mirror of
https://github.com/LCTT/TranslateProject.git
synced 2024-12-26 21:30:55 +08:00
217 lines
12 KiB
Markdown
217 lines
12 KiB
Markdown
[#]: collector: (lujun9972)
|
||
[#]: translator: (tanloong)
|
||
[#]: reviewer: (wxy)
|
||
[#]: publisher: (wxy)
|
||
[#]: url: (https://linux.cn/article-13628-1.html)
|
||
[#]: subject: (Machine learning made easy with Python)
|
||
[#]: via: (https://opensource.com/article/21/1/machine-learning-python)
|
||
[#]: author: (Girish Managoli https://opensource.com/users/gammay)
|
||
|
||
用 Python 轻松实现机器学习
|
||
======
|
||
|
||
> 用朴素贝叶斯分类器解决现实世界里的机器学习问题。
|
||
|
||
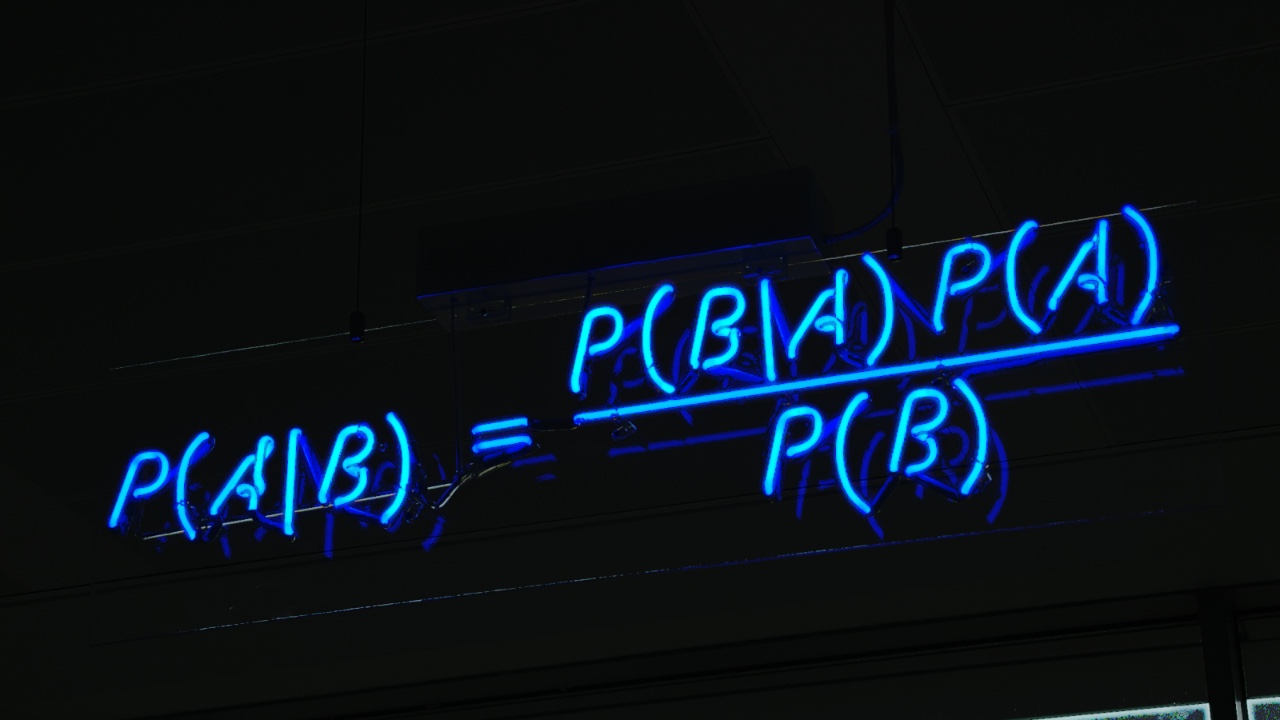
|
||
|
||
<ruby>朴素贝叶斯<rt>Naïve Bayes</rt></ruby>是一种分类技术,它是许多分类器建模算法的基础。基于朴素贝叶斯的分类器是简单、快速和易用的机器学习技术之一,而且在现实世界的应用中很有效。
|
||
|
||
朴素贝叶斯是从 <ruby>[贝叶斯定理][2]<rt>Bayes' theorem</rt></ruby> 发展来的。贝叶斯定理由 18 世纪的统计学家 [托马斯·贝叶斯][3] 提出,它根据与一个事件相关联的其他条件来计算该事件发生的概率。比如,[帕金森氏病][4] 患者通常嗓音会发生变化,因此嗓音变化就是与预测帕金森氏病相关联的症状。贝叶斯定理提供了计算目标事件发生概率的方法,而朴素贝叶斯是对该方法的推广和简化。
|
||
|
||
### 解决一个现实世界里的问题
|
||
|
||
这篇文章展示了朴素贝叶斯分类器解决现实世界问题(相对于完整的商业级应用)的能力。我会假设你对机器学习有基本的了解,所以文章里会跳过一些与机器学习预测不大相关的步骤,比如 <ruby>数据打乱<rt>date shuffling</rt></ruby> 和 <ruby>数据切片<rt>data splitting</rt></ruby>。如果你是机器学习方面的新手或者需要一个进修课程,请查看 《[An introduction to machine learning today][5]》 和 《[Getting started with open source machine learning][6]》。
|
||
|
||
朴素贝叶斯分类器是 <ruby>[有监督的][7]<rt>supervised</rt></ruby>、属于 <ruby>[生成模型][8]<rt>generative</rt></ruby> 的、非线性的、属于 <ruby>[参数模型][9]<rt>parametric</rt></ruby> 的和 <ruby>[基于概率的][10]<rt>probabilistic</rt></ruby>。
|
||
|
||
在这篇文章里,我会演示如何用朴素贝叶斯预测帕金森氏病。需要用到的数据集来自 [UCI 机器学习库][11]。这个数据集包含许多语音信号的指标,用于计算患帕金森氏病的可能性;在这个例子里我们将使用这些指标中的前 8 个:
|
||
|
||
* **MDVP:Fo(Hz)**:平均声带基频
|
||
* **MDVP:Fhi(Hz)**:最高声带基频
|
||
* **MDVP:Flo(Hz)**:最低声带基频
|
||
* **MDVP:Jitter(%)**、**MDVP:Jitter(Abs)**、**MDVP:RAP**、**MDVP:PPQ** 和 **Jitter:DDP**:5 个衡量声带基频变化的指标
|
||
|
||
这个例子里用到的数据集,可以在我的 [GitHub 仓库][12] 里找到。数据集已经事先做了打乱和切片。
|
||
|
||
### 用 Python 实现机器学习
|
||
|
||
接下来我会用 Python 来解决这个问题。我用的软件是:
|
||
|
||
* Python 3.8.2
|
||
* Pandas 1.1.1
|
||
* scikit-learn 0.22.2.post1
|
||
|
||
Python 有多个朴素贝叶斯分类器的实现,都是开源的,包括:
|
||
|
||
* **NLTK Naïve Bayes**:基于标准的朴素贝叶斯算法,用于文本分类
|
||
* **NLTK Positive Naïve Bayes**:NLTK Naïve Bayes 的变体,用于对只标注了一部分的训练集进行二分类
|
||
* **Scikit-learn Gaussian Naïve Bayes**:提供了部分拟合方法来支持数据流或很大的数据集(LCTT 译注:它们可能无法一次性导入内存,用部分拟合可以动态地增加数据)
|
||
* **Scikit-learn Multinomial Naïve Bayes**:针对离散型特征、实例计数、频率等作了优化
|
||
* **Scikit-learn Bernoulli Naïve Bayes**:用于各个特征都是二元变量/布尔特征的情况
|
||
|
||
在这个例子里我将使用 [sklearn Gaussian Naive Bayes][13]。
|
||
|
||
我的 Python 实现在 `naive_bayes_parkinsons.py` 里,如下所示:
|
||
|
||
```
|
||
import pandas as pd
|
||
|
||
# x_rows 是我们所使用的 8 个特征的列名
|
||
x_rows=['MDVP:Fo(Hz)','MDVP:Fhi(Hz)','MDVP:Flo(Hz)',
|
||
'MDVP:Jitter(%)','MDVP:Jitter(Abs)','MDVP:RAP','MDVP:PPQ','Jitter:DDP']
|
||
y_rows=['status'] # y_rows 是类别的列名,若患病,值为 1,若不患病,值为 0
|
||
|
||
# 训练
|
||
|
||
# 读取训练数据
|
||
train_data = pd.read_csv('parkinsons/Data_Parkinsons_TRAIN.csv')
|
||
train_x = train_data[x_rows]
|
||
train_y = train_data[y_rows]
|
||
print("train_x:\n", train_x)
|
||
print("train_y:\n", train_y)
|
||
|
||
# 导入 sklearn Gaussian Naive Bayes,然后进行对训练数据进行拟合
|
||
from sklearn.naive_bayes import GaussianNB
|
||
|
||
gnb = GaussianNB()
|
||
gnb.fit(train_x, train_y)
|
||
|
||
# 对训练数据进行预测
|
||
predict_train = gnb.predict(train_x)
|
||
print('Prediction on train data:', predict_train)
|
||
|
||
# 在训练数据上的准确率
|
||
from sklearn.metrics import accuracy_score
|
||
accuracy_train = accuracy_score(train_y, predict_train)
|
||
print('Accuray score on train data:', accuracy_train)
|
||
|
||
# 测试
|
||
|
||
# 读取测试数据
|
||
test_data = pd.read_csv('parkinsons/Data_Parkinsons_TEST.csv')
|
||
test_x = test_data[x_rows]
|
||
test_y = test_data[y_rows]
|
||
|
||
# 对测试数据进行预测
|
||
predict_test = gnb.predict(test_x)
|
||
print('Prediction on test data:', predict_test)
|
||
|
||
# 在测试数据上的准确率
|
||
accuracy_test = accuracy_score(test_y, predict_test)
|
||
print('Accuray score on test data:', accuracy_train)
|
||
```
|
||
|
||
运行这个 Python 脚本:
|
||
|
||
```
|
||
$ python naive_bayes_parkinsons.py
|
||
|
||
train_x:
|
||
MDVP:Fo(Hz) MDVP:Fhi(Hz) ... MDVP:RAP MDVP:PPQ Jitter:DDP
|
||
0 152.125 161.469 ... 0.00191 0.00226 0.00574
|
||
1 120.080 139.710 ... 0.00180 0.00220 0.00540
|
||
2 122.400 148.650 ... 0.00465 0.00696 0.01394
|
||
3 237.323 243.709 ... 0.00173 0.00159 0.00519
|
||
.. ... ... ... ... ... ...
|
||
155 138.190 203.522 ... 0.00406 0.00398 0.01218
|
||
|
||
[156 rows x 8 columns]
|
||
|
||
train_y:
|
||
status
|
||
0 1
|
||
1 1
|
||
2 1
|
||
3 0
|
||
.. ...
|
||
155 1
|
||
|
||
[156 rows x 1 columns]
|
||
|
||
Prediction on train data: [1 1 1 0 ... 1]
|
||
Accuracy score on train data: 0.6666666666666666
|
||
|
||
Prediction on test data: [1 1 1 1 ... 1
|
||
1 1]
|
||
Accuracy score on test data: 0.6666666666666666
|
||
```
|
||
|
||
在训练集和测试集上的准确率都是 67%。它的性能还可以进一步优化。你想尝试一下吗?你可以在下面的评论区给出你的方法。
|
||
|
||
### 背后原理
|
||
|
||
朴素贝叶斯分类器从贝叶斯定理发展来的。贝叶斯定理用于计算条件概率,或者说贝叶斯定理用于计算当与一个事件相关联的其他事件发生时,该事件发生的概率。简而言之,它解决了这个问题:_如果我们已经知道事件 x 发生在事件 y 之前的概率,那么当事件 x 再次发生时,事件 y 发生的概率是多少?_ 贝叶斯定理用一个先验的预测值来逐渐逼近一个最终的 [后验概率][14]。贝叶斯定理有一个基本假设,就是所有的参数重要性相同(LCTT 译注:即相互独立)。
|
||
|
||
贝叶斯计算主要包括以下步骤:
|
||
|
||
1. 计算总的先验概率:
|
||
$P(患病)$ 和 $P(不患病)$
|
||
2. 计算 8 种指标各自是某个值时的后验概率 (value1,...,value8 分别是 MDVP:Fo(Hz),...,Jitter:DDP 的取值):
|
||
$P(value1,\ldots,value8\ |\ 患病)$
|
||
$P(value1,\ldots,value8\ |\ 不患病)$
|
||
3. 将第 1 步和第 2 步的结果相乘,最终得到患病和不患病的后验概率:
|
||
$P(患病\ |\ value1,\ldots,value8) \propto P(患病) \times P(value1,\ldots,value8\ |\ 患病)$
|
||
$P(不患病\ |\ value1,\ldots,value8) \propto P(不患病) \times P(value1,\ldots,value8\ |\ 不患病)$
|
||
|
||
上面第 2 步的计算非常复杂,朴素贝叶斯将它作了简化:
|
||
|
||
1. 计算总的先验概率:
|
||
$P(患病)$ 和 $P(不患病)$
|
||
2. 对 8 种指标里的每个指标,计算其取某个值时的后验概率:
|
||
$P(value1\ |\ 患病),\ldots,P(value8\ |\ 患病)$
|
||
$P(value1\ |\ 不患病),\ldots,P(value8\ |\ 不患病)$
|
||
3. 将第 1 步和第 2 步的结果相乘,最终得到患病和不患病的后验概率:
|
||
$P(患病\ |\ value1,\ldots,value8) \propto P(患病) \times P(value1\ |\ 患病) \times \ldots \times P(value8\ |\ 患病)$
|
||
$P(不患病\ |\ value1,\ldots,value8) \propto P(不患病) \times P(value1\ |\ 不患病) \times \ldots \times P(value8\ |\ 不患病)$
|
||
|
||
这只是一个很初步的解释,还有很多其他因素需要考虑,比如数据类型的差异,稀疏数据,数据可能有缺失值等。
|
||
|
||
### 超参数
|
||
|
||
朴素贝叶斯作为一个简单直接的算法,不需要超参数。然而,有的版本的朴素贝叶斯实现可能提供一些高级特性(比如超参数)。比如,[GaussianNB][13] 就有 2 个超参数:
|
||
|
||
* **priors**:先验概率,可以事先指定,这样就不必让算法从数据中计算才能得出。
|
||
* **var_smoothing**:考虑数据的分布情况,当数据不满足标准的高斯分布时,这个超参数会发挥作用。
|
||
|
||
### 损失函数
|
||
|
||
为了坚持简单的原则,朴素贝叶斯使用 [0-1 损失函数][15]。如果预测结果与期望的输出相匹配,损失值为 0,否则为 1。
|
||
|
||
### 优缺点
|
||
|
||
**优点**:朴素贝叶斯是最简单、最快速的算法之一。
|
||
**优点**:在数据量较少时,用朴素贝叶斯仍可作出可靠的预测。
|
||
**缺点**:朴素贝叶斯的预测只是估计值,并不准确。它胜在速度而不是准确度。
|
||
**缺点**:朴素贝叶斯有一个基本假设,就是所有特征相互独立,但现实情况并不总是如此。
|
||
|
||
从本质上说,朴素贝叶斯是贝叶斯定理的推广。它是最简单最快速的机器学习算法之一,用来进行简单和快速的训练和预测。朴素贝叶斯提供了足够好、比较准确的预测。朴素贝叶斯假设预测特征之间是相互独立的。已经有许多朴素贝叶斯的开源的实现,它们的特性甚至超过了贝叶斯算法的实现。
|
||
|
||
--------------------------------------------------------------------------------
|
||
|
||
via: https://opensource.com/article/21/1/machine-learning-python
|
||
|
||
作者:[Girish Managoli][a]
|
||
选题:[lujun9972][b]
|
||
译者:[tanloong](https://github.com/tanloong)
|
||
校对:[wxy](https://github.com/wxy)
|
||
|
||
本文由 [LCTT](https://github.com/LCTT/TranslateProject) 原创编译,[Linux中国](https://linux.cn/) 荣誉推出
|
||
|
||
[a]: https://opensource.com/users/gammay
|
||
[b]: https://github.com/lujun9972
|
||
[1]: https://opensource.com/sites/default/files/styles/image-full-size/public/lead-images/fail_progress_cycle_momentum_arrow.png?itok=q-ZFa_Eh (arrows cycle symbol for failing faster)
|
||
[2]: https://en.wikipedia.org/wiki/Bayes%27_theorem
|
||
[3]: https://en.wikipedia.org/wiki/Thomas_Bayes
|
||
[4]: https://en.wikipedia.org/wiki/Parkinson%27s_disease
|
||
[5]: https://opensource.com/article/17/9/introduction-machine-learning
|
||
[6]: https://opensource.com/business/15/9/getting-started-open-source-machine-learning
|
||
[7]: https://en.wikipedia.org/wiki/Supervised_learning
|
||
[8]: https://en.wikipedia.org/wiki/Generative_model
|
||
[9]: https://en.wikipedia.org/wiki/Parametric_model
|
||
[10]: https://en.wikipedia.org/wiki/Probabilistic_classification
|
||
[11]: https://archive.ics.uci.edu/ml/datasets/parkinsons
|
||
[12]: https://github.com/gammay/Machine-learning-made-easy-Naive-Bayes/tree/main/parkinsons
|
||
[13]: https://scikit-learn.org/stable/modules/generated/sklearn.naive_bayes.GaussianNB.html
|
||
[14]: https://en.wikipedia.org/wiki/Posterior_probability
|
||
[15]: https://en.wikipedia.org/wiki/Loss_function#0-1_loss_function
|