mirror of
https://github.com/LCTT/TranslateProject.git
synced 2024-12-29 21:41:00 +08:00
@qhsgz 首发地址: https://linux.cn/article-9335-1.html 您的 LCTT 专页地址: https://linux.cn/lctt/ghsgz
266 lines
7.3 KiB
Markdown
266 lines
7.3 KiB
Markdown
TensorFlow 的简单例子
|
||
======
|
||
|
||
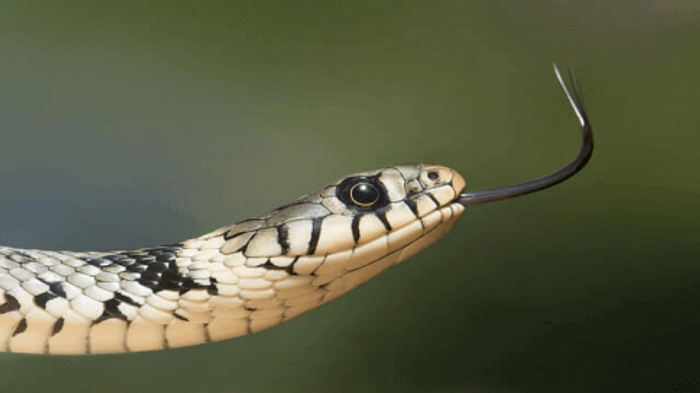
|
||
|
||
在本文中,我们将看一些 TensorFlow 的例子,并从中感受到在定义<ruby>张量<rt>tensor</rt></ruby>和使用张量做数学计算方面有多么容易,我还会举些别的机器学习相关的例子。
|
||
|
||
### TensorFlow 是什么?
|
||
|
||
TensorFlow 是 Google 为了解决复杂的数学计算耗时过久的问题而开发的一个库。
|
||
|
||
事实上,TensorFlow 能干许多事。比如:
|
||
|
||
* 求解复杂数学表达式
|
||
* 机器学习技术。你往其中输入一组数据样本用以训练,接着给出另一组数据样本基于训练的数据而预测结果。这就是人工智能了!
|
||
* 支持 GPU 。你可以使用 GPU(图像处理单元)替代 CPU 以更快的运算。TensorFlow 有两个版本: CPU 版本和 GPU 版本。
|
||
|
||
开始写例子前,需要了解一些基本知识。
|
||
|
||
### 什么是张量?
|
||
|
||
<ruby>张量<rt>tensor</rt></ruby>是 TensorFlow 使用的主要的数据块,它类似于变量,TensorFlow 使用它来处理数据。张量拥有维度和类型的属性。
|
||
|
||
维度指张量的行和列数,读到后面你就知道了,我们可以定义一维张量、二维张量和三维张量。
|
||
|
||
类型指张量元素的数据类型。
|
||
|
||
### 定义一维张量
|
||
|
||
可以这样来定义一个张量:创建一个 NumPy 数组(LCTT 译注:NumPy 系统是 Python 的一种开源数字扩展,包含一个强大的 N 维数组对象 Array,用来存储和处理大型矩阵 )或者一个 [Python 列表][1] ,然后使用 `tf_convert_to_tensor` 函数将其转化成张量。
|
||
|
||
可以像下面这样,使用 NumPy 创建一个数组:
|
||
|
||
```
|
||
import numpy as np arr = np.array([1, 5.5, 3, 15, 20])
|
||
arr = np.array([1, 5.5, 3, 15, 20])
|
||
```
|
||
|
||
运行结果显示了这个数组的维度和形状。
|
||
|
||
```
|
||
import numpy as np
|
||
arr = np.array([1, 5.5, 3, 15, 20])
|
||
print(arr)
|
||
print(arr.ndim)
|
||
print(arr.shape)
|
||
print(arr.dtype)
|
||
```
|
||
|
||
它和 Python 列表很像,但是在这里,元素之间没有逗号。
|
||
|
||
现在使用 `tf_convert_to_tensor` 函数把这个数组转化为张量。
|
||
|
||
```
|
||
import numpy as np
|
||
import tensorflow as tf
|
||
arr = np.array([1, 5.5, 3, 15, 20])
|
||
tensor = tf.convert_to_tensor(arr,tf.float64)
|
||
print(tensor)
|
||
```
|
||
|
||
这次的运行结果显示了张量具体的含义,但是不会展示出张量元素。
|
||
|
||
要想看到张量元素,需要像下面这样,运行一个会话:
|
||
|
||
```
|
||
import numpy as np
|
||
import tensorflow as tf
|
||
arr = np.array([1, 5.5, 3, 15, 20])
|
||
tensor = tf.convert_to_tensor(arr,tf.float64)
|
||
sess = tf.Session()
|
||
print(sess.run(tensor))
|
||
print(sess.run(tensor[1]))
|
||
```
|
||
|
||
### 定义二维张量
|
||
|
||
定义二维张量,其方法和定义一维张量是一样的,但要这样来定义数组:
|
||
|
||
```
|
||
arr = np.array([(1, 5.5, 3, 15, 20),(10, 20, 30, 40, 50), (60, 70, 80, 90, 100)])
|
||
```
|
||
|
||
接着转化为张量:
|
||
|
||
```
|
||
import numpy as np
|
||
import tensorflow as tf
|
||
arr = np.array([(1, 5.5, 3, 15, 20),(10, 20, 30, 40, 50), (60, 70, 80, 90, 100)])
|
||
tensor = tf.convert_to_tensor(arr)
|
||
sess = tf.Session()
|
||
print(sess.run(tensor))
|
||
```
|
||
|
||
现在你应该知道怎么定义张量了,那么,怎么在张量之间跑数学运算呢?
|
||
|
||
### 在张量上进行数学运算
|
||
|
||
假设我们有以下两个数组:
|
||
|
||
```
|
||
arr1 = np.array([(1,2,3),(4,5,6)])
|
||
arr2 = np.array([(7,8,9),(10,11,12)])
|
||
```
|
||
|
||
利用 TenserFlow ,你能做许多数学运算。现在我们需要对这两个数组求和。
|
||
|
||
使用加法函数来求和:
|
||
|
||
```
|
||
import numpy as np
|
||
import tensorflow as tf
|
||
arr1 = np.array([(1,2,3),(4,5,6)])
|
||
arr2 = np.array([(7,8,9),(10,11,12)])
|
||
arr3 = tf.add(arr1,arr2)
|
||
sess = tf.Session()
|
||
tensor = sess.run(arr3)
|
||
print(tensor)
|
||
```
|
||
|
||
也可以把数组相乘:
|
||
|
||
```
|
||
import numpy as np
|
||
import tensorflow as tf
|
||
arr1 = np.array([(1,2,3),(4,5,6)])
|
||
arr2 = np.array([(7,8,9),(10,11,12)])
|
||
arr3 = tf.multiply(arr1,arr2)
|
||
sess = tf.Session()
|
||
tensor = sess.run(arr3)
|
||
print(tensor)
|
||
```
|
||
|
||
现在你知道了吧。
|
||
|
||
## 三维张量
|
||
|
||
我们已经知道了怎么使用一维张量和二维张量,现在,来看一下三维张量吧,不过这次我们不用数字了,而是用一张 RGB 图片。在这张图片上,每一块像素都由 x、y、z 组合表示。
|
||
|
||
这些组合形成了图片的宽度、高度以及颜色深度。
|
||
|
||
首先使用 matplotlib 库导入一张图片。如果你的系统中没有 matplotlib ,可以 [使用 pip][2]来安装它。
|
||
|
||
将图片放在 Python 文件的同一目录下,接着使用 matplotlib 导入图片:
|
||
|
||
```
|
||
import matplotlib.image as img
|
||
myfile = "likegeeks.png"
|
||
myimage = img.imread(myfile)
|
||
print(myimage.ndim)
|
||
print(myimage.shape)
|
||
```
|
||
|
||
从运行结果中,你应该能看到,这张三维图片的宽为 150 、高为 150 、颜色深度为 3 。
|
||
|
||
你还可以查看这张图片:
|
||
|
||
```
|
||
import matplotlib.image as img
|
||
import matplotlib.pyplot as plot
|
||
myfile = "likegeeks.png"
|
||
myimage = img.imread(myfile)
|
||
plot.imshow(myimage)
|
||
plot.show()
|
||
```
|
||
|
||
真酷!
|
||
|
||
那怎么使用 TensorFlow 处理图片呢?超级容易。
|
||
|
||
### 使用 TensorFlow 生成或裁剪图片
|
||
|
||
首先,向一个占位符赋值:
|
||
|
||
```
|
||
myimage = tf.placeholder("int32",[None,None,3])
|
||
```
|
||
|
||
使用裁剪操作来裁剪图像:
|
||
|
||
```
|
||
cropped = tf.slice(myimage,[10,0,0],[16,-1,-1])
|
||
```
|
||
|
||
最后,运行这个会话:
|
||
|
||
```
|
||
result = sess.run(cropped, feed\_dict={slice: myimage})
|
||
```
|
||
|
||
然后,你就能看到使用 matplotlib 处理过的图像了。
|
||
|
||
这是整段代码:
|
||
|
||
```
|
||
import tensorflow as tf
|
||
import matplotlib.image as img
|
||
import matplotlib.pyplot as plot
|
||
myfile = "likegeeks.png"
|
||
myimage = img.imread(myfile)
|
||
slice = tf.placeholder("int32",[None,None,3])
|
||
cropped = tf.slice(myimage,[10,0,0],[16,-1,-1])
|
||
sess = tf.Session()
|
||
result = sess.run(cropped, feed_dict={slice: myimage})
|
||
plot.imshow(result)
|
||
plot.show()
|
||
```
|
||
|
||
是不是很神奇?
|
||
|
||
### 使用 TensorFlow 改变图像
|
||
|
||
在本例中,我们会使用 TensorFlow 做一下简单的转换。
|
||
|
||
首先,指定待处理的图像,并初始化 TensorFlow 变量值:
|
||
|
||
```
|
||
myfile = "likegeeks.png"
|
||
myimage = img.imread(myfile)
|
||
image = tf.Variable(myimage,name='image')
|
||
vars = tf.global_variables_initializer()
|
||
```
|
||
|
||
然后调用 transpose 函数转换,这个函数用来翻转输入网格的 0 轴和 1 轴。
|
||
|
||
```
|
||
sess = tf.Session()
|
||
flipped = tf.transpose(image, perm=[1,0,2])
|
||
sess.run(vars)
|
||
result=sess.run(flipped)
|
||
```
|
||
|
||
接着你就能看到使用 matplotlib 处理过的图像了。
|
||
|
||
```
|
||
import tensorflow as tf
|
||
import matplotlib.image as img
|
||
import matplotlib.pyplot as plot
|
||
myfile = "likegeeks.png"
|
||
myimage = img.imread(myfile)
|
||
image = tf.Variable(myimage,name='image')
|
||
vars = tf.global_variables_initializer()
|
||
sess = tf.Session()
|
||
flipped = tf.transpose(image, perm=[1,0,2])
|
||
sess.run(vars)
|
||
result=sess.run(flipped)
|
||
plot.imshow(result)
|
||
plot.show()
|
||
```
|
||
|
||
以上例子都向你表明了使用 TensorFlow 有多么容易。
|
||
|
||
--------------------------------------------------------------------------------
|
||
|
||
via: https://www.codementor.io/likegeeks/define-and-use-tensors-using-simple-tensorflow-examples-ggdgwoy4u
|
||
|
||
作者:[LikeGeeks][a]
|
||
译者:[ghsgz](https://github.com/ghsgz)
|
||
校对:[wxy](https://github.com/wxy)
|
||
|
||
本文由 [LCTT](https://github.com/LCTT/TranslateProject) 原创编译,[Linux中国](https://linux.cn/) 荣誉推出
|
||
|
||
[a]:https://www.codementor.io/likegeeks
|
||
[1]:https://likegeeks.com/python-list-functions/
|
||
[2]:https://likegeeks.com/import-create-install-reload-alias-python-modules/#Install-Python-Modules-Using-pip
|