Data Systems](ch12.md)
|
||||
* [Glossary](glossary.md)
|
||||
* [Colophon](colophon.md)
|
||||
|
4
en-us/_navbar.md
Normal file
4
en-us/_navbar.md
Normal file
@ -0,0 +1,4 @@
|
||||
- Languages
|
||||
- [Chineses](/)
|
||||
- [Chinese (Traditional)](/zh-tw/)
|
||||
- [English](/en-us/)
|
19
en-us/_sidebar.md
Normal file
19
en-us/_sidebar.md
Normal file
@ -0,0 +1,19 @@
|
||||
- [Preface](preface.md)
|
||||
- [Part I: Foundations of Data Systems](part-i.md)
|
||||
- [1. Reliable, Scalable, and Maintainable Applications](ch1.md)
|
||||
- [2. Data Models and Query Languages](ch2.md)
|
||||
- [3. Storage and Retrieval](ch3.md)
|
||||
- [4. Encoding and Evolution](ch4.md)
|
||||
- [Part II: Distributed Data](part-ii.md)
|
||||
- [5. Replication](ch5.md)
|
||||
- [6. Partitioning](ch6.md)
|
||||
- [7. Transactions](ch7.md)
|
||||
- [8. The Trouble with Distributed Systems](ch8.md)
|
||||
- [9. Consistency and Consensus](ch9.md)
|
||||
- [Part III: Derived Data](part-iii.md)
|
||||
- [10. Batch Processing](ch10.md)
|
||||
- [11. Stream Processing](ch11.md)
|
||||
- [12. The Future of Data Systems](ch12.md)
|
||||
- [Glossary](glossary.md)
|
||||
- [Colophon](colophon.md)
|
||||
|
124
en-us/ch1.md
Normal file
124
en-us/ch1.md
Normal file
@ -0,0 +1,124 @@
|
||||
# 1. Reliable, Scalable, and Maintainable Applications
|
||||
|
||||

|
||||
|
||||
> *The Internet was done so well that most people think of it as a natural resource like the Pacific Ocean, rather than something that was man-made. When was the last time a tech‐ nology with a scale like that was so error-free?*
|
||||
>
|
||||
> — [Alan Kay](http://www.drdobbs.com/architecture-and-design/interview-with-alan-kay/240003442), in interview with *Dr Dobb’s Journal* (2012)
|
||||
|
||||
-----------------------
|
||||
|
||||
Many applications today are *data-intensive*, as opposed to *compute-intensive*. Raw CPU power is rarely a limiting factor for these applications—bigger problems are usually the amount of data, the complexity of data, and the speed at which it is changing.
|
||||
|
||||
A data-intensive application is typically built from standard building blocks that pro‐ vide commonly needed functionality. For example, many applications need to:
|
||||
|
||||
- Store data so that they, or another application, can find it again later (*databases*)
|
||||
- Remember the result of an expensive operation, to speed up reads (*caches*)
|
||||
- Allow users to search data by keyword or filter it in various ways (*search indexes*)
|
||||
- Send a message to another process, to be handled asynchronously (*stream pro‐ cessing*)
|
||||
- Periodically crunch a large amount of accumulated data (*batch processing*)
|
||||
|
||||
If that sounds painfully obvious, that’s just because these *data systems* are such a suc‐ cessful abstraction: we use them all the time without thinking too much. When build‐ ing an application, most engineers wouldn’t dream of writing a new data storage engine from scratch, because databases are a perfectly good tool for the job.
|
||||
|
||||
But reality is not that simple. There are many database systems with different charac‐ teristics, because different applications have different requirements. There are vari‐ ous approaches to caching, several ways of building search indexes, and so on. When building an application, we still need to figure out which tools and which approaches are the most appropriate for the task at hand. And it can be hard to combine tools when you need to do something that a single tool cannot do alone.
|
||||
|
||||
This book is a journey through both the principles and the practicalities of data sys‐ tems, and how you can use them to build data-intensive applications. We will explore what different tools have in common, what distinguishes them, and how they achieve their characteristics.
|
||||
|
||||
In this chapter, we will start by exploring the fundamentals of what we are trying to achieve: reliable, scalable, and maintainable data systems. We’ll clarify what those things mean, outline some ways of thinking about them, and go over the basics that we will need for later chapters. In the following chapters we will continue layer by layer, looking at different design decisions that need to be considered when working on a data-intensive application.
|
||||
|
||||
|
||||
|
||||
## ……
|
||||
|
||||
|
||||
|
||||
## Summary
|
||||
|
||||
In this chapter, we have explored some fundamental ways of thinking about data-intensive applications. These principles will guide us through the rest of the book, where we dive into deep technical detail.
|
||||
|
||||
An application has to meet various requirements in order to be useful. There are *functional requirements* (what it should do, such as allowing data to be stored, retrieved, searched, and processed in various ways), and some *nonfunctional require‐ ments* (general properties like security, reliability, compliance, scalability, compatibil‐ ity, and maintainability). In this chapter we discussed reliability, scalability, and maintainability in detail.
|
||||
|
||||
*Reliability* means making systems work correctly, even when faults occur. Faults can be in hardware (typically random and uncorrelated), software (bugs are typically sys‐ tematic and hard to deal with), and humans (who inevitably make mistakes from time to time). Fault-tolerance techniques can hide certain types of faults from the end user.
|
||||
|
||||
*Scalability* means having strategies for keeping performance good, even when load increases. In order to discuss scalability, we first need ways of describing load and performance quantitatively. We briefly looked at Twitter’s home timelines as an example of describing load, and response time percentiles as a way of measuring performance. In a scalable system, you can add processing capacity in order to remain reliable under high load.
|
||||
|
||||
*Maintainability* has many facets, but in essence it’s about making life better for the engineering and operations teams who need to work with the system. Good abstrac‐ tions can help reduce complexity and make the system easier to modify and adapt for new use cases. Good operability means having good visibility into the system’s health, and having effective ways of managing it.
|
||||
|
||||
There is unfortunately no easy fix for making applications reliable, scalable, or main‐ tainable. However, there are certain patterns and techniques that keep reappearing in different kinds of applications. In the next few chapters we will take a look at some examples of data systems and analyze how they work toward those goals.
|
||||
|
||||
Later in the book, in [Part III](part-iii.md), we will look at patterns for systems that consist of sev‐ eral components working together, such as the one in [Figure 1-1](../img/fig1-1.png).
|
||||
|
||||
|
||||
|
||||
## References
|
||||
--------------------
|
||||
|
||||
1. Michael Stonebraker and Uğur Çetintemel: “['One Size Fits All': An Idea Whose Time Has Come and Gone](http://citeseerx.ist.psu.edu/viewdoc/download?doi=10.1.1.68.9136&rep=rep1&type=pdf),” at *21st International Conference on Data Engineering* (ICDE), April 2005.
|
||||
|
||||
1. Walter L. Heimerdinger and Charles B. Weinstock: “[A Conceptual Framework for System Fault Tolerance](http://www.sei.cmu.edu/reports/92tr033.pdf),” Technical Report CMU/SEI-92-TR-033, Software Engineering Institute, Carnegie Mellon University, October 1992.
|
||||
|
||||
2. Ding Yuan, Yu Luo, Xin Zhuang, et al.: “[Simple Testing Can Prevent Most Critical Failures: An Analysis of Production Failures in Distributed Data-Intensive Systems](https://www.usenix.org/system/files/conference/osdi14/osdi14-paper-yuan.pdf),” at *11th USENIX Symposium on Operating Systems Design and Implementation* (OSDI), October 2014.
|
||||
|
||||
3. Yury Izrailevsky and Ariel Tseitlin: “[The Netflix Simian Army](http://techblog.netflix.com/2011/07/netflix-simian-army.html),” *techblog.netflix.com*, July 19, 2011.
|
||||
|
||||
4. Daniel Ford, François Labelle, Florentina I. Popovici, et al.: “[Availability in Globally Distributed Storage Systems](http://research.google.com/pubs/archive/36737.pdf),” at *9th USENIX Symposium on Operating Systems Design and Implementation* (OSDI),
|
||||
October 2010.
|
||||
|
||||
5. Brian Beach: “[Hard Drive Reliability Update – Sep 2014](https://www.backblaze.com/blog/hard-drive-reliability-update-september-2014/),” *backblaze.com*, September 23, 2014.
|
||||
|
||||
6. Laurie Voss: “[AWS: The Good, the Bad and the Ugly](https://web.archive.org/web/20160429075023/http://blog.awe.sm/2012/12/18/aws-the-good-the-bad-and-the-ugly/),” *blog.awe.sm*, December 18, 2012.
|
||||
|
||||
7. Haryadi S. Gunawi, Mingzhe Hao, Tanakorn Leesatapornwongsa, et al.: “[What Bugs Live in the Cloud?](http://ucare.cs.uchicago.edu/pdf/socc14-cbs.pdf),” at *5th ACM Symposium on Cloud Computing* (SoCC), November 2014. [doi:10.1145/2670979.2670986](http://dx.doi.org/10.1145/2670979.2670986)
|
||||
|
||||
8. Nelson Minar: “[Leap Second Crashes Half the Internet](http://www.somebits.com/weblog/tech/bad/leap-second-2012.html),” *somebits.com*, July 3, 2012.
|
||||
|
||||
9. Amazon Web Services: “[Summary of the Amazon EC2 and Amazon RDS Service Disruption in the US East Region](http://aws.amazon.com/message/65648/),” *aws.amazon.com*, April 29, 2011.
|
||||
|
||||
10. Richard I. Cook: “[How Complex Systems Fail](http://web.mit.edu/2.75/resources/random/How%20Complex%20Systems%20Fail.pdf),” Cognitive Technologies Laboratory, April 2000.
|
||||
|
||||
11. Jay Kreps: “[Getting Real About Distributed System Reliability](http://blog.empathybox.com/post/19574936361/getting-real-about-distributed-system-reliability),” *blog.empathybox.com*, March 19, 2012.
|
||||
|
||||
12. David Oppenheimer, Archana Ganapathi, and David A. Patterson: “[Why Do Internet Services Fail, and What Can Be Done About It?](http://static.usenix.org/legacy/events/usits03/tech/full_papers/oppenheimer/oppenheimer.pdf),” at *4th USENIX Symposium on Internet Technologies and Systems* (USITS), March 2003.
|
||||
|
||||
13. Nathan Marz: “[Principles of Software Engineering, Part 1](http://nathanmarz.com/blog/principles-of-software-engineering-part-1.html),” *nathanmarz.com*, April 2, 2013.
|
||||
|
||||
14. Michael Jurewitz:“[The Human Impact of Bugs](http://jury.me/blog/2013/3/14/the-human-impact-of-bugs),” *jury.me*, March 15, 2013.
|
||||
|
||||
15. Raffi Krikorian: “[Timelines at Scale](http://www.infoq.com/presentations/Twitter-Timeline-Scalability),” at *QCon San Francisco*, November 2012.
|
||||
|
||||
16. Martin Fowler: *Patterns of Enterprise Application Architecture*. Addison Wesley, 2002. ISBN: 978-0-321-12742-6
|
||||
|
||||
17. Kelly Sommers: “[After all that run around, what caused 500ms disk latency even when we replaced physical server?](https://twitter.com/kellabyte/status/532930540777635840)” *twitter.com*, November 13, 2014.
|
||||
|
||||
18. Giuseppe DeCandia, Deniz Hastorun, Madan Jampani, et al.: “[Dynamo: Amazon's Highly Available Key-Value Store](http://www.allthingsdistributed.com/files/amazon-dynamo-sosp2007.pdf),” at *21st ACM Symposium on Operating Systems Principles* (SOSP), October 2007.
|
||||
|
||||
19. Greg Linden: “[Make Data Useful](http://glinden.blogspot.co.uk/2006/12/slides-from-my-talk-at-stanford.html),” slides from presentation at Stanford University Data Mining class (CS345), December 2006.
|
||||
|
||||
20. Tammy Everts: “[The Real Cost of Slow Time vs Downtime](http://www.webperformancetoday.com/2014/11/12/real-cost-slow-time-vs-downtime-slides/),” *webperformancetoday.com*, November 12, 2014.
|
||||
|
||||
21. Jake Brutlag:“[Speed Matters for Google Web Search](http://googleresearch.blogspot.co.uk/2009/06/speed-matters.html),” *googleresearch.blogspot.co.uk*, June 22, 2009.
|
||||
|
||||
22. Tyler Treat: “[Everything You Know About Latency Is Wrong](http://bravenewgeek.com/everything-you-know-about-latency-is-wrong/),” *bravenewgeek.com*, December 12, 2015.
|
||||
|
||||
23. Jeffrey Dean and Luiz André Barroso: “[The Tail at Scale](http://cacm.acm.org/magazines/2013/2/160173-the-tail-at-scale/fulltext),” *Communications of the ACM*, volume 56, number 2, pages 74–80, February 2013. [doi:10.1145/2408776.2408794](http://dx.doi.org/10.1145/2408776.2408794)
|
||||
|
||||
24. Graham Cormode, Vladislav Shkapenyuk, Divesh Srivastava, and Bojian Xu: “[Forward Decay: A Practical Time Decay Model for Streaming Systems](http://dimacs.rutgers.edu/~graham/pubs/papers/fwddecay.pdf),” at *25th IEEE International Conference on Data Engineering* (ICDE), March 2009.
|
||||
|
||||
25. Ted Dunning and Otmar Ertl: “[Computing Extremely Accurate Quantiles Using t-Digests](https://github.com/tdunning/t-digest),” *github.com*, March 2014.
|
||||
|
||||
26. Gil Tene: “[HdrHistogram](http://www.hdrhistogram.org/),” *hdrhistogram.org*.
|
||||
|
||||
27. Baron Schwartz: “[Why Percentiles Don’t Work the Way You Think](https://www.vividcortex.com/blog/why-percentiles-dont-work-the-way-you-think),” *vividcortex.com*, December 7, 2015.
|
||||
|
||||
28. James Hamilton: “[On Designing and Deploying Internet-Scale Services](https://www.usenix.org/legacy/events/lisa07/tech/full_papers/hamilton/hamilton.pdf),” at *21st Large Installation
|
||||
System Administration Conference* (LISA), November 2007.
|
||||
|
||||
29. Brian Foote and Joseph Yoder: “[Big Ball of Mud](http://www.laputan.org/pub/foote/mud.pdf),” at *4th Conference on Pattern Languages of Programs* (PLoP), September 1997.
|
||||
|
||||
30. Frederick P Brooks: “No Silver Bullet – Essence and Accident in Software Engineering,” in *The Mythical Man-Month*, Anniversary edition, Addison-Wesley, 1995. ISBN: 978-0-201-83595-3
|
||||
|
||||
31. Ben Moseley and Peter Marks: “[Out of the Tar Pit](http://citeseerx.ist.psu.edu/viewdoc/summary?doi=10.1.1.93.8928),” at *BCS Software Practice Advancement* (SPA), 2006.
|
||||
|
||||
32. Rich Hickey: “[Simple Made Easy](http://www.infoq.com/presentations/Simple-Made-Easy),” at *Strange Loop*, September 2011.
|
||||
|
||||
33. Hongyu Pei Breivold, Ivica Crnkovic, and Peter J. Eriksson: “[Analyzing Software Evolvability](http://www.mrtc.mdh.se/publications/1478.pdf),” at *32nd Annual IEEE International Computer Software and Applications Conference* (COMPSAC), July 2008. [doi:10.1109/COMPSAC.2008.50](http://dx.doi.org/10.1109/COMPSAC.2008.50)
|
257
en-us/ch10.md
Normal file
257
en-us/ch10.md
Normal file
@ -0,0 +1,257 @@
|
||||
# 10. Batch Processing
|
||||
|
||||

|
||||
|
||||
> *A system cannot be successful if it is too strongly influenced by a single person. Once the initial design is complete and fairly robust, the real test begins as people with many different viewpoints undertake their own experiments.*
|
||||
>
|
||||
> — Donald Knuth
|
||||
|
||||
---------------
|
||||
|
||||
In the first two parts of this book we talked a lot about *requests* and *queries*, and the corresponding *responses* or *results*. This style of data processing is assumed in many modern data systems: you ask for something, or you send an instruction, and some time later the system (hopefully) gives you an answer. Databases, caches, search indexes, web servers, and many other systems work this way.
|
||||
|
||||
In such *online* systems, whether it’s a web browser requesting a page or a service call‐ ing a remote API, we generally assume that the request is triggered by a human user, and that the user is waiting for the response. They shouldn’t have to wait too long, so we pay a lot of attention to the *response time* of these systems (see “[Describing Performance](ch1.md#describing-performance)”).
|
||||
|
||||
The web, and increasing numbers of HTTP/REST-based APIs, has made the request/ response style of interaction so common that it’s easy to take it for granted. But we should remember that it’s not the only way of building systems, and that other approaches have their merits too. Let’s distinguish three different types of systems:
|
||||
|
||||
***Services (online systems)***
|
||||
|
||||
A service waits for a request or instruction from a client to arrive. When one is received, the service tries to handle it as quickly as possible and sends a response back. Response time is usually the primary measure of performance of a service, and availability is often very important (if the client can’t reach the service, the user will probably get an error message).
|
||||
|
||||
***Batch processing systems (offline systems)***
|
||||
|
||||
A batch processing system takes a large amount of input data, runs a *job* to pro‐ cess it, and produces some output data. Jobs often take a while (from a few minutes to several days), so there normally isn’t a user waiting for the job to fin‐ ish. Instead, batch jobs are often scheduled to run periodically (for example, once a day). The primary performance measure of a batch job is usually *throughput* (the time it takes to crunch through an input dataset of a certain size). We dis‐ cuss batch processing in this chapter.
|
||||
|
||||
***Stream processing systems (near-real-time systems)***
|
||||
|
||||
Stream processing is somewhere between online and offline/batch processing (so it is sometimes called *near-real-time* or *nearline* processing). Like a batch pro‐ cessing system, a stream processor consumes inputs and produces outputs (rather than responding to requests). However, a stream job operates on events shortly after they happen, whereas a batch job operates on a fixed set of input data. This difference allows stream processing systems to have lower latency than the equivalent batch systems. As stream processing builds upon batch process‐ ing, we discuss it in [Chapter 11](ch11.md).
|
||||
|
||||
As we shall see in this chapter, batch processing is an important building block in our quest to build reliable, scalable, and maintainable applications. For example, Map‐ Reduce, a batch processing algorithm published in 2004 [1], was (perhaps over- enthusiastically) called “the algorithm that makes Google so massively scalable” [2]. It was subsequently implemented in various open source data systems, including Hadoop, CouchDB, and MongoDB.
|
||||
|
||||
MapReduce is a fairly low-level programming model compared to the parallel pro‐ cessing systems that were developed for data warehouses many years previously [3, 4], but it was a major step forward in terms of the scale of processing that could be achieved on commodity hardware. Although the importance of MapReduce is now declining [5], it is still worth understanding, because it provides a clear picture of why and how batch processing is useful.
|
||||
|
||||
In fact, batch processing is a very old form of computing. Long before programmable digital computers were invented, punch card tabulating machines—such as the Hol‐ lerith machines used in the 1890 US Census [6]—implemented a semi-mechanized form of batch processing to compute aggregate statistics from large inputs. And Map‐ Reduce bears an uncanny resemblance to the electromechanical IBM card-sorting machines that were widely used for business data processing in the 1940s and 1950s [7]. As usual, history has a tendency of repeating itself.
|
||||
|
||||
In this chapter, we will look at MapReduce and several other batch processing algo‐ rithms and frameworks, and explore how they are used in modern data systems. But first, to get started, we will look at data processing using standard Unix tools. Even if you are already familiar with them, a reminder about the Unix philosophy is worthwhile because the ideas and lessons from Unix carry over to large-scale, heterogene‐ ous distributed data systems.
|
||||
|
||||
|
||||
|
||||
## ……
|
||||
|
||||
|
||||
|
||||
## Summary
|
||||
|
||||
|
||||
In this chapter we explored the topic of batch processing. We started by looking at Unix tools such as awk, grep, and sort, and we saw how the design philosophy of those tools is carried forward into MapReduce and more recent dataflow engines. Some of those design principles are that inputs are immutable, outputs are intended to become the input to another (as yet unknown) program, and complex problems are solved by composing small tools that “do one thing well.”
|
||||
|
||||
In the Unix world, the uniform interface that allows one program to be composed with another is files and pipes; in MapReduce, that interface is a distributed filesys‐ tem. We saw that dataflow engines add their own pipe-like data transport mecha‐ nisms to avoid materializing intermediate state to the distributed filesystem, but the initial input and final output of a job is still usually HDFS.
|
||||
|
||||
The two main problems that distributed batch processing frameworks need to solve are:
|
||||
|
||||
***Partitioning***
|
||||
|
||||
In MapReduce, mappers are partitioned according to input file blocks. The out‐ put of mappers is repartitioned, sorted, and merged into a configurable number of reducer partitions. The purpose of this process is to bring all the related data— e.g., all the records with the same key—together in the same place.
|
||||
|
||||
Post-MapReduce dataflow engines try to avoid sorting unless it is required, but they otherwise take a broadly similar approach to partitioning.
|
||||
|
||||
***Fault tolerance***
|
||||
|
||||
MapReduce frequently writes to disk, which makes it easy to recover from an individual failed task without restarting the entire job but slows down execution in the failure-free case. Dataflow engines perform less materialization of inter‐ mediate state and keep more in memory, which means that they need to recom‐ pute more data if a node fails. Deterministic operators reduce the amount of data that needs to be recomputed.
|
||||
|
||||
|
||||
|
||||
We discussed several join algorithms for MapReduce, most of which are also inter‐ nally used in MPP databases and dataflow engines. They also provide a good illustra‐ tion of how partitioned algorithms work:
|
||||
|
||||
***Sort-merge joins***
|
||||
|
||||
Each of the inputs being joined goes through a mapper that extracts the join key. By partitioning, sorting, and merging, all the records with the same key end up going to the same call of the reducer. This function can then output the joined records.
|
||||
|
||||
***Broadcast hash joins***
|
||||
|
||||
One of the two join inputs is small, so it is not partitioned and it can be entirely loaded into a hash table. Thus, you can start a mapper for each partition of the large join input, load the hash table for the small input into each mapper, and then scan over the large input one record at a time, querying the hash table for each record.
|
||||
|
||||
***Partitioned hash joins***
|
||||
|
||||
If the two join inputs are partitioned in the same way (using the same key, same hash function, and same number of partitions), then the hash table approach can be used independently for each partition.
|
||||
|
||||
|
||||
|
||||
Distributed batch processing engines have a deliberately restricted programming model: callback functions (such as mappers and reducers) are assumed to be stateless and to have no externally visible side effects besides their designated output. This restriction allows the framework to hide some of the hard distributed systems prob‐ lems behind its abstraction: in the face of crashes and network issues, tasks can be retried safely, and the output from any failed tasks is discarded. If several tasks for a partition succeed, only one of them actually makes its output visible.
|
||||
|
||||
Thanks to the framework, your code in a batch processing job does not need to worry about implementing fault-tolerance mechanisms: the framework can guarantee that the final output of a job is the same as if no faults had occurred, even though in real‐ ity various tasks perhaps had to be retried. These reliable semantics are much stron‐ ger than what you usually have in online services that handle user requests and that write to databases as a side effect of processing a request.
|
||||
|
||||
The distinguishing feature of a batch processing job is that it reads some input data and produces some output data, without modifying the input—in other words, the output is derived from the input. Crucially, the input data is *bounded*: it has a known, fixed size (for example, it consists of a set of log files at some point in time, or a snap‐ shot of a database’s contents). Because it is bounded, a job knows when it has finished reading the entire input, and so a job eventually completes when it is done.
|
||||
|
||||
In the next chapter, we will turn to stream processing, in which the input is *unboun‐ ded*—that is, you still have a job, but its inputs are never-ending streams of data. In this case, a job is never complete, because at any time there may still be more work coming in. We shall see that stream and batch processing are similar in some respects, but the assumption of unbounded streams also changes a lot about how we build systems.
|
||||
|
||||
|
||||
|
||||
## References
|
||||
--------------------
|
||||
|
||||
1. Jeffrey Dean and Sanjay Ghemawat: “[MapReduce: Simplified Data Processing on Large Clusters](http://research.google.com/archive/mapreduce.html),” at *6th USENIX Symposium on Operating System Design and Implementation* (OSDI), December 2004.
|
||||
|
||||
1. Joel Spolsky: “[The Perils of JavaSchools](http://www.joelonsoftware.com/articles/ThePerilsofJavaSchools.html),” *joelonsoftware.com*, December 25, 2005.
|
||||
|
||||
1. Shivnath Babu and Herodotos Herodotou: “[Massively Parallel Databases and MapReduce Systems](http://research.microsoft.com/pubs/206464/db-mr-survey-final.pdf),” *Foundations and Trends in Databases*, volume 5, number 1, pages 1–104, November 2013. [doi:10.1561/1900000036](http://dx.doi.org/10.1561/1900000036)
|
||||
|
||||
1. David J. DeWitt and Michael Stonebraker: “[MapReduce: A Major Step Backwards](https://homes.cs.washington.edu/~billhowe/mapreduce_a_major_step_backwards.html),” originally published at *databasecolumn.vertica.com*, January 17, 2008.
|
||||
|
||||
1. Henry Robinson: “[The Elephant Was a Trojan Horse: On the Death of Map-Reduce at Google](http://the-paper-trail.org/blog/the-elephant-was-a-trojan-horse-on-the-death-of-map-reduce-at-google/),”
|
||||
*the-paper-trail.org*, June 25, 2014.
|
||||
|
||||
1. “[The Hollerith Machine](https://www.census.gov/history/www/innovations/technology/the_hollerith_tabulator.html),” United States Census Bureau, *census.gov*.
|
||||
|
||||
1. “[IBM 82, 83, and 84 Sorters Reference Manual](http://www.textfiles.com/bitsavers/pdf/ibm/punchedCard/Sorter/A24-1034-1_82-83-84_sorters.pdf),” Edition A24-1034-1, International Business
|
||||
Machines Corporation, July 1962.
|
||||
|
||||
1. Adam Drake: “[Command-Line Tools Can Be 235x Faster than Your Hadoop Cluster](http://aadrake.com/command-line-tools-can-be-235x-faster-than-your-hadoop-cluster.html),” *aadrake.com*, January 25, 2014.
|
||||
|
||||
1. “[GNU Coreutils 8.23 Documentation](http://www.gnu.org/software/coreutils/manual/html_node/index.html),” Free Software Foundation, Inc., 2014.
|
||||
|
||||
1. Martin Kleppmann: “[Kafka, Samza, and the Unix Philosophy of Distributed Data](http://martin.kleppmann.com/2015/08/05/kafka-samza-unix-philosophy-distributed-data.html),” *martin.kleppmann.com*, August 5, 2015.
|
||||
|
||||
1. Doug McIlroy:[Internal Bell Labs memo](http://cm.bell-labs.com/cm/cs/who/dmr/mdmpipe.pdf), October 1964. Cited in: Dennis M. Richie: “[Advice from Doug McIlroy](https://www.bell-labs.com/usr/dmr/www/mdmpipe.html),” *cm.bell-labs.com*.
|
||||
|
||||
1. M. D. McIlroy, E. N. Pinson, and B. A. Tague: “[UNIX Time-Sharing System: Foreword](https://archive.org/details/bstj57-6-1899),” *The Bell System Technical Journal*, volume 57, number 6, pages 1899–1904, July 1978.
|
||||
|
||||
1. Eric S. Raymond: <a href="http://www.catb.org/~esr/writings/taoup/html/">*The Art of UNIX Programming*</a>. Addison-Wesley, 2003. ISBN: 978-0-13-142901-7
|
||||
|
||||
1. Ronald Duncan: “[Text File Formats – ASCII Delimited Text – Not CSV or TAB Delimited Text](https://ronaldduncan.wordpress.com/2009/10/31/text-file-formats-ascii-delimited-text-not-csv-or-tab-delimited-text/),”
|
||||
*ronaldduncan.wordpress.com*, October 31, 2009.
|
||||
|
||||
1. Alan Kay: “[Is 'Software Engineering' an Oxymoron?](http://tinlizzie.org/~takashi/IsSoftwareEngineeringAnOxymoron.pdf),” *tinlizzie.org*.
|
||||
|
||||
1. Martin Fowler: “[InversionOfControl](http://martinfowler.com/bliki/InversionOfControl.html),” *martinfowler.com*, June 26, 2005.
|
||||
|
||||
1. Daniel J. Bernstein: “[Two File Descriptors for Sockets](http://cr.yp.to/tcpip/twofd.html),” *cr.yp.to*.
|
||||
|
||||
1. Rob Pike and Dennis M. Ritchie: “[The Styx Architecture for Distributed Systems](http://doc.cat-v.org/inferno/4th_edition/styx),” *Bell Labs Technical Journal*, volume 4, number 2, pages 146–152, April 1999.
|
||||
|
||||
1. Sanjay Ghemawat, Howard Gobioff, and Shun-Tak Leung: “[The Google File System](http://research.google.com/archive/gfs-sosp2003.pdf),” at *19th ACM Symposium on Operating Systems Principles* (SOSP), October 2003. [doi:10.1145/945445.945450](http://dx.doi.org/10.1145/945445.945450)
|
||||
|
||||
1. Michael Ovsiannikov, Silvius Rus, Damian Reeves, et al.: “[The Quantcast File System](http://db.disi.unitn.eu/pages/VLDBProgram/pdf/industry/p808-ovsiannikov.pdf),” *Proceedings of the VLDB Endowment*, volume 6, number 11, pages 1092–1101, August 2013. [doi:10.14778/2536222.2536234](http://dx.doi.org/10.14778/2536222.2536234)
|
||||
|
||||
1. “[OpenStack Swift 2.6.1 Developer Documentation](http://docs.openstack.org/developer/swift/),” OpenStack Foundation, *docs.openstack.org*, March 2016.
|
||||
|
||||
1. Zhe Zhang, Andrew Wang, Kai Zheng, et al.: “[Introduction to HDFS Erasure Coding in Apache Hadoop](http://blog.cloudera.com/blog/2015/09/introduction-to-hdfs-erasure-coding-in-apache-hadoop/),” *blog.cloudera.com*, September 23, 2015.
|
||||
|
||||
1. Peter Cnudde: “[Hadoop Turns 10](http://yahoohadoop.tumblr.com/post/138739227316/hadoop-turns-10),” *yahoohadoop.tumblr.com*, February 5, 2016.
|
||||
|
||||
1. Eric Baldeschwieler: “[Thinking About the HDFS vs. Other Storage Technologies](http://hortonworks.com/blog/thinking-about-the-hdfs-vs-other-storage-technologies/),” *hortonworks.com*, July 25, 2012.
|
||||
|
||||
1. Brendan Gregg: “[Manta: Unix Meets Map Reduce](http://dtrace.org/blogs/brendan/2013/06/25/manta-unix-meets-map-reduce/),” *dtrace.org*, June 25, 2013.
|
||||
|
||||
1. Tom White: *Hadoop: The Definitive Guide*, 4th edition. O'Reilly Media, 2015. ISBN: 978-1-491-90163-2
|
||||
|
||||
1. Jim N. Gray: “[Distributed Computing Economics](http://arxiv.org/pdf/cs/0403019.pdf),” Microsoft Research Tech Report MSR-TR-2003-24, March 2003.
|
||||
|
||||
1. Márton Trencséni: “[Luigi vs Airflow vs Pinball](http://bytepawn.com/luigi-airflow-pinball.html),” *bytepawn.com*, February 6, 2016.
|
||||
|
||||
1. Roshan Sumbaly, Jay Kreps, and Sam Shah: “[The 'Big Data' Ecosystem at LinkedIn](http://www.slideshare.net/s_shah/the-big-data-ecosystem-at-linkedin-23512853),” at *ACM International Conference on Management of Data (SIGMOD)*, July 2013. [doi:10.1145/2463676.2463707](http://dx.doi.org/10.1145/2463676.2463707)
|
||||
|
||||
1. Alan F. Gates, Olga Natkovich, Shubham Chopra, et al.: “[Building a High-Level Dataflow System on Top of Map-Reduce: The Pig Experience](http://www.vldb.org/pvldb/2/vldb09-1074.pdf),” at *35th International Conference on Very Large Data Bases* (VLDB), August 2009.
|
||||
|
||||
1. Ashish Thusoo, Joydeep Sen Sarma, Namit Jain, et al.: “[Hive – A Petabyte Scale Data Warehouse Using Hadoop](http://i.stanford.edu/~ragho/hive-icde2010.pdf),” at *26th IEEE International Conference on Data Engineering* (ICDE), March 2010. [doi:10.1109/ICDE.2010.5447738](http://dx.doi.org/10.1109/ICDE.2010.5447738)
|
||||
|
||||
1. “[Cascading 3.0 User Guide](http://docs.cascading.org/cascading/3.0/userguide/),” Concurrent, Inc., *docs.cascading.org*, January 2016.
|
||||
|
||||
1. “[Apache Crunch User Guide](https://crunch.apache.org/user-guide.html),” Apache Software Foundation, *crunch.apache.org*.
|
||||
|
||||
1. Craig Chambers, Ashish Raniwala, Frances Perry, et al.: “[FlumeJava: Easy, Efficient Data-Parallel Pipelines](https://research.google.com/pubs/archive/35650.pdf),” at *31st ACM SIGPLAN Conference on Programming Language Design and Implementation* (PLDI), June 2010. [doi:10.1145/1806596.1806638](http://dx.doi.org/10.1145/1806596.1806638)
|
||||
|
||||
1. Jay Kreps: “[Why Local State is a Fundamental Primitive in Stream Processing](https://www.oreilly.com/ideas/why-local-state-is-a-fundamental-primitive-in-stream-processing),” *oreilly.com*, July 31, 2014.
|
||||
|
||||
1. Martin Kleppmann: “[Rethinking Caching in Web Apps](http://martin.kleppmann.com/2012/10/01/rethinking-caching-in-web-apps.html),” *martin.kleppmann.com*, October 1, 2012.
|
||||
|
||||
1. Mark Grover, Ted Malaska, Jonathan Seidman, and Gwen Shapira: *[Hadoop Application Architectures](http://shop.oreilly.com/product/0636920033196.do)*. O'Reilly Media, 2015. ISBN: 978-1-491-90004-8
|
||||
|
||||
1. Philippe Ajoux, Nathan Bronson, Sanjeev Kumar, et al.: “[Challenges to Adopting Stronger Consistency at Scale](https://www.usenix.org/system/files/conference/hotos15/hotos15-paper-ajoux.pdf),” at *15th USENIX Workshop on Hot Topics in Operating Systems* (HotOS), May 2015.
|
||||
|
||||
1. Sriranjan Manjunath: “[Skewed Join](https://wiki.apache.org/pig/PigSkewedJoinSpec),” *wiki.apache.org*, 2009.
|
||||
|
||||
1. David J. DeWitt, Jeffrey F. Naughton, Donovan A.Schneider, and S. Seshadri: “[Practical Skew Handling in Parallel Joins](http://www.vldb.org/conf/1992/P027.PDF),” at *18th International Conference on Very Large Data Bases* (VLDB), August 1992.
|
||||
|
||||
1. Marcel Kornacker, Alexander Behm, Victor Bittorf, et al.: “[Impala: A Modern, Open-Source SQL Engine for Hadoop](http://pandis.net/resources/cidr15impala.pdf),” at *7th Biennial Conference on Innovative Data Systems Research* (CIDR), January 2015.
|
||||
|
||||
1. Matthieu Monsch: “[Open-Sourcing PalDB, a Lightweight Companion for Storing Side Data](https://engineering.linkedin.com/blog/2015/10/open-sourcing-paldb--a-lightweight-companion-for-storing-side-da),” *engineering.linkedin.com*, October 26, 2015.
|
||||
|
||||
1. Daniel Peng and Frank Dabek: “[Large-Scale Incremental Processing Using Distributed Transactions and Notifications](https://www.usenix.org/legacy/event/osdi10/tech/full_papers/Peng.pdf),” at *9th USENIX conference on Operating Systems Design and Implementation* (OSDI), October 2010.
|
||||
|
||||
1. “["Cloudera Search User Guide,"](http://www.cloudera.com/documentation/cdh/5-1-x/Search/Cloudera-Search-User-Guide/Cloudera-Search-User-Guide.html) Cloudera, Inc., September 2015.
|
||||
|
||||
1. Lili Wu, Sam Shah, Sean Choi, et al.:
|
||||
“[The Browsemaps: Collaborative Filtering at LinkedIn](http://ls13-www.cs.uni-dortmund.de/homepage/rsweb2014/papers/rsweb2014_submission_3.pdf),” at *6th Workshop on Recommender Systems and the Social Web* (RSWeb), October 2014.
|
||||
|
||||
1. Roshan Sumbaly, Jay Kreps, Lei Gao, et al.: “[Serving Large-Scale Batch Computed Data with Project Voldemort](http://static.usenix.org/events/fast12/tech/full_papers/Sumbaly.pdf),” at *10th USENIX Conference on File and Storage Technologies* (FAST), February 2012.
|
||||
|
||||
1. Varun Sharma: “[Open-Sourcing Terrapin: A Serving System for Batch Generated Data](https://engineering.pinterest.com/blog/open-sourcing-terrapin-serving-system-batch-generated-data-0),” *engineering.pinterest.com*, September 14, 2015.
|
||||
|
||||
1. Nathan Marz: “[ElephantDB](http://www.slideshare.net/nathanmarz/elephantdb),” *slideshare.net*, May 30, 2011.
|
||||
|
||||
1. Jean-Daniel (JD) Cryans: “[How-to: Use HBase Bulk Loading, and Why](http://blog.cloudera.com/blog/2013/09/how-to-use-hbase-bulk-loading-and-why/),” *blog.cloudera.com*, September 27, 2013.
|
||||
|
||||
1. Nathan Marz: “[How to Beat the CAP Theorem](http://nathanmarz.com/blog/how-to-beat-the-cap-theorem.html),” *nathanmarz.com*, October 13, 2011.
|
||||
|
||||
1. Molly Bartlett Dishman and Martin Fowler: “[Agile Architecture](http://conferences.oreilly.com/software-architecture/sa2015/public/schedule/detail/40388),” at *O'Reilly Software Architecture Conference*, March 2015.
|
||||
|
||||
1. David J. DeWitt and Jim N. Gray: “[Parallel Database Systems: The Future of High Performance Database Systems](http://www.cs.cmu.edu/~pavlo/courses/fall2013/static/papers/dewittgray92.pdf),” *Communications of the ACM*, volume 35, number 6, pages 85–98, June 1992. [doi:10.1145/129888.129894](http://dx.doi.org/10.1145/129888.129894)
|
||||
|
||||
1. Jay Kreps: “[But the multi-tenancy thing is actually really really hard](https://twitter.com/jaykreps/status/528235702480142336),” tweetstorm, *twitter.com*, October 31, 2014.
|
||||
|
||||
1. Jeffrey Cohen, Brian Dolan, Mark Dunlap, et al.: “[MAD Skills: New Analysis Practices for Big Data](http://www.vldb.org/pvldb/2/vldb09-219.pdf),” *Proceedings of the VLDB Endowment*, volume 2, number 2, pages 1481–1492, August 2009. [doi:10.14778/1687553.1687576](http://dx.doi.org/10.14778/1687553.1687576)
|
||||
|
||||
1. Ignacio Terrizzano, Peter Schwarz, Mary Roth, and John E. Colino: “[Data Wrangling: The Challenging Journey from the Wild to the Lake](http://cidrdb.org/cidr2015/Papers/CIDR15_Paper2.pdf),” at *7th Biennial Conference on Innovative Data Systems Research* (CIDR), January 2015.
|
||||
|
||||
1. Paige Roberts: “[To Schema on Read or to Schema on Write, That Is the Hadoop Data Lake Question](http://adaptivesystemsinc.com/blog/to-schema-on-read-or-to-schema-on-write-that-is-the-hadoop-data-lake-question/),” *adaptivesystemsinc.com*, July 2, 2015.
|
||||
|
||||
1. Bobby Johnson and Joseph Adler: “[The Sushi Principle: Raw Data Is Better](https://vimeo.com/123985284),” at *Strata+Hadoop World*, February 2015.
|
||||
|
||||
1. Vinod Kumar Vavilapalli, Arun C. Murthy, Chris Douglas, et al.: “[Apache Hadoop YARN: Yet Another Resource Negotiator](http://www.socc2013.org/home/program/a5-vavilapalli.pdf),” at *4th ACM Symposium on Cloud Computing* (SoCC), October 2013. [doi:10.1145/2523616.2523633](http://dx.doi.org/10.1145/2523616.2523633)
|
||||
|
||||
1. Abhishek Verma, Luis Pedrosa, Madhukar Korupolu, et al.: “[Large-Scale Cluster Management at Google with Borg](http://research.google.com/pubs/pub43438.html),” at *10th European Conference on Computer Systems* (EuroSys), April 2015. [doi:10.1145/2741948.2741964](http://dx.doi.org/10.1145/2741948.2741964)
|
||||
|
||||
1. Malte Schwarzkopf: “[The Evolution of Cluster Scheduler Architectures](http://www.firmament.io/blog/scheduler-architectures.html),” *firmament.io*, March 9, 2016.
|
||||
|
||||
1. Matei Zaharia, Mosharaf Chowdhury, Tathagata Das, et al.: “[Resilient Distributed Datasets: A Fault-Tolerant Abstraction for In-Memory Cluster Computing](https://www.usenix.org/system/files/conference/nsdi12/nsdi12-final138.pdf),” at *9th USENIX Symposium on Networked Systems Design and Implementation* (NSDI), April 2012.
|
||||
|
||||
1. Holden Karau, Andy Konwinski, Patrick Wendell, and Matei Zaharia: *Learning Spark*. O'Reilly Media, 2015. ISBN: 978-1-449-35904-1
|
||||
|
||||
1. Bikas Saha and Hitesh Shah: “[Apache Tez: Accelerating Hadoop Query Processing](http://www.slideshare.net/Hadoop_Summit/w-1205phall1saha),” at *Hadoop Summit*, June 2014.
|
||||
|
||||
1. Bikas Saha, Hitesh Shah, Siddharth Seth, et al.: “[Apache Tez: A Unifying Framework for Modeling and Building Data Processing Applications](http://home.cse.ust.hk/~weiwa/teaching/Fall15-COMP6611B/reading_list/Tez.pdf),” at *ACM International Conference on Management of Data* (SIGMOD), June 2015. [doi:10.1145/2723372.2742790](http://dx.doi.org/10.1145/2723372.2742790)
|
||||
|
||||
1. Kostas Tzoumas: “[Apache Flink: API, Runtime, and Project Roadmap](http://www.slideshare.net/KostasTzoumas/apache-flink-api-runtime-and-project-roadmap),” *slideshare.net*, January 14, 2015.
|
||||
|
||||
1. Alexander Alexandrov, Rico Bergmann, Stephan Ewen, et al.: “[The Stratosphere Platform for Big Data Analytics](https://ssc.io/pdf/2014-VLDBJ_Stratosphere_Overview.pdf),” *The VLDB Journal*, volume 23, number 6, pages 939–964, May 2014. [doi:10.1007/s00778-014-0357-y](http://dx.doi.org/10.1007/s00778-014-0357-y)
|
||||
|
||||
1. Michael Isard, Mihai Budiu, Yuan Yu, et al.: “[Dryad: Distributed Data-Parallel Programs from Sequential Building Blocks](http://research.microsoft.com/en-us/projects/dryad/eurosys07.pdf),” at *European Conference on Computer Systems* (EuroSys), March 2007. [doi:10.1145/1272996.1273005](http://dx.doi.org/10.1145/1272996.1273005)
|
||||
|
||||
1. Daniel Warneke and Odej Kao: “[Nephele: Efficient Parallel Data Processing in the Cloud](https://stratosphere2.dima.tu-berlin.de/assets/papers/Nephele_09.pdf),” at *2nd Workshop on Many-Task Computing on Grids and Supercomputers* (MTAGS), November 2009. [doi:10.1145/1646468.1646476](http://dx.doi.org/10.1145/1646468.1646476)
|
||||
|
||||
1. Lawrence Page, Sergey Brin, Rajeev Motwani, and Terry Winograd: ["The PageRank"](http://ilpubs.stanford.edu:8090/422/)
|
||||
|
||||
1. Leslie G. Valiant: “[A Bridging Model for Parallel Computation](http://dl.acm.org/citation.cfm?id=79181),” *Communications of the ACM*, volume 33, number 8, pages 103–111, August 1990. [doi:10.1145/79173.79181](http://dx.doi.org/10.1145/79173.79181)
|
||||
|
||||
1. Stephan Ewen, Kostas Tzoumas, Moritz Kaufmann, and Volker Markl: “[Spinning Fast Iterative Data Flows](http://vldb.org/pvldb/vol5/p1268_stephanewen_vldb2012.pdf),” *Proceedings of the VLDB Endowment*, volume 5, number 11, pages 1268-1279, July 2012. [doi:10.14778/2350229.2350245](http://dx.doi.org/10.14778/2350229.2350245)
|
||||
|
||||
1. Grzegorz Malewicz, Matthew H.Austern, Aart J. C. Bik, et al.: “[Pregel: A System for Large-Scale Graph Processing](https://kowshik.github.io/JPregel/pregel_paper.pdf),” at *ACM International Conference on Management of Data* (SIGMOD), June 2010. [doi:10.1145/1807167.1807184](http://dx.doi.org/10.1145/1807167.1807184)
|
||||
|
||||
1. Frank McSherry, Michael Isard, and Derek G. Murray: “[Scalability! But at What COST?](http://www.frankmcsherry.org/assets/COST.pdf),” at *15th USENIX Workshop on Hot Topics in Operating Systems* (HotOS), May 2015.
|
||||
|
||||
1. Ionel Gog, Malte Schwarzkopf, Natacha Crooks, et al.: “[Musketeer: All for One, One for All in Data Processing Systems](http://www.cl.cam.ac.uk/research/srg/netos/camsas/pubs/eurosys15-musketeer.pdf),” at *10th European Conference on Computer Systems* (EuroSys), April 2015. [doi:10.1145/2741948.2741968](http://dx.doi.org/10.1145/2741948.2741968)
|
||||
|
||||
1. Aapo Kyrola, Guy Blelloch, and Carlos Guestrin: “[GraphChi: Large-Scale Graph Computation on Just a PC](https://www.usenix.org/system/files/conference/osdi12/osdi12-final-126.pdf),” at *10th USENIX Symposium on Operating Systems Design and Implementation* (OSDI), October 2012.
|
||||
|
||||
1. Andrew Lenharth, Donald Nguyen, and Keshav Pingali: “[Parallel Graph Analytics](http://cacm.acm.org/magazines/2016/5/201591-parallel-graph-analytics/fulltext),” *Communications of the ACM*, volume 59, number 5, pages 78–87, May [doi:10.1145/2901919](http://dx.doi.org/10.1145/2901919)
|
||||
|
||||
1. Fabian Hüske: “[Peeking into Apache Flink's Engine Room](http://flink.apache.org/news/2015/03/13/peeking-into-Apache-Flinks-Engine-Room.html),” *flink.apache.org*, March 13, 2015.
|
||||
|
||||
1. Mostafa Mokhtar: “[Hive 0.14 Cost Based Optimizer (CBO) Technical Overview](http://hortonworks.com/blog/hive-0-14-cost-based-optimizer-cbo-technical-overview/),” *hortonworks.com*, March 2, 2015.
|
||||
|
||||
1. Michael Armbrust, Reynold S Xin, Cheng Lian, et al.: “[Spark SQL: Relational Data Processing in Spark](http://people.csail.mit.edu/matei/papers/2015/sigmod_spark_sql.pdf),” at *ACM International Conference on Management of Data* (SIGMOD), June 2015. [doi:10.1145/2723372.2742797](http://dx.doi.org/10.1145/2723372.2742797)
|
||||
|
||||
1. Daniel Blazevski: “[Planting Quadtrees for Apache Flink](http://insightdataengineering.com/blog/flink-knn/),” *insightdataengineering.com*, March 25, 2016.
|
||||
|
||||
1. Tom White: “[Genome Analysis Toolkit: Now Using Apache Spark for Data Processing](http://blog.cloudera.com/blog/2016/04/genome-analysis-toolkit-now-using-apache-spark-for-data-processing/),” *blog.cloudera.com*, April 6, 2016.
|
271
en-us/ch11.md
Normal file
271
en-us/ch11.md
Normal file
@ -0,0 +1,271 @@
|
||||
# 11. Stream Processing
|
||||
|
||||

|
||||
|
||||
> *A complex system that works is invariably found to have evolved from a simple system that works. The inverse proposition also appears to be true: A complex system designed from scratch never works and cannot be made to work.*
|
||||
>
|
||||
> — John Gall, *Systemantics* (1975)
|
||||
|
||||
---------------
|
||||
|
||||
In [Chapter 10](ch10.md) we discussed batch processing—techniques that read a set of files as input and produce a new set of output files. The output is a form of *derived data*; that is, a dataset that can be recreated by running the batch process again if necessary. We saw how this simple but powerful idea can be used to create search indexes, recom‐ mendation systems, analytics, and more.
|
||||
|
||||
However, one big assumption remained throughout [Chapter 10](ch10.md): namely, that the input is bounded—i.e., of a known and finite size—so the batch process knows when it has finished reading its input. For example, the sorting operation that is central to MapReduce must read its entire input before it can start producing output: it could happen that the very last input record is the one with the lowest key, and thus needs to be the very first output record, so starting the output early is not an option.
|
||||
|
||||
In reality, a lot of data is unbounded because it arrives gradually over time: your users produced data yesterday and today, and they will continue to produce more data tomorrow. Unless you go out of business, this process never ends, and so the dataset is never “complete” in any meaningful way [1]. Thus, batch processors must artifi‐ cially divide the data into chunks of fixed duration: for example, processing a day’s worth of data at the end of every day, or processing an hour’s worth of data at the end of every hour.
|
||||
|
||||
The problem with daily batch processes is that changes in the input are only reflected in the output a day later, which is too slow for many impatient users. To reduce the delay, we can run the processing more frequently—say, processing a second’s worth of data at the end of every second—or even continuously, abandoning the fixed time slices entirely and simply processing every event as it happens. That is the idea behind *stream processing*.
|
||||
|
||||
In general, a “stream” refers to data that is incrementally made available over time. The concept appears in many places: in the stdin and stdout of Unix, programming languages (lazy lists) [2], filesystem APIs (such as Java’s `FileInputStream`), TCP con‐ nections, delivering audio and video over the internet, and so on.
|
||||
|
||||
In this chapter we will look at *event streams* as a data management mechanism: the unbounded, incrementally processed counterpart to the batch data we saw in the last chapter. We will first discuss how streams are represented, stored, and transmit‐ ted over a network. In “[Databases and Streams](#databases-and-streams)” we will investigate the relationship between streams and databases. And finally, in “[Processing Streams](#processing-streams)” we will explore approaches and tools for processing those streams continually, and ways that they can be used to build applications.
|
||||
|
||||
|
||||
## ……
|
||||
|
||||
|
||||
|
||||
## Summary
|
||||
|
||||
In this chapter we have discussed event streams, what purposes they serve, and how to process them. In some ways, stream processing is very much like the batch pro‐ cessing we discussed in [Chapter 10](ch10.md), but done continuously on unbounded (neverending) streams rather than on a fixed-size input. From this perspective, message brokers and event logs serve as the streaming equivalent of a filesystem.
|
||||
|
||||
We spent some time comparing two types of message brokers:
|
||||
|
||||
***AMQP/JMS-style message broker***
|
||||
|
||||
The broker assigns individual messages to consumers, and consumers acknowl‐ edge individual messages when they have been successfully processed. Messages are deleted from the broker once they have been acknowledged. This approach is appropriate as an asynchronous form of RPC (see also “[Message-Passing Data‐ flow]()”), for example in a task queue, where the exact order of mes‐ sage processing is not important and where there is no need to go back and read old messages again after they have been processed.
|
||||
|
||||
***Log-based message broker***
|
||||
|
||||
The broker assigns all messages in a partition to the same consumer node, and always delivers messages in the same order. Parallelism is achieved through par‐ titioning, and consumers track their progress by checkpointing the offset of the last message they have processed. The broker retains messages on disk, so it is possible to jump back and reread old messages if necessary.
|
||||
|
||||
The log-based approach has similarities to the replication logs found in databases (see [Chapter 5](ch5.md)) and log-structured storage engines (see [Chapter 3](ch3.md)). We saw that this approach is especially appropriate for stream processing systems that consume input streams and generate derived state or derived output streams.
|
||||
|
||||
In terms of where streams come from, we discussed several possibilities: user activity events, sensors providing periodic readings, and data feeds (e.g., market data in finance) are naturally represented as streams. We saw that it can also be useful to think of the writes to a database as a stream: we can capture the changelog—i.e., the history of all changes made to a database—either implicitly through change data cap‐ ture or explicitly through event sourcing. Log compaction allows the stream to retain a full copy of the contents of a database.
|
||||
|
||||
Representing databases as streams opens up powerful opportunities for integrating systems. You can keep derived data systems such as search indexes, caches, and analytics systems continually up to date by consuming the log of changes and applying them to the derived system. You can even build fresh views onto existing data by starting from scratch and consuming the log of changes from the beginning all the way to the present.
|
||||
|
||||
The facilities for maintaining state as streams and replaying messages are also the basis for the techniques that enable stream joins and fault tolerance in various stream processing frameworks. We discussed several purposes of stream processing, including searching for event patterns (complex event processing), computing windowed aggregations (stream analytics), and keeping derived data systems up to date (materialized views).
|
||||
|
||||
We then discussed the difficulties of reasoning about time in a stream processor, including the distinction between processing time and event timestamps, and the problem of dealing with straggler events that arrive after you thought your window was complete.
|
||||
|
||||
We distinguished three types of joins that may appear in stream processes:
|
||||
|
||||
***Stream-stream joins***
|
||||
|
||||
Both input streams consist of activity events, and the join operator searches for related events that occur within some window of time. For example, it may match two actions taken by the same user within 30 minutes of each other. The two join inputs may in fact be the same stream (a *self-join*) if you want to find related events within that one stream.
|
||||
|
||||
***Stream-table joins***
|
||||
|
||||
One input stream consists of activity events, while the other is a database change‐ log. The changelog keeps a local copy of the database up to date. For each activity event, the join operator queries the database and outputs an enriched activity event.
|
||||
|
||||
***Table-table joins***
|
||||
|
||||
Both input streams are database changelogs. In this case, every change on one side is joined with the latest state of the other side. The result is a stream of changes to the materialized view of the join between the two tables.
|
||||
|
||||
Finally, we discussed techniques for achieving fault tolerance and exactly-once semantics in a stream processor. As with batch processing, we need to discard the partial output of any failed tasks. However, since a stream process is long-running and produces output continuously, we can’t simply discard all output. Instead, a finer-grained recovery mechanism can be used, based on microbatching, checkpoint‐ ing, transactions, or idempotent writes.
|
||||
|
||||
|
||||
|
||||
|
||||
## References
|
||||
--------------------
|
||||
|
||||
1. Tyler Akidau, Robert Bradshaw, Craig Chambers, et al.: “[The Dataflow Model: A Practical Approach to Balancing Correctness, Latency, and Cost in Massive-Scale, Unbounded, Out-of-Order Data Processing](http://www.vldb.org/pvldb/vol8/p1792-Akidau.pdf),”
|
||||
*Proceedings of the VLDB Endowment*, volume 8, number 12, pages 1792–1803, August 2015. [doi:10.14778/2824032.2824076](http://dx.doi.org/10.14778/2824032.2824076)
|
||||
|
||||
1. Harold Abelson, Gerald Jay Sussman, and Julie Sussman: <a href="https://mitpress.mit.edu/sicp/">*Structure and Interpretation of Computer Programs*</a>, 2nd edition. MIT Press, 1996. ISBN: 978-0-262-51087-5, available online at *mitpress.mit.edu*
|
||||
|
||||
1. Patrick Th. Eugster, Pascal A. Felber, Rachid Guerraoui, and Anne-Marie Kermarrec: “[The Many Faces of Publish/Subscribe](http://www.cs.ru.nl/~pieter/oss/manyfaces.pdf),” *ACM Computing Surveys*, volume 35, number 2, pages 114–131, June 2003.
|
||||
[doi:10.1145/857076.857078](http://dx.doi.org/10.1145/857076.857078)
|
||||
|
||||
1. Joseph M. Hellerstein and Michael Stonebraker: <a href="http://redbook.cs.berkeley.edu/">*Readings in Database Systems*</a>, 4th edition. MIT Press, 2005. ISBN: 978-0-262-69314-1, available online at *redbook.cs.berkeley.edu*
|
||||
|
||||
1. Don Carney, Uğur Çetintemel, Mitch Cherniack, et al.: “[Monitoring Streams – A New Class of Data Management Applications](http://www.vldb.org/conf/2002/S07P02.pdf),” at *28th International Conference on Very Large Data Bases* (VLDB), August 2002.
|
||||
|
||||
1. Matthew Sackman: “[Pushing Back](http://www.lshift.net/blog/2016/05/05/pushing-back/),” *lshift.net*, May 5, 2016. Vicent Martí: “[Brubeck, a statsd-Compatible Metrics Aggregator](http://githubengineering.com/brubeck/),” *githubengineering.com*, June 15, 2015. Seth Lowenberger: “[MoldUDP64 Protocol Specification V 1.00](http://www.nasdaqtrader.com/content/technicalsupport/specifications/dataproducts/moldudp64.pdf),” *nasdaqtrader.com*, July 2009.
|
||||
|
||||
1. Pieter Hintjens: <a href="http://zguide.zeromq.org/page:all">*ZeroMQ – The Guide*</a>. O'Reilly Media, 2013. ISBN: 978-1-449-33404-8
|
||||
|
||||
1. Ian Malpass: “[Measure Anything, Measure Everything](https://codeascraft.com/2011/02/15/measure-anything-measure-everything/),” *codeascraft.com*, February 15, 2011.
|
||||
|
||||
1. Dieter Plaetinck: “[25 Graphite, Grafana and statsd Gotchas](https://blog.raintank.io/25-graphite-grafana-and-statsd-gotchas/),” *blog.raintank.io*, March 3, 2016.
|
||||
|
||||
1. Jeff Lindsay: “[Web Hooks to Revolutionize the Web](http://progrium.com/blog/2007/05/03/web-hooks-to-revolutionize-the-web/),” *progrium.com*, May 3, 2007.
|
||||
|
||||
1. Jim N. Gray: “[Queues Are Databases](http://research.microsoft.com/pubs/69641/tr-95-56.pdf),” Microsoft Research Technical Report MSR-TR-95-56, December 1995.
|
||||
|
||||
1. Mark Hapner, Rich Burridge, Rahul Sharma, et al.: “[JSR-343 Java Message Service (JMS) 2.0 Specification](https://jcp.org/en/jsr/detail?id=343),” *jms-spec.java.net*, March 2013.
|
||||
|
||||
1. Sanjay Aiyagari, Matthew Arrott, Mark Atwell, et al.: “[AMQP: Advanced Message Queuing Protocol Specification](http://www.rabbitmq.com/resources/specs/amqp0-9-1.pdf),” Version 0-9-1, November 2008.
|
||||
|
||||
1. “[Google Cloud Pub/Sub: A Google-Scale Messaging Service](https://cloud.google.com/pubsub/architecture),” *cloud.google.com*, 2016.
|
||||
|
||||
1. “[Apache Kafka 0.9 Documentation](http://kafka.apache.org/documentation.html),” *kafka.apache.org*, November 2015.
|
||||
|
||||
1. Jay Kreps, Neha Narkhede, and Jun Rao: “[Kafka: A Distributed Messaging System for Log Processing](http://www.longyu23.com/doc/Kafka.pdf),” at *6th International Workshop on Networking Meets Databases* (NetDB), June 2011.
|
||||
|
||||
1. “[Amazon Kinesis Streams Developer Guide](http://docs.aws.amazon.com/streams/latest/dev/introduction.html),” *docs.aws.amazon.com*, April 2016.
|
||||
|
||||
1. Leigh Stewart and Sijie Guo: “[Building DistributedLog: Twitter’s High-Performance Replicated Log Service](https://blog.twitter.com/2015/building-distributedlog-twitter-s-high-performance-replicated-log-service),” *blog.twitter.com*, September 16, 2015.
|
||||
|
||||
1. “[DistributedLog Documentation](http://distributedlog.incubator.apache.org/docs/latest/),” Twitter, Inc., *distributedlog.io*, May 2016. Jay Kreps:
|
||||
|
||||
“[Benchmarking Apache Kafka: 2 Million Writes Per Second (On Three Cheap Machines)](https://engineering.linkedin.com/kafka/benchmarking-apache-kafka-2-million-writes-second-three-cheap-machines),” *engineering.linkedin.com*, April 27, 2014.
|
||||
|
||||
1. Kartik Paramasivam: “[How We’re Improving and Advancing Kafka at LinkedIn](https://engineering.linkedin.com/apache-kafka/how-we_re-improving-and-advancing-kafka-linkedin),” *engineering.linkedin.com*, September 2, 2015.
|
||||
|
||||
1. Jay Kreps: “[The Log: What Every Software Engineer Should Know About Real-Time Data's Unifying Abstraction](http://engineering.linkedin.com/distributed-systems/log-what-every-software-engineer-should-know-about-real-time-datas-unifying),” *engineering.linkedin.com*, December 16, 2013.
|
||||
|
||||
1. Shirshanka Das, Chavdar Botev, Kapil Surlaker, et al.: “[All Aboard the Databus!](http://www.socc2012.org/s18-das.pdf),” at *3rd ACM Symposium on Cloud Computing* (SoCC), October 2012.
|
||||
|
||||
1. Yogeshwer Sharma, Philippe Ajoux, Petchean Ang, et al.: “[Wormhole: Reliable Pub-Sub to Support Geo-Replicated Internet Services](https://www.usenix.org/system/files/conference/nsdi15/nsdi15-paper-sharma.pdf),” at *12th USENIX Symposium on Networked Systems Design and Implementation* (NSDI), May 2015.
|
||||
|
||||
1. P. P. S. Narayan: “[Sherpa Update](http://web.archive.org/web/20160801221400/https://developer.yahoo.com/blogs/ydn/sherpa-7992.html),” *developer.yahoo.com*, June 8, .
|
||||
|
||||
1. Martin Kleppmann: “[Bottled Water: Real-Time Integration of PostgreSQL and Kafka](http://martin.kleppmann.com/2015/04/23/bottled-water-real-time-postgresql-kafka.html),” *martin.kleppmann.com*, April 23, 2015.
|
||||
|
||||
1. Ben Osheroff: “[Introducing Maxwell, a mysql-to-kafka Binlog Processor](https://developer.zendesk.com/blog/introducing-maxwell-a-mysql-to-kafka-binlog-processor),” *developer.zendesk.com*, August 20, 2015.
|
||||
|
||||
1. Randall Hauch: “[Debezium 0.2.1 Released](http://debezium.io/blog/2016/06/10/Debezium-0/),” *debezium.io*, June 10, 2016.
|
||||
|
||||
1. Prem Santosh Udaya Shankar: “[Streaming MySQL Tables in Real-Time to Kafka](https://engineeringblog.yelp.com/2016/08/streaming-mysql-tables-in-real-time-to-kafka.html),” *engineeringblog.yelp.com*, August 1, 2016.
|
||||
|
||||
1. “[Mongoriver](https://github.com/stripe/mongoriver),” Stripe, Inc., *github.com*, September 2014.
|
||||
|
||||
1. Dan Harvey: “[Change Data Capture with Mongo + Kafka](http://www.slideshare.net/danharvey/change-data-capture-with-mongodb-and-kafka),” at *Hadoop Users Group UK*, August 2015.
|
||||
|
||||
1. “[Oracle GoldenGate 12c: Real-Time Access to Real-Time Information](http://www.oracle.com/us/products/middleware/data-integration/oracle-goldengate-realtime-access-2031152.pdf),” Oracle White Paper, March 2015.
|
||||
|
||||
1. “[Oracle GoldenGate Fundamentals: How Oracle GoldenGate Works](https://www.youtube.com/watch?v=6H9NibIiPQE),” Oracle Corporation, *youtube.com*, November 2012.
|
||||
|
||||
1. Slava Akhmechet: “[Advancing the Realtime Web](http://rethinkdb.com/blog/realtime-web/),” *rethinkdb.com*, January 27, 2015.
|
||||
|
||||
1. “[Firebase Realtime Database Documentation](https://firebase.google.com/docs/database/),” Google, Inc., *firebase.google.com*, May 2016.
|
||||
|
||||
1. “[Apache CouchDB 1.6 Documentation](http://docs.couchdb.org/en/latest/),” *docs.couchdb.org*, 2014.
|
||||
|
||||
1. Matt DeBergalis: “[Meteor 0.7.0: Scalable Database Queries Using MongoDB Oplog Instead of Poll-and-Diff](http://info.meteor.com/blog/meteor-070-scalable-database-queries-using-mongodb-oplog-instead-of-poll-and-diff),” *info.meteor.com*, December 17, 2013.
|
||||
|
||||
1. “[Chapter 15. Importing and Exporting Live Data](https://docs.voltdb.com/UsingVoltDB/ChapExport.php),” VoltDB 6.4 User Manual, *docs.voltdb.com*, June 2016.
|
||||
|
||||
1. Neha Narkhede: “[Announcing Kafka Connect: Building Large-Scale Low-Latency Data Pipelines](http://www.confluent.io/blog/announcing-kafka-connect-building-large-scale-low-latency-data-pipelines),” *confluent.io*, February 18, 2016.
|
||||
|
||||
1. Greg Young: “[CQRS and Event Sourcing](https://www.youtube.com/watch?v=JHGkaShoyNs),” at *Code on the Beach*, August 2014.
|
||||
|
||||
1. Martin Fowler: “[Event Sourcing](http://martinfowler.com/eaaDev/EventSourcing.html),” *martinfowler.com*, December 12, 2005.
|
||||
|
||||
1. Vaughn Vernon: <a href="https://vaughnvernon.co/?page_id=168">*Implementing Domain-Driven Design*</a>. Addison-Wesley Professional, 2013. ISBN: 978-0-321-83457-7
|
||||
|
||||
1. H. V. Jagadish, Inderpal Singh Mumick, and Abraham Silberschatz: “[View Maintenance Issues for the Chronicle Data Model](http://www.mathcs.emory.edu/~cheung/papers/StreamDB/Histogram/1995-Jagadish-Histo.pdf),” at *14th ACM SIGACT-SIGMOD-SIGART Symposium on Principles of Database Systems* (PODS), May 1995. [doi:10.1145/212433.220201](http://dx.doi.org/10.1145/212433.220201)
|
||||
|
||||
1. “[Event Store 3.5.0 Documentation](http://docs.geteventstore.com/),” Event Store LLP, *docs.geteventstore.com*, February 2016.
|
||||
|
||||
1. Martin Kleppmann: <a href="http://www.oreilly.com/data/free/stream-processing.csp">*Making Sense of Stream Processing*</a>. Report, O'Reilly Media, May 2016.
|
||||
|
||||
1. Sander Mak: “[Event-Sourced Architectures with Akka](http://www.slideshare.net/SanderMak/eventsourced-architectures-with-akka),” at *JavaOne*, September 2014.
|
||||
|
||||
1. Julian Hyde: [personal communication](https://twitter.com/julianhyde/status/743374145006641153), June 2016.
|
||||
|
||||
1. Ashish Gupta and Inderpal Singh Mumick: *Materialized Views: Techniques, Implementations, and Applications*. MIT Press, 1999. ISBN: 978-0-262-57122-7
|
||||
|
||||
1. Timothy Griffin and Leonid Libkin: “[Incremental Maintenance of Views with Duplicates](http://homepages.inf.ed.ac.uk/libkin/papers/sigmod95.pdf),” at *ACM International Conference on Management of Data* (SIGMOD), May 1995. [doi:10.1145/223784.223849](http://dx.doi.org/10.1145/223784.223849)
|
||||
|
||||
1. Pat Helland: “[Immutability Changes Everything](http://www.cidrdb.org/cidr2015/Papers/CIDR15_Paper16.pdf),” at *7th Biennial Conference on Innovative Data Systems Research* (CIDR), January 2015.
|
||||
|
||||
1. Martin Kleppmann: “[Accounting for Computer Scientists](http://martin.kleppmann.com/2011/03/07/accounting-for-computer-scientists.html),” *martin.kleppmann.com*, March 7, 2011.
|
||||
|
||||
1. Pat Helland: “[Accountants Don't Use Erasers](https://blogs.msdn.microsoft.com/pathelland/2007/06/14/accountants-dont-use-erasers/),” *blogs.msdn.com*, June 14, 2007.
|
||||
|
||||
1. Fangjin Yang: “[Dogfooding with Druid, Samza, and Kafka: Metametrics at Metamarkets](https://metamarkets.com/2015/dogfooding-with-druid-samza-and-kafka-metametrics-at-metamarkets/),” *metamarkets.com*, June 3, 2015.
|
||||
|
||||
1. Gavin Li, Jianqiu Lv, and Hang Qi: “[Pistachio: Co-Locate the Data and Compute for Fastest Cloud Compute](http://yahoohadoop.tumblr.com/post/116365275781/pistachio-co-locate-the-data-and-compute-for),” *yahoohadoop.tumblr.com*, April 13, 2015.
|
||||
|
||||
1. Kartik Paramasivam: “[Stream Processing Hard Problems – Part 1: Killing Lambda](https://engineering.linkedin.com/blog/2016/06/stream-processing-hard-problems-part-1-killing-lambda),” *engineering.linkedin.com*, June 27, 2016.
|
||||
|
||||
1. Martin Fowler: “[CQRS](http://martinfowler.com/bliki/CQRS.html),” *martinfowler.com*, July 14, 2011.
|
||||
|
||||
1. Greg Young: “[CQRS Documents](https://cqrs.files.wordpress.com/2010/11/cqrs_documents.pdf),” *cqrs.files.wordpress.com*, November 2010.
|
||||
|
||||
1. Baron Schwartz: “[Immutability, MVCC, and Garbage Collection](http://www.xaprb.com/blog/2013/12/28/immutability-mvcc-and-garbage-collection/),” *xaprb.com*, December 28, 2013.
|
||||
|
||||
1. Daniel Eloff, Slava Akhmechet, Jay Kreps, et al.: ["Re: Turning the Database Inside-out with Apache Samza](https://news.ycombinator.com/item?id=9145197)," *Hacker News discussion, news.ycombinator.com*, March 4, 2015.
|
||||
|
||||
1. “[Datomic Development Resources: Excision](http://docs.datomic.com/excision.html),” Cognitect, Inc., *docs.datomic.com*.
|
||||
|
||||
1. “[Fossil Documentation: Deleting Content from Fossil](http://fossil-scm.org/index.html/doc/trunk/www/shunning.wiki),” *fossil-scm.org*, 2016.
|
||||
|
||||
1. Jay Kreps: “[The irony of distributed systems is that data loss is really easy but deleting data is surprisingly hard,](https://twitter.com/jaykreps/status/582580836425330688)” *twitter.com*, March 30, 2015.
|
||||
|
||||
1. David C. Luckham: “[What’s the Difference Between ESP and CEP?](http://www.complexevents.com/2006/08/01/what%E2%80%99s-the-difference-between-esp-and-cep/),” *complexevents.com*, August 1, 2006.
|
||||
|
||||
1. Srinath Perera: “[How Is Stream Processing and Complex Event Processing (CEP) Different?](https://www.quora.com/How-is-stream-processing-and-complex-event-processing-CEP-different),” *quora.com*, December 3, 2015.
|
||||
|
||||
1. Arvind Arasu, Shivnath Babu, and Jennifer Widom: “[The CQL Continuous Query Language: Semantic Foundations and Query Execution](http://research.microsoft.com/pubs/77607/cql.pdf),” *The VLDB Journal*, volume 15, number 2, pages 121–142, June 2006. [doi:10.1007/s00778-004-0147-z](http://dx.doi.org/10.1007/s00778-004-0147-z)
|
||||
|
||||
1. Julian Hyde: “[Data in Flight: How Streaming SQL Technology Can Help Solve the Web 2.0 Data Crunch](http://queue.acm.org/detail.cfm?id=1667562),” *ACM Queue*, volume 7, number 11, December 2009. [doi:10.1145/1661785.1667562](http://dx.doi.org/10.1145/1661785.1667562)
|
||||
|
||||
1. “[Esper Reference, Version 5.4.0](http://www.espertech.com/esper/release-5.4.0/esper-reference/html_single/index.html),” EsperTech, Inc., *espertech.com*, April 2016.
|
||||
|
||||
1. Zubair Nabi, Eric Bouillet, Andrew Bainbridge, and Chris Thomas:
|
||||
“[Of Streams and Storms](https://developer.ibm.com/streamsdev/wp-content/uploads/sites/15/2014/04/Streams-and-Storm-April-2014-Final.pdf),” IBM technical report, *developer.ibm.com*, April 2014.
|
||||
|
||||
1. Milinda Pathirage, Julian Hyde, Yi Pan, and Beth Plale: “[SamzaSQL: Scalable Fast Data Management with Streaming SQL](https://github.com/milinda/samzasql-hpbdc2016/blob/master/samzasql-hpbdc2016.pdf),” at *IEEE International Workshop on High-Performance Big Data Computing* (HPBDC), May 2016. [doi:10.1109/IPDPSW.2016.141](http://dx.doi.org/10.1109/IPDPSW.2016.141)
|
||||
|
||||
1. Philippe Flajolet, Éric Fusy, Olivier Gandouet, and Frédéric Meunier: “[HyperLo⁠g​Log: The Analysis of a Near-Optimal Cardinality Estimation Algorithm](http://algo.inria.fr/flajolet/Publications/FlFuGaMe07.pdf),” at *Conference on Analysis of Algorithms* (AofA), June 2007.
|
||||
|
||||
1. Jay Kreps: “[Questioning the Lambda Architecture](https://www.oreilly.com/ideas/questioning-the-lambda-architecture),” *oreilly.com*, July 2, 2014.
|
||||
|
||||
1. Ian Hellström: “[An Overview of Apache Streaming Technologies](https://databaseline.wordpress.com/2016/03/12/an-overview-of-apache-streaming-technologies/),” *databaseline.wordpress.com*, March 12, 2016.
|
||||
|
||||
1. Jay Kreps: “[Why Local State Is a Fundamental Primitive in Stream Processing](https://www.oreilly.com/ideas/why-local-state-is-a-fundamental-primitive-in-stream-processing),” *oreilly.com*, July 31, 2014.
|
||||
|
||||
1. Shay Banon: “[Percolator](https://www.elastic.co/blog/percolator),” *elastic.co*, February 8, 2011.
|
||||
|
||||
1. Alan Woodward and Martin Kleppmann: “[Real-Time Full-Text Search with Luwak and Samza](http://martin.kleppmann.com/2015/04/13/real-time-full-text-search-luwak-samza.html),” *martin.kleppmann.com*, April 13, 2015.
|
||||
|
||||
1. “[Apache Storm 1.0.1 Documentation](https://storm.apache.org/releases/1.0.1/index.html),” *storm.apache.org*, May 2016.
|
||||
|
||||
1. Tyler Akidau: “[The World Beyond Batch: Streaming 102](https://www.oreilly.com/ideas/the-world-beyond-batch-streaming-102),” *oreilly.com*, January 20, 2016.
|
||||
|
||||
1. Stephan Ewen: “[Streaming Analytics with Apache Flink](http://www.confluent.io/kafka-summit-2016-systems-advanced-streaming-analytics-with-apache-flink-and-apache-kafka),” at *Kafka Summit*, April 2016.
|
||||
|
||||
1. Tyler Akidau, Alex Balikov, Kaya Bekiroğlu, et al.: “[MillWheel: Fault-Tolerant Stream Processing at Internet Scale](http://research.google.com/pubs/pub41378.html),” at *39th International Conference on Very Large Data Bases* (VLDB), August 2013.
|
||||
|
||||
1. Alex Dean: “[Improving Snowplow's Understanding of Time](http://snowplowanalytics.com/blog/2015/09/15/improving-snowplows-understanding-of-time/),” *snowplowanalytics.com*, September 15, 2015.
|
||||
|
||||
1. “[Windowing (Azure Stream Analytics)](https://msdn.microsoft.com/en-us/library/azure/dn835019.aspx),” Microsoft Azure Reference, *msdn.microsoft.com*, April 2016.
|
||||
|
||||
1. “[State Management](http://samza.apache.org/learn/documentation/0.10/container/state-management.html),” Apache Samza 0.10 Documentation, *samza.apache.org*, December 2015.
|
||||
|
||||
1. Rajagopal Ananthanarayanan, Venkatesh Basker, Sumit Das, et al.: “[Photon: Fault-Tolerant and Scalable Joining of Continuous Data Streams](http://research.google.com/pubs/pub41318.html),” at *ACM International Conference on Management of Data* (SIGMOD), June 2013. [doi:10.1145/2463676.2465272](http://dx.doi.org/10.1145/2463676.2465272)
|
||||
|
||||
1. Martin Kleppmann: “[Samza Newsfeed Demo](https://github.com/ept/newsfeed),” *github.com*, September 2014.
|
||||
|
||||
1. Ben Kirwin: “[Doing the Impossible: Exactly-Once Messaging Patterns in Kafka](http://ben.kirw.in/2014/11/28/kafka-patterns/),” *ben.kirw.in*, November 28, 2014.
|
||||
|
||||
1. Pat Helland: “[Data on the Outside Versus Data on the Inside](http://cidrdb.org/cidr2005/papers/P12.pdf),” at *2nd Biennial Conference on Innovative Data Systems Research* (CIDR), January 2005.
|
||||
|
||||
1. Ralph Kimball and Margy Ross: *The Data Warehouse Toolkit: The Definitive Guide to Dimensional Modeling*, 3rd edition. John Wiley & Sons, 2013. ISBN: 978-1-118-53080-1
|
||||
|
||||
1. Viktor Klang: “[I'm coining the phrase 'effectively-once' for message processing with at-least-once + idempotent operations](https://twitter.com/viktorklang/status/789036133434978304),” *twitter.com*, October 20, 2016.
|
||||
|
||||
1. Matei Zaharia, Tathagata Das, Haoyuan Li, et al.: “[Discretized Streams: An Efficient and Fault-Tolerant Model for Stream Processing on Large Clusters](https://www.usenix.org/system/files/conference/hotcloud12/hotcloud12-final28.pdf),” at *4th USENIX Conference in Hot Topics in Cloud Computing* (HotCloud), June 2012.
|
||||
|
||||
1. Kostas Tzoumas, Stephan Ewen, and Robert Metzger: “[High-Throughput, Low-Latency, and Exactly-Once Stream Processing with Apache Flink](http://data-artisans.com/high-throughput-low-latency-and-exactly-once-stream-processing-with-apache-flink/),” *data-artisans.com*, August 5, 2015.
|
||||
|
||||
1. Paris Carbone, Gyula Fóra, Stephan Ewen, et al.: “[Lightweight Asynchronous Snapshots for Distributed Dataflows](http://arxiv.org/abs/1506.08603),” arXiv:1506.08603 [cs.DC], June 29, 2015.
|
||||
|
||||
1. Ryan Betts and John Hugg: <a href="http://www.oreilly.com/data/free/fast-data-smart-and-at-scale.csp">*Fast Data: Smart and at Scale*</a>. Report, O'Reilly Media, October 2015.
|
||||
|
||||
1. Flavio Junqueira: “[Making Sense of Exactly-Once Semantics](http://conferences.oreilly.com/strata/hadoop-big-data-eu/public/schedule/detail/49690),” at *Strata+Hadoop World London*, June 2016.
|
||||
|
||||
1. Jason Gustafson, Flavio Junqueira, Apurva Mehta, Sriram Subramanian, and Guozhang Wang: “[KIP-98 – Exactly Once Delivery and Transactional Messaging](https://cwiki.apache.org/confluence/display/KAFKA/KIP-98+-+Exactly+Once+Delivery+and+Transactional+Messaging),” *cwiki.apache.org*, November 2016.
|
||||
|
||||
1. Pat Helland: “[Idempotence Is Not a Medical Condition](http://citeseerx.ist.psu.edu/viewdoc/download?doi=10.1.1.401.1539&rep=rep1&type=pdf),” *Communications of the ACM*, volume 55, number 5, page 56, May 2012. [doi:10.1145/2160718.2160734](http://dx.doi.org/10.1145/2160718.2160734)
|
||||
|
||||
1. Jay Kreps: “[Re: Trying to Achieve Deterministic Behavior on Recovery/Rewind](http://mail-archives.apache.org/mod_mbox/samza-dev/201409.mbox/%3CCAOeJiJg%2Bc7Ei%3DgzCuOz30DD3G5Hm9yFY%3DUJ6SafdNUFbvRgorg%40mail.gmail.com%3E),” email to *samza-dev* mailing list, September 9, 2014.
|
||||
|
||||
1. E. N. (Mootaz) Elnozahy, Lorenzo Alvisi, Yi-Min Wang, and David B. Johnson: “[A Survey of Rollback-Recovery Protocols in Message-Passing Systems](http://www.cs.utexas.edu/~lorenzo/papers/SurveyFinal.pdf),” *ACM Computing Surveys*, volume 34, number 3, pages 375–408, September 2002. [doi:10.1145/568522.568525](http://dx.doi.org/10.1145/568522.568525)
|
||||
|
||||
1. Adam Warski: “[Kafka Streams – How Does It Fit the Stream Processing Landscape?](https://softwaremill.com/kafka-streams-how-does-it-fit-stream-landscape/),” *softwaremill.com*, June 1, 2016.
|
281
en-us/ch12.md
Normal file
281
en-us/ch12.md
Normal file
@ -0,0 +1,281 @@
|
||||
# 12. The Future of Data Systems
|
||||
|
||||

|
||||
|
||||
> *If a thing be ordained to another as to its end, its last end cannot consist in the preservation of its being. Hence a captain does not intend as a last end, the preservation of the ship entrusted to him, since a ship is ordained to something else as its end, viz. to navigation.*
|
||||
>
|
||||
> *(Often quoted as: If the highest aim of a captain was the preserve his ship, he would keep it in port forever.)*
|
||||
>
|
||||
> — St. Thomas Aquinas, *Summa Theologica* (1265–1274)
|
||||
|
||||
---------------
|
||||
|
||||
So far, this book has been mostly about describing things as they *are* at present. In this final chapter, we will shift our perspective toward the future and discuss how things *should be*: I will propose some ideas and approaches that, I believe, may funda‐ mentally improve the ways we design and build applications.
|
||||
|
||||
Opinions and speculation about the future are of course subjective, and so I will use the first person in this chapter when writing about my personal opinions. You are welcome to disagree with them and form your own opinions, but I hope that the ideas in this chapter will at least be a starting point for a productive discussion and bring some clarity to concepts that are often confused.
|
||||
|
||||
The goal of this book was outlined in [Chapter 1](ch1.md): to explore how to create applications and systems that are *reliable*, *scalable*, and *maintainable*. These themes have run through all of the chapters: for example, we discussed many fault-tolerance algo‐ rithms that help improve reliability, partitioning to improve scalability, and mecha‐ nisms for evolution and abstraction that improve maintainability. In this chapter we will bring all of these ideas together, and build on them to envisage the future. Our goal is to discover how to design applications that are better than the ones of today— robust, correct, evolvable, and ultimately beneficial to humanity.
|
||||
|
||||
|
||||
## ……
|
||||
|
||||
|
||||
|
||||
## Summary
|
||||
|
||||
In this chapter we discussed new approaches to designing data systems, and I included my personal opinions and speculations about the future. We started with the observation that there is no one single tool that can efficiently serve all possible use cases, and so applications necessarily need to compose several different pieces of software to accomplish their goals. We discussed how to solve this *data integration* problem by using batch processing and event streams to let data changes flow between different systems.
|
||||
|
||||
In this approach, certain systems are designated as systems of record, and other data is derived from them through transformations. In this way we can maintain indexes, materialized views, machine learning models, statistical summaries, and more. By making these derivations and transformations asynchronous and loosely coupled, a problem in one area is prevented from spreading to unrelated parts of the system, increasing the robustness and fault-tolerance of the system as a whole.
|
||||
|
||||
Expressing dataflows as transformations from one dataset to another also helps evolve applications: if you want to change one of the processing steps, for example to change the structure of an index or cache, you can just rerun the new transformation code on the whole input dataset in order to rederive the output. Similarly, if some‐ thing goes wrong, you can fix the code and reprocess the data in order to recover.
|
||||
|
||||
These processes are quite similar to what databases already do internally, so we recast the idea of dataflow applications as *unbundling* the components of a database, and building an application by composing these loosely coupled components.
|
||||
|
||||
Derived state can be updated by observing changes in the underlying data. Moreover, the derived state itself can further be observed by downstream consumers. We can even take this dataflow all the way through to the end-user device that is displaying the data, and thus build user interfaces that dynamically update to reflect data changes and continue to work offline.
|
||||
|
||||
Next, we discussed how to ensure that all of this processing remains correct in the presence of faults. We saw that strong integrity guarantees can be implemented scala‐ bly with asynchronous event processing, by using end-to-end operation identifiers to make operations idempotent and by checking constraints asynchronously. Clients can either wait until the check has passed, or go ahead without waiting but risk hav‐ ing to apologize about a constraint violation. This approach is much more scalable and robust than the traditional approach of using distributed transactions, and fits with how many business processes work in practice.
|
||||
|
||||
By structuring applications around dataflow and checking constraints asynchro‐ nously, we can avoid most coordination and create systems that maintain integrity but still perform well, even in geographically distributed scenarios and in the pres‐ ence of faults. We then talked a little about using audits to verify the integrity of data and detect corruption.
|
||||
|
||||
Finally, we took a step back and examined some ethical aspects of building data- intensive applications. We saw that although data can be used to do good, it can also do significant harm: making justifying decisions that seriously affect people’s lives and are difficult to appeal against, leading to discrimination and exploitation, nor‐ malizing surveillance, and exposing intimate information. We also run the risk of data breaches, and we may find that a well-intentioned use of data has unintended consequences.
|
||||
|
||||
As software and data are having such a large impact on the world, we engineers must remember that we carry a responsibility to work toward the kind of world that we want to live in: a world that treats people with humanity and respect. I hope that we can work together toward that goal.
|
||||
|
||||
## References
|
||||
--------------------
|
||||
|
||||
1. Rachid Belaid: “[Postgres Full-Text Search is Good Enough!](http://rachbelaid.com/postgres-full-text-search-is-good-enough/),” *rachbelaid.com*, July 13, 2015.
|
||||
|
||||
1. Philippe Ajoux, Nathan Bronson, Sanjeev Kumar, et al.: “[Challenges to Adopting Stronger Consistency at Scale](https://www.usenix.org/system/files/conference/hotos15/hotos15-paper-ajoux.pdf),” at *15th USENIX Workshop on Hot Topics in Operating Systems* (HotOS), May 2015.
|
||||
|
||||
1. Pat Helland and Dave Campbell: “[Building on Quicksand](https://database.cs.wisc.edu/cidr/cidr2009/Paper_133.pdf),” at *4th Biennial Conference on Innovative Data Systems Research* (CIDR), January 2009.
|
||||
|
||||
1. Jessica Kerr: “[Provenance and Causality in Distributed Systems](http://blog.jessitron.com/2016/09/provenance-and-causality-in-distributed.html),” *blog.jessitron.com*, September 25, 2016.
|
||||
|
||||
1. Kostas Tzoumas: “[Batch Is a Special Case of Streaming](http://data-artisans.com/batch-is-a-special-case-of-streaming/),” *data-artisans.com*, September 15, 2015.
|
||||
|
||||
1. Shinji Kim and Robert Blafford: “[Stream Windowing Performance Analysis: Concord and Spark Streaming](http://concord.io/posts/windowing_performance_analysis_w_spark_streaming),” *concord.io*, July 6, 2016.
|
||||
|
||||
1. Jay Kreps: “[The Log: What Every Software Engineer Should Know About Real-Time Data's Unifying Abstraction](http://engineering.linkedin.com/distributed-systems/log-what-every-software-engineer-should-know-about-real-time-datas-unifying),” *engineering.linkedin.com*, December 16, 2013.
|
||||
|
||||
1. Pat Helland: “[Life Beyond Distributed Transactions: An Apostate’s Opinion](http://www-db.cs.wisc.edu/cidr/cidr2007/papers/cidr07p15.pdf),” at *3rd Biennial Conference on Innovative Data Systems Research* (CIDR), January 2007.
|
||||
|
||||
1. “[Great Western Railway (1835–1948)](https://www.networkrail.co.uk/VirtualArchive/great-western/),” Network Rail Virtual Archive, *networkrail.co.uk*.
|
||||
|
||||
1. Jacqueline Xu: “[Online Migrations at Scale](https://stripe.com/blog/online-migrations),” *stripe.com*, February 2, 2017.
|
||||
|
||||
1. Molly Bartlett Dishman and Martin Fowler: “[Agile Architecture](http://conferences.oreilly.com/software-architecture/sa2015/public/schedule/detail/40388),” at *O'Reilly Software Architecture Conference*, March 2015.
|
||||
|
||||
1. Nathan Marz and James Warren: <a href="https://www.manning.com/books/big-data">*Big Data: Principles and Best Practices of Scalable Real-Time Data Systems*</a>. Manning, 2015. ISBN: 978-1-617-29034-3
|
||||
|
||||
1. Oscar Boykin, Sam Ritchie, Ian O'Connell, and Jimmy Lin: “[Summingbird: A Framework for Integrating Batch and Online MapReduce Computations](http://www.vldb.org/pvldb/vol7/p1441-boykin.pdf),” at *40th International Conference on Very Large Data Bases* (VLDB), September 2014.
|
||||
|
||||
1. Jay Kreps: “[Questioning the Lambda Architecture](https://www.oreilly.com/ideas/questioning-the-lambda-architecture),” *oreilly.com*, July 2, 2014.
|
||||
|
||||
1. Raul Castro Fernandez, Peter Pietzuch, Jay Kreps, et al.: “[Liquid: Unifying Nearline and Offline Big Data Integration](http://www.cidrdb.org/cidr2015/Papers/CIDR15_Paper25u.pdf),” at *7th Biennial Conference on Innovative Data Systems Research* (CIDR), January 2015.
|
||||
|
||||
1. Dennis M. Ritchie and Ken Thompson: “[The UNIX Time-Sharing System](http://www.cs.virginia.edu/~zaher/classes/CS656/p365-ritchie.pdf),” *Communications of the ACM*, volume 17, number 7, pages 365–375, July 1974. [doi:10.1145/361011.361061](http://dx.doi.org/10.1145/361011.361061)
|
||||
|
||||
1. Eric A. Brewer and Joseph M. Hellerstein: “[CS262a: Advanced Topics in Computer Systems](http://people.eecs.berkeley.edu/~brewer/cs262/systemr.html),” lecture notes, University of California, Berkeley, *cs.berkeley.edu*, August 2011.
|
||||
|
||||
1. Michael Stonebraker: “[The Case for Polystores](http://wp.sigmod.org/?p=1629),” *wp.sigmod.org*, July 13, 2015.
|
||||
|
||||
1. Jennie Duggan, Aaron J. Elmore, Michael Stonebraker, et al.: “[The BigDAWG Polystore System](http://dspace.mit.edu/openaccess-disseminate/1721.1/100936),” *ACM SIGMOD Record*, volume 44, number 2, pages 11–16, June 2015. [doi:10.1145/2814710.2814713](http://dx.doi.org/10.1145/2814710.2814713)
|
||||
|
||||
1. Patrycja Dybka: “[Foreign Data Wrappers for PostgreSQL](http://www.vertabelo.com/blog/technical-articles/foreign-data-wrappers-for-postgresql),” *vertabelo.com*, March 24, 2015.
|
||||
|
||||
1. David B. Lomet, Alan Fekete, Gerhard Weikum, and Mike Zwilling: “[Unbundling Transaction Services in the Cloud](https://www.microsoft.com/en-us/research/publication/unbundling-transaction-services-in-the-cloud/),” at *4th Biennial Conference on Innovative Data Systems Research* (CIDR), January 2009.
|
||||
|
||||
1. Martin Kleppmann and Jay Kreps: “[Kafka, Samza and the Unix Philosophy of Distributed Data](http://martin.kleppmann.com/papers/kafka-debull15.pdf),” *IEEE Data Engineering Bulletin*, volume 38, number 4, pages 4–14, December 2015.
|
||||
|
||||
1. John Hugg: “[Winning Now and in the Future: Where VoltDB Shines](https://voltdb.com/blog/winning-now-and-future-where-voltdb-shines),” *voltdb.com*, March 23, 2016.
|
||||
|
||||
1. Frank McSherry, Derek G. Murray, Rebecca Isaacs, and Michael Isard: “[Differential Dataflow](http://cidrdb.org/cidr2013/Papers/CIDR13_Paper111.pdf),”
|
||||
at *6th Biennial Conference on Innovative Data Systems Research* (CIDR), January 2013.
|
||||
|
||||
1. Derek G Murray, Frank McSherry, Rebecca Isaacs, et al.: “[Naiad: A Timely Dataflow System](http://research.microsoft.com/pubs/201100/naiad_sosp2013.pdf),”
|
||||
at *24th ACM Symposium on Operating Systems Principles* (SOSP), pages 439–455, November 2013.
|
||||
[doi:10.1145/2517349.2522738](http://dx.doi.org/10.1145/2517349.2522738)
|
||||
|
||||
1. Gwen Shapira: “[We have a bunch of customers who are implementing ‘database inside-out’ concept and they all ask ‘is anyone else doing it? are we crazy?’](https://twitter.com/gwenshap/status/758800071110430720)” *twitter.com*, July 28, 2016.
|
||||
|
||||
1. Martin Kleppmann: “[Turning the Database Inside-out with Apache Samza,](http://martin.kleppmann.com/2015/03/04/turning-the-database-inside-out.html)” at *Strange Loop*, September 2014.
|
||||
|
||||
1. Peter Van Roy and Seif Haridi: <a href="http://www.epsa.org/forms/uploadFiles/3B6300000000.filename.booksingle.pdf">*Concepts, Techniques, and Models of Computer Programming*</a>. MIT Press, 2004. ISBN: 978-0-262-22069-9
|
||||
|
||||
1. “[Juttle Documentation](http://juttle.github.io/juttle/),” *juttle.github.io*, 2016.
|
||||
|
||||
1. Evan Czaplicki and Stephen Chong: “[Asynchronous Functional Reactive Programming for GUIs](http://people.seas.harvard.edu/~chong/pubs/pldi13-elm.pdf),” at *34th ACM SIGPLAN Conference on Programming Language Design and Implementation* (PLDI), June 2013. [doi:10.1145/2491956.2462161](http://dx.doi.org/10.1145/2491956.2462161)
|
||||
|
||||
1. Engineer Bainomugisha, Andoni Lombide Carreton, Tom van Cutsem, Stijn Mostinckx, and Wolfgang de Meuter: “[A Survey on Reactive Programming](http://soft.vub.ac.be/Publications/2012/vub-soft-tr-12-13.pdf),” *ACM Computing Surveys*, volume 45, number 4, pages 1–34, August 2013. [doi:10.1145/2501654.2501666](http://dx.doi.org/10.1145/2501654.2501666)
|
||||
|
||||
1. Peter Alvaro, Neil Conway, Joseph M. Hellerstein, and William R. Marczak: “[Consistency Analysis in Bloom: A CALM and Collected Approach](http://www.eecs.berkeley.edu/~palvaro/cidr11.pdf),” at *5th Biennial Conference on Innovative Data Systems Research*
|
||||
(CIDR), January 2011.
|
||||
|
||||
1. Felienne Hermans: “[Spreadsheets Are Code](https://vimeo.com/145492419),” at *Code Mesh*, November 2015.
|
||||
|
||||
1. Dan Bricklin and Bob Frankston: “[VisiCalc: Information from Its Creators](http://danbricklin.com/visicalc.htm),” *danbricklin.com*.
|
||||
|
||||
1. D. Sculley, Gary Holt, Daniel Golovin, et al.: “[Machine Learning: The High-Interest Credit Card of Technical Debt](http://research.google.com/pubs/pub43146.html),” at *NIPS Workshop on Software Engineering for Machine Learning* (SE4ML), December 2014.
|
||||
|
||||
1. Peter Bailis, Alan Fekete, Michael J Franklin, et al.: “[Feral Concurrency Control: An Empirical Investigation of Modern Application Integrity](http://www.bailis.org/papers/feral-sigmod2015.pdf),” at *ACM International Conference on Management of Data* (SIGMOD), June 2015. [doi:10.1145/2723372.2737784](http://dx.doi.org/10.1145/2723372.2737784)
|
||||
|
||||
1. Guy Steele: “[Re: Need for Macros (Was Re: Icon)](https://people.csail.mit.edu/gregs/ll1-discuss-archive-html/msg01134.html),” email to *ll1-discuss* mailing list, *people.csail.mit.edu*, December 24, 2001.
|
||||
|
||||
1. David Gelernter: “[Generative Communication in Linda](http://cseweb.ucsd.edu/groups/csag/html/teaching/cse291s03/Readings/p80-gelernter.pdf),” *ACM Transactions on Programming Languages and Systems* (TOPLAS), volume 7, number 1, pages 80–112, January 1985. [doi:10.1145/2363.2433](http://dx.doi.org/10.1145/2363.2433)
|
||||
|
||||
1. Patrick Th. Eugster, Pascal A. Felber, Rachid Guerraoui, and Anne-Marie Kermarrec: “[The Many Faces of Publish/Subscribe](http://www.cs.ru.nl/~pieter/oss/manyfaces.pdf),” *ACM Computing Surveys*, volume 35, number 2, pages 114–131, June 2003. [doi:10.1145/857076.857078](http://dx.doi.org/10.1145/857076.857078)
|
||||
|
||||
1. Ben Stopford: “[Microservices in a Streaming World](https://www.infoq.com/presentations/microservices-streaming),” at *QCon London*, March 2016.
|
||||
|
||||
1. Christian Posta: “[Why Microservices Should Be Event Driven: Autonomy vs Authority](http://blog.christianposta.com/microservices/why-microservices-should-be-event-driven-autonomy-vs-authority/),” *blog.christianposta.com*, May 27, 2016.
|
||||
|
||||
1. Alex Feyerke: “[Say Hello to Offline First](http://hood.ie/blog/say-hello-to-offline-first.html),” *hood.ie*, November 5, 2013.
|
||||
|
||||
1. Sebastian Burckhardt, Daan Leijen, Jonathan Protzenko, and Manuel Fähndrich: “[Global Sequence Protocol: A Robust Abstraction for Replicated Shared State](http://drops.dagstuhl.de/opus/volltexte/2015/5238/),” at *29th European Conference on Object-Oriented Programming* (ECOOP), July 2015. [doi:10.4230/LIPIcs.ECOOP.2015.568](http://dx.doi.org/10.4230/LIPIcs.ECOOP.2015.568)
|
||||
|
||||
1. Mark Soper: “[Clearing Up React Data Management Confusion with Flux, Redux, and Relay](https://medium.com/@marksoper/clearing-up-react-data-management-confusion-with-flux-redux-and-relay-aad504e63cae),” *medium.com*, December 3, 2015.
|
||||
|
||||
1. Eno Thereska, Damian Guy, Michael Noll, and Neha Narkhede: “[Unifying Stream Processing and Interactive Queries in Apache Kafka](http://www.confluent.io/blog/unifying-stream-processing-and-interactive-queries-in-apache-kafka/),” *confluent.io*, October 26, 2016.
|
||||
|
||||
1. Frank McSherry: “[Dataflow as Database](https://github.com/frankmcsherry/blog/blob/master/posts/2016-07-17.md),” *github.com*, July 17, 2016.
|
||||
|
||||
1. Peter Alvaro: “[I See What You Mean](https://www.youtube.com/watch?v=R2Aa4PivG0g),” at *Strange Loop*, September 2015.
|
||||
|
||||
1. Nathan Marz: “[Trident: A High-Level Abstraction for Realtime Computation](https://blog.twitter.com/2012/trident-a-high-level-abstraction-for-realtime-computation),” *blog.twitter.com*, August 2, 2012.
|
||||
|
||||
1. Edi Bice: “[Low Latency Web Scale Fraud Prevention with Apache Samza, Kafka and Friends](http://www.slideshare.net/edibice/extremely-low-latency-web-scale-fraud-prevention-with-apache-samza-kafka-and-friends),” at *Merchant Risk Council MRC Vegas Conference*, March 2016.
|
||||
|
||||
1. Charity Majors: “[The Accidental DBA](https://charity.wtf/2016/10/02/the-accidental-dba/),” *charity.wtf*, October 2, 2016.
|
||||
|
||||
1. Arthur J. Bernstein, Philip M. Lewis, and Shiyong Lu: “[Semantic Conditions for Correctness at Different Isolation Levels](http://db.cs.berkeley.edu/cs286/papers/isolation-icde2000.pdf),” at *16th International Conference on Data Engineering* (ICDE), February 2000. [doi:10.1109/ICDE.2000.839387](http://dx.doi.org/10.1109/ICDE.2000.839387)
|
||||
|
||||
1. Sudhir Jorwekar, Alan Fekete, Krithi Ramamritham, and S. Sudarshan: “[Automating the Detection of Snapshot Isolation Anomalies](http://www.vldb.org/conf/2007/papers/industrial/p1263-jorwekar.pdf),” at *33rd International Conference on Very Large Data Bases* (VLDB), September 2007.
|
||||
|
||||
1. Kyle Kingsbury: [Jepsen blog post series](https://aphyr.com/tags/jepsen), *aphyr.com*, 2013–2016.
|
||||
|
||||
1. Michael Jouravlev: “[Redirect After Post](http://www.theserverside.com/news/1365146/Redirect-After-Post),” *theserverside.com*, August 1, 2004.
|
||||
|
||||
1. Jerome H. Saltzer, David P. Reed, and David D. Clark: “[End-to-End Arguments in System Design](http://www.ece.drexel.edu/courses/ECE-C631-501/SalRee1984.pdf),” *ACM Transactions on Computer Systems*, volume 2, number 4, pages 277–288, November 1984. [doi:10.1145/357401.357402](http://dx.doi.org/10.1145/357401.357402)
|
||||
|
||||
1. Peter Bailis, Alan Fekete, Michael J. Franklin, et al.: “[Coordination-Avoiding Database Systems](http://arxiv.org/pdf/1402.2237.pdf),”
|
||||
*Proceedings of the VLDB Endowment*, volume 8, number 3, pages 185–196, November 2014.
|
||||
|
||||
1. Alex Yarmula: “[Strong Consistency in Manhattan](https://blog.twitter.com/2016/strong-consistency-in-manhattan),” *blog.twitter.com*, March 17, 2016.
|
||||
|
||||
1. Douglas B Terry, Marvin M Theimer, Karin Petersen, et al.: “[Managing Update Conflicts in Bayou, a Weakly Connected Replicated Storage System](http://css.csail.mit.edu/6.824/2014/papers/bayou-conflicts.pdf),” at *15th ACM Symposium on Operating Systems Principles* (SOSP), pages 172–182, December 1995. [doi:10.1145/224056.224070](http://dx.doi.org/10.1145/224056.224070)
|
||||
|
||||
1. Jim Gray: “[The Transaction Concept: Virtues and Limitations](http://research.microsoft.com/en-us/um/people/gray/papers/theTransactionConcept.pdf),” at *7th International Conference on Very Large Data Bases* (VLDB), September 1981.
|
||||
|
||||
1. Hector Garcia-Molina and Kenneth Salem: “[Sagas](http://www.cs.cornell.edu/andru/cs711/2002fa/reading/sagas.pdf),” at *ACM International Conference on Management of Data* (SIGMOD), May 1987. [doi:10.1145/38713.38742](http://dx.doi.org/10.1145/38713.38742)
|
||||
|
||||
1. Pat Helland: “[Memories, Guesses, and Apologies](http://blogs.msdn.com/b/pathelland/archive/2007/05/15/memories-guesses-and-apologies.aspx),” *blogs.msdn.com*, May 15, 2007.
|
||||
|
||||
1. Yoongu Kim, Ross Daly, Jeremie Kim, et al.: “[Flipping Bits in Memory Without Accessing Them: An Experimental Study of DRAM Disturbance Errors](https://users.ece.cmu.edu/~yoonguk/papers/kim-isca14.pdf),” at *41st Annual International Symposium on Computer Architecture* (ISCA), June 2014. [doi:10.1145/2678373.2665726](http://dx.doi.org/10.1145/2678373.2665726)
|
||||
|
||||
1. Mark Seaborn and Thomas Dullien: “[Exploiting the DRAM Rowhammer Bug to Gain Kernel Privileges](https://googleprojectzero.blogspot.co.uk/2015/03/exploiting-dram-rowhammer-bug-to-gain.html),” *googleprojectzero.blogspot.co.uk*, March 9, 2015.
|
||||
|
||||
1. Jim N. Gray and Catharine van Ingen: “[Empirical Measurements of Disk Failure Rates and Error Rates](https://www.microsoft.com/en-us/research/publication/empirical-measurements-of-disk-failure-rates-and-error-rates/),” Microsoft Research, MSR-TR-2005-166, December 2005.
|
||||
|
||||
1. Annamalai Gurusami and Daniel Price: “[Bug #73170: Duplicates in Unique Secondary Index Because of Fix of Bug#68021](http://bugs.mysql.com/bug.php?id=73170),” *bugs.mysql.com*, July 2014.
|
||||
|
||||
1. Gary Fredericks: “[Postgres Serializability Bug](https://github.com/gfredericks/pg-serializability-bug),” *github.com*, September 2015.
|
||||
|
||||
1. Xiao Chen: “[HDFS DataNode Scanners and Disk Checker Explained](http://blog.cloudera.com/blog/2016/12/hdfs-datanode-scanners-and-disk-checker-explained/),” *blog.cloudera.com*, December 20,
|
||||
2016.
|
||||
|
||||
1. Jay Kreps: “[Getting Real About Distributed System Reliability](http://blog.empathybox.com/post/19574936361/getting-real-about-distributed-system-reliability),” *blog.empathybox.com*, March 19, 2012.
|
||||
|
||||
1. Martin Fowler: “[The LMAX Architecture](http://martinfowler.com/articles/lmax.html),” *martinfowler.com*, July 12, 2011.
|
||||
|
||||
1. Sam Stokes: “[Move Fast with Confidence](http://blog.samstokes.co.uk/blog/2016/07/11/move-fast-with-confidence/),” *blog.samstokes.co.uk*, July 11, 2016.
|
||||
|
||||
1. “[Sawtooth Lake Documentation](http://intelledger.github.io/introduction.html),” Intel Corporation, *intelledger.github.io*, 2016.
|
||||
|
||||
1. Richard Gendal Brown: “[Introducing R3 Corda™: A Distributed Ledger Designed for Financial Services](https://gendal.me/2016/04/05/introducing-r3-corda-a-distributed-ledger-designed-for-financial-services/),” *gendal.me*, April 5, 2016.
|
||||
|
||||
1. Trent McConaghy, Rodolphe Marques, Andreas Müller, et al.: “[BigchainDB: A Scalable Blockchain Database](https://www.bigchaindb.com/whitepaper/bigchaindb-whitepaper.pdf),” *bigchaindb.com*, June 8, 2016.
|
||||
|
||||
1. Ralph C. Merkle: “[A Digital Signature Based on a Conventional Encryption Function](https://people.eecs.berkeley.edu/~raluca/cs261-f15/readings/merkle.pdf),” at *CRYPTO '87*, August 1987. [doi:10.1007/3-540-48184-2_32](http://dx.doi.org/10.1007/3-540-48184-2_32)
|
||||
|
||||
1. Ben Laurie: “[Certificate Transparency](http://queue.acm.org/detail.cfm?id=2668154),” *ACM Queue*, volume 12, number 8, pages 10-19, August 2014. [doi:10.1145/2668152.2668154](http://dx.doi.org/10.1145/2668152.2668154)
|
||||
|
||||
1. Mark D. Ryan: “[Enhanced Certificate Transparency and End-to-End Encrypted Mail](http://www.internetsociety.org/doc/enhanced-certificate-transparency-and-end-end-encrypted-mail),” at *Network and Distributed System Security Symposium* (NDSS), February 2014. [doi:10.14722/ndss.2014.23379](http://dx.doi.org/10.14722/ndss.2014.23379)
|
||||
|
||||
1. “<a href="http://www.acm.org/about/se-code">Software Engineering Code of Ethics and Professional
|
||||
Practice</a>,” Association for Computing Machinery, *acm.org*, 1999.
|
||||
|
||||
1. François Chollet: “[Software development is starting to involve important ethical choices](https://twitter.com/fchollet/status/792958695722201088),” *twitter.com*, October 30, 2016.
|
||||
|
||||
1. Igor Perisic: “[Making Hard Choices: The Quest for Ethics in Machine Learning](https://engineering.linkedin.com/blog/2016/11/making-hard-choices--the-quest-for-ethics-in-machine-learning),” *engineering.linkedin.com*, November 2016.
|
||||
|
||||
1. John Naughton: “[Algorithm Writers Need a Code of Conduct](https://www.theguardian.com/commentisfree/2015/dec/06/algorithm-writers-should-have-code-of-conduct),” *theguardian.com*, December 6, 2015.
|
||||
|
||||
1. Logan Kugler: “[What Happens When Big Data Blunders?](http://cacm.acm.org/magazines/2016/6/202655-what-happens-when-big-data-blunders/fulltext),” *Communications of the ACM*, volume 59, number 6, pages 15–16, June 2016. [doi:10.1145/2911975](http://dx.doi.org/10.1145/2911975)
|
||||
|
||||
1. Bill Davidow: “[Welcome to Algorithmic Prison](http://www.theatlantic.com/technology/archive/2014/02/welcome-to-algorithmic-prison/283985/),” *theatlantic.com*, February 20, 2014.
|
||||
|
||||
1. Don Peck: “[They're Watching You at Work](http://www.theatlantic.com/magazine/archive/2013/12/theyre-watching-you-at-work/354681/),” *theatlantic.com*, December 2013.
|
||||
|
||||
1. Leigh Alexander: “[Is an Algorithm Any Less Racist Than a Human?](https://www.theguardian.com/technology/2016/aug/03/algorithm-racist-human-employers-work)” *theguardian.com*, August 3, 2016.
|
||||
|
||||
1. Jesse Emspak: “[How a Machine Learns Prejudice](https://www.scientificamerican.com/article/how-a-machine-learns-prejudice/),” *scientificamerican.com*, December 29, 2016.
|
||||
|
||||
1. Maciej Cegłowski: “[The Moral Economy of Tech](http://idlewords.com/talks/sase_panel.htm),” *idlewords.com*, June 2016.
|
||||
|
||||
1. Cathy O'Neil: <a href="https://weaponsofmathdestructionbook.com/">*Weapons of Math Destruction: How Big Data Increases Inequality and Threatens Democracy*</a>. Crown Publishing, 2016. ISBN: 978-0-553-41881-1
|
||||
|
||||
1. Julia Angwin: “[Make Algorithms Accountable](http://www.nytimes.com/2016/08/01/opinion/make-algorithms-accountable.html),” *nytimes.com*, August 1, 2016.
|
||||
|
||||
1. Bryce Goodman and Seth Flaxman: “[European Union Regulations on Algorithmic Decision-Making and a ‘Right to Explanation’](https://arxiv.org/abs/1606.08813),” *arXiv:1606.08813*, August 31, 2016.
|
||||
|
||||
1. “[A Review of the Data Broker Industry: Collection, Use, and Sale of Consumer Data for Marketing Purposes](https://www.commerce.senate.gov/public/index.cfm/reports?ID=57C428EC-8F20-44EE-BFB8-A570E9BE0CCC),” Staff Report, *United States Senate Committee on Commerce, Science, and Transportation*, *commerce.senate.gov*, December 2013.
|
||||
|
||||
1. Olivia Solon: “[Facebook’s Failure: Did Fake News and Polarized Politics Get Trump Elected?](https://www.theguardian.com/technology/2016/nov/10/facebook-fake-news-election-conspiracy-theories)” *theguardian.com*, November 10, 2016.
|
||||
|
||||
1. Donella H. Meadows and Diana Wright: *Thinking in Systems: A Primer*. Chelsea Green Publishing, 2008. ISBN: 978-1-603-58055-7
|
||||
|
||||
1. Daniel J. Bernstein: “[Listening to a ‘big data’/‘data science’ talk](https://twitter.com/hashbreaker/status/598076230437568512),” *twitter.com*, May 12, 2015.
|
||||
|
||||
1. Marc Andreessen: “[Why Software Is Eating the World](http://genius.com/Marc-andreessen-why-software-is-eating-the-world-annotated),” *The Wall Street Journal*, 20 August 2011.
|
||||
|
||||
1. J. M. Porup: “[‘Internet of Things’ Security Is Hilariously Broken and Getting Worse](http://arstechnica.com/security/2016/01/how-to-search-the-internet-of-things-for-photos-of-sleeping-babies/),” *arstechnica.com*, January 23, 2016.
|
||||
|
||||
1. Bruce Schneier: <a href="https://www.schneier.com/books/data_and_goliath/">*Data and Goliath: The Hidden Battles to Collect Your Data and Control Your World*</a>. W. W. Norton, 2015. ISBN: 978-0-393-35217-7
|
||||
|
||||
1. The Grugq: “[Nothing to Hide](https://grugq.tumblr.com/post/142799983558/nothing-to-hide),” *grugq.tumblr.com*, April 15, 2016.
|
||||
|
||||
1. Tony Beltramelli: “[Deep-Spying: Spying Using Smartwatch and Deep Learning](https://arxiv.org/abs/1512.05616),” Masters Thesis, IT University of Copenhagen, December 2015. Available at *arxiv.org/abs/1512.05616*
|
||||
|
||||
1. Shoshana Zuboff: “[Big Other: Surveillance Capitalism and the Prospects of an Information Civilization](http://papers.ssrn.com/sol3/papers.cfm?abstract_id=2594754),” *Journal of Information Technology*, volume 30, number 1, pages 75–89, April 2015.[doi:10.1057/jit.2015.5](http://dx.doi.org/10.1057/jit.2015.5)
|
||||
|
||||
1. Carina C. Zona: “[Consequences of an Insightful Algorithm](https://www.youtube.com/watch?v=YRI40A4tyWU),” at *GOTO Berlin*, November 2016.
|
||||
|
||||
1. Bruce Schneier: “[Data Is a Toxic Asset, So Why Not Throw It Out?](https://www.schneier.com/essays/archives/2016/03/data_is_a_toxic_asse.html),” *schneier.com*, March 1, 2016.
|
||||
|
||||
1. John E. Dunn: “[The UK’s 15 Most Infamous Data Breaches](http://www.techworld.com/security/uks-most-infamous-data-breaches-2016-3604586/),” *techworld.com*, November 18, 2016.
|
||||
|
||||
1. Cory Scott: “[Data is not toxic - which implies no benefit - but rather hazardous material, where we must balance need vs. want](https://twitter.com/cory_scott/status/706586399483437056),” *twitter.com*, March 6, 2016.
|
||||
|
||||
1. Bruce Schneier: “[Mission Creep: When Everything Is Terrorism](https://www.schneier.com/essays/archives/2013/07/mission_creep_when_e.html),” *schneier.com*, July 16, 2013.
|
||||
|
||||
1. Lena Ulbricht and Maximilian von Grafenstein: “[Big Data: Big Power Shifts?](http://policyreview.info/articles/analysis/big-data-big-power-shifts),” *Internet Policy Review*, volume 5, number 1, March 2016. [doi:10.14763/2016.1.406](http://dx.doi.org/10.14763/2016.1.406)
|
||||
|
||||
1. Ellen P. Goodman and Julia Powles: “[Facebook and Google: Most Powerful and Secretive Empires We've Ever Known](https://www.theguardian.com/technology/2016/sep/28/google-facebook-powerful-secretive-empire-transparency),” *theguardian.com*, September 28, 2016.
|
||||
|
||||
1. [Directive 95/46/EC on the protection of individuals with regard to the processing of personal data and on the free movement of such data](http://eur-lex.europa.eu/legal-content/EN/TXT/?uri=CELEX:31995L0046), Official Journal of the European Communities No. L 281/31,
|
||||
*eur-lex.europa.eu*, November 1995.
|
||||
|
||||
1. Brendan Van Alsenoy: “[Regulating Data Protection: The Allocation of Responsibility and Risk Among Actors Involved in Personal Data Processing](https://lirias.kuleuven.be/handle/123456789/545027),” Thesis, KU Leuven Centre for IT and IP Law, August 2016.
|
||||
|
||||
1. Michiel Rhoen: “[Beyond Consent: Improving Data Protection Through Consumer Protection Law](http://policyreview.info/articles/analysis/beyond-consent-improving-data-protection-through-consumer-protection-law),” *Internet Policy Review*, volume 5, number 1, March 2016. [doi:10.14763/2016.1.404](http://dx.doi.org/10.14763/2016.1.404)
|
||||
|
||||
1. Jessica Leber: “[Your Data Footprint Is Affecting Your Life in Ways You Can’t Even Imagine](https://www.fastcoexist.com/3057514/your-data-footprint-is-affecting-your-life-in-ways-you-cant-even-imagine),” *fastcoexist.com*, March 15, 2016.
|
||||
|
||||
1. Maciej Cegłowski: “[Haunted by Data](http://idlewords.com/talks/haunted_by_data.htm),” *idlewords.com*, October 2015.
|
||||
|
||||
1. Sam Thielman: “[You Are Not What You Read: Librarians Purge User Data to Protect Privacy](https://www.theguardian.com/us-news/2016/jan/13/us-library-records-purged-data-privacy),” *theguardian.com*, January 13, 2016.
|
||||
|
||||
1. Conor Friedersdorf: “[Edward Snowden’s Other Motive for Leaking](http://www.theatlantic.com/politics/archive/2014/05/edward-snowdens-other-motive-for-leaking/370068/),” *theatlantic.com*, May 13, 2014.
|
||||
|
||||
1. Phillip Rogaway: “[The Moral Character of Cryptographic Work](http://web.cs.ucdavis.edu/~rogaway/papers/moral-fn.pdf),” Cryptology ePrint 2015/1162, December 2015.
|
170
en-us/ch2.md
Normal file
170
en-us/ch2.md
Normal file
@ -0,0 +1,170 @@
|
||||
# 2. Data Models and Query Languages
|
||||
|
||||

|
||||
|
||||
> *The limits of my language mean the limits of my world.*
|
||||
>
|
||||
> — Ludwig Wittgenstein, *Tractatus Logico-Philosophicus* (1922)
|
||||
|
||||
-------------------
|
||||
|
||||
Data models are perhaps the most important part of developing software, because they have such a profound effect: not only on how the software is written, but also on how we *think about the problem* that we are solving.
|
||||
|
||||
Most applications are built by layering one data model on top of another. For each layer, the key question is: how is it *represented* in terms of the next-lower layer? For example:
|
||||
|
||||
1. As an application developer, you look at the real world (in which there are peo‐ ple, organizations, goods, actions, money flows, sensors, etc.) and model it in terms of objects or data structures, and APIs that manipulate those data struc‐ tures. Those structures are often specific to your application.
|
||||
2. When you want to store those data structures, you express them in terms of a general-purpose data model, such as JSON or XML documents, tables in a rela‐ tional database, or a graph model.
|
||||
3. The engineers who built your database software decided on a way of representing that JSON/XML/relational/graph data in terms of bytes in memory, on disk, or on a network. The representation may allow the data to be queried, searched, manipulated, and processed in various ways.
|
||||
4. On yet lower levels, hardware engineers have figured out how to represent bytes in terms of electrical currents, pulses of light, magnetic fields, and more.
|
||||
|
||||
In a complex application there may be more intermediary levels, such as APIs built upon APIs, but the basic idea is still the same: each layer hides the complexity of the layers below it by providing a clean data model. These abstractions allow different groups of people—for example, the engineers at the database vendor and the applica‐ tion developers using their database—to work together effectively.
|
||||
|
||||
There are many different kinds of data models, and every data model embodies assumptions about how it is going to be used. Some kinds of usage are easy and some are not supported; some operations are fast and some perform badly; some data transformations feel natural and some are awkward.
|
||||
|
||||
It can take a lot of effort to master just one data model (think how many books there are on relational data modeling). Building software is hard enough, even when work‐ ing with just one data model and without worrying about its inner workings. But since the data model has such a profound effect on what the software above it can and can’t do, it’s important to choose one that is appropriate to the application.
|
||||
|
||||
In this chapter we will look at a range of general-purpose data models for data stor‐ age and querying (point 2 in the preceding list). In particular, we will compare the relational model, the document model, and a few graph-based data models. We will also look at various query languages and compare their use cases. In [Chapter 3](ch3.md) we will discuss how storage engines work; that is, how these data models are actually implemented (point 3 in the list).
|
||||
|
||||
|
||||
|
||||
## ……
|
||||
|
||||
|
||||
|
||||
## Summary
|
||||
|
||||
|
||||
Data models are a huge subject, and in this chapter we have taken a quick look at a broad variety of different models. We didn’t have space to go into all the details of each model, but hopefully the overview has been enough to whet your appetite to find out more about the model that best fits your application’s requirements.
|
||||
|
||||
Historically, data started out being represented as one big tree (the hierarchical model), but that wasn’t good for representing many-to-many relationships, so the relational model was invented to solve that problem. More recently, developers found that some applications don’t fit well in the relational model either. New nonrelational “NoSQL” datastores have diverged in two main directions:
|
||||
|
||||
1. *Document databases* target use cases where data comes in self-contained docu‐ ments and relationships between one document and another are rare.
|
||||
|
||||
2. *Graph databases* go in the opposite direction, targeting use cases where anything is potentially related to everything.
|
||||
|
||||
All three models (document, relational, and graph) are widely used today, and each is good in its respective domain. One model can be emulated in terms of another model —for example, graph data can be represented in a relational database—but the result is often awkward. That’s why we have different systems for different purposes, not a single one-size-fits-all solution.
|
||||
|
||||
One thing that document and graph databases have in common is that they typically don’t enforce a schema for the data they store, which can make it easier to adapt applications to changing requirements. However, your application most likely still assumes that data has a certain structure; it’s just a question of whether the schema is explicit (enforced on write) or implicit (handled on read).
|
||||
|
||||
Each data model comes with its own query language or framework, and we discussed several examples: SQL, MapReduce, MongoDB’s aggregation pipeline, Cypher, SPARQL, and Datalog. We also touched on CSS and XSL/XPath, which aren’t data‐ base query languages but have interesting parallels.
|
||||
|
||||
Although we have covered a lot of ground, there are still many data models left unmentioned. To give just a few brief examples:
|
||||
|
||||
* Researchers working with genome data often need to perform *sequence- similarity searches*, which means taking one very long string (representing a DNA molecule) and matching it against a large database of strings that are simi‐ lar, but not identical. None of the databases described here can handle this kind of usage, which is why researchers have written specialized genome database software like GenBank [48].
|
||||
|
||||
- Particle physicists have been doing Big Data–style large-scale data analysis for decades, and projects like the Large Hadron Collider (LHC) now work with hun‐ dreds of petabytes! At such a scale custom solutions are required to stop the hardware cost from spiraling out of control [49].
|
||||
- *Full-text search* is arguably a kind of data model that is frequently used alongside databases. Information retrieval is a large specialist subject that we won’t cover in great detail in this book, but we’ll touch on search indexes in [Chapter 3](ch3.md) and [Part III](part-iii.md).
|
||||
|
||||
We have to leave it there for now. In the next chapter we will discuss some of the trade-offs that come into play when *implementing* the data models described in this chapter.
|
||||
|
||||
|
||||
|
||||
## References
|
||||
--------------------
|
||||
|
||||
1. Edgar F. Codd: “[A Relational Model of Data for Large Shared Data Banks](https://www.seas.upenn.edu/~zives/03f/cis550/codd.pdf),” *Communications of the ACM*, volume 13, number 6, pages 377–387, June 1970. [doi:10.1145/362384.362685](http://dx.doi.org/10.1145/362384.362685)
|
||||
|
||||
1. Michael Stonebraker and Joseph M. Hellerstein: “[What Goes Around Comes Around](http://mitpress2.mit.edu/books/chapters/0262693143chapm1.pdf),”
|
||||
in *Readings in Database Systems*, 4th edition, MIT Press, pages 2–41, 2005. ISBN: 978-0-262-69314-1
|
||||
|
||||
1. Pramod J. Sadalage and Martin Fowler: *NoSQL Distilled*. Addison-Wesley, August 2012. ISBN:
|
||||
978-0-321-82662-6
|
||||
|
||||
1. Eric Evans: “[NoSQL: What's in a Name?](http://blog.sym-link.com/2009/10/30/nosql_whats_in_a_name.html),” *blog.sym-link.com*, October 30, 2009.
|
||||
|
||||
1. James Phillips: “[Surprises in Our NoSQL Adoption Survey](http://blog.couchbase.com/nosql-adoption-survey-surprises),” *blog.couchbase.com*, February 8, 2012.
|
||||
|
||||
1. Michael Wagner: *SQL/XML:2006 – Evaluierung der Standardkonformität ausgewählter Datenbanksysteme*. Diplomica Verlag, Hamburg, 2010. ISBN: 978-3-836-64609-3
|
||||
|
||||
1. “[XML Data in SQL Server](http://technet.microsoft.com/en-us/library/bb522446.aspx),” SQL Server 2012 documentation, *technet.microsoft.com*, 2013.
|
||||
|
||||
1. “[PostgreSQL 9.3.1 Documentation](http://www.postgresql.org/docs/9.3/static/index.html),” The PostgreSQL Global Development Group, 2013.
|
||||
|
||||
1. “[The MongoDB 2.4 Manual](http://docs.mongodb.org/manual/),” MongoDB, Inc., 2013.
|
||||
|
||||
1. “[RethinkDB 1.11 Documentation](http://www.rethinkdb.com/docs/),” *rethinkdb.com*, 2013.
|
||||
|
||||
1. “[Apache CouchDB 1.6 Documentation](http://docs.couchdb.org/en/latest/),” *docs.couchdb.org*, 2014.
|
||||
|
||||
1. Lin Qiao, Kapil Surlaker, Shirshanka Das, et al.: “[On Brewing Fresh Espresso: LinkedIn’s Distributed Data Serving Platform](http://www.slideshare.net/amywtang/espresso-20952131),” at *ACM International Conference on Management of Data* (SIGMOD), June 2013.
|
||||
|
||||
1. Rick Long, Mark Harrington, Robert Hain, and Geoff Nicholls: <a href="http://www.redbooks.ibm.com/redbooks/pdfs/sg245352.pdf">*IMS Primer*</a>. IBM Redbook SG24-5352-00, IBM International Technical Support Organization, January 2000.
|
||||
|
||||
1. Stephen D. Bartlett: “[IBM’s IMS—Myths, Realities, and Opportunities](ftp://public.dhe.ibm.com/software/data/ims/pdf/TCG2013015LI.pdf),” The Clipper Group Navigator, TCG2013015LI, July 2013.
|
||||
|
||||
1. Sarah Mei: “[Why You Should Never Use MongoDB](http://www.sarahmei.com/blog/2013/11/11/why-you-should-never-use-mongodb/),” *sarahmei.com*, November 11, 2013.
|
||||
|
||||
1. J. S. Knowles and D. M. R. Bell: “The CODASYL Model,” in *Databases—Role and Structure: An Advanced Course*, edited by P. M. Stocker, P. M. D. Gray, and M. P. Atkinson, pages 19–56, Cambridge University Press, 1984. ISBN: 978-0-521-25430-4
|
||||
|
||||
1. Charles W. Bachman: “[The Programmer as Navigator](http://dl.acm.org/citation.cfm?id=362534),” *Communications of the ACM*, volume 16, number 11, pages 653–658, November 1973. [doi:10.1145/355611.362534](http://dx.doi.org/10.1145/355611.362534)
|
||||
|
||||
1. Joseph M. Hellerstein, Michael Stonebraker, and James Hamilton: “[Architecture of a Database System](http://db.cs.berkeley.edu/papers/fntdb07-architecture.pdf),”
|
||||
*Foundations and Trends in Databases*, volume 1, number 2, pages 141–259, November 2007. [doi:10.1561/1900000002](http://dx.doi.org/10.1561/1900000002)
|
||||
|
||||
1. Sandeep Parikh and Kelly Stirman: “[Schema Design for Time Series Data in MongoDB](http://blog.mongodb.org/post/65517193370/schema-design-for-time-series-data-in-mongodb),” *blog.mongodb.org*, October 30, 2013.
|
||||
|
||||
1. Martin Fowler: “[Schemaless Data Structures](http://martinfowler.com/articles/schemaless/),” *martinfowler.com*, January 7, 2013.
|
||||
|
||||
1. Amr Awadallah: “[Schema-on-Read vs. Schema-on-Write](http://www.slideshare.net/awadallah/schemaonread-vs-schemaonwrite),” at *Berkeley EECS RAD Lab Retreat*, Santa Cruz, CA, May 2009.
|
||||
|
||||
1. Martin Odersky: “[The Trouble with Types](http://www.infoq.com/presentations/data-types-issues),” at *Strange Loop*, September 2013.
|
||||
|
||||
1. Conrad Irwin: “[MongoDB—Confessions of a PostgreSQL Lover](https://speakerdeck.com/conradirwin/mongodb-confessions-of-a-postgresql-lover),” at *HTML5DevConf*, October 2013.
|
||||
|
||||
1. “[Percona Toolkit Documentation: pt-online-schema-change](http://www.percona.com/doc/percona-toolkit/2.2/pt-online-schema-change.html),” Percona Ireland Ltd., 2013.
|
||||
|
||||
1. Rany Keddo, Tobias Bielohlawek, and Tobias Schmidt: “[Large Hadron Migrator](https://github.com/soundcloud/lhm),” SoundCloud, 2013. Shlomi Noach:
|
||||
|
||||
“[gh-ost: GitHub's Online Schema Migration Tool for MySQL](http://githubengineering.com/gh-ost-github-s-online-migration-tool-for-mysql/),” *githubengineering.com*, August 1, 2016.
|
||||
|
||||
1. James C. Corbett, Jeffrey Dean, Michael Epstein, et al.: “[Spanner: Google’s Globally-Distributed Database](http://research.google.com/archive/spanner.html),” at *10th USENIX Symposium on Operating System Design and Implementation* (OSDI),
|
||||
October 2012.
|
||||
|
||||
1. Donald K. Burleson: “[Reduce I/O with Oracle Cluster Tables](http://www.dba-oracle.com/oracle_tip_hash_index_cluster_table.htm),” *dba-oracle.com*.
|
||||
|
||||
1. Fay Chang, Jeffrey Dean, Sanjay Ghemawat, et al.: “[Bigtable: A Distributed Storage System for Structured Data](http://research.google.com/archive/bigtable.html),” at *7th USENIX Symposium on Operating System Design and Implementation* (OSDI), November 2006.
|
||||
|
||||
1. Bobbie J. Cochrane and Kathy A. McKnight: “[DB2 JSON Capabilities, Part 1: Introduction to DB2 JSON](http://www.ibm.com/developerworks/data/library/techarticle/dm-1306nosqlforjson1/),” IBM developerWorks, June 20, 2013.
|
||||
|
||||
1. Herb Sutter: “[The Free Lunch Is Over: A Fundamental Turn Toward Concurrency in Software](http://www.gotw.ca/publications/concurrency-ddj.htm),” *Dr. Dobb's Journal*, volume 30, number 3, pages 202-210, March 2005.
|
||||
|
||||
1. Joseph M. Hellerstein: “[The Declarative Imperative: Experiences and Conjectures in Distributed Logic](http://www.eecs.berkeley.edu/Pubs/TechRpts/2010/EECS-2010-90.pdf),” Electrical Engineering and Computer Sciences, University of California at Berkeley, Tech report UCB/EECS-2010-90, June 2010.
|
||||
|
||||
1. Jeffrey Dean and Sanjay Ghemawat: “[MapReduce: Simplified Data Processing on Large Clusters](http://research.google.com/archive/mapreduce.html),” at *6th USENIX Symposium on Operating System Design and Implementation* (OSDI), December 2004.
|
||||
|
||||
1. Craig Kerstiens: “[JavaScript in Your Postgres](https://blog.heroku.com/javascript_in_your_postgres),” *blog.heroku.com*, June 5, 2013.
|
||||
|
||||
1. Nathan Bronson, Zach Amsden, George Cabrera, et al.: “[TAO: Facebook’s Distributed Data Store for the Social Graph](https://www.usenix.org/conference/atc13/technical-sessions/presentation/bronson),” at *USENIX Annual Technical Conference* (USENIX ATC), June 2013.
|
||||
|
||||
1. “[Apache TinkerPop3.2.3 Documentation](http://tinkerpop.apache.org/docs/3.2.3/reference/),” *tinkerpop.apache.org*, October 2016.
|
||||
|
||||
1. “[The Neo4j Manual v2.0.0](http://docs.neo4j.org/chunked/2.0.0/index.html),” Neo Technology, 2013. Emil Eifrem: [Twitter correspondence](https://twitter.com/emileifrem/status/419107961512804352), January 3, 2014.
|
||||
|
||||
1. David Beckett and Tim Berners-Lee: “[Turtle – Terse RDF Triple Language](http://www.w3.org/TeamSubmission/turtle/),” W3C Team Submission, March 28, 2011.
|
||||
|
||||
1. “[Datomic Development Resources](http://docs.datomic.com/),” Metadata Partners, LLC, 2013. W3C RDF Working Group: “[Resource Description Framework (RDF)](http://www.w3.org/RDF/),” *w3.org*, 10 February 2004.
|
||||
|
||||
1. “[Apache Jena](http://jena.apache.org/),” Apache Software Foundation.
|
||||
|
||||
1. Steve Harris, Andy Seaborne, and Eric Prud'hommeaux: “[SPARQL 1.1 Query Language](http://www.w3.org/TR/sparql11-query/),”
|
||||
W3C Recommendation, March 2013.
|
||||
|
||||
1. Todd J. Green, Shan Shan Huang, Boon Thau Loo, and Wenchao Zhou: “[Datalog and Recursive Query Processing](http://blogs.evergreen.edu/sosw/files/2014/04/Green-Vol5-DBS-017.pdf),” *Foundations and Trends in Databases*, volume 5, number 2, pages 105–195, November 2013. [doi:10.1561/1900000017](http://dx.doi.org/10.1561/1900000017)
|
||||
|
||||
1. Stefano Ceri, Georg Gottlob, and Letizia Tanca: “[What You Always Wanted to Know About Datalog (And Never Dared to Ask)](https://www.researchgate.net/profile/Letizia_Tanca/publication/3296132_What_you_always_wanted_to_know_about_Datalog_and_never_dared_to_ask/links/0fcfd50ca2d20473ca000000.pdf),” *IEEE Transactions on Knowledge and Data Engineering*, volume 1, number 1, pages 146–166, March 1989. [doi:10.1109/69.43410](http://dx.doi.org/10.1109/69.43410)
|
||||
|
||||
1. Serge Abiteboul, Richard Hull, and Victor Vianu: <a href="http://webdam.inria.fr/Alice/">*Foundations of Databases*</a>. Addison-Wesley, 1995. ISBN: 978-0-201-53771-0, available online at *webdam.inria.fr/Alice*
|
||||
|
||||
1. Nathan Marz: “[Cascalog](http://cascalog.org/)," *cascalog.org*. Dennis A. Benson, Ilene Karsch-Mizrachi, David J. Lipman, et al.:
|
||||
|
||||
“[GenBank](http://nar.oxfordjournals.org/content/36/suppl_1/D25.full-text-lowres.pdf),” *Nucleic Acids Research*, volume 36, Database issue, pages D25–D30, December 2007. [doi:10.1093/nar/gkm929](http://dx.doi.org/10.1093/nar/gkm929)
|
||||
|
||||
1. Fons Rademakers: “[ROOT for Big Data Analysis](http://indico.cern.ch/getFile.py/access?contribId=13&resId=0&materialId=slides&confId=246453),” at *Workshop on the Future of Big Data Management*,
|
||||
London, UK, June 2013.
|
||||
|
||||
------
|
||||
|
||||
| 上一章 | 目录 | 下一章 |
|
||||
| -------------------------------------------- | ------------------------------- | ---------------------------- |
|
||||
| [第一章:可靠性、可伸缩性、可维护性](ch1.md) | [设计数据密集型应用](README.md) | [第三章:存储与检索](ch3.md) |
|
||||
|
194
en-us/ch3.md
Normal file
194
en-us/ch3.md
Normal file
@ -0,0 +1,194 @@
|
||||
# 3. Storage and Retrieval
|
||||
|
||||

|
||||
|
||||
> *Wer Ordnung hält, ist nur zu faul zum Suchen.
|
||||
> (If you keep things tidily ordered, you’re just too lazy to go searching.)*
|
||||
>
|
||||
> — German proverb
|
||||
|
||||
-------------------
|
||||
|
||||
On the most fundamental level, a database needs to do two things: when you give it some data, it should store the data, and when you ask it again later, it should give the data back to you.
|
||||
|
||||
In [Chapter 2](ch2.md) we discussed data models and query languages—i.e., the format in which you (the application developer) give the database your data, and the mecha‐ nism by which you can ask for it again later. In this chapter we discuss the same from the database’s point of view: how we can store the data that we’re given, and how we can find it again when we’re asked for it.
|
||||
|
||||
Why should you, as an application developer, care how the database handles storage and retrieval internally? You’re probably not going to implement your own storage engine from scratch, but you *do* need to select a storage engine that is appropriate for your application, from the many that are available. In order to tune a storage engine to perform well on your kind of workload, you need to have a rough idea of what the storage engine is doing under the hood.
|
||||
|
||||
In particular, there is a big difference between storage engines that are optimized for transactional workloads and those that are optimized for analytics. We will explore that distinction later in “[Transaction Processing or Analytics?](#transaction-processing-or-analytics)”, and in “[Column-Oriented Storage](#column-oriented-storage)” we’ll discuss a family of storage engines that is optimized for analytics.
|
||||
|
||||
However, first we’ll start this chapter by talking about storage engines that are used in the kinds of databases that you’re probably familiar with: traditional relational data‐ bases, and also most so-called NoSQL databases. We will examine two families of storage engines: *log-structured* storage engines, and *page-oriented* storage engines such as B-trees.
|
||||
|
||||
|
||||
|
||||
## ……
|
||||
|
||||
|
||||
|
||||
## Summary
|
||||
|
||||
|
||||
In this chapter we tried to get to the bottom of how databases handle storage and retrieval. What happens when you store data in a database, and what does the data‐ base do when you query for the data again later?
|
||||
|
||||
On a high level, we saw that storage engines fall into two broad categories: those opti‐ mized for transaction processing (OLTP), and those optimized for analytics (OLAP). There are big differences between the access patterns in those use cases:
|
||||
|
||||
- OLTP systems are typically user-facing, which means that they may see a huge volume of requests. In order to handle the load, applications usually only touch a small number of records in each query. The application requests records using some kind of key, and the storage engine uses an index to find the data for the requested key. Disk seek time is often the bottleneck here.
|
||||
|
||||
- Data warehouses and similar analytic systems are less well known, because they are primarily used by business analysts, not by end users. They handle a much lower volume of queries than OLTP systems, but each query is typically very demanding, requiring many millions of records to be scanned in a short time. Disk bandwidth (not seek time) is often the bottleneck here, and column- oriented storage is an increasingly popular solution for this kind of workload.
|
||||
|
||||
On the OLTP side, we saw storage engines from two main schools of thought:
|
||||
|
||||
- The log-structured school, which only permits appending to files and deleting obsolete files, but never updates a file that has been written. Bitcask, SSTables, LSM-trees, LevelDB, Cassandra, HBase, Lucene, and others belong to this group.
|
||||
|
||||
- The update-in-place school, which treats the disk as a set of fixed-size pages that can be overwritten. B-trees are the biggest example of this philosophy, being used in all major relational databases and also many nonrelational ones.
|
||||
|
||||
Log-structured storage engines are a comparatively recent development. Their key idea is that they systematically turn random-access writes into sequential writes on disk, which enables higher write throughput due to the performance characteristics of hard drives and SSDs.
|
||||
|
||||
Finishing off the OLTP side, we did a brief tour through some more complicated indexing structures, and databases that are optimized for keeping all data in memory.
|
||||
|
||||
We then took a detour from the internals of storage engines to look at the high-level architecture of a typical data warehouse. This background illustrated why analytic workloads are so different from OLTP: when your queries require sequentially scan‐ ning across a large number of rows, indexes are much less relevant. Instead it becomes important to encode data very compactly, to minimize the amount of data that the query needs to read from disk. We discussed how column-oriented storage helps achieve this goal.
|
||||
|
||||
As an application developer, if you’re armed with this knowledge about the internals of storage engines, you are in a much better position to know which tool is best suited for your particular application. If you need to adjust a database’s tuning parameters, this understanding allows you to imagine what effect a higher or a lower value may have.
|
||||
|
||||
Although this chapter couldn’t make you an expert in tuning any one particular stor‐ age engine, it has hopefully equipped you with enough vocabulary and ideas that you can make sense of the documentation for the database of your choice.
|
||||
|
||||
## References
|
||||
--------------------
|
||||
|
||||
|
||||
1. Alfred V. Aho, John E. Hopcroft, and Jeffrey D. Ullman: *Data Structures and Algorithms*. Addison-Wesley, 1983. ISBN: 978-0-201-00023-8
|
||||
|
||||
1. Thomas H. Cormen, Charles E. Leiserson, Ronald L. Rivest, and Clifford Stein: *Introduction to Algorithms*, 3rd edition. MIT Press, 2009. ISBN: 978-0-262-53305-8
|
||||
|
||||
1. Justin Sheehy and David Smith: “[Bitcask: A Log-Structured Hash Table for Fast Key/Value Data](http://basho.com/wp-content/uploads/2015/05/bitcask-intro.pdf),” Basho Technologies, April 2010.
|
||||
|
||||
1. Yinan Li, Bingsheng He, Robin Jun Yang, et al.: “[Tree Indexing on Solid State Drives](http://www.vldb.org/pvldb/vldb2010/papers/R106.pdf),” *Proceedings of the VLDB Endowment*, volume 3, number 1, pages 1195–1206, September 2010.
|
||||
|
||||
1. Goetz Graefe: “[Modern B-Tree Techniques](http://citeseerx.ist.psu.edu/viewdoc/download?doi=10.1.1.219.7269&rep=rep1&type=pdf),” *Foundations and Trends in Databases*, volume 3, number 4, pages 203–402, August 2011. [doi:10.1561/1900000028](http://dx.doi.org/10.1561/1900000028)
|
||||
|
||||
1. Jeffrey Dean and Sanjay Ghemawat: “[LevelDB Implementation Notes](https://github.com/google/leveldb/blob/master/doc/impl.html),” *leveldb.googlecode.com*.
|
||||
|
||||
1. Dhruba Borthakur: “[The History of RocksDB](http://rocksdb.blogspot.com/),” *rocksdb.blogspot.com*, November 24, 2013.
|
||||
|
||||
1. Matteo Bertozzi: “[Apache HBase I/O – HFile](http://blog.cloudera.com/blog/2012/06/hbase-io-hfile-input-output/),” *blog.cloudera.com*, June, 29 2012.
|
||||
|
||||
1. Fay Chang, Jeffrey Dean, Sanjay Ghemawat, et al.: “[Bigtable: A Distributed Storage System for Structured Data](http://research.google.com/archive/bigtable.html),” at *7th USENIX Symposium on Operating System Design and Implementation* (OSDI), November 2006.
|
||||
|
||||
1. Patrick O'Neil, Edward Cheng, Dieter Gawlick, and Elizabeth O'Neil: “[The Log-Structured Merge-Tree (LSM-Tree)](http://www.cs.umb.edu/~poneil/lsmtree.pdf),” *Acta Informatica*, volume 33, number 4, pages 351–385, June 1996. [doi:10.1007/s002360050048](http://dx.doi.org/10.1007/s002360050048)
|
||||
|
||||
1. Mendel Rosenblum and John K. Ousterhout: “[The Design and Implementation of a Log-Structured File System](http://research.cs.wisc.edu/areas/os/Qual/papers/lfs.pdf),” *ACM Transactions on Computer Systems*, volume 10, number 1, pages 26–52, February 1992.
|
||||
[doi:10.1145/146941.146943](http://dx.doi.org/10.1145/146941.146943)
|
||||
|
||||
1. Adrien Grand: “[What Is in a Lucene Index?](http://www.slideshare.net/lucenerevolution/what-is-inaluceneagrandfinal),” at *Lucene/Solr Revolution*, November 14, 2013.
|
||||
|
||||
1. Deepak Kandepet: “[Hacking Lucene—The Index Format]( http://hackerlabs.github.io/blog/2011/10/01/hacking-lucene-the-index-format/index.html),” *hackerlabs.org*, October 1, 2011.
|
||||
|
||||
1. Michael McCandless: “[Visualizing Lucene's Segment Merges](http://blog.mikemccandless.com/2011/02/visualizing-lucenes-segment-merges.html),” *blog.mikemccandless.com*, February 11, 2011.
|
||||
|
||||
1. Burton H. Bloom: “[Space/Time Trade-offs in Hash Coding with Allowable Errors](http://www.cs.upc.edu/~diaz/p422-bloom.pdf),” *Communications of the ACM*, volume 13, number 7, pages 422–426, July 1970. [doi:10.1145/362686.362692](http://dx.doi.org/10.1145/362686.362692)
|
||||
|
||||
1. “[Operating Cassandra: Compaction](https://cassandra.apache.org/doc/latest/operating/compaction.html),” Apache Cassandra Documentation v4.0, 2016.
|
||||
|
||||
1. Rudolf Bayer and Edward M. McCreight: “[Organization and Maintenance of Large Ordered Indices](http://www.dtic.mil/cgi-bin/GetTRDoc?AD=AD0712079),” Boeing Scientific Research Laboratories, Mathematical and Information Sciences Laboratory, report no. 20, July 1970.
|
||||
|
||||
1. Douglas Comer: “[The Ubiquitous B-Tree](http://citeseerx.ist.psu.edu/viewdoc/download?doi=10.1.1.96.6637&rep=rep1&type=pdf),” *ACM Computing Surveys*, volume 11, number 2, pages 121–137, June 1979. [doi:10.1145/356770.356776](http://dx.doi.org/10.1145/356770.356776)
|
||||
|
||||
1. Emmanuel Goossaert: “[Coding for SSDs](http://codecapsule.com/2014/02/12/coding-for-ssds-part-1-introduction-and-table-of-contents/),” *codecapsule.com*, February 12, 2014.
|
||||
|
||||
1. C. Mohan and Frank Levine: “[ARIES/IM: An Efficient and High Concurrency Index Management Method Using Write-Ahead Logging](http://www.ics.uci.edu/~cs223/papers/p371-mohan.pdf),” at *ACM International Conference on Management of Data* (SIGMOD), June 1992. [doi:10.1145/130283.130338](http://dx.doi.org/10.1145/130283.130338)
|
||||
|
||||
1. Howard Chu: “[LDAP at Lightning Speed]( https://buildstuff14.sched.com/event/08a1a368e272eb599a52e08b4c3c779d),” at *Build Stuff '14*, November 2014.
|
||||
|
||||
1. Bradley C. Kuszmaul: “[A Comparison of Fractal Trees to Log-Structured Merge (LSM) Trees](http://insideanalysis.com/wp-content/uploads/2014/08/Tokutek_lsm-vs-fractal.pdf),” *tokutek.com*, April 22, 2014.
|
||||
|
||||
1. Manos Athanassoulis, Michael S. Kester, Lukas M. Maas, et al.: “[Designing Access Methods: The RUM Conjecture](http://openproceedings.org/2016/conf/edbt/paper-12.pdf),” at *19th International Conference on Extending Database Technology* (EDBT), March 2016.
|
||||
[doi:10.5441/002/edbt.2016.42](http://dx.doi.org/10.5441/002/edbt.2016.42)
|
||||
|
||||
1. Peter Zaitsev: “[Innodb Double Write](https://www.percona.com/blog/2006/08/04/innodb-double-write/),” *percona.com*, August 4, 2006.
|
||||
|
||||
1. Tomas Vondra: “[On the Impact of Full-Page Writes](http://blog.2ndquadrant.com/on-the-impact-of-full-page-writes/),” *blog.2ndquadrant.com*, November 23, 2016.
|
||||
|
||||
1. Mark Callaghan: “[The Advantages of an LSM vs a B-Tree](http://smalldatum.blogspot.co.uk/2016/01/summary-of-advantages-of-lsm-vs-b-tree.html),” *smalldatum.blogspot.co.uk*, January 19, 2016.
|
||||
|
||||
1. Mark Callaghan: “[Choosing Between Efficiency and Performance with RocksDB](http://www.codemesh.io/codemesh/mark-callaghan),” at *Code Mesh*, November 4, 2016.
|
||||
|
||||
1. Michi Mutsuzaki: “[MySQL vs. LevelDB](https://github.com/m1ch1/mapkeeper/wiki/MySQL-vs.-LevelDB),” *github.com*, August 2011.
|
||||
|
||||
1. Benjamin Coverston, Jonathan Ellis, et al.: “[CASSANDRA-1608: Redesigned Compaction](https://issues.apache.org/jira/browse/CASSANDRA-1608), *issues.apache.org*, July 2011.
|
||||
|
||||
1. Igor Canadi, Siying Dong, and Mark Callaghan: “[RocksDB Tuning Guide](https://github.com/facebook/rocksdb/wiki/RocksDB-Tuning-Guide),”
|
||||
*github.com*, 2016.
|
||||
|
||||
1. [*MySQL 5.7 Reference Manual*](http://dev.mysql.com/doc/refman/5.7/en/index.html). Oracle, 2014.
|
||||
|
||||
1. [*Books Online for SQL Server 2012*](http://msdn.microsoft.com/en-us/library/ms130214.aspx). Microsoft, 2012.
|
||||
|
||||
1. Joe Webb: “[Using Covering Indexes to Improve Query Performance](https://www.simple-talk.com/sql/learn-sql-server/using-covering-indexes-to-improve-query-performance/),” *simple-talk.com*, 29 September 2008.
|
||||
|
||||
1. Frank Ramsak, Volker Markl, Robert Fenk, et al.: “[Integrating the UB-Tree into a Database System Kernel](http://www.vldb.org/conf/2000/P263.pdf),” at *26th International Conference on Very Large Data Bases* (VLDB), September 2000.
|
||||
|
||||
1. The PostGIS Development Group: “[PostGIS 2.1.2dev Manual](http://postgis.net/docs/manual-2.1/),” *postgis.net*, 2014.
|
||||
|
||||
1. Robert Escriva, Bernard Wong, and Emin Gün Sirer: “[HyperDex: A Distributed, Searchable Key-Value Store](http://www.cs.princeton.edu/courses/archive/fall13/cos518/papers/hyperdex.pdf),” at *ACM SIGCOMM Conference*, August 2012. [doi:10.1145/2377677.2377681](http://dx.doi.org/10.1145/2377677.2377681)
|
||||
|
||||
1. Michael McCandless: “[Lucene's FuzzyQuery Is 100 Times Faster in 4.0](http://blog.mikemccandless.com/2011/03/lucenes-fuzzyquery-is-100-times-faster.html),” *blog.mikemccandless.com*, March 24, 2011.
|
||||
|
||||
1. Steffen Heinz, Justin Zobel, and Hugh E. Williams: “[Burst Tries: A Fast, Efficient Data Structure for String Keys](http://citeseer.ist.psu.edu/viewdoc/summary?doi=10.1.1.18.3499),” *ACM Transactions on Information Systems*, volume 20, number 2, pages 192–223, April 2002. [doi:10.1145/506309.506312](http://dx.doi.org/10.1145/506309.506312)
|
||||
|
||||
1. Klaus U. Schulz and Stoyan Mihov: “[Fast String Correction with Levenshtein Automata](http://citeseerx.ist.psu.edu/viewdoc/summary?doi=10.1.1.16.652),” *International Journal on Document Analysis and Recognition*, volume 5, number 1, pages 67–85, November 2002. [doi:10.1007/s10032-002-0082-8](http://dx.doi.org/10.1007/s10032-002-0082-8)
|
||||
|
||||
1. Christopher D. Manning, Prabhakar Raghavan, and Hinrich Schütze: [*Introduction to Information Retrieval*](http://nlp.stanford.edu/IR-book/). Cambridge University Press, 2008. ISBN: 978-0-521-86571-5, available online at *nlp.stanford.edu/IR-book*
|
||||
|
||||
1. Michael Stonebraker, Samuel Madden, Daniel J. Abadi, et al.: “[The End of an Architectural Era (It’s Time for a Complete Rewrite)](http://citeseerx.ist.psu.edu/viewdoc/download?doi=10.1.1.137.3697&rep=rep1&type=pdf),” at *33rd International Conference on Very Large Data Bases* (VLDB), September 2007.
|
||||
|
||||
1. “[VoltDB Technical Overview White Paper](https://www.voltdb.com/wptechnicaloverview),” VoltDB, 2014.
|
||||
|
||||
1. Stephen M. Rumble, Ankita Kejriwal, and John K. Ousterhout: “[Log-Structured Memory for DRAM-Based Storage](https://www.usenix.org/system/files/conference/fast14/fast14-paper_rumble.pdf),” at *12th USENIX Conference on File and Storage Technologies* (FAST), February 2014.
|
||||
|
||||
1. Stavros Harizopoulos, Daniel J. Abadi, Samuel Madden, and Michael Stonebraker: “[OLTP Through the Looking Glass, and What We Found There](http://hstore.cs.brown.edu/papers/hstore-lookingglass.pdf),” at *ACM International Conference on Management of Data*
|
||||
(SIGMOD), June 2008. [doi:10.1145/1376616.1376713](http://dx.doi.org/10.1145/1376616.1376713)
|
||||
|
||||
1. Justin DeBrabant, Andrew Pavlo, Stephen Tu, et al.: “[Anti-Caching: A New Approach to Database Management System Architecture](http://www.vldb.org/pvldb/vol6/p1942-debrabant.pdf),” *Proceedings of the VLDB Endowment*, volume 6, number 14, pages 1942–1953, September 2013.
|
||||
|
||||
1. Joy Arulraj, Andrew Pavlo, and Subramanya R. Dulloor: “[Let's Talk About Storage & Recovery Methods for Non-Volatile Memory Database Systems](http://www.pdl.cmu.edu/PDL-FTP/NVM/storage.pdf),” at *ACM International Conference on Management of Data* (SIGMOD), June 2015. [doi:10.1145/2723372.2749441](http://dx.doi.org/10.1145/2723372.2749441)
|
||||
|
||||
1. Edgar F. Codd, S. B. Codd, and C. T. Salley: “[Providing OLAP to User-Analysts: An IT Mandate](http://www.minet.uni-jena.de/dbis/lehre/ss2005/sem_dwh/lit/Cod93.pdf),” E. F. Codd Associates, 1993.
|
||||
|
||||
1. Surajit Chaudhuri and Umeshwar Dayal: “[An Overview of Data Warehousing and OLAP Technology](https://www.microsoft.com/en-us/research/wp-content/uploads/2016/02/sigrecord.pdf),” *ACM SIGMOD Record*, volume 26, number 1, pages 65–74, March 1997. [doi:10.1145/248603.248616](http://dx.doi.org/10.1145/248603.248616)
|
||||
|
||||
1. Per-Åke Larson, Cipri Clinciu, Campbell Fraser, et al.: “[Enhancements to SQL Server Column Stores](http://research.microsoft.com/pubs/193599/Apollo3%20-%20Sigmod%202013%20-%20final.pdf),” at *ACM International Conference on Management of Data* (SIGMOD), June 2013.
|
||||
|
||||
1. Franz Färber, Norman May, Wolfgang Lehner, et al.: “[The SAP HANA Database – An Architecture Overview](http://sites.computer.org/debull/A12mar/hana.pdf),” *IEEE Data Engineering Bulletin*, volume 35, number 1, pages 28–33, March 2012.
|
||||
|
||||
1. Michael Stonebraker: “[The Traditional RDBMS Wisdom Is (Almost Certainly) All Wrong](http://slideshot.epfl.ch/talks/166),” presentation at *EPFL*, May 2013.
|
||||
|
||||
1. Daniel J. Abadi: “[Classifying the SQL-on-Hadoop Solutions](https://web.archive.org/web/20150622074951/http://hadapt.com/blog/2013/10/02/classifying-the-sql-on-hadoop-solutions/),” *hadapt.com*, October 2, 2013.
|
||||
|
||||
1. Marcel Kornacker, Alexander Behm, Victor Bittorf, et al.: “[Impala: A Modern, Open-Source SQL Engine for Hadoop](http://pandis.net/resources/cidr15impala.pdf),” at *7th Biennial Conference on Innovative Data Systems Research* (CIDR), January 2015.
|
||||
|
||||
1. Sergey Melnik, Andrey Gubarev, Jing Jing Long, et al.: “[Dremel: Interactive Analysis of Web-Scale Datasets](http://research.google.com/pubs/pub36632.html),” at *36th International Conference on Very Large Data Bases* (VLDB), pages
|
||||
330–339, September 2010.
|
||||
|
||||
1. Ralph Kimball and Margy Ross: *The Data Warehouse Toolkit: The Definitive Guide to Dimensional Modeling*, 3rd edition. John Wiley & Sons, July 2013. ISBN: 978-1-118-53080-1
|
||||
|
||||
1. Derrick Harris: “[Why Apple, eBay, and Walmart Have Some of the Biggest Data Warehouses You’ve Ever Seen](http://gigaom.com/2013/03/27/why-apple-ebay-and-walmart-have-some-of-the-biggest-data-warehouses-youve-ever-seen/),” *gigaom.com*, March 27, 2013.
|
||||
|
||||
1. Julien Le Dem: “[Dremel Made Simple with Parquet](https://blog.twitter.com/2013/dremel-made-simple-with-parquet),” *blog.twitter.com*, September 11, 2013.
|
||||
|
||||
1. Daniel J. Abadi, Peter Boncz, Stavros Harizopoulos, et al.: “[The Design and Implementation of Modern Column-Oriented Database Systems](http://cs-www.cs.yale.edu/homes/dna/papers/abadi-column-stores.pdf),” *Foundations and Trends in Databases*, volume 5, number 3, pages 197–280, December 2013. [doi:10.1561/1900000024](http://dx.doi.org/10.1561/1900000024)
|
||||
|
||||
1. Peter Boncz, Marcin Zukowski, and Niels Nes: “[MonetDB/X100: Hyper-Pipelining Query Execution](http://www.cidrdb.org/cidr2005/papers/P19.pdf),”
|
||||
at *2nd Biennial Conference on Innovative Data Systems Research* (CIDR), January 2005.
|
||||
|
||||
1. Jingren Zhou and Kenneth A. Ross: “[Implementing Database Operations Using SIMD Instructions](http://www1.cs.columbia.edu/~kar/pubsk/simd.pdf),”
|
||||
at *ACM International Conference on Management of Data* (SIGMOD), pages 145–156, June 2002.
|
||||
[doi:10.1145/564691.564709](http://dx.doi.org/10.1145/564691.564709)
|
||||
|
||||
1. Michael Stonebraker, Daniel J. Abadi, Adam Batkin, et al.: “[C-Store: A Column-oriented DBMS](http://www.vldb2005.org/program/paper/thu/p553-stonebraker.pdf),”
|
||||
at *31st International Conference on Very Large Data Bases* (VLDB), pages 553–564, September 2005.
|
||||
|
||||
1. Andrew Lamb, Matt Fuller, Ramakrishna Varadarajan, et al.: “[The Vertica Analytic Database: C-Store 7 Years Later](http://vldb.org/pvldb/vol5/p1790_andrewlamb_vldb2012.pdf),” *Proceedings of the VLDB Endowment*, volume 5, number 12, pages 1790–1801, August 2012.
|
||||
|
||||
1. Julien Le Dem and Nong Li: “[Efficient Data Storage for Analytics with Apache Parquet 2.0](http://www.slideshare.net/julienledem/th-210pledem),” at *Hadoop Summit*, San Jose, June 2014.
|
||||
|
||||
1. Jim Gray, Surajit Chaudhuri, Adam Bosworth, et al.: “[Data Cube: A Relational Aggregation Operator Generalizing Group-By, Cross-Tab, and Sub-Totals](http://arxiv.org/pdf/cs/0701155.pdf),” *Data Mining and Knowledge Discovery*, volume 1, number 1, pages 29–53, March 2007. [doi:10.1023/A:1009726021843](http://dx.doi.org/10.1023/A:1009726021843)
|
178
en-us/ch4.md
Normal file
178
en-us/ch4.md
Normal file
@ -0,0 +1,178 @@
|
||||
# 4. Encoding and Evolution
|
||||
|
||||

|
||||
|
||||
> *Everything changes and nothing stands still.*
|
||||
>
|
||||
> — Heraclitus of Ephesus, as quoted by Plato in *Cratylus* (360 BCE)
|
||||
|
||||
-------------------
|
||||
|
||||
Applications inevitably change over time. Features are added or modified as new products are launched, user requirements become better understood, or business cir‐ cumstances change. In [Chapter 1](ch1.mdj) we introduced the idea of *evolvability*: we should aim to build systems that make it easy to adapt to change (see “[Evolvability: Making Change Easy](ch1.md#evolvability-making-change-easy)”).
|
||||
|
||||
In most cases, a change to an application’s features also requires a change to data that it stores: perhaps a new field or record type needs to be captured, or perhaps existing data needs to be presented in a new way.
|
||||
|
||||
The data models we discussed in [Chapter 2](ch2.md) have different ways of coping with such change. Relational databases generally assume that all data in the database conforms to one schema: although that schema can be changed (through schema migrations; i.e., ALTER statements), there is exactly one schema in force at any one point in time. By contrast, schema-on-read (“schemaless”) databases don’t enforce a schema, so the database can contain a mixture of older and newer data formats written at different times (see “[Schema flexibility in the document model](ch3.md#schema-flexibility-in-the-document-model)”).
|
||||
|
||||
When a data format or schema changes, a corresponding change to application code often needs to happen (for example, you add a new field to a record, and the applica‐ tion code starts reading and writing that field). However, in a large application, code changes often cannot happen instantaneously:
|
||||
|
||||
- With server-side applications you may want to perform a *rolling upgrade* (also known as a *staged rollout*), deploying the new version to a few nodes at a time, checking whether the new version is running smoothly, and gradually working your way through all the nodes. This allows new versions to be deployed without service downtime, and thus encourages more frequent releases and better evolva‐ bility.
|
||||
- With client-side applications you’re at the mercy of the user, who may not install the update for some time.
|
||||
|
||||
This means that old and new versions of the code, and old and new data formats, may potentially all coexist in the system at the same time. In order for the system to continue running smoothly, we need to maintain compatibility in both directions:
|
||||
|
||||
***Backward compatibility***
|
||||
|
||||
Newer code can read data that was written by older code.
|
||||
|
||||
***Forward compatibility***
|
||||
|
||||
Older code can read data that was written by newer code.
|
||||
|
||||
Backward compatibility is normally not hard to achieve: as author of the newer code, you know the format of data written by older code, and so you can explicitly handle it (if necessary by simply keeping the old code to read the old data). Forward compati‐ bility can be trickier, because it requires older code to ignore additions made by a newer version of the code.
|
||||
|
||||
In this chapter we will look at several formats for encoding data, including JSON, XML, Protocol Buffers, Thrift, and Avro. In particular, we will look at how they han‐ dle schema changes and how they support systems where old and new data and code need to coexist. We will then discuss how those formats are used for data storage and for communication: in web services, Representational State Transfer (REST), and remote procedure calls (RPC), as well as message-passing systems such as actors and message queues.
|
||||
|
||||
|
||||
|
||||
## ……
|
||||
|
||||
|
||||
|
||||
## Summary
|
||||
|
||||
In this chapter we looked at several ways of turning data structures into bytes on the network or bytes on disk. We saw how the details of these encodings affect not only their efficiency, but more importantly also the architecture of applications and your options for deploying them.
|
||||
|
||||
In particular, many services need to support rolling upgrades, where a new version of a service is gradually deployed to a few nodes at a time, rather than deploying to all nodes simultaneously. Rolling upgrades allow new versions of a service to be released without downtime (thus encouraging frequent small releases over rare big releases) and make deployments less risky (allowing faulty releases to be detected and rolled back before they affect a large number of users). These properties are hugely benefi‐ cial for *evolvability*, the ease of making changes to an application.
|
||||
|
||||
During rolling upgrades, or for various other reasons, we must assume that different nodes are running the different versions of our application’s code. Thus, it is impor‐ tant that all data flowing around the system is encoded in a way that provides back‐ ward compatibility (new code can read old data) and forward compatibility (old code can read new data).
|
||||
|
||||
We discussed several data encoding formats and their compatibility properties:
|
||||
|
||||
- Programming language–specific encodings are restricted to a single program‐ ming language and often fail to provide forward and backward compatibility.
|
||||
- Textual formats like JSON, XML, and CSV are widespread, and their compatibil‐ ity depends on how you use them. They have optional schema languages, which are sometimes helpful and sometimes a hindrance. These formats are somewhat vague about datatypes, so you have to be careful with things like numbers and binary strings.
|
||||
- Binary schema–driven formats like Thrift, Protocol Buffers, and Avro allow compact, efficient encoding with clearly defined forward and backward compati‐ bility semantics. The schemas can be useful for documentation and code genera‐ tion in statically typed languages. However, they have the downside that data needs to be decoded before it is human-readable.
|
||||
|
||||
We also discussed several modes of dataflow, illustrating different scenarios in which data encodings are important:
|
||||
|
||||
- Databases, where the process writing to the database encodes the data and the process reading from the database decodes it
|
||||
- RPC and REST APIs, where the client encodes a request, the server decodes the request and encodes a response, and the client finally decodes the response
|
||||
- Asynchronous message passing (using message brokers or actors), where nodes communicate by sending each other messages that are encoded by the sender and decoded by the recipient
|
||||
|
||||
We can conclude that with a bit of care, backward/forward compatibility and rolling upgrades are quite achievable. May your application’s evolution be rapid and your deployments be frequent.
|
||||
|
||||
## References
|
||||
|
||||
--------------------
|
||||
|
||||
|
||||
1. “[Java Object Serialization Specification](http://docs.oracle.com/javase/7/docs/platform/serialization/spec/serialTOC.html),” *docs.oracle.com*, 2010.
|
||||
|
||||
1. “[Ruby 2.2.0 API Documentation](http://ruby-doc.org/core-2.2.0/),” *ruby-doc.org*, Dec 2014.
|
||||
|
||||
1. “[The Python 3.4.3 Standard Library Reference Manual](https://docs.python.org/3/library/pickle.html),” *docs.python.org*, February 2015.
|
||||
|
||||
1. “[EsotericSoftware/kryo](https://github.com/EsotericSoftware/kryo),” *github.com*, October 2014.
|
||||
|
||||
1. “[CWE-502: Deserialization of Untrusted Data](http://cwe.mitre.org/data/definitions/502.html),” Common Weakness Enumeration, *cwe.mitre.org*,
|
||||
July 30, 2014.
|
||||
|
||||
1. Steve Breen: “[What Do WebLogic, WebSphere, JBoss, Jenkins, OpenNMS, and Your Application Have in Common? This Vulnerability](http://foxglovesecurity.com/2015/11/06/what-do-weblogic-websphere-jboss-jenkins-opennms-and-your-application-have-in-common-this-vulnerability/),” *foxglovesecurity.com*, November 6, 2015.
|
||||
|
||||
1. Patrick McKenzie: “[What the Rails Security Issue Means for Your Startup](http://www.kalzumeus.com/2013/01/31/what-the-rails-security-issue-means-for-your-startup/),” *kalzumeus.com*, January 31, 2013.
|
||||
|
||||
1. Eishay Smith: “[jvm-serializers wiki](https://github.com/eishay/jvm-serializers/wiki),” *github.com*, November 2014.
|
||||
|
||||
1. “[XML Is a Poor Copy of S-Expressions](http://c2.com/cgi/wiki?XmlIsaPoorCopyOfEssExpressions),” *c2.com* wiki.
|
||||
|
||||
1. Matt Harris: “[Snowflake: An Update and Some Very Important Information](https://groups.google.com/forum/#!topic/twitter-development-talk/ahbvo3VTIYI),” email to *Twitter Development Talk* mailing list, October 19, 2010.
|
||||
|
||||
1. Shudi (Sandy) Gao, C. M. Sperberg-McQueen, and Henry S. Thompson: “[XML Schema 1.1](http://www.w3.org/XML/Schema),” W3C Recommendation, May 2001.
|
||||
|
||||
1. Francis Galiegue, Kris Zyp, and Gary Court: “[JSON Schema](http://json-schema.org/),” IETF Internet-Draft, February 2013.
|
||||
|
||||
1. Yakov Shafranovich: “[RFC 4180: Common Format and MIME Type for Comma-Separated Values (CSV) Files](https://tools.ietf.org/html/rfc4180),” October 2005.
|
||||
|
||||
1. “[MessagePack Specification](http://msgpack.org/),” *msgpack.org*. Mark Slee, Aditya Agarwal, and Marc Kwiatkowski: “[Thrift: Scalable Cross-Language Services Implementation](http://thrift.apache.org/static/files/thrift-20070401.pdf),” Facebook technical report, April 2007.
|
||||
|
||||
1. “[Protocol Buffers Developer Guide](https://developers.google.com/protocol-buffers/docs/overview),” Google, Inc., *developers.google.com*.
|
||||
|
||||
1. Igor Anishchenko: “[Thrift vs Protocol Buffers vs Avro - Biased Comparison](http://www.slideshare.net/IgorAnishchenko/pb-vs-thrift-vs-avro),” *slideshare.net*, September 17, 2012.
|
||||
|
||||
1. “[A Matrix of the Features Each Individual Language Library Supports](http://wiki.apache.org/thrift/LibraryFeatures),” *wiki.apache.org*.
|
||||
|
||||
1. Martin Kleppmann: “[Schema Evolution in Avro, Protocol Buffers and Thrift](http://martin.kleppmann.com/2012/12/05/schema-evolution-in-avro-protocol-buffers-thrift.html),” *martin.kleppmann.com*, December 5, 2012.
|
||||
|
||||
1. “[Apache Avro 1.7.7 Documentation](http://avro.apache.org/docs/1.7.7/),” *avro.apache.org*, July 2014.
|
||||
|
||||
1. Doug Cutting, Chad Walters, Jim Kellerman, et al.:
|
||||
“[[PROPOSAL] New Subproject: Avro](http://mail-archives.apache.org/mod_mbox/hadoop-general/200904.mbox/%3C49D53694.1050906@apache.org%3E),” email thread on *hadoop-general* mailing list,
|
||||
*mail-archives.apache.org*, April 2009.
|
||||
|
||||
1. Tony Hoare: “[Null References: The Billion Dollar Mistake](http://www.infoq.com/presentations/Null-References-The-Billion-Dollar-Mistake-Tony-Hoare),” at *QCon London*, March 2009.
|
||||
|
||||
1. Aditya Auradkar and Tom Quiggle: “[Introducing Espresso—LinkedIn's Hot New Distributed Document Store](https://engineering.linkedin.com/espresso/introducing-espresso-linkedins-hot-new-distributed-document-store),” *engineering.linkedin.com*, January 21, 2015.
|
||||
|
||||
1. Jay Kreps: “[Putting Apache Kafka to Use: A Practical Guide to Building a Stream Data Platform (Part 2)](http://blog.confluent.io/2015/02/25/stream-data-platform-2/),” *blog.confluent.io*, February 25, 2015.
|
||||
|
||||
1. Gwen Shapira: “[The Problem of Managing Schemas](http://radar.oreilly.com/2014/11/the-problem-of-managing-schemas.html),” *radar.oreilly.com*, November 4, 2014.
|
||||
|
||||
1. “[Apache Pig 0.14.0 Documentation](http://pig.apache.org/docs/r0.14.0/),” *pig.apache.org*, November 2014.
|
||||
|
||||
1. John Larmouth: [*ASN.1Complete*](http://www.oss.com/asn1/resources/books-whitepapers-pubs/larmouth-asn1-book.pdf). Morgan Kaufmann, 1999. ISBN: 978-0-122-33435-1
|
||||
|
||||
1. Russell Housley, Warwick Ford, Tim Polk, and David Solo: “[RFC 2459: Internet X.509 Public Key Infrastructure: Certificate and CRL Profile](https://www.ietf.org/rfc/rfc2459.txt),” IETF Network Working Group, Standards Track,
|
||||
January 1999.
|
||||
|
||||
1. Lev Walkin: “[Question: Extensibility and Dropping Fields](http://lionet.info/asn1c/blog/2010/09/21/question-extensibility-removing-fields/),” *lionet.info*, September 21, 2010.
|
||||
|
||||
1. Jesse James Garrett: “[Ajax: A New Approach to Web Applications](http://www.adaptivepath.com/ideas/ajax-new-approach-web-applications/),” *adaptivepath.com*, February 18, 2005.
|
||||
|
||||
1. Sam Newman: *Building Microservices*. O'Reilly Media, 2015. ISBN: 978-1-491-95035-7
|
||||
|
||||
1. Chris Richardson: “[Microservices: Decomposing Applications for Deployability and Scalability](http://www.infoq.com/articles/microservices-intro),” *infoq.com*, May 25, 2014.
|
||||
|
||||
1. Pat Helland: “[Data on the Outside Versus Data on the Inside](http://cidrdb.org/cidr2005/papers/P12.pdf),” at *2nd Biennial Conference on Innovative Data Systems Research* (CIDR), January 2005.
|
||||
|
||||
1. Roy Thomas Fielding: “[Architectural Styles and the Design of Network-Based Software Architectures](https://www.ics.uci.edu/~fielding/pubs/dissertation/fielding_dissertation.pdf),” PhD Thesis, University of California, Irvine, 2000.
|
||||
|
||||
1. Roy Thomas Fielding: “[REST APIs Must Be Hypertext-Driven](http://roy.gbiv.com/untangled/2008/rest-apis-must-be-hypertext-driven),” *roy.gbiv.com*, October 20 2008.
|
||||
|
||||
1. “[REST in Peace, SOAP](http://royal.pingdom.com/2010/10/15/rest-in-peace-soap/),” *royal.pingdom.com*, October 15, 2010.
|
||||
|
||||
1. “[Web Services Standards as of Q1 2007](https://www.innoq.com/resources/ws-standards-poster/),” *innoq.com*, February 2007.
|
||||
|
||||
1. Pete Lacey: “[The S Stands for Simple](http://harmful.cat-v.org/software/xml/soap/simple),” *harmful.cat-v.org*, November 15, 2006.
|
||||
|
||||
1. Stefan Tilkov: “[Interview: Pete Lacey Criticizes Web Services](http://www.infoq.com/articles/pete-lacey-ws-criticism),” *infoq.com*, December 12, 2006.
|
||||
|
||||
1. “[OpenAPI Specification (fka Swagger RESTful API Documentation Specification) Version 2.0](http://swagger.io/specification/),” *swagger.io*, September 8, 2014.
|
||||
|
||||
1. Michi Henning: “[The Rise and Fall of CORBA](http://queue.acm.org/detail.cfm?id=1142044),” *ACM Queue*, volume 4, number 5, pages 28–34, June 2006.
|
||||
[doi:10.1145/1142031.1142044](http://dx.doi.org/10.1145/1142031.1142044)
|
||||
|
||||
1. Andrew D. Birrell and Bruce Jay Nelson: “[Implementing Remote Procedure Calls](http://www.cs.princeton.edu/courses/archive/fall03/cs518/papers/rpc.pdf),” *ACM Transactions on Computer Systems* (TOCS), volume 2, number 1, pages 39–59, February 1984. [doi:10.1145/2080.357392](http://dx.doi.org/10.1145/2080.357392)
|
||||
|
||||
1. Jim Waldo, Geoff Wyant, Ann Wollrath, and Sam Kendall: “[A Note on Distributed Computing](http://m.mirror.facebook.net/kde/devel/smli_tr-94-29.pdf),” Sun Microsystems Laboratories, Inc., Technical Report TR-94-29, November 1994.
|
||||
|
||||
1. Steve Vinoski: “[Convenience over Correctness](http://steve.vinoski.net/pdf/IEEE-Convenience_Over_Correctness.pdf),” *IEEE Internet Computing*, volume 12, number 4, pages 89–92, July 2008. [doi:10.1109/MIC.2008.75](http://dx.doi.org/10.1109/MIC.2008.75)
|
||||
|
||||
1. Marius Eriksen: “[Your Server as a Function](http://monkey.org/~marius/funsrv.pdf),” at *7th Workshop on Programming Languages and Operating Systems* (PLOS), November 2013. [doi:10.1145/2525528.2525538](http://dx.doi.org/10.1145/2525528.2525538)
|
||||
|
||||
1. “[grpc-common Documentation](https://github.com/grpc/grpc-common),” Google, Inc., *github.com*, February 2015.
|
||||
|
||||
1. Aditya Narayan and Irina Singh: “[Designing and Versioning Compatible Web Services](http://www.ibm.com/developerworks/websphere/library/techarticles/0705_narayan/0705_narayan.html),” *ibm.com*, March 28, 2007.
|
||||
|
||||
1. Troy Hunt: “[Your API Versioning Is Wrong, Which Is Why I Decided to Do It 3 Different Wrong Ways](http://www.troyhunt.com/2014/02/your-api-versioning-is-wrong-which-is.html),” *troyhunt.com*, February 10, 2014.
|
||||
|
||||
1. “[API Upgrades](https://stripe.com/docs/upgrades),” Stripe, Inc., April 2015.
|
||||
|
||||
1. Jonas Bonér: “[Upgrade in an Akka Cluster](http://grokbase.com/t/gg/akka-user/138wd8j9e3/upgrade-in-an-akka-cluster),” email to *akka-user* mailing list, *grokbase.com*, August 28, 2013.
|
||||
|
||||
1. Philip A. Bernstein, Sergey Bykov, Alan Geller, et al.: “[Orleans: Distributed Virtual Actors for Programmability and Scalability](http://research.microsoft.com/pubs/210931/Orleans-MSR-TR-2014-41.pdf),” Microsoft Research Technical Report MSR-TR-2014-41, March 2014.
|
||||
|
||||
1. “[Microsoft Project Orleans Documentation](http://dotnet.github.io/orleans/),” Microsoft Research, *dotnet.github.io*, 2015.
|
||||
|
||||
1. David Mercer, Sean Hinde, Yinso Chen, and Richard A O'Keefe: “[beginner: Updating Data Structures](http://erlang.org/pipermail/erlang-questions/2007-October/030318.html),” email thread on *erlang-questions* mailing list, *erlang.com*, October 29, 2007.
|
||||
|
||||
1. Fred Hebert: “[Postscript: Maps](http://learnyousomeerlang.com/maps),” *learnyousomeerlang.com*, April 9, 2014.
|
226
en-us/ch5.md
Normal file
226
en-us/ch5.md
Normal file
@ -0,0 +1,226 @@
|
||||
# 5. Replication
|
||||
|
||||

|
||||
|
||||
> *The major difference between a thing that might go wrong and a thing that cannot possibly go wrong is that when a thing that cannot possibly go wrong goes wrong it usually turns out to be impossible to get at or repair.*
|
||||
>
|
||||
> — Douglas Adams, *Mostly Harmless* (1992)
|
||||
|
||||
------
|
||||
|
||||
In [Part I](part-i.md) of this book, we discussed aspects of data systems that apply when data is stored on a single machine. Now, in [Part II](part-ii.md), we move up a level and ask: what happens if multiple machines are involved in storage and retrieval of data?
|
||||
|
||||
There are various reasons why you might want to distribute a database across multi‐ ple machines:
|
||||
|
||||
***Scalability***
|
||||
|
||||
If your data volume, read load, or write load grows bigger than a single machine can handle, you can potentially spread the load across multiple machines.
|
||||
|
||||
***Fault tolerance/high availability***
|
||||
|
||||
If your application needs to continue working even if one machine (or several machines, or the network, or an entire datacenter) goes down, you can use multi‐ ple machines to give you redundancy. When one fails, another one can take over.
|
||||
|
||||
***Latency***
|
||||
|
||||
If you have users around the world, you might want to have servers at various locations worldwide so that each user can be served from a datacenter that is geo‐ graphically close to them. That avoids the users having to wait for network pack‐ ets to travel halfway around the world.
|
||||
|
||||
|
||||
|
||||
## ……
|
||||
|
||||
|
||||
|
||||
## Summary
|
||||
|
||||
In this chapter we looked at the issue of replication. Replication can serve several purposes:
|
||||
|
||||
***High availability***
|
||||
|
||||
Keeping the system running, even when one machine (or several machines, or an entire datacenter) goes down
|
||||
|
||||
***Disconnected operation***
|
||||
|
||||
Allowing an application to continue working when there is a network interrup‐ tion
|
||||
|
||||
***Latency***
|
||||
|
||||
Placing data geographically close to users, so that users can interact with it faster
|
||||
|
||||
***Scalability***
|
||||
|
||||
Being able to handle a higher volume of reads than a single machine could han‐ dle, by performing reads on replicas
|
||||
|
||||
|
||||
|
||||
Despite being a simple goal—keeping a copy of the same data on several machines— replication turns out to be a remarkably tricky problem. It requires carefully thinking about concurrency and about all the things that can go wrong, and dealing with the consequences of those faults. At a minimum, we need to deal with unavailable nodes and network interruptions (and that’s not even considering the more insidious kinds of fault, such as silent data corruption due to software bugs).
|
||||
|
||||
We discussed three main approaches to replication:
|
||||
|
||||
***Single-leader replication***
|
||||
|
||||
Clients send all writes to a single node (the leader), which sends a stream of data change events to the other replicas (followers). Reads can be performed on any replica, but reads from followers might be stale.
|
||||
|
||||
***Multi-leader replication***
|
||||
|
||||
Clients send each write to one of several leader nodes, any of which can accept writes. The leaders send streams of data change events to each other and to any follower nodes.
|
||||
|
||||
***Leaderless replication***
|
||||
|
||||
Clients send each write to several nodes, and read from several nodes in parallel in order to detect and correct nodes with stale data.
|
||||
|
||||
Each approach has advantages and disadvantages. Single-leader replication is popular because it is fairly easy to understand and there is no conflict resolution to worry about. Multi-leader and leaderless replication can be more robust in the presence of faulty nodes, network interruptions, and latency spikes—at the cost of being harder to reason about and providing only very weak consistency guarantees.
|
||||
|
||||
Replication can be synchronous or asynchronous, which has a profound effect on the system behavior when there is a fault. Although asynchronous replication can be fast when the system is running smoothly, it’s important to figure out what happens when replication lag increases and servers fail. If a leader fails and you promote an asynchronously updated follower to be the new leader, recently committed data may be lost.
|
||||
|
||||
We looked at some strange effects that can be caused by replication lag, and we dis‐ cussed a few consistency models which are helpful for deciding how an application should behave under replication lag:
|
||||
|
||||
***Read-after-write consistency***
|
||||
|
||||
Users should always see data that they submitted themselves.
|
||||
|
||||
***Monotonic reads***
|
||||
|
||||
After users have seen the data at one point in time, they shouldn’t later see the data from some earlier point in time.
|
||||
|
||||
***Consistent prefix reads***
|
||||
|
||||
Users should see the data in a state that makes causal sense: for example, seeing a question and its reply in the correct order.
|
||||
|
||||
Finally, we discussed the concurrency issues that are inherent in multi-leader and leaderless replication approaches: because they allow multiple writes to happen con‐ currently, conflicts may occur. We examined an algorithm that a database might use to determine whether one operation happened before another, or whether they hap‐ pened concurrently. We also touched on methods for resolving conflicts by merging together concurrent updates.
|
||||
|
||||
In the next chapter we will continue looking at data that is distributed across multiple machines, through the counterpart of replication: splitting a large dataset into *partitions*.
|
||||
|
||||
|
||||
|
||||
## References
|
||||
--------------------
|
||||
|
||||
1. Bruce G. Lindsay, Patricia Griffiths Selinger, C. Galtieri, et al.:
|
||||
“[Notes on Distributed Databases](http://domino.research.ibm.com/library/cyberdig.nsf/papers/A776EC17FC2FCE73852579F100578964/$File/RJ2571.pdf),” IBM Research, Research Report RJ2571(33471), July 1979.
|
||||
|
||||
1. “[Oracle Active Data Guard Real-Time Data Protection and Availability](http://www.oracle.com/technetwork/database/availability/active-data-guard-wp-12c-1896127.pdf),” Oracle White Paper, June 2013.
|
||||
|
||||
1. “[AlwaysOn Availability Groups](http://msdn.microsoft.com/en-us/library/hh510230.aspx),” in *SQL Server Books Online*, Microsoft, 2012.
|
||||
|
||||
1. Lin Qiao, Kapil Surlaker, Shirshanka Das, et al.: “[On Brewing Fresh Espresso: LinkedIn’s Distributed Data Serving Platform](http://www.slideshare.net/amywtang/espresso-20952131),” at *ACM International Conference on Management of Data* (SIGMOD), June 2013.
|
||||
|
||||
1. Jun Rao: “[Intra-Cluster Replication for Apache Kafka](http://www.slideshare.net/junrao/kafka-replication-apachecon2013),” at *ApacheCon North America*, February 2013.
|
||||
|
||||
1. “[Highly Available Queues](https://www.rabbitmq.com/ha.html),” in *RabbitMQ Server Documentation*, Pivotal Software, Inc., 2014.
|
||||
|
||||
1. Yoshinori Matsunobu: “[Semi-Synchronous Replication at Facebook](http://yoshinorimatsunobu.blogspot.co.uk/2014/04/semi-synchronous-replication-at-facebook.html),” *yoshinorimatsunobu.blogspot.co.uk*, April 1, 2014.
|
||||
|
||||
1. Robbert van Renesse and Fred B. Schneider: “[Chain Replication for Supporting High Throughput and Availability](http://static.usenix.org/legacy/events/osdi04/tech/full_papers/renesse/renesse.pdf),” at *6th USENIX Symposium on Operating System Design and Implementation* (OSDI), December 2004.
|
||||
|
||||
1. Jeff Terrace and Michael J. Freedman: “[Object Storage on CRAQ: High-Throughput Chain Replication for Read-Mostly Workloads](https://www.usenix.org/legacy/event/usenix09/tech/full_papers/terrace/terrace.pdf),” at *USENIX Annual Technical Conference* (ATC), June 2009.
|
||||
|
||||
1. Brad Calder, Ju Wang, Aaron Ogus, et al.: “[Windows Azure Storage: A Highly Available Cloud Storage Service with Strong Consistency](http://sigops.org/sosp/sosp11/current/2011-Cascais/printable/11-calder.pdf),” at *23rd ACM Symposium on Operating Systems Principles* (SOSP), October 2011.
|
||||
|
||||
1. Andrew Wang: “[Windows Azure Storage](http://umbrant.com/blog/2016/windows_azure_storage.html),” *umbrant.com*, February 4, 2016.
|
||||
|
||||
1. “[Percona Xtrabackup - Documentation](https://www.percona.com/doc/percona-xtrabackup/2.1/index.html),” Percona LLC, 2014.
|
||||
|
||||
1. Jesse Newland: “[GitHub Availability This Week](https://github.com/blog/1261-github-availability-this-week),” *github.com*, September 14, 2012.
|
||||
|
||||
1. Mark Imbriaco: “[Downtime Last Saturday](https://github.com/blog/1364-downtime-last-saturday),” *github.com*, December 26, 2012.
|
||||
|
||||
1. John Hugg: “[‘All in’ with Determinism for Performance and Testing in Distributed Systems](https://www.youtube.com/watch?v=gJRj3vJL4wE),” at *Strange Loop*, September 2015. Amit Kapila: “[WAL Internals of PostgreSQL](http://www.pgcon.org/2012/schedule/attachments/258_212_Internals%20Of%20PostgreSQL%20Wal.pdf),” at *PostgreSQL Conference* (PGCon), May 2012.
|
||||
|
||||
1. [*MySQL Internals Manual*](http://dev.mysql.com/doc/internals/en/index.html). Oracle, 2014.
|
||||
|
||||
1. Yogeshwer Sharma, Philippe Ajoux, Petchean Ang, et al.: “[Wormhole: Reliable Pub-Sub to Support Geo-Replicated Internet Services](https://www.usenix.org/system/files/conference/nsdi15/nsdi15-paper-sharma.pdf),” at *12th USENIX Symposium on Networked Systems Design and Implementation* (NSDI), May 2015.
|
||||
|
||||
1. “[Oracle GoldenGate 12c: Real-Time Access to Real-Time Information](http://www.oracle.com/us/products/middleware/data-integration/oracle-goldengate-realtime-access-2031152.pdf),” Oracle White Paper, October 2013.
|
||||
|
||||
1. Shirshanka Das, Chavdar Botev, Kapil Surlaker, et al.: “[All Aboard the Databus!](http://www.socc2012.org/s18-das.pdf),” at
|
||||
*ACM Symposium on Cloud Computing* (SoCC), October 2012.
|
||||
|
||||
1. Greg Sabino Mullane: “[Version 5 of Bucardo Database Replication System](http://blog.endpoint.com/2014/06/bucardo-5-multimaster-postgres-released.html),” *blog.endpoint.com*, June 23, 2014.
|
||||
|
||||
1. Werner Vogels: “[Eventually Consistent](http://queue.acm.org/detail.cfm?id=1466448),” *ACM Queue*, volume 6, number 6, pages 14–19, October 2008.
|
||||
[doi:10.1145/1466443.1466448](http://dx.doi.org/10.1145/1466443.1466448)
|
||||
|
||||
1. Douglas B. Terry: “[Replicated Data Consistency Explained Through Baseball](http://research.microsoft.com/pubs/157411/ConsistencyAndBaseballReport.pdf),” Microsoft Research, Technical Report MSR-TR-2011-137, October 2011.
|
||||
|
||||
1. Douglas B. Terry, Alan J. Demers, Karin Petersen, et al.: “[Session Guarantees for Weakly Consistent Replicated Data](http://citeseerx.ist.psu.edu/viewdoc/download?doi=10.1.1.71.2269&rep=rep1&type=pdf),” at *3rd International Conference on Parallel and Distributed Information Systems* (PDIS), September 1994. [doi:10.1109/PDIS.1994.331722](http://dx.doi.org/10.1109/PDIS.1994.331722)
|
||||
|
||||
1. Terry Pratchett: *Reaper Man: A Discworld Novel*. Victor Gollancz, 1991. ISBN: 978-0-575-04979-6
|
||||
|
||||
1. “[Tungsten Replicator](http://tungsten-replicator.org/),” Continuent, Inc., 2014.
|
||||
|
||||
1. “[BDR 0.10.0 Documentation](http://bdr-project.org/docs/next/index.html),” The PostgreSQL Global Development Group, *bdr-project.org*, 2015.
|
||||
|
||||
1. Robert Hodges:
|
||||
“[If You *Must* Deploy Multi-Master Replication, Read This First](http://scale-out-blog.blogspot.co.uk/2012/04/if-you-must-deploy-multi-master.html),” *scale-out-blog.blogspot.co.uk*,
|
||||
March 30, 2012.
|
||||
|
||||
1. J. Chris Anderson, Jan Lehnardt, and Noah Slater: *CouchDB: The Definitive Guide*. O'Reilly Media, 2010.
|
||||
ISBN: 978-0-596-15589-6
|
||||
|
||||
1. AppJet, Inc.: “[Etherpad and EasySync Technical Manual](https://github.com/ether/etherpad-lite/blob/e2ce9dc/doc/easysync/easysync-full-description.pdf),” *github.com*, March 26, 2011.
|
||||
|
||||
1. John Day-Richter: “[What’s Different About the New Google Docs: Making Collaboration Fast](http://googledrive.blogspot.com/2010/09/whats-different-about-new-google-docs.html),” *googledrive.blogspot.com*, 23 September 2010.
|
||||
|
||||
1. Martin Kleppmann and Alastair R. Beresford: “[A Conflict-Free Replicated JSON Datatype](http://arxiv.org/abs/1608.03960),”
|
||||
arXiv:1608.03960, August 13, 2016.
|
||||
|
||||
1. Frazer Clement: “[Eventual Consistency – Detecting Conflicts](http://messagepassing.blogspot.co.uk/2011/10/eventual-consistency-detecting.html),” *messagepassing.blogspot.co.uk*, October 20, 2011.
|
||||
|
||||
1. Robert Hodges: “[State of the Art for MySQL Multi-Master Replication](https://www.percona.com/live/mysql-conference-2013/sessions/state-art-mysql-multi-master-replication),” at *Percona Live: MySQL Conference & Expo*, April 2013.
|
||||
|
||||
1. John Daily: “[Clocks Are Bad, or, Welcome to the Wonderful World of Distributed Systems](http://basho.com/clocks-are-bad-or-welcome-to-distributed-systems/),” *basho.com*, November 12, 2013.
|
||||
|
||||
1. Riley Berton: “[Is Bi-Directional Replication (BDR) in Postgres Transactional?](http://sdf.org/~riley/blog/2016/01/04/is-bi-directional-replication-bdr-in-postgres-transactional/),” *sdf.org*, January 4, 2016.
|
||||
|
||||
1. Giuseppe DeCandia, Deniz Hastorun, Madan Jampani, et al.: “[Dynamo: Amazon's Highly Available Key-Value Store](http://www.allthingsdistributed.com/files/amazon-dynamo-sosp2007.pdf),” at *21st ACM Symposium on Operating Systems Principles* (SOSP), October 2007.
|
||||
|
||||
1. Marc Shapiro, Nuno Preguiça, Carlos Baquero, and Marek Zawirski: “[A Comprehensive Study of Convergent and Commutative Replicated Data Types](http://hal.inria.fr/inria-00555588/),” INRIA Research Report no. 7506,
|
||||
January 2011.
|
||||
|
||||
1. Sam Elliott: “[CRDTs: An UPDATE (or Maybe Just a PUT)](https://speakerdeck.com/lenary/crdts-an-update-or-just-a-put),” at *RICON West*, October 2013.
|
||||
|
||||
1. Russell Brown: “[A Bluffers Guide to CRDTs in Riak](https://gist.github.com/russelldb/f92f44bdfb619e089a4d),” *gist.github.com*, October 28, 2013.
|
||||
|
||||
1. Benjamin Farinier, Thomas Gazagnaire, and Anil Madhavapeddy: “[Mergeable Persistent Data Structures](http://gazagnaire.org/pub/FGM15.pdf),” at *26es Journées Francophones des Langages Applicatifs* (JFLA), January 2015.
|
||||
|
||||
1. Chengzheng Sun and Clarence Ellis: “[Operational Transformation in Real-Time Group Editors: Issues, Algorithms, and Achievements](http://citeseerx.ist.psu.edu/viewdoc/download?doi=10.1.1.53.933&rep=rep1&type=pdf),” at *ACM Conference on Computer Supported Cooperative Work* (CSCW), November 1998.
|
||||
|
||||
1. Lars Hofhansl: “[HBASE-7709: Infinite Loop Possible in Master/Master Replication](https://issues.apache.org/jira/browse/HBASE-7709),” *issues.apache.org*, January 29, 2013.
|
||||
|
||||
1. David K. Gifford: “[Weighted Voting for Replicated Data](http://citeseerx.ist.psu.edu/viewdoc/summary?doi=10.1.1.84.7698),” at *7th ACM Symposium on Operating Systems Principles* (SOSP), December 1979. [doi:10.1145/800215.806583](http://dx.doi.org/10.1145/800215.806583)
|
||||
|
||||
1. Heidi Howard, Dahlia Malkhi, and Alexander Spiegelman: “[Flexible Paxos: Quorum Intersection Revisited](https://arxiv.org/abs/1608.06696),” *arXiv:1608.06696*, August 24, 2016.
|
||||
|
||||
1. Joseph Blomstedt: “[Re: Absolute Consistency](http://lists.basho.com/pipermail/riak-users_lists.basho.com/2012-January/007157.html),” email to *riak-users* mailing list, *lists.basho.com*,
|
||||
January 11, 2012.
|
||||
|
||||
1. Joseph Blomstedt: “[Bringing Consistency to Riak](https://vimeo.com/51973001),” at *RICON West*, October 2012.
|
||||
|
||||
1. Peter Bailis, Shivaram Venkataraman, Michael J. Franklin, et al.: “[Quantifying Eventual Consistency with PBS](http://www.bailis.org/papers/pbs-cacm2014.pdf),” *Communications of the ACM*, volume 57, number 8, pages 93–102, August 2014. [doi:10.1145/2632792](http://dx.doi.org/10.1145/2632792)
|
||||
|
||||
1. Jonathan Ellis: “[Modern Hinted Handoff](http://www.datastax.com/dev/blog/modern-hinted-handoff),” *datastax.com*, December 11, 2012.
|
||||
|
||||
1. “[Project Voldemort Wiki](https://github.com/voldemort/voldemort/wiki),” *github.com*, 2013.
|
||||
|
||||
1. “[Apache Cassandra 2.0 Documentation](http://www.datastax.com/documentation/cassandra/2.0/index.html),” DataStax, Inc., 2014.
|
||||
|
||||
1. “[Riak Enterprise: Multi-Datacenter Replication](http://basho.com/assets/MultiDatacenter_Replication.pdf).” Technical whitepaper, Basho Technologies, Inc.,
|
||||
September 2014.
|
||||
|
||||
1. Jonathan Ellis: “[Why Cassandra Doesn't Need Vector Clocks](http://www.datastax.com/dev/blog/why-cassandra-doesnt-need-vector-clocks),” *datastax.com*, September 2, 2013.
|
||||
|
||||
1. Leslie Lamport: “[Time, Clocks, and the Ordering of Events in a Distributed System](http://research.microsoft.com/en-US/um/people/Lamport/pubs/time-clocks.pdf),” *Communications of the ACM*, volume 21, number 7, pages 558–565, July 1978. [doi:10.1145/359545.359563](http://dx.doi.org/10.1145/359545.359563)
|
||||
|
||||
1. Joel Jacobson: “[Riak 2.0: Data Types](http://blog.joeljacobson.com/riak-2-0-data-types/),” *blog.joeljacobson.com*, March 23, 2014.
|
||||
|
||||
1. D. Stott Parker Jr., Gerald J. Popek, Gerard Rudisin, et al.: “[Detection of Mutual Inconsistency in Distributed Systems](http://zoo.cs.yale.edu/classes/cs426/2013/bib/parker83detection.pdf),” *IEEE Transactions on Software Engineering*, volume 9, number 3, pages 240–247, May 1983. [doi:10.1109/TSE.1983.236733](http://dx.doi.org/10.1109/TSE.1983.236733)
|
||||
|
||||
1. Nuno Preguiça, Carlos Baquero, Paulo Sérgio Almeida, et al.: “[Dotted Version Vectors: Logical Clocks for Optimistic Replication](http://arxiv.org/pdf/1011.5808v1.pdf),” arXiv:1011.5808, November 26, 2010.
|
||||
|
||||
1. Sean Cribbs: “[A Brief History of Time in Riak](https://www.youtube.com/watch?v=HHkKPdOi-ZU),” at *RICON*, October 2014.
|
||||
|
||||
1. Russell Brown: “[Vector Clocks Revisited Part 2: Dotted Version Vectors](http://basho.com/posts/technical/vector-clocks-revisited-part-2-dotted-version-vectors/),” *basho.com*, November 10, 2015.
|
||||
|
||||
1. Carlos Baquero: “[Version Vectors Are Not Vector Clocks](https://haslab.wordpress.com/2011/07/08/version-vectors-are-not-vector-clocks/),” *haslab.wordpress.com*, July 8, 2011.
|
||||
|
||||
1. Reinhard Schwarz and Friedemann Mattern: “[Detecting Causal Relationships in Distributed Computations: In Search of the Holy Grail](http://dcg.ethz.ch/lectures/hs08/seminar/papers/mattern4.pdf),” *Distributed Computing*, volume 7, number 3, pages 149–174, March 1994. [doi:10.1007/BF02277859](http://dx.doi.org/10.1007/BF02277859)
|
139
en-us/ch6.md
Normal file
139
en-us/ch6.md
Normal file
@ -0,0 +1,139 @@
|
||||
# 6. Partitioning
|
||||
|
||||

|
||||
|
||||
> *Clearly, we must break away from the sequential and not limit the computers. We must state definitions and provide for priorities and descriptions of data. We must state relation‐ ships, not procedures.*
|
||||
>
|
||||
> — Grace Murray Hopper, *Management and the Computer of the Future* (1962)
|
||||
|
||||
-------------
|
||||
|
||||
|
||||
|
||||
In [Chapter 5](ch5.md) we discussed replication—that is, having multiple copies of the same data on different nodes. For very large datasets, or very high query throughput, that is not sufficient: we need to break the data up into *partitions*, also known as *sharding*.[^i]
|
||||
|
||||
[^i]: Partitioning, as discussed in this chapter, is a way of intentionally breaking a large database down into smaller ones. It has nothing to do with *network partitions* (netsplits), a type of fault in the network between nodes. We will discuss such faults in [Chapter 8](ch8.md).
|
||||
|
||||
> #### Terminological confusion
|
||||
>
|
||||
> What we call a ***partition*** here is called a ***shard*** in MongoDB, Elasticsearch, and SolrCloud; it’s known as a ***region*** in HBase, a ***tablet*** in Bigtable, a ***vnode*** in Cassandra and Riak, and a ***vBucket*** in Couchbase. However, ***partitioning*** is the most established term, so we’ll stick with that.
|
||||
>
|
||||
|
||||
Normally, partitions are defined in such a way that each piece of data (each record, row, or document) belongs to exactly one partition. There are various ways of achiev‐ ing this, which we discuss in depth in this chapter. In effect, each partition is a small database of its own, although the database may support operations that touch multi‐ ple partitions at the same time.
|
||||
|
||||
The main reason for wanting to partition data is *scalability*. Different partitions can be placed on different nodes in a shared-nothing cluster (see the introduction to [Part II](part-ii.md) for a definition of *shared nothing*). Thus, a large dataset can be distributed across many disks, and the query load can be distributed across many processors.
|
||||
|
||||
For queries that operate on a single partition, each node can independently execute the queries for its own partition, so query throughput can be scaled by adding more nodes. Large, complex queries can potentially be parallelized across many nodes, although this gets significantly harder.
|
||||
|
||||
Partitioned databases were pioneered in the 1980s by products such as Teradata and Tandem NonStop SQL [1], and more recently rediscovered by NoSQL databases and Hadoop-based data warehouses. Some systems are designed for transactional work‐ loads, and others for analytics (see “[Transaction Processing or Analytics?](ch3.md#transaction-processing-or-analytics?)”): this difference affects how the system is tuned, but the fundamentals of partitioning apply to both kinds of workloads.
|
||||
|
||||
In this chapter we will first look at different approaches for partitioning large datasets and observe how the indexing of data interacts with partitioning. We’ll then talk about rebalancing, which is necessary if you want to add or remove nodes in your cluster. Finally, we’ll get an overview of how databases route requests to the right partitions and execute queries.
|
||||
|
||||
|
||||
## ……
|
||||
|
||||
|
||||
|
||||
## Summary
|
||||
|
||||
In this chapter we explored different ways of partitioning a large dataset into smaller subsets. Partitioning is necessary when you have so much data that storing and pro‐ cessing it on a single machine is no longer feasible.
|
||||
|
||||
The goal of partitioning is to spread the data and query load evenly across multiple machines, avoiding hot spots (nodes with disproportionately high load). This requires choosing a partitioning scheme that is appropriate to your data, and reba‐ lancing the partitions when nodes are added to or removed from the cluster.
|
||||
|
||||
We discussed two main approaches to partitioning:
|
||||
|
||||
* ***Key range partitioning***, where keys are sorted, and a partition owns all the keys from some minimum up to some maximum. Sorting has the advantage that effi‐ cient range queries are possible, but there is a risk of hot spots if the application often accesses keys that are close together in the sorted order.
|
||||
|
||||
In this approach, partitions are typically rebalanced dynamically by splitting the range into two subranges when a partition gets too big.
|
||||
|
||||
* ***Hash partitioning***, where a hash function is applied to each key, and a partition owns a range of hashes. This method destroys the ordering of keys, making range queries inefficient, but may distribute load more evenly.
|
||||
|
||||
When partitioning by hash, it is common to create a fixed number of partitions in advance, to assign several partitions to each node, and to move entire parti‐ tions from one node to another when nodes are added or removed. Dynamic partitioning can also be used.
|
||||
|
||||
Hybrid approaches are also possible, for example with a compound key: using one part of the key to identify the partition and another part for the sort order.
|
||||
|
||||
We also discussed the interaction between partitioning and secondary indexes. A sec‐ ondary index also needs to be partitioned, and there are two methods:
|
||||
|
||||
* ***Document-partitioned indexes*** (local indexes), where the secondary indexes are stored in the same partition as the primary key and value. This means that only a single partition needs to be updated on write, but a read of the secondary index requires a scatter/gather across all partitions.
|
||||
|
||||
* ***Term-partitioned indexes*** (global indexes), where the secondary indexes are partitioned separately, using the indexed values. An entry in the secondary index may include records from all partitions of the primary key. When a document is writ‐ ten, several partitions of the secondary index need to be updated; however, a read can be served from a single partition.
|
||||
|
||||
Finally, we discussed techniques for routing queries to the appropriate partition, which range from simple partition-aware load balancing to sophisticated parallel query execution engines.
|
||||
|
||||
By design, every partition operates mostly independently—that’s what allows a parti‐ tioned database to scale to multiple machines. However, operations that need to write to several partitions can be difficult to reason about: for example, what happens if the write to one partition succeeds, but another fails? We will address that question in the following chapters.
|
||||
|
||||
|
||||
|
||||
## References
|
||||
--------------------
|
||||
|
||||
1. David J. DeWitt and Jim N. Gray: “[Parallel Database Systems: The Future of High Performance Database Systems](),”
|
||||
*Communications of the ACM*, volume 35, number 6, pages 85–98, June 1992. [doi:10.1145/129888.129894](http://dx.doi.org/10.1145/129888.129894)
|
||||
|
||||
2. Lars George: “[HBase vs. BigTable Comparison](http://www.larsgeorge.com/2009/11/hbase-vs-bigtable-comparison.html),” *larsgeorge.com*, November 2009.
|
||||
|
||||
3. “[The Apache HBase Reference Guide](https://hbase.apache.org/book/book.html),” Apache Software Foundation, *hbase.apache.org*, 2014.
|
||||
|
||||
4. MongoDB, Inc.: “[New Hash-Based Sharding Feature in MongoDB 2.4](http://blog.mongodb.org/post/47633823714/new-hash-based-sharding-feature-in-mongodb-24),” *blog.mongodb.org*, April 10, 2013.
|
||||
|
||||
5. Ikai Lan: “[App Engine Datastore Tip: Monotonically Increasing Values Are Bad](http://ikaisays.com/2011/01/25/app-engine-datastore-tip-monotonically-increasing-values-are-bad/),” *ikaisays.com*,
|
||||
January 25, 2011.
|
||||
|
||||
6. Martin Kleppmann: “[Java's hashCode Is Not Safe for Distributed Systems](http://martin.kleppmann.com/2012/06/18/java-hashcode-unsafe-for-distributed-systems.html),” *martin.kleppmann.com*, June 18, 2012.
|
||||
|
||||
7. David Karger, Eric Lehman, Tom Leighton, et al.: “[Consistent Hashing and Random Trees: Distributed Caching Protocols for Relieving Hot Spots on the World Wide Web](http://www.akamai.com/dl/technical_publications/ConsistenHashingandRandomTreesDistributedCachingprotocolsforrelievingHotSpotsontheworldwideweb.pdf),” at *29th Annual ACM Symposium on Theory of Computing* (STOC), pages 654–663, 1997. [doi:10.1145/258533.258660](http://dx.doi.org/10.1145/258533.258660)
|
||||
|
||||
8. John Lamping and Eric Veach: “[A Fast, Minimal Memory, Consistent Hash Algorithm](http://arxiv.org/pdf/1406.2294v1.pdf),” *arxiv.org*, June 2014.
|
||||
|
||||
9. Eric Redmond: “[A Little Riak Book](http://littleriakbook.com/),” Version 1.4.0, Basho Technologies, September 2013.
|
||||
|
||||
10. “[Couchbase 2.5 Administrator Guide](http://docs.couchbase.com/couchbase-manual-2.5/cb-admin/),” Couchbase, Inc., 2014.
|
||||
|
||||
11. Avinash Lakshman and Prashant Malik: “[Cassandra – A Decentralized Structured Storage System](http://www.cs.cornell.edu/Projects/ladis2009/papers/Lakshman-ladis2009.PDF),” at *3rd ACM SIGOPS International Workshop on
|
||||
Large Scale Distributed Systems and Middleware* (LADIS), October 2009.
|
||||
|
||||
12. Jonathan Ellis: “[Facebook’s Cassandra Paper, Annotated and Compared to Apache Cassandra 2.0](http://www.datastax.com/documentation/articles/cassandra/cassandrathenandnow.html),”
|
||||
*datastax.com*, September 12, 2013.
|
||||
|
||||
13. “[Introduction to Cassandra Query Language](http://www.datastax.com/documentation/cql/3.1/cql/cql_intro_c.html),” DataStax, Inc., 2014.
|
||||
|
||||
14. Samuel Axon: “[3% of Twitter's Servers Dedicated to Justin Bieber](http://mashable.com/2010/09/07/justin-bieber-twitter/),” *mashable.com*, September 7, 2010.
|
||||
|
||||
15. “[Riak 1.4.8 Docs](http://docs.basho.com/riak/1.4.8/),” Basho Technologies, Inc., 2014.
|
||||
|
||||
16. Richard Low: “[The Sweet Spot for Cassandra Secondary Indexing](http://www.wentnet.com/blog/?p=77),” *wentnet.com*, October 21, 2013.
|
||||
|
||||
17. Zachary Tong: “[Customizing Your Document Routing](http://www.elasticsearch.org/blog/customizing-your-document-routing/),” *elasticsearch.org*, June 3, 2013.
|
||||
|
||||
18. “[Apache Solr Reference Guide](https://cwiki.apache.org/confluence/display/solr/Apache+Solr+Reference+Guide),” Apache Software Foundation, 2014.
|
||||
|
||||
19. Andrew Pavlo: “[H-Store Frequently Asked Questions](http://hstore.cs.brown.edu/documentation/faq/),” *hstore.cs.brown.edu*, October 2013.
|
||||
|
||||
20. “[Amazon DynamoDB Developer Guide](http://docs.aws.amazon.com/amazondynamodb/latest/developerguide/),” Amazon Web Services, Inc., 2014.
|
||||
|
||||
21. Rusty Klophaus: “[Difference Between 2I and Search](http://lists.basho.com/pipermail/riak-users_lists.basho.com/2011-October/006220.html),” email to *riak-users* mailing list, *lists.basho.com*, October 25, 2011.
|
||||
|
||||
22. Donald K. Burleson: “[Object Partitioning in Oracle](http://www.dba-oracle.com/art_partit.htm),”*dba-oracle.com*, November 8, 2000.
|
||||
|
||||
23. Eric Evans: “[Rethinking Topology in Cassandra](http://www.slideshare.net/jericevans/virtual-nodes-rethinking-topology-in-cassandra),” at *ApacheCon Europe*, November 2012.
|
||||
|
||||
24. Rafał Kuć: “[Reroute API Explained](http://elasticsearchserverbook.com/reroute-api-explained/),” *elasticsearchserverbook.com*, September 30, 2013.
|
||||
|
||||
25. “[Project Voldemort Documentation](http://www.project-voldemort.com/voldemort/),” *project-voldemort.com*.
|
||||
|
||||
26. Enis Soztutar: “[Apache HBase Region Splitting and Merging](http://hortonworks.com/blog/apache-hbase-region-splitting-and-merging/),” *hortonworks.com*, February 1, 2013.
|
||||
|
||||
27. Brandon Williams: “[Virtual Nodes in Cassandra 1.2](http://www.datastax.com/dev/blog/virtual-nodes-in-cassandra-1-2),” *datastax.com*, December 4, 2012.
|
||||
|
||||
28. Richard Jones: “[libketama: Consistent Hashing Library for Memcached Clients](https://www.metabrew.com/article/libketama-consistent-hashing-algo-memcached-clients),” *metabrew.com*, April 10, 2007.
|
||||
|
||||
29. Branimir Lambov: “[New Token Allocation Algorithm in Cassandra 3.0](http://www.datastax.com/dev/blog/token-allocation-algorithm),” *datastax.com*, January 28, 2016.
|
||||
|
||||
30. Jason Wilder: “[Open-Source Service Discovery](http://jasonwilder.com/blog/2014/02/04/service-discovery-in-the-cloud/),” *jasonwilder.com*, February 2014.
|
||||
|
||||
31. Kishore Gopalakrishna, Shi Lu, Zhen Zhang, et al.: “[Untangling Cluster Management with Helix](http://www.socc2012.org/helix_onecol.pdf?attredirects=0),” at *ACM Symposium on Cloud Computing* (SoCC), October 2012.
|
||||
[doi:10.1145/2391229.2391248](http://dx.doi.org/10.1145/2391229.2391248)
|
||||
|
||||
32. “[Moxi 1.8 Manual](http://docs.couchbase.com/moxi-manual-1.8/),” Couchbase, Inc., 2014.
|
||||
|
||||
33. Shivnath Babu and Herodotos Herodotou: “[Massively Parallel Databases and MapReduce Systems](http://research.microsoft.com/pubs/206464/db-mr-survey-final.pdf),” *Foundations and Trends in Databases*, volume 5, number 1, pages 1–104, November 2013.[doi:10.1561/1900000036](http://dx.doi.org/10.1561/1900000036)
|
166
en-us/ch7.md
Normal file
166
en-us/ch7.md
Normal file
@ -0,0 +1,166 @@
|
||||
# 7. Transactions
|
||||
|
||||

|
||||
|
||||
> *Some authors have claimed that general two-phase commit is too expensive to support, because of the performance or availability problems that it brings. We believe it is better to have application programmers deal with performance problems due to overuse of transac‐ tions as bottlenecks arise, rather than always coding around the lack of transactions.*
|
||||
>
|
||||
> — James Corbett et al., *Spanner: Google’s Globally-Distributed Database* (2012)
|
||||
|
||||
------
|
||||
|
||||
In the harsh reality of data systems, many things can go wrong:
|
||||
|
||||
- The database software or hardware may fail at any time (including in the middle of a write operation).
|
||||
|
||||
- The application may crash at any time (including halfway through a series of operations).
|
||||
|
||||
- Interruptions in the network can unexpectedly cut off the application from the database, or one database node from another.
|
||||
|
||||
- Several clients may write to the database at the same time, overwriting each other’s changes.
|
||||
|
||||
- A client may read data that doesn’t make sense because it has only partially been updated.
|
||||
|
||||
- Race conditions between clients can cause surprising bugs.
|
||||
|
||||
In order to be reliable, a system has to deal with these faults and ensure that they don’t cause catastrophic failure of the entire system. However, implementing fault- tolerance mechanisms is a lot of work. It requires a lot of careful thinking about all the things that can go wrong, and a lot of testing to ensure that the solution actually works.
|
||||
|
||||
For decades, ***transactions*** have been the mechanism of choice for simplifying these issues. A transaction is a way for an application to group several reads and writes together into a logical unit. Conceptually, all the reads and writes in a transaction are executed as one operation: either the entire transaction succeeds (*commit*) or it fails (*abort*, *rollback*). If it fails, the application can safely retry. With transactions, error handling becomes much simpler for an application, because it doesn’t need to worry about partial failure—i.e., the case where some operations succeed and some fail (for whatever reason).
|
||||
|
||||
If you have spent years working with transactions, they may seem obvious, but we shouldn’t take them for granted. Transactions are not a law of nature; they were cre‐ ated with a purpose, namely to *simplify the programming model* for applications accessing a database. By using transactions, the application is free to ignore certain potential error scenarios and concurrency issues, because the database takes care of them instead (we call these *safety guarantees*).
|
||||
|
||||
Not every application needs transactions, and sometimes there are advantages to weakening transactional guarantees or abandoning them entirely (for example, to achieve higher performance or higher availability). Some safety properties can be achieved without transactions.
|
||||
|
||||
How do you figure out whether you need transactions? In order to answer that ques‐ tion, we first need to understand exactly what safety guarantees transactions can pro‐ vide, and what costs are associated with them. Although transactions seem straightforward at first glance, there are actually many subtle but important details that come into play.
|
||||
|
||||
In this chapter, we will examine many examples of things that can go wrong, and explore the algorithms that databases use to guard against those issues. We will go especially deep in the area of concurrency control, discussing various kinds of race conditions that can occur and how databases implement isolation levels such as *read committed*, *snapshot isolation*, and *serializability*.
|
||||
|
||||
This chapter applies to both single-node and distributed databases; in Chapter 8 we will focus the discussion on the particular challenges that arise only in distributed systems.
|
||||
|
||||
|
||||
## ……
|
||||
|
||||
|
||||
|
||||
## Summary
|
||||
|
||||
Transactions are an abstraction layer that allows an application to pretend that cer‐ tain concurrency problems and certain kinds of hardware and software faults don’t exist. A large class of errors is reduced down to a simple *transaction abort*, and the application just needs to try again.
|
||||
|
||||
In this chapter we saw many examples of problems that transactions help prevent. Not all applications are susceptible to all those problems: an application with very simple access patterns, such as reading and writing only a single record, can probably manage without transactions. However, for more complex access patterns, transac‐ tions can hugely reduce the number of potential error cases you need to think about.
|
||||
|
||||
Without transactions, various error scenarios (processes crashing, network interrup‐ tions, power outages, disk full, unexpected concurrency, etc.) mean that data can become inconsistent in various ways. For example, denormalized data can easily go out of sync with the source data. Without transactions, it becomes very difficult to reason about the effects that complex interacting accesses can have on the database.
|
||||
|
||||
In this chapter, we went particularly deep into the topic of concurrency control. We discussed several widely used isolation levels, in particular *read committed*, *snapshot isolation* (sometimes called *repeatable read*), and *serializable*. We characterized those isolation levels by discussing various examples of race conditions:
|
||||
|
||||
***Dirty reads***
|
||||
|
||||
One client reads another client’s writes before they have been committed. The read committed isolation level and stronger levels prevent dirty reads.
|
||||
|
||||
***Dirty writes***
|
||||
|
||||
One client overwrites data that another client has written, but not yet committed. Almost all transaction implementations prevent dirty writes.
|
||||
|
||||
***Read skew (nonrepeatable reads)***
|
||||
|
||||
A client sees different parts of the database at different points in time. This issue is most commonly prevented with snapshot isolation, which allows a transaction to read from a consistent snapshot at one point in time. It is usually implemented with *multi-version concurrency control* (MVCC).
|
||||
|
||||
***Lost updates***
|
||||
|
||||
Two clients concurrently perform a read-modify-write cycle. One overwrites the other’s write without incorporating its changes, so data is lost. Some implemen‐ tations of snapshot isolation prevent this anomaly automatically, while others require a manual lock (SELECT FOR UPDATE).
|
||||
|
||||
***Write skew***
|
||||
|
||||
A transaction reads something, makes a decision based on the value it saw, and writes the decision to the database. However, by the time the write is made, the premise of the decision is no longer true. Only serializable isolation prevents this anomaly.
|
||||
|
||||
***Phantom reads***
|
||||
|
||||
A transaction reads objects that match some search condition. Another client makes a write that affects the results of that search. Snapshot isolation prevents straightforward phantom reads, but phantoms in the context of write skew require special treatment, such as index-range locks.
|
||||
|
||||
|
||||
|
||||
Weak isolation levels protect against some of those anomalies but leave you, the application developer, to handle others manually (e.g., using explicit locking). Only serializable isolation protects against all of these issues. We discussed three different approaches to implementing serializable transactions:
|
||||
|
||||
***Literally executing transactions in a serial order***
|
||||
|
||||
If you can make each transaction very fast to execute, and the transaction throughput is low enough to process on a single CPU core, this is a simple and effective option.
|
||||
|
||||
***Two-phase locking***
|
||||
|
||||
For decades this has been the standard way of implementing serializability, but many applications avoid using it because of its performance characteristics.
|
||||
|
||||
***Serializable snapshot isolation (SSI)***
|
||||
|
||||
A fairly new algorithm that avoids most of the downsides of the previous approaches. It uses an optimistic approach, allowing transactions to proceed without blocking. When a transaction wants to commit, it is checked, and it is aborted if the execution was not serializable.
|
||||
|
||||
The examples in this chapter used a relational data model. However, as discussed in “[The need for multi-object transactions](ch7.md#the-need-for-multi-object-transactions)”, transactions are a valuable database feature, no matter which data model is used.
|
||||
|
||||
In this chapter, we explored ideas and algorithms mostly in the context of a database running on a single machine. Transactions in distributed databases open a new set of difficult challenges, which we’ll discuss in the next two chapters.
|
||||
|
||||
|
||||
|
||||
## References
|
||||
|
||||
--------------------
|
||||
|
||||
1. Donald D. Chamberlin, Morton M. Astrahan, Michael W. Blasgen, et al.: “[A History and Evaluation of System R](http://citeseerx.ist.psu.edu/viewdoc/download?doi=10.1.1.84.348&rep=rep1&type=pdf),” *Communications of the ACM*, volume 24, number 10, pages 632–646, October 1981.
|
||||
[doi:10.1145/358769.358784](http://dx.doi.org/10.1145/358769.358784)
|
||||
2. Jim N. Gray, Raymond A. Lorie, Gianfranco R. Putzolu, and Irving L. Traiger: “[Granularity of Locks and Degrees of Consistency in a Shared Data Base](http://citeseer.ist.psu.edu/viewdoc/download?doi=10.1.1.92.8248&rep=rep1&type=pdf),” in *Modelling in Data Base Management Systems: Proceedings of the IFIP Working Conference on Modelling in Data Base Management Systems*, edited by G. M. Nijssen, pages 364–394, Elsevier/North Holland Publishing, 1976. Also in *Readings in Database Systems*, 4th edition, edited by Joseph M. Hellerstein and Michael Stonebraker, MIT Press, 2005. ISBN: 978-0-262-69314-1
|
||||
3. Kapali P. Eswaran, Jim N. Gray, Raymond A. Lorie, and Irving L. Traiger: “[The Notions of Consistency and Predicate Locks in a Database System](http://research.microsoft.com/en-us/um/people/gray/papers/On%20the%20Notions%20of%20Consistency%20and%20Predicate%20Locks%20in%20a%20Database%20System%20CACM.pdf),” *Communications of the ACM*, volume 19, number 11, pages 624–633, November 1976.
|
||||
4. “[ACID Transactions Are Incredibly Helpful](http://web.archive.org/web/20150320053809/https://foundationdb.com/acid-claims),” FoundationDB, LLC, 2013.
|
||||
5. John D. Cook: “[ACID Versus BASE for Database Transactions](http://www.johndcook.com/blog/2009/07/06/brewer-cap-theorem-base/),” *johndcook.com*, July 6, 2009.
|
||||
6. Gavin Clarke: “[NoSQL's CAP Theorem Busters: We Don't Drop ACID](http://www.theregister.co.uk/2012/11/22/foundationdb_fear_of_cap_theorem/),” *theregister.co.uk*, November 22, 2012.
|
||||
7. Theo Härder and Andreas Reuter: “[Principles of Transaction-Oriented Database Recovery](http://citeseerx.ist.psu.edu/viewdoc/download?doi=10.1.1.87.2812&rep=rep1&type=pdf),” *ACM Computing Surveys*, volume 15, number 4, pages 287–317, December 1983. [doi:10.1145/289.291](http://dx.doi.org/10.1145/289.291)
|
||||
8. Peter Bailis, Alan Fekete, Ali Ghodsi, et al.: “[HAT, not CAP: Towards Highly Available Transactions](http://www.bailis.org/papers/hat-hotos2013.pdf),”
|
||||
at *14th USENIX Workshop on Hot Topics in Operating Systems* (HotOS), May 2013.
|
||||
9. Armando Fox, Steven D. Gribble, Yatin Chawathe, et al.: “[Cluster-Based Scalable Network Services](http://www.cs.berkeley.edu/~brewer/cs262b/TACC.pdf),” at
|
||||
*16th ACM Symposium on Operating Systems Principles* (SOSP), October 1997.
|
||||
10. Philip A. Bernstein, Vassos Hadzilacos, and Nathan Goodman: [*Concurrency Control and Recovery in Database Systems*](http://research.microsoft.com/en-us/people/philbe/ccontrol.aspx). Addison-Wesley, 1987. ISBN: 978-0-201-10715-9, available online at *research.microsoft.com*.
|
||||
11. Alan Fekete, Dimitrios Liarokapis, Elizabeth O'Neil, et al.: “[Making Snapshot Isolation Serializable](https://www.cse.iitb.ac.in/infolab/Data/Courses/CS632/2009/Papers/p492-fekete.pdf),” *ACM Transactions on Database Systems*, volume 30, number 2, pages 492–528, June 2005.
|
||||
[doi:10.1145/1071610.1071615](http://dx.doi.org/10.1145/1071610.1071615)
|
||||
12. Mai Zheng, Joseph Tucek, Feng Qin, and Mark Lillibridge: “[Understanding the Robustness of SSDs Under Power Fault](https://www.usenix.org/system/files/conference/fast13/fast13-final80.pdf),” at *11th USENIX Conference on File and Storage Technologies* (FAST), February 2013.
|
||||
13. Laurie Denness: “[SSDs: A Gift and a Curse](https://laur.ie/blog/2015/06/ssds-a-gift-and-a-curse/),” *laur.ie*, June 2, 2015.
|
||||
14. Adam Surak: “[When Solid State Drives Are Not That Solid](https://blog.algolia.com/when-solid-state-drives-are-not-that-solid/),” *blog.algolia.com*, June 15, 2015.
|
||||
15. Thanumalayan Sankaranarayana Pillai, Vijay Chidambaram, Ramnatthan Alagappan, et al.: “[All File Systems Are Not Created Equal: On the Complexity of Crafting Crash-Consistent Applications](http://research.cs.wisc.edu/wind/Publications/alice-osdi14.pdf),” at *11th USENIX Symposium on Operating Systems Design and Implementation* (OSDI),
|
||||
October 2014.
|
||||
16. Chris Siebenmann: “[Unix's File Durability Problem](https://utcc.utoronto.ca/~cks/space/blog/unix/FileSyncProblem),” *utcc.utoronto.ca*, April 14, 2016.
|
||||
17. Lakshmi N. Bairavasundaram, Garth R. Goodson, Bianca Schroeder, et al.: “[An Analysis of Data Corruption in the Storage Stack](http://research.cs.wisc.edu/adsl/Publications/corruption-fast08.pdf),” at *6th USENIX Conference on File and Storage Technologies* (FAST), February 2008.
|
||||
18. Bianca Schroeder, Raghav Lagisetty, and Arif Merchant: “[Flash Reliability in Production: The Expected and the Unexpected](https://www.usenix.org/conference/fast16/technical-sessions/presentation/schroeder),” at *14th USENIX Conference on File and Storage Technologies* (FAST), February 2016.
|
||||
19. Don Allison: “[SSD Storage – Ignorance of Technology Is No Excuse](https://blog.korelogic.com/blog/2015/03/24),” *blog.korelogic.com*, March 24, 2015.
|
||||
20. Dave Scherer: “[Those Are Not Transactions (Cassandra 2.0)](http://web.archive.org/web/20150526065247/http://blog.foundationdb.com/those-are-not-transactions-cassandra-2-0),” *blog.foundationdb.com*, September 6, 2013.
|
||||
21. Kyle Kingsbury: “[Call Me Maybe: Cassandra](http://aphyr.com/posts/294-call-me-maybe-cassandra/),” *aphyr.com*, September 24, 2013.
|
||||
22. “[ACID Support in Aerospike](http://www.aerospike.com/docs/architecture/assets/AerospikeACIDSupport.pdf),” Aerospike, Inc., June 2014.
|
||||
23. Martin Kleppmann: “[Hermitage: Testing the 'I' in ACID](http://martin.kleppmann.com/2014/11/25/hermitage-testing-the-i-in-acid.html),” *martin.kleppmann.com*, November 25, 2014.
|
||||
24. Tristan D'Agosta: “[BTC Stolen from Poloniex](https://bitcointalk.org/index.php?topic=499580),” *bitcointalk.org*, March 4, 2014.
|
||||
25. bitcointhief2: “[How I Stole Roughly 100 BTC from an Exchange and How I Could Have Stolen More!](http://www.reddit.com/r/Bitcoin/comments/1wtbiu/how_i_stole_roughly_100_btc_from_an_exchange_and/),” *reddit.com*, February 2, 2014.
|
||||
26. Sudhir Jorwekar, Alan Fekete, Krithi Ramamritham, and S. Sudarshan: “[Automating the Detection of Snapshot Isolation Anomalies](http://www.vldb.org/conf/2007/papers/industrial/p1263-jorwekar.pdf),” at *33rd International Conference on Very Large Data Bases* (VLDB), September 2007.
|
||||
27. Michael Melanson: “[Transactions: The Limits of Isolation](http://www.michaelmelanson.net/2014/03/20/transactions/),” *michaelmelanson.net*, March 20, 2014.
|
||||
28. Hal Berenson, Philip A. Bernstein, Jim N. Gray, et al.: “[A Critique of ANSI SQL Isolation Levels](http://research.microsoft.com/pubs/69541/tr-95-51.pdf),”
|
||||
at *ACM International Conference on Management of Data* (SIGMOD), May 1995.
|
||||
29. Atul Adya: “[Weak Consistency: A Generalized Theory and Optimistic Implementations for Distributed Transactions](http://pmg.csail.mit.edu/papers/adya-phd.pdf),” PhD Thesis, Massachusetts Institute of Technology, March 1999.
|
||||
30. Peter Bailis, Aaron Davidson, Alan Fekete, et al.: “[Highly Available Transactions: Virtues and Limitations (Extended Version)](http://arxiv.org/pdf/1302.0309.pdf),” at *40th International Conference on Very Large Data Bases* (VLDB), September 2014.
|
||||
31. Bruce Momjian: “[MVCC Unmasked](http://momjian.us/main/presentations/internals.html#mvcc),” *momjian.us*, July 2014.
|
||||
32. Annamalai Gurusami: “[Repeatable Read Isolation Level in InnoDB – How Consistent Read View Works](https://blogs.oracle.com/mysqlinnodb/entry/repeatable_read_isolation_level_in),” *blogs.oracle.com*, January 15, 2013.
|
||||
33. Nikita Prokopov: “[Unofficial Guide to Datomic Internals](http://tonsky.me/blog/unofficial-guide-to-datomic-internals/),” *tonsky.me*, May 6, 2014.
|
||||
34. Baron Schwartz: “[Immutability, MVCC, and Garbage Collection](http://www.xaprb.com/blog/2013/12/28/immutability-mvcc-and-garbage-collection/),” *xaprb.com*, December 28, 2013.
|
||||
35. J. Chris Anderson, Jan Lehnardt, and Noah Slater: *CouchDB: The Definitive Guide*. O'Reilly Media, 2010.
|
||||
ISBN: 978-0-596-15589-6
|
||||
36. Rikdeb Mukherjee: “[Isolation in DB2 (Repeatable Read, Read Stability, Cursor Stability, Uncommitted Read) with Examples](http://mframes.blogspot.co.uk/2013/07/isolation-in-cursor.html),” *mframes.blogspot.co.uk*, July 4, 2013.
|
||||
37. Steve Hilker: “[Cursor Stability (CS) – IBM DB2 Community](http://www.toadworld.com/platforms/ibmdb2/w/wiki/6661.cursor-stability-cs.aspx),” *toadworld.com*, March 14, 2013.
|
||||
38. Nate Wiger: “[An Atomic Rant](http://www.nateware.com/an-atomic-rant.html),” *nateware.com*, February 18, 2010.
|
||||
39. Joel Jacobson: “[Riak 2.0: Data Types](http://blog.joeljacobson.com/riak-2-0-data-types/),” *blog.joeljacobson.com*, March 23, 2014.
|
||||
40. Michael J. Cahill, Uwe Röhm, and Alan Fekete: “[Serializable Isolation for Snapshot Databases](http://www.cs.nyu.edu/courses/fall12/CSCI-GA.2434-001/p729-cahill.pdf),” at *ACM International Conference on Management of Data* (SIGMOD), June 2008. [doi:10.1145/1376616.1376690](http://dx.doi.org/10.1145/1376616.1376690)
|
||||
41. Dan R. K. Ports and Kevin Grittner: “[Serializable Snapshot Isolation in PostgreSQL](http://drkp.net/papers/ssi-vldb12.pdf),” at *38th International Conference on Very Large Databases* (VLDB), August 2012.
|
||||
42. Tony Andrews: “[Enforcing Complex Constraints in Oracle](http://tonyandrews.blogspot.co.uk/2004/10/enforcing-complex-constraints-in.html),” *tonyandrews.blogspot.co.uk*, October 15, 2004.
|
||||
43. Douglas B. Terry, Marvin M. Theimer, Karin Petersen, et al.: “[Managing Update Conflicts in Bayou, a Weakly Connected Replicated Storage System](http://citeseerx.ist.psu.edu/viewdoc/download?doi=10.1.1.141.7889&rep=rep1&type=pdf),” at *15th ACM Symposium on Operating Systems Principles* (SOSP), December 1995. [doi:10.1145/224056.224070](http://dx.doi.org/10.1145/224056.224070)
|
||||
44. Gary Fredericks: “[Postgres Serializability Bug](https://github.com/gfredericks/pg-serializability-bug),” *github.com*, September 2015.
|
||||
45. Michael Stonebraker, Samuel Madden, Daniel J. Abadi, et al.: “[The End of an Architectural Era (It’s Time for a Complete Rewrite)](http://citeseerx.ist.psu.edu/viewdoc/download?doi=10.1.1.137.3697&rep=rep1&type=pdf),” at *33rd International Conference on Very Large Data Bases* (VLDB), September 2007.
|
||||
46. John Hugg: “[H-Store/VoltDB Architecture vs. CEP Systems and Newer Streaming Architectures](https://www.youtube.com/watch?v=hD5M4a1UVz8),” at *Data @Scale Boston*, November 2014.
|
||||
47. Robert Kallman, Hideaki Kimura, Jonathan Natkins, et al.: “[H-Store: A High-Performance, Distributed Main Memory Transaction Processing System](http://www.vldb.org/pvldb/1/1454211.pdf),” *Proceedings of the VLDB Endowment*, volume 1, number 2, pages 1496–1499, August 2008.
|
||||
48. Rich Hickey: “[The Architecture of Datomic](http://www.infoq.com/articles/Architecture-Datomic),” *infoq.com*, November 2, 2012.
|
||||
49. John Hugg: “[Debunking Myths About the VoltDB In-Memory Database](http://voltdb.com/blog/debunking-myths-about-voltdb-memory-database),” *voltdb.com*, May 12, 2014.
|
||||
50. Joseph M. Hellerstein, Michael Stonebraker, and James Hamilton: “[Architecture of a Database System](http://db.cs.berkeley.edu/papers/fntdb07-architecture.pdf),”
|
||||
*Foundations and Trends in Databases*, volume 1, number 2, pages 141–259, November 2007.
|
||||
[doi:10.1561/1900000002](http://dx.doi.org/10.1561/1900000002)
|
||||
51. Michael J. Cahill: “[Serializable Isolation for Snapshot Databases](http://cahill.net.au/wp-content/uploads/2010/02/cahill-thesis.pdf),” PhD Thesis, University of Sydney, July 2009.
|
||||
52. D. Z. Badal: “[Correctness of Concurrency Control and Implications in Distributed Databases](http://ieeexplore.ieee.org/abstract/document/762563/),” at *3rd International IEEE Computer Software and Applications Conference* (COMPSAC), November 1979.
|
||||
53. Rakesh Agrawal, Michael J. Carey, and Miron Livny: “[Concurrency Control Performance Modeling: Alternatives and Implications](http://www.eecs.berkeley.edu/~brewer/cs262/ConcControl.pdf),” *ACM Transactions on Database Systems* (TODS), volume 12, number 4, pages 609–654, December 1987. [doi:10.1145/32204.32220](http://dx.doi.org/10.1145/32204.32220)
|
||||
54. Dave Rosenthal: “[Databases at 14.4MHz](http://web.archive.org/web/20150427041746/http://blog.foundationdb.com/databases-at-14.4mhz),” *blog.foundationdb.com*, December 10, 2014.
|
163
en-us/ch8.md
Normal file
163
en-us/ch8.md
Normal file
@ -0,0 +1,163 @@
|
||||
# 8. The Trouble with Distributed Systems
|
||||
|
||||

|
||||
|
||||
> *Hey I just met you*
|
||||
> *The network’s laggy*
|
||||
> *But here’s my data*
|
||||
> *So store it maybe*
|
||||
>
|
||||
> — Kyle Kingsbury, *Carly Rae Jepsen and the Perils of Network Partitions* (2013)
|
||||
|
||||
---------
|
||||
|
||||
A recurring theme in the last few chapters has been how systems handle things going wrong. For example, we discussed replica failover (“[Handling Node Outages](ch5.md#handing-node-outages)”), replication lag (“[Problems with Replication Lag](ch5.md#problems-with-replication-lag)”), and con‐ currency control for transactions (“[Weak Isolation Levels](ch7.md#weak-isolation-levels)”). As we come to understand various edge cases that can occur in real systems, we get better at handling them.
|
||||
|
||||
However, even though we have talked a lot about faults, the last few chapters have still been too optimistic. The reality is even darker. We will now turn our pessimism to the maximum and assume that anything that *can* go wrong *will* go wrong.[^i] (Experienced systems operators will tell you that is a reasonable assumption. If you ask nicely, they might tell you some frightening stories while nursing their scars of past battles.)
|
||||
|
||||
[^i]: With one exception: we will assume that faults are *non-Byzantine* (see “[Byzantine Faults](ch8.md#byzantine-faults)”).
|
||||
|
||||
Working with distributed systems is fundamentally different from writing software on a single computer—and the main difference is that there are lots of new and excit‐ ing ways for things to go wrong [1, 2]. In this chapter, we will get a taste of the prob‐ lems that arise in practice, and an understanding of the things we can and cannot rely on.
|
||||
|
||||
In the end, our task as engineers is to build systems that do their job (i.e., meet the guarantees that users are expecting), in spite of everything going wrong. In [Chapter 9](ch9.md), we will look at some examples of algorithms that can provide such guarantees in a distributed system. But first, in this chapter, we must understand what challenges we are up against.
|
||||
|
||||
This chapter is a thoroughly pessimistic and depressing overview of things that may go wrong in a distributed system. We will look into problems with networks (“[Unreliable Networks](#unreliable-networks)”); clocks and timing issues (“[Unreliable Clocks](#unreliable-clocks)”); and we’ll discuss to what degree they are avoidable. The consequences of all these issues are disorienting, so we’ll explore how to think about the state of a dis‐ tributed system and how to reason about things that have happened (“[Knowledge, Truth, and Lies](#knowledge-truth-and-lies)”).
|
||||
|
||||
|
||||
|
||||
## ……
|
||||
|
||||
|
||||
|
||||
## Summary
|
||||
|
||||
In this chapter we have discussed a wide range of problems that can occur in dis‐ tributed systems, including:
|
||||
|
||||
- Whenever you try to send a packet over the network, it may be lost or arbitrarily delayed. Likewise, the reply may be lost or delayed, so if you don’t get a reply, you have no idea whether the message got through.
|
||||
- A node’s clock may be significantly out of sync with other nodes (despite your best efforts to set up NTP), it may suddenly jump forward or back in time, and relying on it is dangerous because you most likely don’t have a good measure of your clock’s error interval.
|
||||
- A process may pause for a substantial amount of time at any point in its execu‐ tion (perhaps due to a stop-the-world garbage collector), be declared dead by other nodes, and then come back to life again without realizing that it was paused.
|
||||
|
||||
The fact that such *partial failures* can occur is the defining characteristic of dis‐ tributed systems. Whenever software tries to do anything involving other nodes, there is the possibility that it may occasionally fail, or randomly go slow, or not respond at all (and eventually time out). In distributed systems, we try to build tolerance of partial failures into software, so that the system as a whole may continue functioning even when some of its constituent parts are broken.
|
||||
|
||||
To tolerate faults, the first step is to *detect* them, but even that is hard. Most systems don’t have an accurate mechanism of detecting whether a node has failed, so most distributed algorithms rely on timeouts to determine whether a remote node is still available. However, timeouts can’t distinguish between network and node failures, and variable network delay sometimes causes a node to be falsely suspected of crash‐ ing. Moreover, sometimes a node can be in a degraded state: for example, a Gigabit network interface could suddenly drop to 1 Kb/s throughput due to a driver bug [94]. Such a node that is “limping” but not dead can be even more difficult to deal with than a cleanly failed node.
|
||||
|
||||
Once a fault is detected, making a system tolerate it is not easy either: there is no global variable, no shared memory, no common knowledge or any other kind of shared state between the machines. Nodes can’t even agree on what time it is, let alone on anything more profound. The only way information can flow from one node to another is by sending it over the unreliable network. Major decisions cannot be safely made by a single node, so we require protocols that enlist help from other nodes and try to get a quorum to agree.
|
||||
|
||||
If you’re used to writing software in the idealized mathematical perfection of a single computer, where the same operation always deterministically returns the same result, then moving to the messy physical reality of distributed systems can be a bit of a shock. Conversely, distributed systems engineers will often regard a problem as triv‐ ial if it can be solved on a single computer [5], and indeed a single computer can do a lot nowadays [95]. If you can avoid opening Pandora’s box and simply keep things on a single machine, it is generally worth doing so.
|
||||
|
||||
However, as discussed in the introduction to [Part II](part-ii.md), scalability is not the only reason for wanting to use a distributed system. Fault tolerance and low latency (by placing data geographically close to users) are equally important goals, and those things can‐ not be achieved with a single node.
|
||||
|
||||
In this chapter we also went on some tangents to explore whether the unreliability of networks, clocks, and processes is an inevitable law of nature. We saw that it isn’t: it is possible to give hard real-time response guarantees and bounded delays in net‐ works, but doing so is very expensive and results in lower utilization of hardware resources. Most non-safety-critical systems choose cheap and unreliable over expen‐ sive and reliable.
|
||||
|
||||
We also touched on supercomputers, which assume reliable components and thus have to be stopped and restarted entirely when a component does fail. By contrast, distributed systems can run forever without being interrupted at the service level, because all faults and maintenance can be handled at the node level—at least in theory. (In practice, if a bad configuration change is rolled out to all nodes, that will still bring a distributed system to its knees.)
|
||||
|
||||
This chapter has been all about problems, and has given us a bleak outlook. In the next chapter we will move on to solutions, and discuss some algorithms that have been designed to cope with all the problems in distributed systems.
|
||||
|
||||
## References
|
||||
--------------------
|
||||
|
||||
1. Mark Cavage: Just No Getting Around It: You’re Building a Distributed System](http://queue.acm.org/detail.cfm?id=2482856),” *ACM Queue*, volume 11, number 4, pages 80-89, April 2013.
|
||||
[doi:10.1145/2466486.2482856](http://dx.doi.org/10.1145/2466486.2482856)
|
||||
1. Jay Kreps: “[Getting Real About Distributed System Reliability](http://blog.empathybox.com/post/19574936361/getting-real-about-distributed-system-reliability),” *blog.empathybox.com*, March 19, 2012.
|
||||
1. Sydney Padua: *The Thrilling Adventures of Lovelace and Babbage: The (Mostly) True Story of the First Computer*. Particular Books, April ISBN: 978-0-141-98151-2
|
||||
1. Coda Hale: “[You Can’t Sacrifice Partition Tolerance](http://codahale.com/you-cant-sacrifice-partition-tolerance/),” *codahale.com*, October 7, 2010.
|
||||
1. Jeff Hodges: “[Notes on Distributed Systems for Young Bloods](http://www.somethingsimilar.com/2013/01/14/notes-on-distributed-systems-for-young-bloods/),” *somethingsimilar.com*, January 14, 2013.
|
||||
1. Antonio Regalado: “[Who Coined 'Cloud Computing’?](http://www.technologyreview.com/news/425970/who-coined-cloud-computing/),” *technologyreview.com*, October 31, 2011.
|
||||
1. Luiz André Barroso, Jimmy Clidaras, and Urs Hölzle: “[The Datacenter as a Computer: An Introduction to the Design of Warehouse-Scale Machines, Second Edition](http://www.morganclaypool.com/doi/abs/10.2200/S00516ED2V01Y201306CAC024),” *Synthesis Lectures on Computer Architecture*, volume 8, number 3, Morgan & Claypool Publishers, July 2013.[doi:10.2200/S00516ED2V01Y201306CAC024](http://dx.doi.org/10.2200/S00516ED2V01Y201306CAC024), ISBN: 978-1-627-05010-4
|
||||
1. David Fiala, Frank Mueller, Christian Engelmann, et al.: “[Detection and Correction of Silent Data Corruption for Large-Scale High-Performance Computing](http://moss.csc.ncsu.edu/~mueller/ftp/pub/mueller/papers/sc12.pdf),” at *International Conference for High Performance Computing, Networking, Storage and Analysis* (SC12), November 2012.
|
||||
1. Arjun Singh, Joon Ong, Amit Agarwal, et al.: “[Jupiter Rising: A Decade of Clos Topologies and Centralized Control in Google’s Datacenter Network](http://conferences.sigcomm.org/sigcomm/2015/pdf/papers/p183.pdf),” at *Annual Conference of the ACM Special Interest Group on Data Communication* (SIGCOMM), August 2015. [doi:10.1145/2785956.2787508](http://dx.doi.org/10.1145/2785956.2787508)
|
||||
1. Glenn K. Lockwood: “[Hadoop's Uncomfortable Fit in HPC](http://glennklockwood.blogspot.co.uk/2014/05/hadoops-uncomfortable-fit-in-hpc.html),” *glennklockwood.blogspot.co.uk*, May 16, 2014.
|
||||
1. John von Neumann: “[Probabilistic Logics and the Synthesis of Reliable Organisms from Unreliable Components](https://ece.uwaterloo.ca/~ssundara/courses/prob_logics.pdf),” in *Automata Studies (AM-34)*, edited by Claude E. Shannon and John McCarthy, Princeton University Press, 1956. ISBN: 978-0-691-07916-5
|
||||
1. Richard W. Hamming: *The Art of Doing Science and Engineering*. Taylor & Francis, 1997. ISBN: 978-9-056-99500-3
|
||||
1. Claude E. Shannon: “[A Mathematical Theory of Communication](http://cs.brynmawr.edu/Courses/cs380/fall2012/shannon1948.pdf),” *The Bell System Technical Journal*, volume 27, number 3, pages 379–423 and 623–656, July 1948.
|
||||
1. Peter Bailis and Kyle Kingsbury: “[The Network Is Reliable](https://queue.acm.org/detail.cfm?id=2655736),” *ACM Queue*, volume 12, number 7, pages 48-55, July 2014. [doi:10.1145/2639988.2639988](http://dx.doi.org/10.1145/2639988.2639988)
|
||||
1. Joshua B. Leners, Trinabh Gupta, Marcos K. Aguilera, and Michael Walfish: “[Taming Uncertainty in Distributed Systems with Help from the Network](http://www.cs.nyu.edu/~mwalfish/papers/albatross-eurosys15.pdf),” at *10th European Conference on Computer Systems* (EuroSys), April 2015. [doi:10.1145/2741948.2741976](http://dx.doi.org/10.1145/2741948.2741976)
|
||||
1. Phillipa Gill, Navendu Jain, and Nachiappan Nagappan: “[Understanding Network Failures in Data Centers: Measurement, Analysis, and Implications](http://conferences.sigcomm.org/sigcomm/2011/papers/sigcomm/p350.pdf),” at *ACM SIGCOMM Conference*, August 2011.
|
||||
[doi:10.1145/2018436.2018477](http://dx.doi.org/10.1145/2018436.2018477)
|
||||
1. Mark Imbriaco: “[Downtime Last Saturday](https://github.com/blog/1364-downtime-last-saturday),” *github.com*, December 26, 2012.
|
||||
1. Will Oremus: “[The Global Internet Is Being Attacked by Sharks, Google Confirms](http://www.slate.com/blogs/future_tense/2014/08/15/shark_attacks_threaten_google_s_undersea_internet_cables_video.html),” *slate.com*, August 15,
|
||||
2014.
|
||||
1. Marc A. Donges: “[Re: bnx2 cards Intermittantly Going Offline](http://www.spinics.net/lists/netdev/msg210485.html),” Message to Linux *netdev* mailing list, *spinics.net*, September 13, 2012.
|
||||
1. Kyle Kingsbury: “[Call Me Maybe: Elasticsearch](https://aphyr.com/posts/317-call-me-maybe-elasticsearch),” *aphyr.com*, June 15, 2014.
|
||||
1. Salvatore Sanfilippo: “[A Few Arguments About Redis Sentinel Properties and Fail Scenarios](http://antirez.com/news/80),” *antirez.com*, October 21, 2014.
|
||||
1. Bert Hubert: “[The Ultimate SO_LINGER Page, or: Why Is My TCP Not Reliable](http://blog.netherlabs.nl/articles/2009/01/18/the-ultimate-so_linger-page-or-why-is-my-tcp-not-reliable),” *blog.netherlabs.nl*, January 18, 2009.
|
||||
1. Nicolas Liochon: “[CAP: If All You Have Is a Timeout, Everything Looks Like a Partition](http://blog.thislongrun.com/2015/05/CAP-theorem-partition-timeout-zookeeper.html),” *blog.thislongrun.com*, May 25, 2015.
|
||||
1. Jerome H. Saltzer, David P. Reed, and David D. Clark: “[End-To-End Arguments in System Design](http://www.ece.drexel.edu/courses/ECE-C631-501/SalRee1984.pdf),” *ACM Transactions on Computer Systems*, volume 2, number 4, pages 277–288, November 1984. [doi:10.1145/357401.357402](http://dx.doi.org/10.1145/357401.357402)
|
||||
1. Matthew P. Grosvenor, Malte Schwarzkopf, Ionel Gog, et al.: “[Queues Don’t Matter When You Can JUMP Them!](https://www.usenix.org/system/files/conference/nsdi15/nsdi15-paper-grosvenor_update.pdf),” at *12th USENIX Symposium on Networked Systems Design and Implementation* (NSDI), May 2015.
|
||||
1. Guohui Wang and T. S. Eugene Ng: “[The Impact of Virtualization on Network Performance of Amazon EC2 Data Center](http://www.cs.rice.edu/~eugeneng/papers/INFOCOM10-ec2.pdf),” at *29th IEEE International Conference on Computer Communications* (INFOCOM), March 2010. [doi:10.1109/INFCOM.2010.5461931](http://dx.doi.org/10.1109/INFCOM.2010.5461931)
|
||||
1. Van Jacobson: “[Congestion Avoidance and Control](http://www.cs.usask.ca/ftp/pub/discus/seminars2002-2003/p314-jacobson.pdf),” at *ACM Symposium on Communications Architectures and Protocols* (SIGCOMM), August 1988. [doi:10.1145/52324.52356](http://dx.doi.org/10.1145/52324.52356)
|
||||
1. Brandon Philips: “[etcd: Distributed Locking and Service Discovery](https://www.youtube.com/watch?v=HJIjTTHWYnE),” at *Strange Loop*, September 2014.
|
||||
1. Steve Newman: “[A Systematic Look at EC2 I/O](http://blog.scalyr.com/2012/10/a-systematic-look-at-ec2-io/),” *blog.scalyr.com*, October 16, 2012.
|
||||
1. Naohiro Hayashibara, Xavier Défago, Rami Yared, and Takuya Katayama: “[The ϕ Accrual Failure Detector](http://hdl.handle.net/10119/4784),” Japan Advanced Institute of Science and Technology, School of Information Science, Technical Report IS-RR-2004-010, May 2004.
|
||||
1. Jeffrey Wang: “[Phi Accrual Failure Detector](http://ternarysearch.blogspot.co.uk/2013/08/phi-accrual-failure-detector.html),” *ternarysearch.blogspot.co.uk*, August 11, 2013.
|
||||
1. Srinivasan Keshav: *An Engineering Approach to Computer Networking: ATM Networks, the Internet, and the Telephone Network*. Addison-Wesley Professional, May 1997. ISBN: 978-0-201-63442-6
|
||||
1. Cisco, “[Integrated Services Digital Network](http://docwiki.cisco.com/wiki/Integrated_Services_Digital_Network),” *docwiki.cisco.com*.
|
||||
1. Othmar Kyas: *ATM Networks*. International Thomson Publishing, 1995. ISBN: 978-1-850-32128-6
|
||||
1. “[InfiniBand FAQ](http://www.mellanox.com/related-docs/whitepapers/InfiniBandFAQ_FQ_100.pdf),” Mellanox Technologies, December 22, 2014.
|
||||
1. Jose Renato Santos, Yoshio Turner, and G. (John) Janakiraman: “[End-to-End Congestion Control for InfiniBand](http://www.hpl.hp.com/techreports/2002/HPL-2002-359.pdf),” at *22nd Annual Joint Conference of the IEEE Computer and Communications Societies* (INFOCOM), April 2003. Also published by HP Laboratories Palo
|
||||
Alto, Tech Report HPL-2002-359. [doi:10.1109/INFCOM.2003.1208949](http://dx.doi.org/10.1109/INFCOM.2003.1208949)
|
||||
1. Ulrich Windl, David Dalton, Marc Martinec, and Dale R. Worley: “[The NTP FAQ and HOWTO](http://www.ntp.org/ntpfaq/NTP-a-faq.htm),” *ntp.org*, November 2006.
|
||||
1. John Graham-Cumming: “[How and why the leap second affected Cloudflare DNS](https://blog.cloudflare.com/how-and-why-the-leap-second-affected-cloudflare-dns/),” *blog.cloudflare.com*, January 1, 2017.
|
||||
1. David Holmes: “[Inside the Hotspot VM: Clocks, Timers and Scheduling Events – Part I – Windows](https://blogs.oracle.com/dholmes/entry/inside_the_hotspot_vm_clocks),” *blogs.oracle.com*, October 2, 2006.
|
||||
1. Steve Loughran: “[Time on Multi-Core, Multi-Socket Servers](http://steveloughran.blogspot.co.uk/2015/09/time-on-multi-core-multi-socket-servers.html),” *steveloughran.blogspot.co.uk*, September 17, 2015.
|
||||
1. James C. Corbett, Jeffrey Dean, Michael Epstein, et al.: “[Spanner: Google’s Globally-Distributed Database](http://research.google.com/archive/spanner.html),” at *10th USENIX Symposium on Operating System Design and Implementation* (OSDI), October 2012.
|
||||
1. M. Caporaloni and R. Ambrosini: “[How Closely Can a Personal Computer Clock Track the UTC Timescale Via the Internet?](https://iopscience.iop.org/0143-0807/23/4/103/),” *European Journal of Physics*, volume 23, number 4, pages L17–L21, June 2012. [doi:10.1088/0143-0807/23/4/103](http://dx.doi.org/10.1088/0143-0807/23/4/103)
|
||||
1. Nelson Minar: “[A Survey of the NTP Network](http://alumni.media.mit.edu/~nelson/research/ntp-survey99/),” *alumni.media.mit.edu*, December 1999.
|
||||
1. Viliam Holub: “[Synchronizing Clocks in a Cassandra Cluster Pt. 1 – The Problem](https://blog.logentries.com/2014/03/synchronizing-clocks-in-a-cassandra-cluster-pt-1-the-problem/),” *blog.logentries.com*, March 14, 2014.
|
||||
1. Poul-Henning Kamp: “[The One-Second War (What Time Will You Die?)](http://queue.acm.org/detail.cfm?id=1967009),” *ACM Queue*, volume 9, number 4, pages 44–48, April 2011. [doi:10.1145/1966989.1967009](http://dx.doi.org/10.1145/1966989.1967009)
|
||||
1. Nelson Minar: “[Leap Second Crashes Half the Internet](http://www.somebits.com/weblog/tech/bad/leap-second-2012.html),” *somebits.com*, July 3, 2012.
|
||||
1. Christopher Pascoe: “[Time, Technology and Leaping Seconds](http://googleblog.blogspot.co.uk/2011/09/time-technology-and-leaping-seconds.html),” *googleblog.blogspot.co.uk*, September 15, 2011.
|
||||
1. Mingxue Zhao and Jeff Barr: “[Look Before You Leap – The Coming Leap Second and AWS](https://aws.amazon.com/blogs/aws/look-before-you-leap-the-coming-leap-second-and-aws/),” *aws.amazon.com*, May 18, 2015.
|
||||
1. Darryl Veitch and Kanthaiah Vijayalayan: “[Network Timing and the 2015 Leap Second](http://crin.eng.uts.edu.au/~darryl/Publications/LeapSecond_camera.pdf),” at *17th International Conference on Passive and Active Measurement* (PAM), April 2016. [doi:10.1007/978-3-319-30505-9_29](http://dx.doi.org/10.1007/978-3-319-30505-9_29)
|
||||
1. “[Timekeeping in VMware Virtual Machines](http://www.vmware.com/resources/techresources/238),” Information Guide, VMware, Inc., December 2011.
|
||||
1. “[MiFID II / MiFIR: Regulatory Technical and Implementing Standards – Annex I (Draft)](https://www.esma.europa.eu/sites/default/files/library/2015/11/2015-esma-1464_annex_i_-_draft_rts_and_its_on_mifid_ii_and_mifir.pdf),”
|
||||
European Securities and Markets Authority, Report ESMA/2015/1464, September 2015.
|
||||
1. Luke Bigum: “[Solving MiFID II Clock Synchronisation With Minimum Spend (Part 1)](https://www.lmax.com/blog/staff-blogs/2015/11/27/solving-mifid-ii-clock-synchronisation-minimum-spend-part-1/),” *lmax.com*, November 27, 2015.
|
||||
1. Kyle Kingsbury: “[Call Me Maybe: Cassandra](https://aphyr.com/posts/294-call-me-maybe-cassandra/),” *aphyr.com*, September 24, 2013.
|
||||
1. John Daily: “[Clocks Are Bad, or, Welcome to the Wonderful World of Distributed Systems](http://basho.com/clocks-are-bad-or-welcome-to-distributed-systems/),” *basho.com*, November 12, 2013.
|
||||
1. Kyle Kingsbury: “[The Trouble with Timestamps](https://aphyr.com/posts/299-the-trouble-with-timestamps),” *aphyr.com*, October 12, 2013.
|
||||
1. Leslie Lamport: “[Time, Clocks, and the Ordering of Events in a Distributed System](http://research.microsoft.com/en-US/um/people/Lamport/pubs/time-clocks.pdf),” *Communications of the ACM*, volume 21, number 7, pages 558–565, July 1978. [doi:10.1145/359545.359563](http://dx.doi.org/10.1145/359545.359563)
|
||||
1. Sandeep Kulkarni, Murat Demirbas, Deepak Madeppa, et al.: “[Logical Physical Clocks and Consistent Snapshots in Globally Distributed Databases](http://www.cse.buffalo.edu/tech-reports/2014-04.pdf),” State University of New York at Buffalo, Computer Science and Engineering Technical Report 2014-04, May 2014.
|
||||
1. Justin Sheehy: “[There Is No Now: Problems With Simultaneity in Distributed Systems](https://queue.acm.org/detail.cfm?id=2745385),” *ACM Queue*, volume 13, number 3, pages 36–41, March 2015. [doi:10.1145/2733108](http://dx.doi.org/10.1145/2733108)
|
||||
1. Murat Demirbas: “[Spanner: Google's Globally-Distributed Database](http://muratbuffalo.blogspot.co.uk/2013/07/spanner-googles-globally-distributed_4.html),” *muratbuffalo.blogspot.co.uk*, July 4, 2013.
|
||||
1. Dahlia Malkhi and Jean-Philippe Martin: “[Spanner's Concurrency Control](http://www.cs.cornell.edu/~ie53/publications/DC-col51-Sep13.pdf),” *ACM SIGACT News*, volume 44, number 3, pages 73–77, September 2013. [doi:10.1145/2527748.2527767](http://dx.doi.org/10.1145/2527748.2527767)
|
||||
1. Manuel Bravo, Nuno Diegues, Jingna Zeng, et al.: “[On the Use of Clocks to Enforce Consistency in the Cloud](http://sites.computer.org/debull/A15mar/p18.pdf),” *IEEE Data Engineering Bulletin*,
|
||||
volume 38, number 1, pages 18–31, March 2015.
|
||||
1. Spencer Kimball: “[Living Without Atomic Clocks](http://www.cockroachlabs.com/blog/living-without-atomic-clocks/),” *cockroachlabs.com*, February 17, 2016.
|
||||
1. Cary G. Gray and David R. Cheriton:“[Leases: An Efficient Fault-Tolerant Mechanism for Distributed File Cache Consistency](http://web.stanford.edu/class/cs240/readings/89-leases.pdf),” at *12th ACM Symposium on Operating Systems Principles* (SOSP), December 1989.
|
||||
[doi:10.1145/74850.74870](http://dx.doi.org/10.1145/74850.74870)
|
||||
1. Todd Lipcon: “[Avoiding Full GCs in Apache HBase with MemStore-Local Allocation Buffers: Part 1](http://blog.cloudera.com/blog/2011/02/avoiding-full-gcs-in-hbase-with-memstore-local-allocation-buffers-part-1/),”
|
||||
*blog.cloudera.com*, February 24, 2011.
|
||||
1. Martin Thompson: “[Java Garbage Collection Distilled](http://mechanical-sympathy.blogspot.co.uk/2013/07/java-garbage-collection-distilled.html),” *mechanical-sympathy.blogspot.co.uk*, July 16, 2013.
|
||||
1. Alexey Ragozin: “[How to Tame Java GC Pauses? Surviving 16GiB Heap and Greater](http://java.dzone.com/articles/how-tame-java-gc-pauses),” *java.dzone.com*, June 28, 2011.
|
||||
1. Christopher Clark, Keir Fraser, Steven Hand, et al.: “[Live Migration of Virtual Machines](http://www.cl.cam.ac.uk/research/srg/netos/papers/2005-nsdi-migration.pdf),” at *2nd USENIX Symposium on Symposium on Networked Systems Design & Implementation* (NSDI), May 2005.
|
||||
1. Mike Shaver: “[fsyncers and Curveballs](http://shaver.off.net/diary/2008/05/25/fsyncers-and-curveballs/),” *shaver.off.net*, May 25, 2008.
|
||||
1. Zhenyun Zhuang and Cuong Tran: “[Eliminating Large JVM GC Pauses Caused by Background IO Traffic](https://engineering.linkedin.com/blog/2016/02/eliminating-large-jvm-gc-pauses-caused-by-background-io-traffic),” *engineering.linkedin.com*, February 10, 2016.
|
||||
1. David Terei and Amit Levy: “[Blade: A Data Center Garbage Collector](http://arxiv.org/pdf/1504.02578.pdf),” arXiv:1504.02578, April 13, 2015.
|
||||
1. Martin Maas, Tim Harris, Krste Asanović, and John Kubiatowicz: “[Trash Day: Coordinating Garbage Collection in Distributed Systems](https://timharris.uk/papers/2015-hotos.pdf),” at *15th USENIX Workshop on Hot Topics in Operating Systems* (HotOS), May 2015.
|
||||
1. “[Predictable Low Latency](http://cdn2.hubspot.net/hubfs/1624455/Website_2016/content/White%20papers/Cinnober%20on%20GC%20pause%20free%20Java%20applications.pdf),” Cinnober Financial Technology AB, *cinnober.com*, November 24, 2013.
|
||||
1. Martin Fowler: “[The LMAX Architecture](http://martinfowler.com/articles/lmax.html),” *martinfowler.com*, July 12, 2011.
|
||||
1. Flavio P. Junqueira and Benjamin Reed: *ZooKeeper: Distributed Process Coordination*. O'Reilly Media, 2013. ISBN: 978-1-449-36130-3
|
||||
1. Enis Söztutar: “[HBase and HDFS: Understanding Filesystem Usage in HBase](http://www.slideshare.net/enissoz/hbase-and-hdfs-understanding-filesystem-usage),” at *HBaseCon*, June 2013.
|
||||
1. Caitie McCaffrey: “[Clients Are Jerks: AKA How Halo 4 DoSed the Services at Launch & How We Survived](http://caitiem.com/2015/06/23/clients-are-jerks-aka-how-halo-4-dosed-the-services-at-launch-how-we-survived/),” *caitiem.com*, June 23, 2015.
|
||||
1. Leslie Lamport, Robert Shostak, and Marshall Pease: “[The Byzantine Generals Problem](http://research.microsoft.com/en-us/um/people/lamport/pubs/byz.pdf),” *ACM Transactions on Programming Languages and Systems* (TOPLAS), volume 4, number 3, pages 382–401, July 1982. [doi:10.1145/357172.357176](http://dx.doi.org/10.1145/357172.357176)
|
||||
1. Jim N. Gray: “[Notes on Data Base Operating Systems](http://research.microsoft.com/en-us/um/people/gray/papers/DBOS.pdf),” in *Operating Systems: An Advanced Course*, Lecture
|
||||
Notes in Computer Science, volume 60, edited by R. Bayer, R. M. Graham, and G. Seegmüller,
|
||||
pages 393–481, Springer-Verlag, 1978. ISBN: 978-3-540-08755-7
|
||||
1. Brian Palmer: “[How Complicated Was the Byzantine Empire?](http://www.slate.com/articles/news_and_politics/explainer/2011/10/the_byzantine_tax_code_how_complicated_was_byzantium_anyway_.html),” *slate.com*, October 20, 2011.
|
||||
1. Leslie Lamport: “[My Writings](http://research.microsoft.com/en-us/um/people/lamport/pubs/pubs.html),” *research.microsoft.com*, December 16, 2014. This page can be found by searching the web for the 23-character string obtained by removing the hyphens from the string `allla-mport-spubso-ntheweb`.
|
||||
1. John Rushby: “[Bus Architectures for Safety-Critical Embedded Systems](http://www.csl.sri.com/papers/emsoft01/emsoft01.pdf),” at *1st International Workshop on Embedded Software* (EMSOFT), October 2001.
|
||||
1. Jake Edge: “[ELC: SpaceX Lessons Learned](http://lwn.net/Articles/540368/),” *lwn.net*, March 6, 2013.
|
||||
1. Andrew Miller and Joseph J. LaViola, Jr.: “[Anonymous Byzantine Consensus from Moderately-Hard Puzzles: A Model for Bitcoin](http://nakamotoinstitute.org/static/docs/anonymous-byzantine-consensus.pdf),” University of Central Florida, Technical Report CS-TR-14-01, April 2014.
|
||||
1. James Mickens: “[The Saddest Moment](https://www.usenix.org/system/files/login-logout_1305_mickens.pdf),” *USENIX ;login: logout*, May 2013.
|
||||
1. Evan Gilman: “[The Discovery of Apache ZooKeeper’s Poison Packet](http://www.pagerduty.com/blog/the-discovery-of-apache-zookeepers-poison-packet/),” *pagerduty.com*, May 7, 2015.
|
||||
1. Jonathan Stone and Craig Partridge: “[When the CRC and TCP Checksum Disagree](http://citeseerx.ist.psu.edu/viewdoc/download?doi=10.1.1.27.7611&rep=rep1&type=pdf),” at *ACM Conference on Applications, Technologies, Architectures, and Protocols for Computer Communication* (SIGCOMM), August 2000. [doi:10.1145/347059.347561](http://dx.doi.org/10.1145/347059.347561)
|
||||
1. Evan Jones: “[How Both TCP and Ethernet Checksums Fail](http://www.evanjones.ca/tcp-and-ethernet-checksums-fail.html),” *evanjones.ca*, October 5, 2015.
|
||||
1. Cynthia Dwork, Nancy Lynch, and Larry Stockmeyer: “[Consensus in the Presence of Partial Synchrony](http://www.net.t-labs.tu-berlin.de/~petr/ADC-07/papers/DLS88.pdf),” *Journal of the ACM*, volume 35, number 2, pages 288–323, April 1988. [doi:10.1145/42282.42283](http://dx.doi.org/10.1145/42282.42283)
|
||||
1. Peter Bailis and Ali Ghodsi: “[Eventual Consistency Today: Limitations, Extensions, and Beyond](http://queue.acm.org/detail.cfm?id=2462076),” *ACM Queue*, volume 11, number 3, pages 55-63, March 2013. [doi:10.1145/2460276.2462076](http://dx.doi.org/10.1145/2460276.2462076)
|
||||
1. Bowen Alpern and Fred B. Schneider: “[Defining Liveness](https://www.cs.cornell.edu/fbs/publications/DefLiveness.pdf),” *Information Processing Letters*, volume 21, number 4, pages 181–185, October 1985. [doi:10.1016/0020-0190(85)90056-0](http://dx.doi.org/10.1016/0020-0190(85)90056-0)
|
||||
1. Flavio P. Junqueira: “[Dude, Where’s My Metadata?](http://fpj.me/2015/05/28/dude-wheres-my-metadata/),” *fpj.me*, May 28, 2015.
|
||||
1. Scott Sanders: “[January 28th Incident Report](https://github.com/blog/2106-january-28th-incident-report),” *github.com*, February 3, 2016.
|
||||
1. Jay Kreps: “[A Few Notes on Kafka and Jepsen](http://blog.empathybox.com/post/62279088548/a-few-notes-on-kafka-and-jepsen),” *blog.empathybox.com*, September 25, 2013.
|
||||
1. Thanh Do, Mingzhe Hao, Tanakorn Leesatapornwongsa, et al.: “[Limplock: Understanding the Impact of Limpware on Scale-out Cloud Systems](http://ucare.cs.uchicago.edu/pdf/socc13-limplock.pdf),” at *4th ACM Symposium on Cloud Computing* (SoCC), October 2013. [doi:10.1145/2523616.2523627](http://dx.doi.org/10.1145/2523616.2523627)
|
||||
1. Frank McSherry, Michael Isard, and Derek G. Murray: “[Scalability! But at What COST?](http://www.frankmcsherry.org/assets/COST.pdf),” at *15th USENIX Workshop on Hot Topics in Operating Systems* (HotOS), May 2015.
|
324
en-us/ch9.md
Normal file
324
en-us/ch9.md
Normal file
@ -0,0 +1,324 @@
|
||||
# 9. Consistency and Consensus
|
||||
|
||||

|
||||
|
||||
> *Is it better to be alive and wrong or right and dead?*
|
||||
>
|
||||
> — Jay Kreps, *A Few Notes on Kafka and Jepsen* (2013)
|
||||
|
||||
---------------
|
||||
|
||||
Lots of things can go wrong in distributed systems, as discussed in [Chapter 8](ch8.md). The simplest way of handling such faults is to simply let the entire service fail, and show the user an error message. If that solution is unacceptable, we need to find ways of *tolerating* faults—that is, of keeping the service functioning correctly, even if some internal component is faulty.
|
||||
|
||||
In this chapter, we will talk about some examples of algorithms and protocols for building fault-tolerant distributed systems. We will assume that all the problems from [Chapter 8](ch8.md) can occur: packets can be lost, reordered, duplicated, or arbitrarily delayed in the network; clocks are approximate at best; and nodes can pause (e.g., due to garbage collection) or crash at any time.
|
||||
|
||||
The best way of building fault-tolerant systems is to find some general-purpose abstractions with useful guarantees, implement them once, and then let applications rely on those guarantees. This is the same approach as we used with transactions in [Chapter 7](ch7.md): by using a transaction, the application can pretend that there are no crashes (atomicity), that nobody else is concurrently accessing the database (isola‐ tion), and that storage devices are perfectly reliable (durability). Even though crashes, race conditions, and disk failures do occur, the transaction abstraction hides those problems so that the application doesn’t need to worry about them.
|
||||
|
||||
We will now continue along the same lines, and seek abstractions that can allow an application to ignore some of the problems with distributed systems. For example, one of the most important abstractions for distributed systems is *consensus*: that is, getting all of the nodes to agree on something. As we shall see in this chapter, reliably reaching consensus in spite of network faults and process failures is a surprisingly tricky problem.
|
||||
|
||||
Once you have an implementation of consensus, applications can use it for various purposes. For example, say you have a database with single-leader replication. If the leader dies and you need to fail over to another node, the remaining database nodes can use consensus to elect a new leader. As discussed in “[Handling Node Outages](ch5.md#handling-onde-outages)” on page 156, it’s important that there is only one leader, and that all nodes agree who the leader is. If two nodes both believe that they are the leader, that situation is called *split brain*, and it often leads to data loss. Correct implementations of consensus help avoid such problems.
|
||||
|
||||
Later in this chapter, in “[Distributed Transactions and Consensus](#distributed-transactions-and-consensus)”, we will look into algorithms to solve consensus and related problems. But first we first need to explore the range of guarantees and abstractions that can be provided in a distributed system.
|
||||
|
||||
We need to understand the scope of what can and cannot be done: in some situa‐ tions, it’s possible for the system to tolerate faults and continue working; in other sit‐ uations, that is not possible. The limits of what is and isn’t possible have been explored in depth, both in theoretical proofs and in practical implementations. We will get an overview of those fundamental limits in this chapter.
|
||||
|
||||
Researchers in the field of distributed systems have been studying these topics for decades, so there is a lot of material—we’ll only be able to scratch the surface. In this book we don’t have space to go into details of the formal models and proofs, so we will stick with informal intuitions. The literature references offer plenty of additional depth if you’re interested.
|
||||
|
||||
|
||||
## ……
|
||||
|
||||
|
||||
|
||||
## Summary
|
||||
|
||||
In this chapter we examined the topics of consistency and consensus from several different angles. We looked in depth at linearizability, a popular consistency model: its goal is to make replicated data appear as though there were only a single copy, and to make all operations act on it atomically. Although linearizability is appealing because it is easy to understand—it makes a database behave like a variable in a single-threaded program — it has the downside of being slow, especially in environments with large network delays.
|
||||
|
||||
We also explored causality, which imposes an ordering on events in a system (what happened before what, based on cause and effect). Unlike linearizability, which puts all operations in a single, totally ordered timeline, causality provides us with a weaker consistency model: some things can be concurrent, so the version history is like a timeline with branching and merging. Causal consistency does not have the coordi‐ nation overhead of linearizability and is much less sensitive to network problems.
|
||||
|
||||
However, even if we capture the causal ordering (for example using Lamport timestamps), we saw that some things cannot be implemented this way: in “Timestamp ordering is not sufficient” on page 347 we considered the example of ensuring that a username is unique and rejecting concurrent registrations for the same username. If one node is going to accept a registration, it needs to somehow know that another node isn’t concurrently in the process of registering the same name. This problem led us toward *consensus*.
|
||||
|
||||
We saw that achieving consensus means deciding something in such a way that all nodes agree on what was decided, and such that the decision is irrevocable. With some digging, it turns out that a wide range of problems are actually reducible to consensus and are equivalent to each other (in the sense that if you have a solution for one of them, you can easily transform it into a solution for one of the others). Such equivalent problems include:
|
||||
|
||||
***Linearizable compare-and-set registers***
|
||||
|
||||
The register needs to atomically *decide* whether to set its value, based on whether its current value equals the parameter given in the operation.
|
||||
|
||||
***Atomic transaction commit***
|
||||
|
||||
A database must *decide* whether to commit or abort a distributed transaction.
|
||||
|
||||
***Total order broadcast***
|
||||
|
||||
The messaging system must *decide* on the order in which to deliver messages.
|
||||
|
||||
***Locks and leases***
|
||||
|
||||
When several clients are racing to grab a lock or lease, the lock *decides* which one successfully acquired it.
|
||||
|
||||
***Membership/coordination service***
|
||||
|
||||
Given a failure detector (e.g., timeouts), the system must *decide* which nodes are alive, and which should be considered dead because their sessions timed out.
|
||||
|
||||
***Uniqueness constraint***
|
||||
|
||||
When several transactions concurrently try to create conflicting records with the same key, the constraint must *decide* which one to allow and which should fail with a constraint violation.
|
||||
|
||||
|
||||
|
||||
All of these are straightforward if you only have a single node, or if you are willing to assign the decision-making capability to a single node. This is what happens in a single-leader database: all the power to make decisions is vested in the leader, which is why such databases are able to provide linearizable operations, uniqueness con‐ straints, a totally ordered replication log, and more.
|
||||
|
||||
However, if that single leader fails, or if a network interruption makes the leader unreachable, such a system becomes unable to make any progress. There are three ways of handling that situation:
|
||||
|
||||
1. Wait for the leader to recover, and accept that the system will be blocked in the meantime. Many XA/JTA transaction coordinators choose this option. This approach does not fully solve consensus because it does not satisfy the termina‐ tion property: if the leader does not recover, the system can be blocked forever.
|
||||
|
||||
2. Manually fail over by getting humans to choose a new leader node and reconfig‐ ure the system to use it. Many relational databases take this approach. It is a kind of consensus by “act of God”—the human operator, outside of the computer sys‐ tem, makes the decision. The speed of failover is limited by the speed at which humans can act, which is generally slower than computers.
|
||||
|
||||
3. Use an algorithm to automatically choose a new leader. This approach requires a consensus algorithm, and it is advisable to use a proven algorithm that correctly handles adverse network conditions [107].
|
||||
|
||||
Although a single-leader database can provide linearizability without executing a consensus algorithm on every write, it still requires consensus to maintain its leader‐ ship and for leadership changes. Thus, in some sense, having a leader only “kicks the can down the road”: consensus is still required, only in a different place, and less fre‐ quently. The good news is that fault-tolerant algorithms and systems for consensus exist, and we briefly discussed them in this chapter.
|
||||
|
||||
Tools like ZooKeeper play an important role in providing an “outsourced” consen‐ sus, failure detection, and membership service that applications can use. It’s not easy to use, but it is much better than trying to develop your own algorithms that can withstand all the problems discussed in [Chapter 8](ch8.md). If you find yourself wanting to do one of those things that is reducible to consensus, and you want it to be fault-tolerant, then it is advisable to use something like ZooKeeper.
|
||||
|
||||
Nevertheless, not every system necessarily requires consensus: for example, leaderless and multi-leader replication systems typically do not use global consensus. The con‐ flicts that occur in these systems (see “[Handling Write Conflicts](ch5.md#handling-write-conflicts)”) are a consequence of not having consensus across different leaders, but maybe that’s okay: maybe we simply need to cope without linearizability and learn to work better with data that has branching and merging version histories.
|
||||
|
||||
This chapter referenced a large body of research on the theory of distributed systems. Although the theoretical papers and proofs are not always easy to understand, and sometimes make unrealistic assumptions, they are incredibly valuable for informing practical work in this field: they help us reason about what can and cannot be done, and help us find the counterintuitive ways in which distributed systems are often flawed. If you have the time, the references are well worth exploring.
|
||||
|
||||
This brings us to the end of [Part II](part-ii.md) of this book, in which we covered replication ([Chapter 5](ch5.md)), partitioning ([Chapter 6](ch6.md)), transactions ([Chapter 7](ch7.md)), distributed system failure models ([Chapter 8](ch8.md)), and finally consistency and consensus ([Chapter 9](ch9.md)). Now that we have laid a firm foundation of theory, in [Part III](part-iii.md) we will turn once again to more practical systems, and discuss how to build powerful applications from heterogeneous building blocks.
|
||||
|
||||
## References
|
||||
--------------------
|
||||
|
||||
|
||||
1. Peter Bailis and Ali Ghodsi: “[Eventual Consistency Today: Limitations, Extensions, and Beyond](http://queue.acm.org/detail.cfm?id=2462076),” *ACM Queue*, volume 11, number 3, pages 55-63, March 2013. [doi:10.1145/2460276.2462076](http://dx.doi.org/10.1145/2460276.2462076)
|
||||
|
||||
1. Prince Mahajan, Lorenzo Alvisi, and Mike Dahlin: “[Consistency, Availability, and Convergence](http://apps.cs.utexas.edu/tech_reports/reports/tr/TR-2036.pdf),” University of Texas at Austin, Department of Computer Science, Tech Report UTCS TR-11-22, May 2011.
|
||||
|
||||
1. Alex Scotti: “[Adventures in Building Your Own Database](http://www.slideshare.net/AlexScotti1/allyourbase-55212398),” at *All Your Base*, November 2015.
|
||||
|
||||
1. Peter Bailis, Aaron Davidson, Alan Fekete, et al.: “[Highly Available Transactions: Virtues and Limitations](http://arxiv.org/pdf/1302.0309.pdf),” at *40th International Conference on Very Large Data Bases* (VLDB), September 2014. Extended version published as pre-print arXiv:1302.0309 [cs.DB].
|
||||
|
||||
1. Paolo Viotti and Marko Vukolić: “[Consistency in Non-Transactional Distributed Storage Systems](http://arxiv.org/abs/1512.00168),” arXiv:1512.00168, 12 April 2016.
|
||||
|
||||
1. Maurice P. Herlihy and Jeannette M. Wing: “[Linearizability: A Correctness Condition for Concurrent Objects](http://cs.brown.edu/~mph/HerlihyW90/p463-herlihy.pdf),” *ACM Transactions on Programming Languages and Systems* (TOPLAS), volume 12, number 3, pages 463–492, July 1990. [doi:10.1145/78969.78972](http://dx.doi.org/10.1145/78969.78972)
|
||||
|
||||
1. Leslie Lamport: “[On interprocess communication](http://research.microsoft.com/en-us/um/people/lamport/pubs/interprocess.pdf),” *Distributed Computing*, volume 1, number 2, pages 77–101, June 1986. [doi:10.1007/BF01786228](http://dx.doi.org/10.1007/BF01786228)
|
||||
|
||||
1. David K. Gifford: “[Information Storage in a Decentralized Computer System](http://www.mirrorservice.org/sites/www.bitsavers.org/pdf/xerox/parc/techReports/CSL-81-8_Information_Storage_in_a_Decentralized_Computer_System.pdf),” Xerox Palo Alto Research Centers, CSL-81-8, June 1981.
|
||||
|
||||
1. Martin Kleppmann: “[Please Stop Calling Databases CP or AP](http://martin.kleppmann.com/2015/05/11/please-stop-calling-databases-cp-or-ap.html),” *martin.kleppmann.com*, May 11, 2015.
|
||||
|
||||
1. Kyle Kingsbury: “[Call Me Maybe: MongoDB Stale Reads](https://aphyr.com/posts/322-call-me-maybe-mongodb-stale-reads),” *aphyr.com*, April 20, 2015.
|
||||
|
||||
1. Kyle Kingsbury: “[Computational Techniques in Knossos](https://aphyr.com/posts/314-computational-techniques-in-knossos),” *aphyr.com*, May 17, 2014.
|
||||
|
||||
1. Peter Bailis: “[Linearizability Versus Serializability](http://www.bailis.org/blog/linearizability-versus-serializability/),” *bailis.org*, September 24, 2014.
|
||||
|
||||
1. Philip A. Bernstein, Vassos Hadzilacos, and Nathan Goodman: [*Concurrency Control and Recovery in Database Systems*](http://research.microsoft.com/en-us/people/philbe/ccontrol.aspx). Addison-Wesley, 1987. ISBN: 978-0-201-10715-9, available online at *research.microsoft.com*.
|
||||
|
||||
1. Mike Burrows: “[The Chubby Lock Service for Loosely-Coupled Distributed Systems](http://research.google.com/archive/chubby.html),” at *7th USENIX Symposium on Operating System Design and Implementation* (OSDI), November 2006.
|
||||
|
||||
1. Flavio P. Junqueira and Benjamin Reed: *ZooKeeper: Distributed Process Coordination*. O'Reilly Media, 2013. ISBN: 978-1-449-36130-3
|
||||
|
||||
1. “[etcd 2.0.12 Documentation](https://coreos.com/etcd/docs/2.0.12/),” CoreOS, Inc., 2015.
|
||||
|
||||
1. “[Apache Curator](http://curator.apache.org/),” Apache Software Foundation, *curator.apache.org*, 2015.
|
||||
|
||||
1. Morali Vallath: *Oracle 10g RAC Grid, Services & Clustering*. Elsevier Digital Press, 2006. ISBN: 978-1-555-58321-7
|
||||
|
||||
1. Peter Bailis, Alan Fekete, Michael J Franklin, et al.: “[Coordination-Avoiding Database Systems](http://arxiv.org/pdf/1402.2237.pdf),” *Proceedings of the VLDB Endowment*, volume 8, number 3, pages 185–196, November 2014.
|
||||
|
||||
1. Kyle Kingsbury: “[Call Me Maybe: etcd and Consul](https://aphyr.com/posts/316-call-me-maybe-etcd-and-consul),” *aphyr.com*, June 9, 2014.
|
||||
|
||||
1. Flavio P. Junqueira, Benjamin C. Reed, and Marco Serafini: “[Zab: High-Performance Broadcast for Primary-Backup Systems](https://pdfs.semanticscholar.org/b02c/6b00bd5dbdbd951fddb00b906c82fa80f0b3.pdf),” at *41st IEEE International Conference on Dependable Systems and Networks* (DSN), June 2011. [doi:10.1109/DSN.2011.5958223](http://dx.doi.org/10.1109/DSN.2011.5958223)
|
||||
|
||||
1. Diego Ongaro and John K. Ousterhout: “[In Search of an Understandable Consensus Algorithm (Extended Version)](http://ramcloud.stanford.edu/raft.pdf),” at *USENIX Annual Technical Conference* (ATC), June 2014.
|
||||
|
||||
1. Hagit Attiya, Amotz Bar-Noy, and Danny Dolev: “[Sharing Memory Robustly in Message-Passing Systems](http://www.cse.huji.ac.il/course/2004/dist/p124-attiya.pdf),” *Journal of the ACM*, volume 42, number 1, pages 124–142, January 1995. [doi:10.1145/200836.200869](http://dx.doi.org/10.1145/200836.200869)
|
||||
|
||||
1. Nancy Lynch and Alex Shvartsman: “[Robust Emulation of Shared Memory Using Dynamic Quorum-Acknowledged Broadcasts](http://groups.csail.mit.edu/tds/papers/Lynch/FTCS97.pdf),” at *27th Annual International Symposium on Fault-Tolerant Computing* (FTCS), June 1997. [doi:10.1109/FTCS.1997.614100](http://dx.doi.org/10.1109/FTCS.1997.614100)
|
||||
|
||||
1. Christian Cachin, Rachid Guerraoui, and Luís Rodrigues: [*Introduction to Reliable and Secure Distributed Programming*](http://www.distributedprogramming.net/), 2nd edition. Springer, 2011. ISBN: 978-3-642-15259-7, [doi:10.1007/978-3-642-15260-3](http://dx.doi.org/10.1007/978-3-642-15260-3)
|
||||
|
||||
1. Sam Elliott, Mark Allen, and Martin Kleppmann: [personal communication](https://twitter.com/lenary/status/654761711933648896), thread on *twitter.com*, October 15, 2015.
|
||||
|
||||
1. Niklas Ekström, Mikhail Panchenko, and Jonathan Ellis: “[Possible Issue with Read Repair?](http://mail-archives.apache.org/mod_mbox/cassandra-dev/201210.mbox/%3CFA480D1DC3964E2C8B0A14E0880094C9%40Robotech%3E),” email thread on *cassandra-dev* mailing list, October 2012.
|
||||
|
||||
1. Maurice P. Herlihy: “[Wait-Free Synchronization](https://cs.brown.edu/~mph/Herlihy91/p124-herlihy.pdf),” *ACM Transactions on Programming Languages and Systems* (TOPLAS), volume 13, number 1, pages 124–149, January 1991. [doi:10.1145/114005.102808](http://dx.doi.org/10.1145/114005.102808)
|
||||
|
||||
1. Armando Fox and Eric A. Brewer: “[Harvest, Yield, and Scalable Tolerant Systems](http://radlab.cs.berkeley.edu/people/fox/static/pubs/pdf/c18.pdf),” at *7th Workshop on Hot Topics in Operating Systems* (HotOS), March 1999. [doi:10.1109/HOTOS.1999.798396](http://dx.doi.org/10.1109/HOTOS.1999.798396)
|
||||
|
||||
1. Seth Gilbert and Nancy Lynch: “[Brewer’s Conjecture and the Feasibility of Consistent, Available, Partition-Tolerant Web Services](http://www.comp.nus.edu.sg/~gilbert/pubs/BrewersConjecture-SigAct.pdf),” *ACM SIGACT News*, volume 33, number 2, pages 51–59, June 2002. [doi:10.1145/564585.564601](http://dx.doi.org/10.1145/564585.564601)
|
||||
|
||||
1. Seth Gilbert and Nancy Lynch: “[Perspectives on the CAP Theorem](http://groups.csail.mit.edu/tds/papers/Gilbert/Brewer2.pdf),” *IEEE Computer Magazine*, volume 45, number 2, pages 30–36, February 2012. [doi:10.1109/MC.2011.389](http://dx.doi.org/10.1109/MC.2011.389)
|
||||
|
||||
1. Eric A. Brewer: “[CAP Twelve Years Later: How the 'Rules' Have Changed](http://cs609.cs.ua.edu/CAP12.pdf),” *IEEE Computer Magazine*, volume 45, number 2, pages 23–29, February 2012. [doi:10.1109/MC.2012.37](http://dx.doi.org/10.1109/MC.2012.37)
|
||||
|
||||
1. Susan B. Davidson, Hector Garcia-Molina, and Dale Skeen: “[Consistency in Partitioned Networks](http://delab.csd.auth.gr/~dimitris/courses/mpc_fall05/papers/invalidation/acm_csur85_partitioned_network_consistency.pdf),” *ACM Computing Surveys*, volume 17, number 3, pages 341–370, September 1985. [doi:10.1145/5505.5508](http://dx.doi.org/10.1145/5505.5508)
|
||||
|
||||
1. Paul R. Johnson and Robert H. Thomas: “[RFC 677: The Maintenance of Duplicate Databases](https://tools.ietf.org/html/rfc677),” Network Working Group, January 27, 1975.
|
||||
|
||||
1. Bruce G. Lindsay, Patricia Griffiths Selinger, C. Galtieri, et al.: “[Notes on Distributed Databases](http://domino.research.ibm.com/library/cyberdig.nsf/papers/A776EC17FC2FCE73852579F100578964/$File/RJ2571.pdf),” IBM Research, Research Report RJ2571(33471), July 1979.
|
||||
|
||||
1. Michael J. Fischer and Alan Michael: “[Sacrificing Serializability to Attain High Availability of Data in an Unreliable Network](http://www.cs.ucsb.edu/~agrawal/spring2011/ugrad/p70-fischer.pdf),” at *1st ACM Symposium on Principles of Database Systems* (PODS), March 1982.
|
||||
[doi:10.1145/588111.588124](http://dx.doi.org/10.1145/588111.588124)
|
||||
|
||||
1. Eric A. Brewer: “[NoSQL: Past, Present, Future](http://www.infoq.com/presentations/NoSQL-History),” at *QCon San Francisco*, November 2012.
|
||||
|
||||
1. Henry Robinson: “[CAP Confusion: Problems with 'Partition Tolerance,'](http://blog.cloudera.com/blog/2010/04/cap-confusion-problems-with-partition-tolerance/)” *blog.cloudera.com*, April 26, 2010.
|
||||
|
||||
1. Adrian Cockcroft: “[Migrating to Microservices](http://www.infoq.com/presentations/migration-cloud-native),” at *QCon London*, March 2014.
|
||||
|
||||
1. Martin Kleppmann: “[A Critique of the CAP Theorem](http://arxiv.org/abs/1509.05393),” arXiv:1509.05393, September 17, 2015.
|
||||
|
||||
1. Nancy A. Lynch: “[A Hundred Impossibility Proofs for Distributed Computing](http://groups.csail.mit.edu/tds/papers/Lynch/podc89.pdf),” at *8th ACM Symposium on Principles of Distributed Computing* (PODC), August 1989. [doi:10.1145/72981.72982](http://dx.doi.org/10.1145/72981.72982)
|
||||
|
||||
1. Hagit Attiya, Faith Ellen, and Adam Morrison: “[Limitations of Highly-Available Eventually-Consistent Data Stores](http://www.cs.technion.ac.il/people/mad/online-publications/podc2015-replds.pdf),” at *ACM Symposium on Principles of Distributed Computing* (PODC), July 2015. doi:10.1145/2767386.2767419](http://dx.doi.org/10.1145/2767386.2767419)
|
||||
|
||||
1. Peter Sewell, Susmit Sarkar, Scott Owens, et al.: “[x86-TSO: A Rigorous and Usable Programmer's Model for x86 Multiprocessors](http://www.cl.cam.ac.uk/~pes20/weakmemory/cacm.pdf),” *Communications of the ACM*, volume 53, number 7, pages 89–97, July 2010.
|
||||
[doi:10.1145/1785414.1785443](http://dx.doi.org/10.1145/1785414.1785443)
|
||||
|
||||
1. Martin Thompson: “[Memory Barriers/Fences](http://mechanical-sympathy.blogspot.co.uk/2011/07/memory-barriersfences.html),” *mechanical-sympathy.blogspot.co.uk*, July 24, 2011.
|
||||
|
||||
1. Ulrich Drepper: “[What Every Programmer Should Know About Memory](http://www.akkadia.org/drepper/cpumemory.pdf),” *akkadia.org*, November 21, 2007.
|
||||
|
||||
1. Daniel J. Abadi: “[Consistency Tradeoffs in Modern Distributed Database System Design](http://cs-www.cs.yale.edu/homes/dna/papers/abadi-pacelc.pdf),” *IEEE Computer Magazine*, volume 45, number 2, pages 37–42, February 2012. [doi:10.1109/MC.2012.33](http://dx.doi.org/10.1109/MC.2012.33)
|
||||
|
||||
1. Hagit Attiya and Jennifer L. Welch: “[Sequential Consistency Versus Linearizability](http://courses.csail.mit.edu/6.852/01/papers/p91-attiya.pdf),” *ACM Transactions on Computer Systems* (TOCS), volume 12, number 2, pages 91–122, May 1994. [doi:10.1145/176575.176576](http://dx.doi.org/10.1145/176575.176576)
|
||||
|
||||
1. Mustaque Ahamad, Gil Neiger, James E. Burns, et al.: “[Causal Memory: Definitions, Implementation, and Programming](http://www-i2.informatik.rwth-aachen.de/i2/fileadmin/user_upload/documents/Seminar_MCMM11/Causal_memory_1996.pdf),” *Distributed Computing*, volume 9, number 1, pages 37–49, March 1995. [doi:10.1007/BF01784241](http://dx.doi.org/10.1007/BF01784241)
|
||||
|
||||
1. Wyatt Lloyd, Michael J. Freedman, Michael Kaminsky, and David G. Andersen: “[Stronger Semantics for Low-Latency Geo-Replicated Storage](https://www.usenix.org/system/files/conference/nsdi13/nsdi13-final149.pdf),” at *10th USENIX Symposium on Networked Systems Design and Implementation* (NSDI), April 2013.
|
||||
|
||||
1. Marek Zawirski, Annette Bieniusa, Valter Balegas, et al.: “[SwiftCloud: Fault-Tolerant Geo-Replication Integrated All the Way to the Client Machine](http://arxiv.org/abs/1310.3107),” INRIA Research Report 8347, August 2013.
|
||||
|
||||
1. Peter Bailis, Ali Ghodsi, Joseph M Hellerstein, and Ion Stoica: “[Bolt-on Causal Consistency](http://db.cs.berkeley.edu/papers/sigmod13-bolton.pdf),” at
|
||||
*ACM International Conference on Management of Data* (SIGMOD), June 2013.
|
||||
|
||||
1. Philippe Ajoux, Nathan Bronson, Sanjeev Kumar, et al.: “[Challenges to Adopting Stronger Consistency at Scale](https://www.usenix.org/system/files/conference/hotos15/hotos15-paper-ajoux.pdf),” at *15th USENIX Workshop on Hot Topics in Operating Systems* (HotOS), May 2015.
|
||||
|
||||
1. Peter Bailis: “[Causality Is Expensive (and What to Do About It)](http://www.bailis.org/blog/causality-is-expensive-and-what-to-do-about-it/),” *bailis.org*, February 5, 2014.
|
||||
|
||||
1. Ricardo Gonçalves, Paulo Sérgio Almeida, Carlos Baquero, and Victor Fonte: “[Concise Server-Wide Causality Management for Eventually Consistent Data Stores](http://haslab.uminho.pt/tome/files/global_logical_clocks.pdf),” at *15th IFIP International Conference on Distributed Applications and Interoperable Systems* (DAIS), June 2015. [doi:10.1007/978-3-319-19129-4_6](http://dx.doi.org/10.1007/978-3-319-19129-4_6)
|
||||
|
||||
1. Rob Conery: “[A Better ID Generator for PostgreSQL](http://rob.conery.io/2014/05/29/a-better-id-generator-for-postgresql/),” *rob.conery.io*, May 29, 2014.
|
||||
|
||||
1. Leslie Lamport: “[Time, Clocks, and the Ordering of Events in a Distributed System](http://research.microsoft.com/en-US/um/people/Lamport/pubs/time-clocks.pdf),” *Communications of the ACM*, volume 21, number 7, pages 558–565, July 1978. [doi:10.1145/359545.359563](http://dx.doi.org/10.1145/359545.359563)
|
||||
|
||||
1. Xavier Défago, André Schiper, and Péter Urbán: “[Total Order Broadcast and Multicast Algorithms: Taxonomy and Survey](https://dspace.jaist.ac.jp/dspace/bitstream/10119/4883/1/defago_et_al.pdf),” *ACM Computing Surveys*, volume 36, number 4, pages 372–421, December 2004.
|
||||
[doi:10.1145/1041680.1041682](http://dx.doi.org/10.1145/1041680.1041682)
|
||||
|
||||
1. Hagit Attiya and Jennifer Welch: *Distributed Computing: Fundamentals, Simulations and Advanced Topics*, 2nd edition. John Wiley & Sons, 2004. ISBN: 978-0-471-45324-6, [doi:10.1002/0471478210](http://dx.doi.org/10.1002/0471478210)
|
||||
|
||||
1. Mahesh Balakrishnan, Dahlia Malkhi, Vijayan Prabhakaran, et al.: “[CORFU: A Shared Log Design for Flash Clusters](https://www.usenix.org/system/files/conference/nsdi12/nsdi12-final30.pdf),” at *9th USENIX Symposium on Networked Systems Design and Implementation* (NSDI), April 2012.
|
||||
|
||||
1. Fred B. Schneider: “[Implementing Fault-Tolerant Services Using the State Machine Approach: A Tutorial](http://www.cs.cornell.edu/fbs/publications/smsurvey.pdf),” *ACM Computing Surveys*, volume 22, number 4, pages 299–319, December 1990.
|
||||
|
||||
1. Alexander Thomson, Thaddeus Diamond, Shu-Chun Weng, et al.: “[Calvin: Fast Distributed Transactions for Partitioned Database Systems](http://cs.yale.edu/homes/thomson/publications/calvin-sigmod12.pdf),” at *ACM International Conference on Management of Data* (SIGMOD), May 2012.
|
||||
|
||||
1. Mahesh Balakrishnan, Dahlia Malkhi, Ted Wobber, et al.: “[Tango: Distributed Data Structures over a Shared Log](http://research.microsoft.com/pubs/199947/Tango.pdf),” at *24th ACM Symposium on Operating Systems Principles* (SOSP), November 2013.
|
||||
[doi:10.1145/2517349.2522732](http://dx.doi.org/10.1145/2517349.2522732)
|
||||
|
||||
1. Robbert van Renesse and Fred B. Schneider: “[Chain Replication for Supporting High Throughput and Availability](http://static.usenix.org/legacy/events/osdi04/tech/full_papers/renesse/renesse.pdf),” at *6th USENIX Symposium on Operating System Design and Implementation* (OSDI), December 2004.
|
||||
|
||||
1. Leslie Lamport: “[How to Make a Multiprocessor Computer That Correctly Executes Multiprocess Programs](http://research-srv.microsoft.com/en-us/um/people/lamport/pubs/multi.pdf),” *IEEE Transactions on Computers*, volume 28, number 9, pages 690–691, September 1979.
|
||||
[doi:10.1109/TC.1979.1675439](http://dx.doi.org/10.1109/TC.1979.1675439)
|
||||
|
||||
1. Enis Söztutar, Devaraj Das, and Carter Shanklin: “[Apache HBase High Availability at the Next Level](http://hortonworks.com/blog/apache-hbase-high-availability-next-level/),” *hortonworks.com*, January 22, 2015.
|
||||
|
||||
1. Brian F Cooper, Raghu Ramakrishnan, Utkarsh Srivastava, et al.: “[PNUTS: Yahoo!’s Hosted Data Serving Platform](http://www.mpi-sws.org/~druschel/courses/ds/papers/cooper-pnuts.pdf),” at *34th International Conference on Very Large Data Bases* (VLDB), August 2008.
|
||||
[doi:10.14778/1454159.1454167](http://dx.doi.org/10.14778/1454159.1454167)
|
||||
|
||||
1. Tushar Deepak Chandra and Sam Toueg: “[Unreliable Failure Detectors for Reliable Distributed Systems](http://courses.csail.mit.edu/6.852/08/papers/CT96-JACM.pdf),” *Journal of the ACM*, volume 43, number 2, pages 225–267, March 1996. [doi:10.1145/226643.226647](http://dx.doi.org/10.1145/226643.226647)
|
||||
|
||||
1. Michael J. Fischer, Nancy Lynch, and Michael S. Paterson: “[Impossibility of Distributed Consensus with One Faulty Process](https://groups.csail.mit.edu/tds/papers/Lynch/jacm85.pdf),” *Journal of the ACM*, volume 32, number 2, pages 374–382, April 1985. [doi:10.1145/3149.214121](http://dx.doi.org/10.1145/3149.214121)
|
||||
|
||||
1. Michael Ben-Or: “Another Advantage of Free Choice: Completely Asynchronous Agreement Protocols,” at *2nd ACM Symposium on Principles of Distributed Computing* (PODC), August 1983. [doi:10.1145/800221.806707](http://dl.acm.org/citation.cfm?id=806707)
|
||||
|
||||
1. Jim N. Gray and Leslie Lamport: “[Consensus on Transaction Commit](http://db.cs.berkeley.edu/cs286/papers/paxoscommit-tods2006.pdf),” *ACM Transactions on Database Systems* (TODS), volume 31, number 1, pages 133–160, March 2006. [doi:10.1145/1132863.1132867](http://dx.doi.org/10.1145/1132863.1132867)
|
||||
|
||||
1. Rachid Guerraoui: “[Revisiting the Relationship Between Non-Blocking Atomic Commitment and Consensus](https://pdfs.semanticscholar.org/5d06/489503b6f791aa56d2d7942359c2592e44b0.pdf),” at *9th International Workshop on Distributed Algorithms* (WDAG), September 1995. [doi:10.1007/BFb0022140](http://dx.doi.org/10.1007/BFb0022140)
|
||||
|
||||
1. Thanumalayan Sankaranarayana Pillai, Vijay Chidambaram, Ramnatthan Alagappan, et al.: “[All File Systems Are Not Created Equal: On the Complexity of Crafting Crash-Consistent Applications](http://research.cs.wisc.edu/wind/Publications/alice-osdi14.pdf),”
|
||||
at *11th USENIX Symposium on Operating Systems Design and Implementation* (OSDI),
|
||||
October 2014.
|
||||
|
||||
1. Jim Gray: “[The Transaction Concept: Virtues and Limitations](http://research.microsoft.com/en-us/um/people/gray/papers/theTransactionConcept.pdf),” at *7th International Conference on Very Large Data Bases* (VLDB), September 1981.
|
||||
|
||||
1. Hector Garcia-Molina and Kenneth Salem: “[Sagas](http://www.cs.cornell.edu/andru/cs711/2002fa/reading/sagas.pdf),” at *ACM International Conference on Management of Data* (SIGMOD), May 1987. [doi:10.1145/38713.38742](http://dx.doi.org/10.1145/38713.38742)
|
||||
|
||||
1. C. Mohan, Bruce G. Lindsay, and Ron Obermarck: “[Transaction Management in the R* Distributed Database Management System](https://cs.brown.edu/courses/csci2270/archives/2012/papers/dtxn/p378-mohan.pdf),” *ACM Transactions on Database Systems*, volume 11, number 4, pages 378–396, December 1986. [doi:10.1145/7239.7266](http://dx.doi.org/10.1145/7239.7266)
|
||||
|
||||
1. “[Distributed Transaction Processing: The XA Specification](http://pubs.opengroup.org/onlinepubs/009680699/toc.pdf),” X/Open Company Ltd., Technical Standard
|
||||
XO/CAE/91/300, December 1991. ISBN: 978-1-872-63024-3
|
||||
|
||||
1. Mike Spille: “[XA Exposed, Part II](http://www.jroller.com/pyrasun/entry/xa_exposed_part_ii_schwartz),” *jroller.com*, April 3, 2004.
|
||||
|
||||
1. Ivan Silva Neto and Francisco Reverbel: “[Lessons Learned from Implementing WS-Coordination and WS-AtomicTransaction](http://www.ime.usp.br/~reverbel/papers/icis2008.pdf),” at *7th IEEE/ACIS International Conference on Computer and Information Science* (ICIS), May 2008. [doi:10.1109/ICIS.2008.75](http://dx.doi.org/10.1109/ICIS.2008.75)
|
||||
|
||||
1. James E. Johnson, David E. Langworthy, Leslie Lamport, and Friedrich H. Vogt: “[Formal Specification of a Web Services Protocol](http://research.microsoft.com/en-us/um/people/lamport/pubs/wsfm-web.pdf),” at *1st International Workshop on Web Services and Formal Methods* (WS-FM), February 2004. [doi:10.1016/j.entcs.2004.02.022](http://dx.doi.org/10.1016/j.entcs.2004.02.022)
|
||||
|
||||
1. Dale Skeen: “[Nonblocking Commit Protocols](http://www.cs.utexas.edu/~lorenzo/corsi/cs380d/papers/Ske81.pdf),” at *ACM International Conference on Management of Data* (SIGMOD), April 1981. [doi:10.1145/582318.582339](http://dx.doi.org/10.1145/582318.582339)
|
||||
|
||||
1. Gregor Hohpe: “[Your Coffee Shop Doesn’t Use Two-Phase Commit](http://www.martinfowler.com/ieeeSoftware/coffeeShop.pdf),” *IEEE Software*, volume 22, number 2, pages 64–66, March 2005. [doi:10.1109/MS.2005.52](http://dx.doi.org/10.1109/MS.2005.52)
|
||||
|
||||
1. Pat Helland: “[Life Beyond Distributed Transactions: An Apostate’s Opinion](http://www-db.cs.wisc.edu/cidr/cidr2007/papers/cidr07p15.pdf),” at *3rd Biennial Conference on Innovative Data Systems Research* (CIDR), January 2007.
|
||||
|
||||
1. Jonathan Oliver: “[My Beef with MSDTC and Two-Phase Commits](http://blog.jonathanoliver.com/my-beef-with-msdtc-and-two-phase-commits/),” *blog.jonathanoliver.com*, April 4, 2011.
|
||||
|
||||
1. Oren Eini (Ahende Rahien): “[The Fallacy of Distributed Transactions](http://ayende.com/blog/167362/the-fallacy-of-distributed-transactions),” *ayende.com*, July 17, 2014.
|
||||
|
||||
1. Clemens Vasters: “[Transactions in Windows Azure (with Service Bus) – An Email Discussion](https://blogs.msdn.microsoft.com/clemensv/2012/07/30/transactions-in-windows-azure-with-service-bus-an-email-discussion/),” *vasters.com*, July 30, 2012.
|
||||
|
||||
1. “[Understanding Transactionality in Azure](https://docs.particular.net/nservicebus/azure/understanding-transactionality-in-azure),” NServiceBus Documentation, Particular Software, 2015.
|
||||
|
||||
1. Randy Wigginton, Ryan Lowe, Marcos Albe, and Fernando Ipar: “[Distributed Transactions in MySQL](https://www.percona.com/live/mysql-conference-2013/sites/default/files/slides/XA_final.pdf),” at *MySQL Conference and Expo*, April 2013.
|
||||
|
||||
1. Mike Spille: “[XA Exposed, Part I](http://www.jroller.com/pyrasun/entry/xa_exposed),” *jroller.com*, April 3, 2004.
|
||||
|
||||
1. Ajmer Dhariwal: “[Orphaned MSDTC Transactions (-2 spids)](http://www.eraofdata.com/orphaned-msdtc-transactions-2-spids/),” *eraofdata.com*, December 12, 2008.
|
||||
|
||||
1. Paul Randal: “[Real World Story of DBCC PAGE Saving the Day](http://www.sqlskills.com/blogs/paul/real-world-story-of-dbcc-page-saving-the-day/),” *sqlskills.com*, June 19, 2013.
|
||||
|
||||
1. “[in-doubt xact resolution Server Configuration Option](https://msdn.microsoft.com/en-us/library/ms179586.aspx),” SQL Server 2016 documentation, Microsoft, Inc.,
|
||||
2016.
|
||||
|
||||
1. Cynthia Dwork, Nancy Lynch, and Larry Stockmeyer: “[Consensus in the Presence of Partial Synchrony](http://www.net.t-labs.tu-berlin.de/~petr/ADC-07/papers/DLS88.pdf),” *Journal of the ACM*, volume 35, number 2, pages 288–323, April 1988. [doi:10.1145/42282.42283](http://dx.doi.org/10.1145/42282.42283)
|
||||
|
||||
1. Miguel Castro and Barbara H. Liskov: “[Practical Byzantine Fault Tolerance and Proactive Recovery](http://zoo.cs.yale.edu/classes/cs426/2012/bib/castro02practical.pdf),” *ACM Transactions on Computer Systems*, volume 20, number 4, pages 396–461, November 2002. [doi:10.1145/571637.571640](http://dx.doi.org/10.1145/571637.571640)
|
||||
|
||||
1. Brian M. Oki and Barbara H. Liskov: “[Viewstamped Replication: A New Primary Copy Method to Support Highly-Available Distributed Systems](http://www.cs.princeton.edu/courses/archive/fall11/cos518/papers/viewstamped.pdf),” at *7th ACM Symposium on Principles of Distributed Computing* (PODC), August 1988. [doi:10.1145/62546.62549](http://dx.doi.org/10.1145/62546.62549)
|
||||
|
||||
1. Barbara H. Liskov and James Cowling: “[Viewstamped Replication Revisited](http://pmg.csail.mit.edu/papers/vr-revisited.pdf),” Massachusetts Institute of Technology, Tech Report MIT-CSAIL-TR-2012-021, July 2012.
|
||||
|
||||
1. Leslie Lamport: “[The Part-Time Parliament](http://research.microsoft.com/en-us/um/people/lamport/pubs/lamport-paxos.pdf),” *ACM Transactions on Computer Systems*, volume 16, number 2, pages 133–169, May 1998. [doi:10.1145/279227.279229](http://dx.doi.org/10.1145/279227.279229)
|
||||
|
||||
1. Leslie Lamport: “[Paxos Made Simple](http://research.microsoft.com/en-us/um/people/lamport/pubs/paxos-simple.pdf),” *ACM SIGACT News*, volume 32, number 4, pages 51–58, December 2001.
|
||||
|
||||
1. Tushar Deepak Chandra, Robert Griesemer, and Joshua Redstone: “[Paxos Made Live – An Engineering Perspective](http://www.read.seas.harvard.edu/~kohler/class/08w-dsi/chandra07paxos.pdf),” at *26th ACM Symposium on Principles of Distributed Computing* (PODC), June 2007.
|
||||
|
||||
1. Robbert van Renesse: “[Paxos Made Moderately Complex](http://www.cs.cornell.edu/home/rvr/Paxos/paxos.pdf),” *cs.cornell.edu*, March 2011.
|
||||
|
||||
1. Diego Ongaro: “[Consensus: Bridging Theory and Practice](https://github.com/ongardie/dissertation),” PhD Thesis, Stanford University, August 2014.
|
||||
|
||||
1. Heidi Howard, Malte Schwarzkopf, Anil Madhavapeddy, and Jon Crowcroft: “[Raft Refloated: Do We Have Consensus?](http://www.cl.cam.ac.uk/~ms705/pub/papers/2015-osr-raft.pdf),” *ACM SIGOPS Operating Systems Review*, volume 49, number 1, pages 12–21, January 2015.
|
||||
[doi:10.1145/2723872.2723876](http://dx.doi.org/10.1145/2723872.2723876)
|
||||
|
||||
1. André Medeiros: “[ZooKeeper’s Atomic Broadcast Protocol: Theory and Practice](http://www.tcs.hut.fi/Studies/T-79.5001/reports/2012-deSouzaMedeiros.pdf),” Aalto University School of Science, March 20, 2012.
|
||||
|
||||
1. Robbert van Renesse, Nicolas Schiper, and Fred B. Schneider: “[Vive La Différence: Paxos vs. Viewstamped Replication vs. Zab](http://arxiv.org/abs/1309.5671),” *IEEE Transactions on Dependable and Secure Computing*,
|
||||
volume 12, number 4, pages 472–484, September 2014. [doi:10.1109/TDSC.2014.2355848](http://dx.doi.org/10.1109/TDSC.2014.2355848)
|
||||
|
||||
1. Will Portnoy: “[Lessons Learned from Implementing Paxos](http://blog.willportnoy.com/2012/06/lessons-learned-from-paxos.html),” *blog.willportnoy.com*, June 14, 2012.
|
||||
|
||||
1. Heidi Howard, Dahlia Malkhi, and Alexander Spiegelman: “[Flexible Paxos: Quorum Intersection Revisited](https://arxiv.org/abs/1608.06696),” *arXiv:1608.06696*, August 24, 2016.
|
||||
|
||||
1. Heidi Howard and Jon Crowcroft: “[Coracle: Evaluating Consensus at the Internet Edge](http://www.sigcomm.org/sites/default/files/ccr/papers/2015/August/2829988-2790010.pdf),” at *Annual Conference of the ACM Special Interest Group on Data Communication* (SIGCOMM), August 2015.
|
||||
[doi:10.1145/2829988.2790010](http://dx.doi.org/10.1145/2829988.2790010)
|
||||
|
||||
1. Kyle Kingsbury: “[Call Me Maybe: Elasticsearch 1.5.0](https://aphyr.com/posts/323-call-me-maybe-elasticsearch-1-5-0),” *aphyr.com*, April 27, 2015.
|
||||
|
||||
1. Ivan Kelly: “[BookKeeper Tutorial](https://github.com/ivankelly/bookkeeper-tutorial),” *github.com*, October 2014.
|
||||
|
||||
1. Camille Fournier: “[Consensus Systems for the Skeptical Architect](http://www.ustream.tv/recorded/61483409),” at *Craft Conference*, Budapest, Hungary, April 2015.
|
||||
|
||||
1. Kenneth P. Birman: “[A History of the Virtual Synchrony Replication Model](https://www.truststc.org/pubs/713.html),” in *Replication: Theory and Practice*, Springer LNCS volume 5959, chapter 6, pages 91–120, 2010. ISBN: 978-3-642-11293-5, [doi:10.1007/978-3-642-11294-2_6](http://dx.doi.org/10.1007/978-3-642-11294-2_6)
|
25
en-us/colophon.md
Normal file
25
en-us/colophon.md
Normal file
@ -0,0 +1,25 @@
|
||||
# Colophon
|
||||
|
||||
## About the Author
|
||||
|
||||
**Martin Kleppmann** is a researcher in distributed systems at the University of Cam‐ bridge, UK. Previously he was a software engineer and entrepreneur at internet com‐ panies including LinkedIn and Rapportive, where he worked on large-scale data infrastructure. In the process he learned a few things the hard way, and he hopes this book will save you from repeating the same mistakes.
|
||||
|
||||
Martin is a regular conference speaker, blogger, and open source contributor. He believes that profound technical ideas should be accessible to everyone, and that deeper understanding will help us develop better software.
|
||||
|
||||
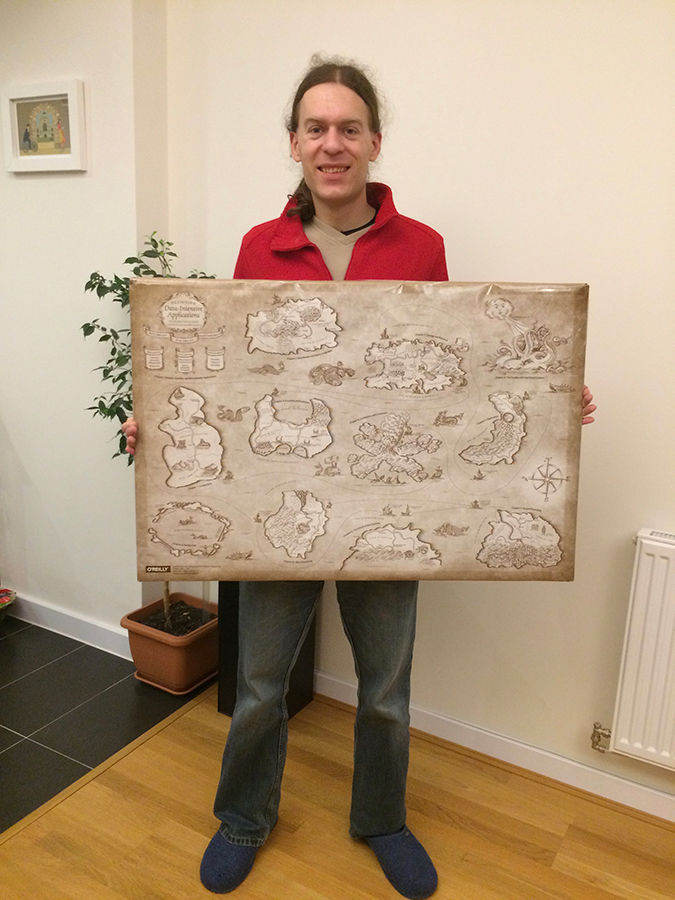
|
||||
|
||||
|
||||
|
||||
## Colophon
|
||||
|
||||
The animal on the cover of *Designing Data-Intensive Applications* is an Indian wild boar (*Sus scrofa cristatus*), a subspecies of wild boar found in India, Myanmar, Nepal, Sri Lanka, and Thailand. They are distinctive from European boars in that they have higher back bristles, no woolly undercoat, and a larger, straighter skull.
|
||||
|
||||
The Indian wild boar has a coat of gray or black hair, with stiff bristles running along the spine. Males have protruding canine teeth (called tushes) that are used to fight with rivals or fend off predators. Males are larger than females, but the species aver‐ ages 33–35 inches tall at the shoulder and 200–300 pounds in weight. Their natural predators include bears, tigers, and various big cats.
|
||||
|
||||
These animals are nocturnal and omnivorous—they eat a wide variety of things, including roots, insects, carrion, nuts, berries, and small animals. Wild boars are also known to root through garbage and crop fields, causing a great deal of destruction and earning the enmity of farmers. They need to eat 4,000–4,500 calories a day. Boars have a well-developed sense of smell, which helps them forage for underground plant material and burrowing animals. However, their eyesight is poor.
|
||||
|
||||
Wild boars have long held significance in human culture. In Hindu lore, the boar is an avatar of the god Vishnu. In ancient Greek funerary monuments, it was a symbol of a gallant loser (in contrast to the victorious lion). Due to its aggression, it was depicted on the armor and weapons of Scandinavian, Germanic, and Anglo-Saxon warriors. In the Chinese zodiac, it symbolizes determination and impetuosity.
|
||||
|
||||
Many of the animals on O’Reilly covers are endangered; all of them are important to the world. To learn more about how you can help, go to *animals.oreilly.com*.
|
||||
|
||||
The cover image is from Shaw’s *Zoology*. The cover fonts are URW Typewriter and Guardian Sans. The text font is Adobe Minion Pro; the font in diagrams is Adobe Myriad Pro; the heading font is Adobe Myriad Condensed; and the code font is Dal‐ ton Maag’s Ubuntu Mono.
|
243
en-us/glossary.md
Normal file
243
en-us/glossary.md
Normal file
@ -0,0 +1,243 @@
|
||||
# Glossary
|
||||
|
||||
> Please note that the definitions in this glossary are short and sim‐ ple, intended to convey the core idea but not the full subtleties of a term. For more detail, please follow the references into the main text.
|
||||
|
||||
[TOC]
|
||||
|
||||
|
||||
### asynchronous
|
||||
|
||||
Not waiting for something to complete (e.g., sending data over the network to another node), and not making any assumptions about how long it is going to take. See “Synchronous Versus Asynchro‐ nous Replication” on page 153, “Synchro‐ nous Versus Asynchronous Networks” on page 284, and “System Model and Reality” on page 306.
|
||||
|
||||
### atomic
|
||||
|
||||
1. In the context of concurrent operations: describing an operation that appears to take effect at a single point in time, so another concurrent process can never encounter the operation in a “half- finished” state. See also *isolation*.
|
||||
2. In the context of transactions: grouping together a set of writes that must either all be committed or all be rolled back, even if faults occur. See “Atomicity” on page 223 and “Atomic Commit and Two-Phase Commit (2PC)” on page 354.
|
||||
|
||||
### backpressure
|
||||
|
||||
Forcing the sender of some data to slow down because the recipient cannot keep
|
||||
|
||||
up with it. Also known as *flow control*. See “Messaging Systems” on page 441.
|
||||
|
||||
### batch process
|
||||
|
||||
A computation that takes some fixed (and usually large) set of data as input and pro‐ duces some other data as output, without modifying the input. See Chapter 10.
|
||||
|
||||
### bounded
|
||||
|
||||
Having some known upper limit or size. Used for example in the context of net‐ work delay (see “Timeouts and Unboun‐ ded Delays” on page 281) and datasets (see the introduction to Chapter 11).
|
||||
|
||||
### Byzantine fault
|
||||
|
||||
A node that behaves incorrectly in some arbitrary way, for example by sending contradictory or malicious messages to other nodes. See “Byzantine Faults” on page 304.
|
||||
|
||||
### cache
|
||||
|
||||
A component that remembers recently used data in order to speed up future reads of the same data. It is generally not complete: thus, if some data is missing from the cache, it has to be fetched from some underlying, slower data storage system that has a complete copy of the data.
|
||||
|
||||
### CAP theorem
|
||||
|
||||
A widely misunderstood theoretical result that is not useful in practice. See “The CAP theorem” on page 336.
|
||||
|
||||
### causality
|
||||
|
||||
The dependency between events that ari‐ ses when one thing “happens before” another thing in a system. For example, a later event that is in response to an earlier event, or builds upon an earlier event, or should be understood in the light of an earlier event. See “The “happens-before” relationship and concurrency” on page 186 and “Ordering and Causality” on page 339.
|
||||
|
||||
### consensus
|
||||
|
||||
A fundamental problem in distributed computing, concerning getting several nodes to agree on something (for exam‐ ple, which node should be the leader for a database cluster). The problem is much harder than it seems at first glance. See “Fault-Tolerant Consensus” on page 364.
|
||||
|
||||
### data warehouse
|
||||
|
||||
A database in which data from several dif‐ ferent OLTP systems has been combined and prepared to be used for analytics pur‐ poses. See “Data Warehousing” on page 91.
|
||||
|
||||
### declarative
|
||||
|
||||
Describing the properties that something should have, but not the exact steps for how to achieve it. In the context of quer‐ ies, a query optimizer takes a declarative query and decides how it should best be executed. See “Query Languages for Data” on page 42.
|
||||
|
||||
### denormalize
|
||||
|
||||
To introduce some amount of redun‐ dancy or duplication in a *normalized* dataset, typically in the form of a *cache* or *index*, in order to speed up reads. A denormalized value is a kind of precom‐ puted query result, similar to a materialized view. See “Single-Object and Multi- Object Operations” on page 228 and “Deriving several views from the same event log” on page 461.
|
||||
|
||||
### derived data
|
||||
|
||||
A dataset that is created from some other data through a repeatable process, which you could run again if necessary. Usually, derived data is needed to speed up a par‐ ticular kind of read access to the data. Indexes, caches, and materialized views are examples of derived data. See the introduction to Part III.
|
||||
|
||||
### deterministic
|
||||
|
||||
Describing a function that always pro‐ duces the same output if you give it the same input. This means it cannot depend on random numbers, the time of day, net‐ work communication, or other unpredict‐ able things.
|
||||
|
||||
### distributed
|
||||
|
||||
Running on several nodes connected by a network. Characterized by *partial failures*: some part of the system may be broken while other parts are still working, and it is often impossible for the software to know what exactly is broken. See “Faults and Partial Failures” on page 274.
|
||||
|
||||
### durable
|
||||
|
||||
Storing data in a way such that you believe it will not be lost, even if various faults occur. See “Durability” on page 226.
|
||||
|
||||
### ETL
|
||||
|
||||
Extract–Transform–Load. The process of extracting data from a source database, transforming it into a form that is more suitable for analytic queries, and loading it into a data warehouse or batch processing system. See “Data Warehousing” on page 91.
|
||||
|
||||
### failover
|
||||
|
||||
In systems that have a single leader, fail‐ over is the process of moving the leader‐ ship role from one node to another. See “Handling Node Outages” on page 156.
|
||||
|
||||
### fault-tolerant
|
||||
|
||||
Able to recover automatically if some‐ thing goes wrong (e.g., if a machine crashes or a network link fails). See “Reli‐ ability” on page 6.
|
||||
|
||||
### flow control
|
||||
|
||||
See *backpressure*.
|
||||
|
||||
### follower
|
||||
|
||||
A replica that does not directly accept any writes from clients, but only processes data changes that it receives from a leader. Also known as a *secondary*, *slave*, *read replica*, or *hot standby*. See “Leaders and Followers” on page 152.
|
||||
|
||||
### full-text search
|
||||
|
||||
Searching text by arbitrary keywords, often with additional features such as matching similarly spelled words or syno‐ nyms. A full-text index is a kind of *secon‐ dary index* that supports such queries. See “Full-text search and fuzzy indexes” on page 88.
|
||||
|
||||
### graph
|
||||
|
||||
A data structure consisting of *vertices* (things that you can refer to, also known as *nodes* or *entities*) and *edges* (connec‐ tions from one vertex to another, also known as *relationships* or *arcs*). See “Graph-Like Data Models” on page 49.
|
||||
|
||||
### hash
|
||||
|
||||
A function that turns an input into a random-looking number. The same input always returns the same number as out‐ put. Two different inputs are very likely to have two different numbers as output, although it is possible that two different inputs produce the same output (this is called a *collision*). See “Partitioning by Hash of Key” on page 203.
|
||||
|
||||
### idempotent
|
||||
|
||||
Describing an operation that can be safely retried; if it is executed more than once, it has the same effect as if it was only exe‐ cuted once. See “Idempotence” on page 478.
|
||||
|
||||
### index
|
||||
|
||||
A data structure that lets you efficiently search for all records that have a particular value in a particular field. See “Data Structures That Power Your Database” on page 70.
|
||||
|
||||
### isolation
|
||||
|
||||
In the context of transactions, describing the degree to which concurrently execut‐ ing transactions can interfere with each other. *Serializable* isolation provides the strongest guarantees, but weaker isolation levels are also used. See “Isolation” on page 225.
|
||||
|
||||
### join
|
||||
|
||||
To bring together records that have some‐ thing in common. Most commonly used in the case where one record has a refer‐ ence to another (a foreign key, a docu‐ ment reference, an edge in a graph) and a query needs to get the record that the ref‐ erence points to. See “Many-to-One and Many-to-Many Relationships” on page 33 and “Reduce-Side Joins and Grouping” on page 403.
|
||||
|
||||
### leader
|
||||
|
||||
When data or a service is replicated across several nodes, the leader is the designated replica that is allowed to make changes. A leader may be elected through some pro‐ tocol, or manually chosen by an adminis‐ trator. Also known as the *primary* or *master*. See “Leaders and Followers” on page 152.
|
||||
|
||||
### linearizable
|
||||
|
||||
Behaving as if there was only a single copy of data in the system, which is updated by atomic operations. See “Linearizability” on page 324.
|
||||
|
||||
### locality
|
||||
|
||||
A performance optimization: putting sev‐ eral pieces of data in the same place if they are frequently needed at the same time. See “Data locality for queries” on page 41.
|
||||
|
||||
### lock
|
||||
|
||||
A mechanism to ensure that only one thread, node, or transaction can access something, and anyone else who wants to access the same thing must wait until the lock is released. See “Two-Phase Locking (2PL)” on page 257 and “The leader and the lock” on page 301.
|
||||
|
||||
### log
|
||||
|
||||
A mechanism to ensure that only one thread, node, or transaction can access something, and anyone else who wants to access the same thing must wait until the lock is released. See “Two-Phase Locking (2PL)” on page 257 and “The leader and the lock” on page 301.
|
||||
|
||||
|
||||
### materialize
|
||||
To perform a computation eagerly and write out its result, as opposed to calculat‐ ing it on demand when requested. See “Aggregation: Data Cubes and Material‐ ized Views” on page 101 and “Materialization of Intermediate State” on page 419.
|
||||
|
||||
|
||||
### node
|
||||
|
||||
An instance of some software running on a computer, which communicates with other nodes via a network in order to accomplish some task.
|
||||
|
||||
|
||||
### normalized
|
||||
An instance of some software running on a computer, which communicates with other nodes via a network in order to accomplish some task.
|
||||
Structured in such a way that there is no redundancy or duplication. In a normal‐ ized database, when some piece of data changes, you only need to change it in one place, not many copies in many different places. See “Many-to-One and Many-to- Many Relationships” on page 33.
|
||||
|
||||
### OLAP
|
||||
Online analytic processing. Access pattern characterized by aggregating (e.g., count, sum, average) over a large number of records. See “Transaction Processing or Analytics?” on page 90.
|
||||
|
||||
|
||||
### OLTP
|
||||
|
||||
Online transaction processing. Access pattern characterized by fast queries that read or write a small number of records, usually indexed by key. See “Transaction Processing or Analytics?” on page 90.
|
||||
|
||||
### partitioning
|
||||
|
||||
Splitting up a large dataset or computa‐ tion that is too big for a single machine into smaller parts and spreading them across several machines. Also known as sharding. See Chapter 6.
|
||||
|
||||
### percentile
|
||||
A way of measuring the distribution of values by counting how many values are above or below some threshold. For example, the 95th percentile response time during some period is the time t such that 95% of requests in that period com‐ plete in less than t, and 5% take longer than t. See “Describing Performance” on page 13.
|
||||
|
||||
### primary key
|
||||
A value (typically a number or a string) that uniquely identifies a record. In many applications, primary keys are generated by the system when a record is created (e.g., sequentially or randomly); they are not usually set by users. See also secondary index.
|
||||
|
||||
### quorum
|
||||
|
||||
The minimum number of nodes that need to vote on an operation before it can be considered successful. See “Quorums for reading and writing” on page 179.
|
||||
|
||||
### rebalance
|
||||
To move data or services from one node to another in order to spread the load fairly. See “Rebalancing Partitions” on page 209.
|
||||
|
||||
### replication
|
||||
Keeping a copy of the same data on sev‐ eral nodes (replicas) so that it remains accessible if a node becomes unreachable. See Chapter 5.
|
||||
|
||||
### schema
|
||||
A description of the structure of some data, including its fields and datatypes. Whether some data conforms to a schema can be checked at various points in the data’s lifetime (see “Schema flexibility in the document model” on page 39), and a schema can change over time (see Chap‐ ter 4).
|
||||
|
||||
### secondary index
|
||||
An additional data structure that is main‐ tained alongside the primary data storage and which allows you to efficiently search for records that match a certain kind of condition. See “Other Indexing Struc‐ tures” on page 85 and “Partitioning and Secondary Indexes” on page 206.
|
||||
|
||||
### serializable
|
||||
A guarantee that if several transactions execute concurrently, they behave the same as if they had executed one at a time, in some serial order. See “Serializability” on page 251.
|
||||
|
||||
### shared-nothing
|
||||
An architecture in which independent nodes—each with their own CPUs, mem‐ ory, and disks—are connected via a con‐ ventional network, in contrast to shared- memory or shared-disk architectures. See the introduction to Part II.
|
||||
|
||||
### skew
|
||||
1. Imbalanced load across partitions, such that some partitions have lots of requests or data, and others have much less. Also known as hot spots. See “Skewed Work‐ loads and Relieving Hot Spots” on page 205 and “Handling skew” on page 407.
|
||||
2. A timing anomaly that causes events to appear in an unexpected, nonsequential order. See the discussions of read skew in “Snapshot Isolation and Repeatable Read” on page 237, write skew in “Write Skew and Phantoms” on page 246, and clock skew in “Timestamps for ordering events” on page 291.
|
||||
|
||||
### split brain
|
||||
A scenario in which two nodes simultane‐ ously believe themselves to be the leader, and which may cause system guarantees to be violated. See “Handling Node Out‐ ages” on page 156 and “The Truth Is Defined by the Majority” on page 300.
|
||||
|
||||
### stored procedure
|
||||
A way of encoding the logic of a transac‐ tion such that it can be entirely executed on a database server, without communi‐ cating back and forth with a client during the transaction. See “Actual Serial Execu‐ tion” on page 252.
|
||||
|
||||
### stream process
|
||||
A continually running computation that consumes a never-ending stream of events as input, and derives some output from it. See Chapter 11.
|
||||
|
||||
### synchronous
|
||||
The opposite of asynchronous.
|
||||
|
||||
### system of record
|
||||
A system that holds the primary, authori‐ tative version of some data, also known as the source of truth. Changes are first writ‐ ten here, and other datasets may be derived from the system of record. See the introduction to Part III.
|
||||
|
||||
### timeout
|
||||
One of the simplest ways of detecting a fault, namely by observing the lack of a response within some amount of time. However, it is impossible to know whether a timeout is due to a problem with the remote node, or an issue in the network. See “Timeouts and Unbounded Delays” on page 281.
|
||||
|
||||
### total order
|
||||
A way of comparing things (e.g., time‐ stamps) that allows you to always say which one of two things is greater and which one is lesser. An ordering in which some things are incomparable (you can‐ not say which is greater or smaller) is called a partial order. See “The causal order is not a total order” on page 341.
|
||||
|
||||
### transaction
|
||||
Grouping together several reads and writes into a logical unit, in order to sim‐ plify error handling and concurrency issues. See Chapter 7.
|
||||
|
||||
### two-phase commit (2PC)
|
||||
An algorithm to ensure that several data‐ base nodes either all commit or all abort a transaction. See “Atomic Commit and Two-Phase Commit (2PC)” on page 354.
|
||||
|
||||
### two-phase locking (2PL)
|
||||
An algorithm for achieving serializable isolation that works by a transaction acquiring a lock on all data it reads or writes, and holding the lock until the end of the transaction. See “Two-Phase Lock‐ ing (2PL)” on page 257.
|
||||
|
||||
### unbounded
|
||||
Not having any known upper limit or size. The opposite of bounded.
|
||||
|
||||
|
||||
|
||||
|
||||
|
||||
|
||||
……
|
13
en-us/part-i.md
Normal file
13
en-us/part-i.md
Normal file
@ -0,0 +1,13 @@
|
||||
# PART I: Foundations of Data Systems
|
||||
|
||||
The first four chapters go through the fundamental ideas that apply to all data sys‐ tems, whether running on a single machine or distributed across a cluster of machines:
|
||||
|
||||
1. [Chapter 1](ch1.md) introduces the terminology and approach that we’re going to use throughout this book. It examines what we actually mean by words like *reliabil‐ ity*, *scalability*, and *maintainability*, and how we can try to achieve these goals.
|
||||
|
||||
2. [Chapter 2](ch2.md) compares several different data models and query languages—the most visible distinguishing factor between databases from a developer’s point of view. We will see how different models are appropriate to different situations.
|
||||
|
||||
3. [Chapter 3](ch4.md) turns to the internals of storage engines and looks at how databases lay out data on disk. Different storage engines are optimized for different workloads, and choosing the right one can have a huge effect on performance.
|
||||
|
||||
4. [Chapter 4](ch4.md) compares various formats for data encoding (serialization) and espe‐ cially examines how they fare in an environment where application requirements change and schemas need to adapt over time.
|
||||
|
||||
Later, [Part II](part-ii.md) will turn to the particular issues of distributed data systems.
|
86
en-us/part-ii.md
Normal file
86
en-us/part-ii.md
Normal file
@ -0,0 +1,86 @@
|
||||
# PART II: Distributed Data
|
||||
|
||||
> *For a successful technology, reality must take precedence over public relations, for nature cannot be fooled.*
|
||||
>
|
||||
> —Richard Feynman, *Rogers Commission Report* (1986)
|
||||
|
||||
-------
|
||||
|
||||
In [Part I](part-i.md) of this book, we discussed aspects of data systems that apply when data is stored on a single machine. Now, in [Part II](part-ii.md), we move up a level and ask: what hap‐ pens if multiple machines are involved in storage and retrieval of data?
|
||||
|
||||
There are various reasons why you might want to distribute a database across multi‐ ple machines:
|
||||
|
||||
***Scalability***
|
||||
|
||||
If your data volume, read load, or write load grows bigger than a single machine can handle, you can potentially spread the load across multiple machines.
|
||||
|
||||
***Fault tolerance/high availability***
|
||||
|
||||
If your application needs to continue working even if one machine (or several machines, or the network, or an entire datacenter) goes down, you can use multi‐ ple machines to give you redundancy. When one fails, another one can take over.
|
||||
|
||||
***Latency***
|
||||
|
||||
If you have users around the world, you might want to have servers at various locations worldwide so that each user can be served from a datacenter that is geo‐ graphically close to them. That avoids the users having to wait for network pack‐ ets to travel halfway around the world.
|
||||
|
||||
|
||||
|
||||
## Scaling to Higher Load
|
||||
|
||||
If all you need is to scale to higher load, the simplest approach is to buy a more pow‐ erful machine (sometimes called *vertical scaling* or *scaling up*). Many CPUs, many RAM chips, and many disks can be joined together under one operating system, and a fast interconnect allows any CPU to access any part of the memory or disk. In this kind of *shared-memory architecture*, all the components can be treated as a single machine [1].[^ii]
|
||||
|
||||
[^i]: In a large machine, although any CPU can access any part of memory, some banks of memory are closer to one CPU than to others (this is called nonuniform memory access, or NUMA [1]). To make efficient use of this architecture, the processing needs to be broken down so that each CPU mostly accesses memory that is nearby—which means that partitioning is still required, even when ostensibly running on one machine.
|
||||
|
||||
The problem with a shared-memory approach is that the cost grows faster than line‐ arly: a machine with twice as many CPUs, twice as much RAM, and twice as much disk capacity as another typically costs significantly more than twice as much. And due to bottlenecks, a machine twice the size cannot necessarily handle twice the load.
|
||||
|
||||
A shared-memory architecture may offer limited fault tolerance—high-end machines have hot-swappable components (you can replace disks, memory modules, and even CPUs without shutting down the machines)—but it is definitely limited to a single geographic location.
|
||||
|
||||
Another approach is the *shared-disk architecture*, which uses several machines with independent CPUs and RAM, but stores data on an array of disks that is shared between the machines, which are connected via a fast network.[^ii] This architecture is used for some data warehousing workloads, but contention and the overhead of lock‐ ing limit the scalability of the shared-disk approach [2].
|
||||
|
||||
[^ii]: Network Attached Storage (NAS) or Storage Area Network (SAN).
|
||||
|
||||
|
||||
|
||||
### Shared-Nothing Architectures
|
||||
|
||||
By contrast, *shared-nothing architectures* [3] (sometimes called *horizontal scaling* or *scaling out*) have gained a lot of popularity. In this approach, each machine or virtual machine running the database software is called a *node*. Each node uses its CPUs, RAM, and disks independently. Any coordination between nodes is done at the soft‐ ware level, using a conventional network.
|
||||
|
||||
No special hardware is required by a shared-nothing system, so you can use whatever machines have the best price/performance ratio. You can potentially distribute data across multiple geographic regions, and thus reduce latency for users and potentially be able to survive the loss of an entire datacenter. With cloud deployments of virtual machines, you don’t need to be operating at Google scale: even for small companies, a multi-region distributed architecture is now feasible.
|
||||
|
||||
In this part of the book, we focus on shared-nothing architectures—not because they are necessarily the best choice for every use case, but rather because they require the most caution from you, the application developer. If your data is distributed across multiple nodes, you need to be aware of the constraints and trade-offs that occur in such a distributed system—the database cannot magically hide these from you.
|
||||
|
||||
While a distributed shared-nothing architecture has many advantages, it usually also incurs additional complexity for applications and sometimes limits the expressive‐ ness of the data models you can use. In some cases, a simple single-threaded program can perform significantly better than a cluster with over 100 CPU cores [4]. On the other hand, shared-nothing systems can be very powerful. The next few chapters go into details on the issues that arise when data is distributed.
|
||||
|
||||
### Replication Versus Partitioning
|
||||
|
||||
There are two common ways data is distributed across multiple nodes:
|
||||
|
||||
***Replication***
|
||||
|
||||
Keeping a copy of the same data on several different nodes, potentially in differ‐ ent locations. Replication provides redundancy: if some nodes are unavailable, the data can still be served from the remaining nodes. Replication can also help improve performance. We discuss replication in [Chapter 5](ch5.md).
|
||||
|
||||
***Partitioning***
|
||||
|
||||
Splitting a big database into smaller subsets called *partitions* so that different par‐ titions can be assigned to different nodes (also known as *sharding*). We discuss partitioning in [Chapter 6](ch6.md).
|
||||
|
||||
These are separate mechanisms, but they often go hand in hand, as illustrated in Figure II-1.
|
||||
|
||||

|
||||
|
||||
> *Figure II-1. A database split into two partitions, with two replicas per partition.*
|
||||
|
||||
With an understanding of those concepts, we can discuss the difficult trade-offs that you need to make in a distributed system. We’ll discuss *transactions* in Chapter 7, as that will help you understand all the many things that can go wrong in a data system, and what you can do about them. We’ll conclude this part of the book by discussing the fundamental limitations of distributed systems in Chapters 8 and 9.
|
||||
|
||||
Later, in Part III of this book, we will discuss how you can take several (potentially distributed) datastores and integrate them into a larger system, satisfying the needs of a complex application. But first, let’s talk about distributed data.
|
||||
|
||||
|
||||
|
||||
## References
|
||||
|
||||
1. Ulrich Drepper: “[What Every Programmer Should Know About Memory](https://people.freebsd.org/~lstewart/articles/cpumemory.pdf),” akka‐dia.org, November 21, 2007.
|
||||
|
||||
2. Ben Stopford: “[Shared Nothing vs. Shared Disk Architectures: An Independent View](http://www.benstopford.com/2009/11/24/understanding-the-shared-nothing-architecture/),” benstopford.com, November 24, 2009.
|
||||
|
||||
|
||||
3. Michael Stonebraker: “[The Case for Shared Nothing](http://db.cs.berkeley.edu/papers/hpts85-nothing.pdf),” IEEE Database EngineeringBulletin, volume 9, number 1, pages 4–9, March 1986.
|
||||
|
||||
4. Frank McSherry, Michael Isard, and Derek G. Murray: “[Scalability! But at What COST?](http://www.frankmcsherry.org/assets/COST.pdf),” at 15th USENIX Workshop on Hot Topics in Operating Systems (HotOS),May 2015.
|
38
en-us/part-iii.md
Normal file
38
en-us/part-iii.md
Normal file
@ -0,0 +1,38 @@
|
||||
# PART III: Derived Data
|
||||
|
||||
In Parts [I](part-i.md) and [II](part-ii.md) of this book, we assembled from the ground up all the major consid‐ erations that go into a distributed database, from the layout of data on disk all the way to the limits of distributed consistency in the presence of faults. However, this discussion assumed that there was only one database in the application.
|
||||
|
||||
In reality, data systems are often more complex. In a large application you often need to be able to access and process data in many different ways, and there is no one data‐ base that can satisfy all those different needs simultaneously. Applications thus com‐ monly use a combination of several different datastores, indexes, caches, analytics systems, etc. and implement mechanisms for moving data from one store to another.
|
||||
|
||||
In this final part of the book, we will examine the issues around integrating multiple different data systems, potentially with different data models and optimized for dif‐ ferent access patterns, into one coherent application architecture. This aspect of system-building is often overlooked by vendors who claim that their product can sat‐ isfy all your needs. In reality, integrating disparate systems is one of the most impor‐ tant things that needs to be done in a nontrivial application.
|
||||
|
||||
## Systems of Record and Derived Data
|
||||
|
||||
On a high level, systems that store and process data can be grouped into two broad categories:
|
||||
|
||||
|
||||
|
||||
***Systems of record***
|
||||
|
||||
A system of record, also known as *source of truth*, holds the authoritative version of your data. When new data comes in, e.g., as user input, it is first written here. Each fact is represented exactly once (the representation is typically *normalized*). If there is any discrepancy between another system and the system of record, then the value in the system of record is (by definition) the correct one.
|
||||
|
||||
***Derived data systems***
|
||||
|
||||
Data in a derived system is the result of taking some existing data from another system and transforming or processing it in some way. If you lose derived data, you can recreate it from the original source. A classic example is a cache: data can be served from the cache if present, but if the cache doesn’t contain what you need, you can fall back to the underlying database. Denormalized values, indexes, and materialized views also fall into this category. In recommendation systems, predictive summary data is often derived from usage logs.
|
||||
|
||||
|
||||
|
||||
Technically speaking, derived data is *redundant*, in the sense that it duplicates exist‐ ing information. However, it is often essential for getting good performance on read queries. It is commonly *denormalized*. You can derive several different datasets from a single source, enabling you to look at the data from different “points of view.”
|
||||
|
||||
Not all systems make a clear distinction between systems of record and derived data in their architecture, but it’s a very helpful distinction to make, because it clarifies the dataflow through your system: it makes explicit which parts of the system have which inputs and which outputs, and how they depend on each other.
|
||||
|
||||
Most databases, storage engines, and query languages are not inherently either a sys‐ tem of record or a derived system. A database is just a tool: how you use it is up to you. The distinction between system of record and derived data system depends not on the tool, but on how you use it in your application.
|
||||
|
||||
By being clear about which data is derived from which other data, you can bring clarity to an otherwise confusing system architecture. This point will be a running theme throughout this part of the book.
|
||||
|
||||
|
||||
|
||||
## Overview of Chapters
|
||||
|
||||
We will start in [Chapter 10](ch10.md) by examining batch-oriented dataflow systems such as MapReduce, and see how they give us good tools and principles for building large- scale data systems. In [Chapter 11](ch11.md) we will take those ideas and apply them to data streams, which allow us to do the same kinds of things with lower delays. [Chapter 12](ch12.md) concludes the book by exploring ideas about how we might use these tools to build reliable, scalable, and maintainable applications in the future.
|
||||
|
124
en-us/preface.md
Normal file
124
en-us/preface.md
Normal file
@ -0,0 +1,124 @@
|
||||
# Preface
|
||||
|
||||
If you have worked in software engineering in recent years, especially in server-side and backend systems, you have probably been bombarded with a plethora of buzz‐ words relating to storage and processing of data. NoSQL! Big Data! Web-scale! Sharding! Eventual consistency! ACID! CAP theorem! Cloud services! MapReduce! Real-time!
|
||||
|
||||
In the last decade we have seen many interesting developments in databases, in dis‐ tributed systems, and in the ways we build applications on top of them. There are various driving forces for these developments:
|
||||
|
||||
- Internet companies such as Google, Yahoo!, Amazon, Facebook, LinkedIn, Microsoft, and Twitter are handling huge volumes of data and traffic, forcing them to create new tools that enable them to efficiently handle such scale.
|
||||
- Businesses need to be agile, test hypotheses cheaply, and respond quickly to new market insights by keeping development cycles short and data models flexible.
|
||||
- Free and open source software has become very successful and is now preferred to commercial or bespoke in-house software in many environments.
|
||||
- CPU clock speeds are barely increasing, but multi-core processors are standard, and networks are getting faster. This means parallelism is only going to increase.
|
||||
- Even if you work on a small team, you can now build systems that are distributed across many machines and even multiple geographic regions, thanks to infra‐ structure as a service (IaaS) such as Amazon Web Services.
|
||||
- Many services are now expected to be highly available; extended downtime due to outages or maintenance is becoming increasingly unacceptable.
|
||||
|
||||
*Data-intensive applications* are pushing the boundaries of what is possible by making use of these technological developments. We call an application *data-intensive* if data is its primary challenge—the quantity of data, the complexity of data, or the speed at which it is changing—as opposed to *compute-intensive*, where CPU cycles are the bottleneck.
|
||||
|
||||
The tools and technologies that help data-intensive applications store and process data have been rapidly adapting to these changes. New types of database systems (“NoSQL”) have been getting lots of attention, but message queues, caches, search indexes, frameworks for batch and stream processing, and related technologies are very important too. Many applications use some combination of these.
|
||||
|
||||
The buzzwords that fill this space are a sign of enthusiasm for the new possibilities, which is a great thing. However, as software engineers and architects, we also need to have a technically accurate and precise understanding of the various technologies and their trade-offs if we want to build good applications. For that understanding, we have to dig deeper than buzzwords.
|
||||
|
||||
Fortunately, behind the rapid changes in technology, there are enduring principles that remain true, no matter which version of a particular tool you are using. If you understand those principles, you’re in a position to see where each tool fits in, how to make good use of it, and how to avoid its pitfalls. That’s where this book comes in.
|
||||
|
||||
The goal of this book is to help you navigate the diverse and fast-changing landscape of technologies for processing and storing data. This book is not a tutorial for one particular tool, nor is it a textbook full of dry theory. Instead, we will look at examples of successful data systems: technologies that form the foundation of many popular applications and that have to meet scalability, performance, and reliability require‐ ments in production every day.
|
||||
|
||||
We will dig into the internals of those systems, tease apart their key algorithms, dis‐ cuss their principles and the trade-offs they have to make. On this journey, we will try to find useful ways of *thinking about* data systems—not just *how* they work, but also *why* they work that way, and what questions we need to ask.
|
||||
|
||||
After reading this book, you will be in a great position to decide which kind of tech‐ nology is appropriate for which purpose, and understand how tools can be combined to form the foundation of a good application architecture. You won’t be ready to build your own database storage engine from scratch, but fortunately that is rarely necessary. You will, however, develop a good intuition for what your systems are doing under the hood so that you can reason about their behavior, make good design decisions, and track down any problems that may arise.
|
||||
|
||||
|
||||
|
||||
## Who Should Read This Book?
|
||||
|
||||
If you develop applications that have some kind of server/backend for storing or pro‐ cessing data, and your applications use the internet (e.g., web applications, mobile apps, or internet-connected sensors), then this book is for you.
|
||||
|
||||
This book is for software engineers, software architects, and technical managers who love to code. It is especially relevant if you need to make decisions about the architec‐ ture of the systems you work on—for example, if you need to choose tools for solving a given problem and figure out how best to apply them. But even if you have no choice over your tools, this book will help you better understand their strengths and weaknesses.
|
||||
|
||||
You should have some experience building web-based applications or network serv‐ ices, and you should be familiar with relational databases and SQL. Any non- relational databases and other data-related tools you know are a bonus, but not required. A general understanding of common network protocols like TCP and HTTP is helpful. Your choice of programming language or framework makes no dif‐ ference for this book.
|
||||
|
||||
If any of the following are true for you, you’ll find this book valuable:
|
||||
|
||||
- You want to learn how to make data systems scalable, for example, to support web or mobile apps with millions of users.
|
||||
- You need to make applications highly available (minimizing downtime) and operationally robust.
|
||||
- You are looking for ways of making systems easier to maintain in the long run, even as they grow and as requirements and technologies change.
|
||||
- You have a natural curiosity for the way things work and want to know what goes on inside major websites and online services. This book breaks down the internals of various databases and data processing systems, and it’s great fun to explore the bright thinking that went into their design.
|
||||
|
||||
Sometimes, when discussing scalable data systems, people make comments along the lines of, “You’re not Google or Amazon. Stop worrying about scale and just use a relational database.” There is truth in that statement: building for scale that you don’t need is wasted effort and may lock you into an inflexible design. In effect, it is a form of premature optimization. However, it’s also important to choose the right tool for the job, and different technologies each have their own strengths and weaknesses. As we shall see, relational databases are important but not the final word on dealing with data.
|
||||
|
||||
|
||||
|
||||
## Scope of This Book
|
||||
|
||||
This book does not attempt to give detailed instructions on how to install or use spe‐ cific software packages or APIs, since there is already plenty of documentation for those things. Instead we discuss the various principles and trade-offs that are funda‐ mental to data systems, and we explore the different design decisions taken by differ‐ ent products.
|
||||
|
||||
In the ebook editions we have included links to the full text of online resources. All links were verified at the time of publication, but unfortunately links tend to break frequently due to the nature of the web. If you come across a broken link, or if you are reading a print copy of this book, you can look up references using a search engine. For academic papers, you can search for the title in Google Scholar to find open-access PDF files. Alternatively, you can find all of the references at [*https:// github.com/ept/ddia-references*](https:// github.com/ept/ddia-references), where we maintain up-to-date links.
|
||||
|
||||
We look primarily at the *architecture* of data systems and the ways they are integrated into data-intensive applications. This book doesn’t have space to cover deployment, operations, security, management, and other areas—those are complex and impor‐ tant topics, and we wouldn’t do them justice by making them superficial side notes in this book. They deserve books of their own.
|
||||
|
||||
Many of the technologies described in this book fall within the realm of the *Big Data* buzzword. However, the term “Big Data” is so overused and underdefined that it is not useful in a serious engineering discussion. This book uses less ambiguous terms, such as single-node versus distributed systems, or online/interactive versus offline/ batch processing systems.
|
||||
|
||||
This book has a bias toward free and open source software (FOSS), because reading, modifying, and executing source code is a great way to understand how something works in detail. Open platforms also reduce the risk of vendor lock-in. However, where appropriate, we also discuss proprietary software (closed-source software, soft‐ ware as a service, or companies’ in-house software that is only described in literature but not released publicly).
|
||||
|
||||
|
||||
## Outline of This Book
|
||||
|
||||
This book is arranged into three parts:
|
||||
|
||||
1. In [Part I](part-i.md), we discuss the fundamental ideas that underpin the design of data- intensive applications. We start in [Chapter 1](ch1.md) by discussing what we’re actually trying to achieve: reliability, scalability, and maintainability; how we need to think about them; and how we can achieve them. In [Chapter 2](ch2.md) we compare sev‐ eral different data models and query languages, and see how they are appropriate to different situations. In [Chapter 3](ch3.md) we talk about storage engines: how databases arrange data on disk so that we can find it again efficiently. [Chapter 4](ch4.md) turns to formats for data encoding (serialization) and evolution of schemas over time.
|
||||
2. [In Part II](part-ii.md), we move from data stored on one machine to data that is distributed across multiple machines. This is often necessary for scalability, but brings with it a variety of unique challenges. We first discuss replication ([Chapter 5](ch5.md)), parti‐ tioning/sharding ([Chapter 6](ch6.md)), and transactions ([Chapter 7](ch7.md)). We then go into more detail on the problems with distributed systems ([Chapter 8](ch8.md)) and what it means to achieve consistency and consensus in a distributed system ([Chapter 9](ch9.md)).
|
||||
|
||||
3. In [Part III](part-iii.md), we discuss systems that derive some datasets from other datasets. Derived data often occurs in heterogeneous systems: when there is no one data‐ base that can do everything well, applications need to integrate several different databases, caches, indexes, and so on. In [Chapter 10](ch10.md) we start with a batch pro‐ cessing approach to derived data, and we build upon it with stream processing in [Chapter 11](ch11.md). Finally, in [Chapter 12](ch12.md) we put everything together and discuss approaches for building reliable, scalable, and maintainable applications in the future.
|
||||
|
||||
|
||||
|
||||
## References and Further Reading
|
||||
|
||||
Most of what we discuss in this book has already been said elsewhere in some form or another—in conference presentations, research papers, blog posts, code, bug trackers, mailing lists, and engineering folklore. This book summarizes the most important ideas from many different sources, and it includes pointers to the original literature throughout the text. The references at the end of each chapter are a great resource if you want to explore an area in more depth, and most of them are freely available online.
|
||||
|
||||
|
||||
|
||||
## O'Reilly Safari
|
||||
|
||||
[Safari](http://oreilly.com/safari) (formerly Safari Books Online) is a membership-based training and reference platform for enterprise, government, educators, and individuals.
|
||||
|
||||
Members have access to thousands of books, training videos, Learning Paths, interac‐ tive tutorials, and curated playlists from over 250 publishers, including O’Reilly Media, Harvard Business Review, Prentice Hall Professional, Addison-Wesley Pro‐ fessional, Microsoft Press, Sams, Que, Peachpit Press, Adobe, Focal Press, Cisco Press, John Wiley & Sons, Syngress, Morgan Kaufmann, IBM Redbooks, Packt, Adobe Press, FT Press, Apress, Manning, New Riders, McGraw-Hill, Jones & Bartlett, and Course Technology, among others.
|
||||
|
||||
For more information, please visit http://oreilly.com/safari.
|
||||
|
||||
|
||||
|
||||
## How to Contact Us
|
||||
|
||||
Please address comments and questions concerning this book to the publisher:
|
||||
|
||||
O’Reilly Media, Inc.
|
||||
1005 Gravenstein Highway North
|
||||
Sebastopol, CA 95472
|
||||
800-998-9938 (in the United States or Canada) 707-829-0515 (international or local) 707-829-0104 (fax)
|
||||
|
||||
We have a web page for this book, where we list errata, examples, and any additional information. You can access this page at *http://bit.ly/designing-data-intensive-apps*.
|
||||
|
||||
To comment or ask technical questions about this book, send email to *bookques‐ tions@oreilly.com*.
|
||||
|
||||
For more information about our books, courses, conferences, and news, see our web‐ site at *http://www.oreilly.com*.
|
||||
|
||||
* Find us on Facebook: [http://facebook.com/oreilly](http://facebook.com/oreilly)
|
||||
* Follow us on Twitter: [http://twitter.com/oreillymedia](#http://twitter.com/oreillymedia)
|
||||
* Watch us on YouTube: [http://www.youtube.com/oreillymedia](#http://www.youtube.com/oreillymedia)
|
||||
|
||||
|
||||
|
||||
## Acknowledgments
|
||||
|
||||
Acknowledgments
|
||||
|
||||
This book is an amalgamation and systematization of a large number of other peo‐ ple’s ideas and knowledge, combining experience from both academic research and industrial practice. In computing we tend to be attracted to things that are new and shiny, but I think we have a huge amount to learn from things that have been done before. This book has over 800 references to articles, blog posts, talks, documenta‐ tion, and more, and they have been an invaluable learning resource for me. I am very grateful to the authors of this material for sharing their knowledge.
|
||||
|
||||
I have also learned a lot from personal conversations, thanks to a large number of people who have taken the time to discuss ideas or patiently explain things to me. In particular, I would like to thank Joe Adler, Ross Anderson, Peter Bailis, Márton Balassi, Alastair Beresford, Mark Callaghan, Mat Clayton, Patrick Collison, Sean Cribbs, Shirshanka Das, Niklas Ekström, Stephan Ewen, Alan Fekete, Gyula Fóra, Camille Fournier, Andres Freund, John Garbutt, Seth Gilbert, Tom Haggett, Pat Hel‐ land, Joe Hellerstein, Jakob Homan, Heidi Howard, John Hugg, Julian Hyde, Conrad Irwin, Evan Jones, Flavio Junqueira, Jessica Kerr, Kyle Kingsbury, Jay Kreps, Carl Lerche, Nicolas Liochon, Steve Loughran, Lee Mallabone, Nathan Marz, Caitie, McCaffrey, Josie McLellan, Christopher Meiklejohn, Ian Meyers, Neha Narkhede, Neha Narula, Cathy O’Neil, Onora O’Neill, Ludovic Orban, Zoran Perkov, Julia Powles, Chris Riccomini, Henry Robinson, David Rosenthal, Jennifer Rullmann, Matthew Sackman, Martin Scholl, Amit Sela, Gwen Shapira, Greg Spurrier, Sam Stokes, Ben Stopford, Tom Stuart, Diana Vasile, Rahul Vohra, Pete Warden, and Brett Wooldridge.
|
||||
|
||||
Several more people have been invaluable to the writing of this book by reviewing drafts and providing feedback. For these contributions I am particularly indebted to Raul Agepati, Tyler Akidau, Mattias Andersson, Sasha Baranov, Veena Basavaraj, David Beyer, Jim Brikman, Paul Carey, Raul Castro Fernandez, Joseph Chow, Derek Elkins, Sam Elliott, Alexander Gallego, Mark Grover, Stu Halloway, Heidi Howard, Nicola Kleppmann, Stefan Kruppa, Bjorn Madsen, Sander Mak, Stefan Podkowinski, Phil Potter, Hamid Ramazani, Sam Stokes, and Ben Summers. Of course, I take all responsibility for any remaining errors or unpalatable opinions in this book.
|
||||
|
||||
For helping this book become real, and for their patience with my slow writing and unusual requests, I am grateful to my editors Marie Beaugureau, Mike Loukides, Ann Spencer, and all the team at O’Reilly. For helping find the right words, I thank Rachel Head. For giving me the time and freedom to write in spite of other work commit‐ ments, I thank Alastair Beresford, Susan Goodhue, Neha Narkhede, and Kevin Scott.
|
||||
|
||||
Very special thanks are due to Shabbir Diwan and Edie Freedman, who illustrated with great care the maps that accompany the chapters. It’s wonderful that they took on the unconventional idea of creating maps, and made them so beautiful and com‐ pelling.
|
||||
|
||||
Finally, my love goes to my family and friends, without whom I would not have been able to get through this writing process that has taken almost four years. You’re the best.
|
Loading…
Reference in New Issue
Block a user