mirror of
https://github.com/LCTT/TranslateProject.git
synced 2024-12-26 21:30:55 +08:00
240 lines
7.9 KiB
Markdown
240 lines
7.9 KiB
Markdown
如何将 Scikit-learn Python 库用于数据科学项目
|
||
======
|
||
|
||
> 灵活多样的 Python 库为数据分析和数据挖掘提供了强力的机器学习工具。
|
||
|
||

|
||
|
||
Scikit-learn Python 库最初于 2007 年发布,通常用于解决各种方面的机器学习和数据科学问题。这个多种功能的库提供了整洁、一致、高效的 API 和全面的在线文档。
|
||
|
||
### 什么是 Scikit-learn?
|
||
|
||
[Scikit-learn][1] 是一个开源 Python 库,拥有强大的数据分析和数据挖掘工具。 在 BSD 许可下可用,并建立在以下机器学习库上:
|
||
|
||
- `NumPy`,一个用于操作多维数组和矩阵的库。它还具有广泛的数学函数汇集,可用于执行各种计算。
|
||
- `SciPy`,一个由各种库组成的生态系统,用于完成技术计算任务。
|
||
- `Matplotlib`,一个用于绘制各种图表和图形的库。
|
||
|
||
Scikit-learn 提供了广泛的内置算法,可以充分用于数据科学项目。
|
||
|
||
以下是使用 Scikit-learn 库的主要方法。
|
||
|
||
#### 1、分类
|
||
|
||
[分类][2]工具识别与提供的数据相关联的类别。例如,它们可用于将电子邮件分类为垃圾邮件或非垃圾邮件。
|
||
|
||
Scikit-learn 中的分类算法包括:
|
||
|
||
- <ruby>支持向量机<rt>Support vector machines</rt></ruby>(SVM)
|
||
- <ruby>最邻近<rt>Nearest neighbors</rt></ruby>
|
||
- <ruby>随机森林<rt>Random forest</rt></ruby>
|
||
|
||
#### 2、回归
|
||
|
||
回归涉及到创建一个模型去试图理解输入和输出数据之间的关系。例如,回归工具可用于理解股票价格的行为。
|
||
|
||
回归算法包括:
|
||
|
||
- <ruby>支持向量机<rt>Support vector machines</rt></ruby>(SVM)
|
||
- <ruby>岭回归<rt>Ridge regression</rt></ruby>
|
||
- Lasso(LCTT 译注:Lasso 即 least absolute shrinkage and selection operator,又译为最小绝对值收敛和选择算子、套索算法)
|
||
|
||
#### 3、聚类
|
||
|
||
Scikit-learn 聚类工具用于自动将具有相同特征的数据分组。 例如,可以根据客户数据的地点对客户数据进行细分。
|
||
|
||
聚类算法包括:
|
||
|
||
- K-means
|
||
- <ruby>谱聚类<rt>Spectral clustering</rt></ruby>
|
||
- Mean-shift
|
||
|
||
#### 4、降维
|
||
|
||
降维降低了用于分析的随机变量的数量。例如,为了提高可视化效率,可能不会考虑外围数据。
|
||
|
||
降维算法包括:
|
||
|
||
- <ruby>主成分分析<rt>Principal component analysis</rt></ruby>(PCA)
|
||
- <ruby>功能选择<rt>Feature selection</rt></ruby>
|
||
- <ruby>非负矩阵分解<rt>Non-negative matrix factorization</rt></ruby>
|
||
|
||
#### 5、模型选择
|
||
|
||
模型选择算法提供了用于比较、验证和选择要在数据科学项目中使用的最佳参数和模型的工具。
|
||
|
||
通过参数调整能够增强精度的模型选择模块包括:
|
||
|
||
- <ruby>网格搜索<rt>Grid search</rt></ruby>
|
||
- <ruby>交叉验证<rt>Cross-validation</rt></ruby>
|
||
- <ruby>指标<rt>Metrics</rt></ruby>
|
||
|
||
#### 6、预处理
|
||
|
||
Scikit-learn 预处理工具在数据分析期间的特征提取和规范化中非常重要。 例如,您可以使用这些工具转换输入数据(如文本)并在分析中应用其特征。
|
||
|
||
预处理模块包括:
|
||
|
||
- 预处理
|
||
- 特征提取
|
||
|
||
### Scikit-learn 库示例
|
||
|
||
让我们用一个简单的例子来说明如何在数据科学项目中使用 Scikit-learn 库。
|
||
|
||
我们将使用[鸢尾花花卉数据集][3],该数据集包含在 Scikit-learn 库中。 鸢尾花数据集包含有关三种花种的 150 个细节,三种花种分别为:
|
||
|
||
- Setosa:标记为 0
|
||
- Versicolor:标记为 1
|
||
- Virginica:标记为 2
|
||
|
||
数据集包括每种花种的以下特征(以厘米为单位):
|
||
|
||
- 萼片长度
|
||
- 萼片宽度
|
||
- 花瓣长度
|
||
- 花瓣宽度
|
||
|
||
#### 第 1 步:导入库
|
||
|
||
由于鸢尾花花卉数据集包含在 Scikit-learn 数据科学库中,我们可以将其加载到我们的工作区中,如下所示:
|
||
|
||
```
|
||
from sklearn import datasets
|
||
iris = datasets.load_iris()
|
||
```
|
||
|
||
这些命令从 `sklearn` 导入数据集 `datasets` 模块,然后使用 `datasets` 中的 `load_iris()` 方法将数据包含在工作空间中。
|
||
|
||
#### 第 2 步:获取数据集特征
|
||
|
||
数据集 `datasets` 模块包含几种方法,使您更容易熟悉处理数据。
|
||
|
||
在 Scikit-learn 中,数据集指的是类似字典的对象,其中包含有关数据的所有详细信息。 使用 `.data` 键存储数据,该数据列是一个数组列表。
|
||
|
||
例如,我们可以利用 `iris.data` 输出有关鸢尾花花卉数据集的信息。
|
||
|
||
```
|
||
print(iris.data)
|
||
```
|
||
|
||
这是输出(结果已被截断):
|
||
|
||
```
|
||
[[5.1 3.5 1.4 0.2]
|
||
[4.9 3. 1.4 0.2]
|
||
[4.7 3.2 1.3 0.2]
|
||
[4.6 3.1 1.5 0.2]
|
||
[5. 3.6 1.4 0.2]
|
||
[5.4 3.9 1.7 0.4]
|
||
[4.6 3.4 1.4 0.3]
|
||
[5. 3.4 1.5 0.2]
|
||
[4.4 2.9 1.4 0.2]
|
||
[4.9 3.1 1.5 0.1]
|
||
[5.4 3.7 1.5 0.2]
|
||
[4.8 3.4 1.6 0.2]
|
||
[4.8 3. 1.4 0.1]
|
||
[4.3 3. 1.1 0.1]
|
||
[5.8 4. 1.2 0.2]
|
||
[5.7 4.4 1.5 0.4]
|
||
[5.4 3.9 1.3 0.4]
|
||
[5.1 3.5 1.4 0.3]
|
||
```
|
||
|
||
我们还使用 `iris.target` 向我们提供有关花朵不同标签的信息。
|
||
|
||
```
|
||
print(iris.target)
|
||
```
|
||
|
||
这是输出:
|
||
|
||
```
|
||
[0 0 0 0 0 0 0 0 0 0 0 0 0 0 0 0 0 0 0 0 0 0 0 0 0 0 0 0 0 0 0 0 0 0 0 0 0
|
||
0 0 0 0 0 0 0 0 0 0 0 0 0 1 1 1 1 1 1 1 1 1 1 1 1 1 1 1 1 1 1 1 1 1 1 1 1
|
||
1 1 1 1 1 1 1 1 1 1 1 1 1 1 1 1 1 1 1 1 1 1 1 1 1 1 2 2 2 2 2 2 2 2 2 2 2
|
||
2 2 2 2 2 2 2 2 2 2 2 2 2 2 2 2 2 2 2 2 2 2 2 2 2 2 2 2 2 2 2 2 2 2 2 2 2
|
||
2 2]
|
||
```
|
||
|
||
如果我们使用 `iris.target_names`,我们将输出数据集中找到的标签名称的数组。
|
||
|
||
```
|
||
print(iris.target_names)
|
||
```
|
||
|
||
以下是运行 Python 代码后的结果:
|
||
|
||
```
|
||
['setosa' 'versicolor' 'virginica']
|
||
```
|
||
|
||
#### 第 3 步:可视化数据集
|
||
|
||
我们可以使用[箱形图][4]来生成鸢尾花数据集的视觉描绘。 箱形图说明了数据如何通过四分位数在平面上分布的。
|
||
|
||
以下是如何实现这一目标:
|
||
|
||
```
|
||
import seaborn as sns
|
||
box_data = iris.data # 表示数据数组的变量
|
||
box_target = iris.target # 表示标签数组的变量
|
||
sns.boxplot(data = box_data,width=0.5,fliersize=5)
|
||
sns.set(rc={'figure.figsize':(2,15)})
|
||
```
|
||
|
||
让我们看看结果:
|
||
|
||
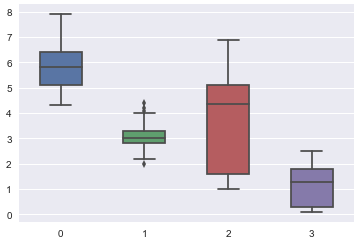
|
||
|
||
在横轴上:
|
||
|
||
* 0 是萼片长度
|
||
* 1 是萼片宽度
|
||
* 2 是花瓣长度
|
||
* 3 是花瓣宽度
|
||
|
||
垂直轴的尺寸以厘米为单位。
|
||
|
||
### 总结
|
||
|
||
以下是这个简单的 Scikit-learn 数据科学教程的完整代码。
|
||
|
||
```
|
||
from sklearn import datasets
|
||
iris = datasets.load_iris()
|
||
print(iris.data)
|
||
print(iris.target)
|
||
print(iris.target_names)
|
||
import seaborn as sns
|
||
box_data = iris.data # 表示数据数组的变量
|
||
box_target = iris.target # 表示标签数组的变量
|
||
sns.boxplot(data = box_data,width=0.5,fliersize=5)
|
||
sns.set(rc={'figure.figsize':(2,15)})
|
||
```
|
||
|
||
Scikit-learn 是一个多功能的 Python 库,可用于高效完成数据科学项目。
|
||
|
||
如果您想了解更多信息,请查看 [LiveEdu][5] 上的教程,例如 Andrey Bulezyuk 关于使用 Scikit-learn 库创建[机器学习应用程序][6]的视频。
|
||
|
||
有什么评价或者疑问吗? 欢迎在下面分享。
|
||
|
||
--------------------------------------------------------------------------------
|
||
|
||
via: https://opensource.com/article/18/9/how-use-scikit-learn-data-science-projects
|
||
|
||
作者:[Dr.Michael J.Garbade][a]
|
||
选题:[lujun9972](https://github.com/lujun9972)
|
||
译者:[Flowsnow](https://github.com/Flowsnow)
|
||
校对:[wxy](https://github.com/wxy)
|
||
|
||
本文由 [LCTT](https://github.com/LCTT/TranslateProject) 原创编译,[Linux中国](https://linux.cn/) 荣誉推出
|
||
|
||
[a]: https://opensource.com/users/drmjg
|
||
[1]: http://scikit-learn.org/stable/index.html
|
||
[2]: https://blog.liveedu.tv/regression-versus-classification-machine-learning-whats-the-difference/
|
||
[3]: https://en.wikipedia.org/wiki/Iris_flower_data_set
|
||
[4]: https://en.wikipedia.org/wiki/Box_plot
|
||
[5]: https://www.liveedu.tv/guides/data-science/
|
||
[6]: https://www.liveedu.tv/andreybu/REaxr-machine-learning-model-python-sklearn-kera/oPGdP-machine-learning-model-python-sklearn-kera/
|