mirror of
https://github.com/LCTT/TranslateProject.git
synced 2025-01-22 23:00:57 +08:00
341 lines
7.5 KiB
Markdown
341 lines
7.5 KiB
Markdown
Simple TensorFlow Examples
|
||
======
|
||
|
||
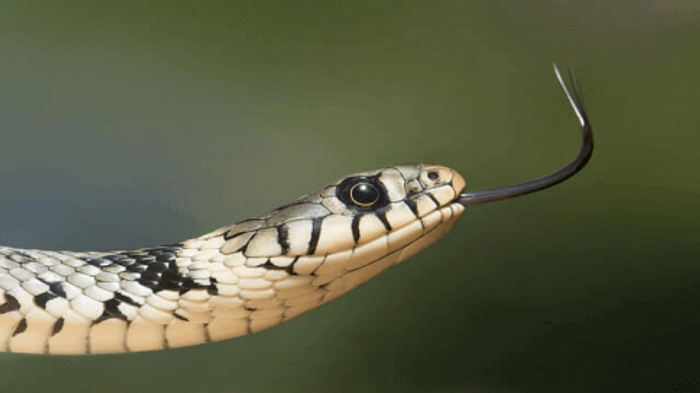
|
||
|
||
In this post, we are going to see some TensorFlow examples and see how it’s easy to define tensors, perform math operations using tensors, and other machine learning examples.
|
||
|
||
## What is TensorFlow?
|
||
|
||
TensorFlow is a library which was developed by Google for solving complicated mathematical problems which takes much time.
|
||
|
||
Actually, TensorFlow can do many things like:
|
||
|
||
* Solving complex mathematical expressions.
|
||
* Machine learning techniques, where you give it a sample of data for training, then you give another sample of data to predict the result based on the training data. This is the artificial intelligence!!
|
||
* GPU support. You can use GPU (Graphical Processing Unit) instead of CPU for faster processing. There are two versions of TensorFlow, CPU version and GPU version.
|
||
|
||
|
||
|
||
Before we start working with TensorFlow examples, we need to know some basics.
|
||
|
||
## What is a Tensor?
|
||
|
||
The tensor is the main blocks of data that TensorFlow uses, it’s like the variables that TensorFlow uses to work with data. Each tensor has a dimension and a type.
|
||
|
||
The dimension is the rows and columns of the tensor, you can define one-dimensional tensor, two-dimensional tensor, and three-dimensional tensor as we will see later.
|
||
|
||
The type is the data type for the elements of the tensor.
|
||
|
||
## Define one-dimensional Tensor
|
||
|
||
To define a tensor, we will create a NumPy array or a [Python list][1] and convert it to a tensor using the tf_convert_to_tensor function.
|
||
|
||
We will use NumPy to create an array like this:
|
||
```
|
||
import numpy as np arr = np.array([1, 5.5, 3, 15, 20])
|
||
|
||
arr = np.array([1, 5.5, 3, 15, 20])
|
||
|
||
```
|
||
|
||
You can see from the results the dimension and shape of the array.
|
||
```
|
||
import numpy as np
|
||
|
||
arr = np.array([1, 5.5, 3, 15, 20])
|
||
|
||
print(arr)
|
||
|
||
print (arr.ndim)
|
||
|
||
print (arr.shape)
|
||
|
||
print (arr.dtype)
|
||
|
||
```
|
||
|
||
It looks like the Python list but here there is no comma between the items.
|
||
|
||
Now we will convert this array to a tensor using tf_convert_to_tensor function.
|
||
```
|
||
import numpy as np
|
||
|
||
import tensorflow as tf
|
||
|
||
arr = np.array([1, 5.5, 3, 15, 20])
|
||
|
||
tensor = tf.convert_to_tensor(arr,tf.float64)
|
||
|
||
print(tensor)
|
||
|
||
```
|
||
|
||
From the results, you can see the tensor definition, but you can’t see the tensor elements.
|
||
|
||
Well, to see the tensor elements, you can run a session like this:
|
||
```
|
||
import numpy as np
|
||
|
||
import tensorflow as tf
|
||
|
||
arr = np.array([1, 5.5, 3, 15, 20])
|
||
|
||
tensor = tf.convert_to_tensor(arr,tf.float64)
|
||
|
||
sess = tf.Session()
|
||
|
||
print(sess.run(tensor))
|
||
|
||
print(sess.run(tensor[1]))
|
||
|
||
```
|
||
|
||
## Define Two-dimensional Tensor
|
||
|
||
The same way as the one-dimensional array, but this time we will define the array like this:
|
||
|
||
```
|
||
arr = np.array([(1, 5.5, 3, 15, 20),(10, 20, 30, 40, 50), (60, 70, 80, 90, 100)])
|
||
```
|
||
|
||
And you can convert it to a tensor like this:
|
||
```
|
||
import numpy as np
|
||
|
||
import tensorflow as tf
|
||
|
||
arr = np.array([(1, 5.5, 3, 15, 20),(10, 20, 30, 40, 50), (60, 70, 80, 90, 100)])
|
||
|
||
tensor = tf.convert_to_tensor(arr)
|
||
|
||
sess = tf.Session()
|
||
|
||
print(sess.run(tensor))
|
||
|
||
```
|
||
|
||
Now you know how to define tensors, what about performing some math operations between them?
|
||
|
||
## Performing Math on Tensors
|
||
|
||
Suppose that we have 2 arrays like this:
|
||
```
|
||
arr1 = np.array([(1,2,3),(4,5,6)])
|
||
|
||
arr2 = np.array([(7,8,9),(10,11,12)])
|
||
|
||
```
|
||
|
||
We need to get the sum of them. You can perform many math operations using TensorFlow.
|
||
|
||
You can use the add function like this:
|
||
```
|
||
import numpy as np
|
||
|
||
import tensorflow as tf
|
||
|
||
arr1 = np.array([(1,2,3),(4,5,6)])
|
||
|
||
arr2 = np.array([(7,8,9),(10,11,12)])
|
||
|
||
arr3 = tf.add(arr1,arr2)
|
||
|
||
sess = tf.Session()
|
||
|
||
tensor = sess.run(arr3)
|
||
|
||
print(tensor)
|
||
|
||
```
|
||
|
||
You can multiply arrays like this:
|
||
```
|
||
import numpy as np
|
||
|
||
import tensorflow as tf
|
||
|
||
arr1 = np.array([(1,2,3),(4,5,6)])
|
||
|
||
arr2 = np.array([(7,8,9),(10,11,12)])
|
||
|
||
arr3 = tf.multiply(arr1,arr2)
|
||
|
||
sess = tf.Session()
|
||
|
||
tensor = sess.run(arr3)
|
||
|
||
print(tensor)
|
||
|
||
```
|
||
|
||
Now you got the idea.
|
||
|
||
## Three-dimensional Tensor
|
||
|
||
We saw how to work with one and two-dimensional tensors, now we will see the three-dimensional tensors, but this time we won’t use numbers, we will use an RGB image where each piece of the image is specified by x, y, and z coordinates.
|
||
|
||
These coordinates are the width, height, and color depth.
|
||
|
||
First, let’s import the image using matplotlib. You can install matplotlib [using pip][2] if it’s not installed on your system.
|
||
|
||
Now, put your file in the same directory with your Python file and import the image using matplotlib like this:
|
||
```
|
||
import matplotlib.image as img
|
||
|
||
myfile = "likegeeks.png"
|
||
|
||
myimage = img.imread(myfile)
|
||
|
||
print(myimage.ndim)
|
||
|
||
print(myimage.shape)
|
||
|
||
```
|
||
|
||
As you can see, it’s a three-dimensional image where the width is 150 and the height is 150 and the color depth is 3.
|
||
|
||
You can view the image like this:
|
||
```
|
||
import matplotlib.image as img
|
||
|
||
import matplotlib.pyplot as plot
|
||
|
||
myfile = "likegeeks.png"
|
||
|
||
myimage = img.imread(myfile)
|
||
|
||
plot.imshow(myimage)
|
||
|
||
plot.show()
|
||
|
||
```
|
||
|
||
Cool!!
|
||
|
||
What about manipulating the image using TensorFlow? Super easy.
|
||
|
||
## Crop Or Slice Image Using TensorFlow
|
||
|
||
First, we put the values on a placeholder like this:
|
||
```
|
||
myimage = tf.placeholder("int32",[None,None,3])
|
||
|
||
```
|
||
|
||
To slice the image, we will use the slice operator like this:
|
||
```
|
||
cropped = tf.slice(myimage,[10,0,0],[16,-1,-1])
|
||
|
||
```
|
||
|
||
Finally, run the session:
|
||
```
|
||
result = sess.run(cropped, feed\_dict={slice: myimage})
|
||
|
||
```
|
||
|
||
Then you can see the result image using matplotlib.
|
||
|
||
So the whole code will be like this:
|
||
```
|
||
import tensorflow as tf
|
||
|
||
import matplotlib.image as img
|
||
|
||
import matplotlib.pyplot as plot
|
||
|
||
myfile = "likegeeks.png"
|
||
|
||
myimage = img.imread(myfile)
|
||
|
||
slice = tf.placeholder("int32",[None,None,3])
|
||
|
||
cropped = tf.slice(myimage,[10,0,0],[16,-1,-1])
|
||
|
||
sess = tf.Session()
|
||
|
||
result = sess.run(cropped, feed_dict={slice: myimage})
|
||
|
||
plot.imshow(result)
|
||
|
||
plot.show()
|
||
|
||
```
|
||
|
||
Awesome!!
|
||
|
||
## Transpose Images using TensorFlow
|
||
|
||
In this TensorFlow example, we will do a simple transformation using TensorFlow.
|
||
|
||
First, specify the input image and initialize TensorFlow variables:
|
||
```
|
||
myfile = "likegeeks.png"
|
||
|
||
myimage = img.imread(myfile)
|
||
|
||
image = tf.Variable(myimage,name='image')
|
||
|
||
vars = tf.global_variables_initializer()
|
||
|
||
```
|
||
|
||
Then we will use the transpose function which flips the 0 and 1 axes of the input grid:
|
||
```
|
||
sess = tf.Session()
|
||
|
||
flipped = tf.transpose(image, perm=[1,0,2])
|
||
|
||
sess.run(vars)
|
||
|
||
result=sess.run(flipped)
|
||
|
||
```
|
||
|
||
Then you can show the resulting image using matplotlib.
|
||
```
|
||
import tensorflow as tf
|
||
|
||
import matplotlib.image as img
|
||
|
||
import matplotlib.pyplot as plot
|
||
|
||
myfile = "likegeeks.png"
|
||
|
||
myimage = img.imread(myfile)
|
||
|
||
image = tf.Variable(myimage,name='image')
|
||
|
||
vars = tf.global_variables_initializer()
|
||
|
||
sess = tf.Session()
|
||
|
||
flipped = tf.transpose(image, perm=[1,0,2])
|
||
|
||
sess.run(vars)
|
||
|
||
result=sess.run(flipped)
|
||
|
||
plot.imshow(result)
|
||
|
||
plot.show()
|
||
|
||
```
|
||
|
||
All these TensorFlow examples show you how easy it’s to work with TensorFlow.
|
||
|
||
--------------------------------------------------------------------------------
|
||
|
||
via: https://www.codementor.io/likegeeks/define-and-use-tensors-using-simple-tensorflow-examples-ggdgwoy4u
|
||
|
||
作者:[LikeGeeks][a]
|
||
译者:[译者ID](https://github.com/译者ID)
|
||
校对:[校对者ID](https://github.com/校对者ID)
|
||
|
||
本文由 [LCTT](https://github.com/LCTT/TranslateProject) 原创编译,[Linux中国](https://linux.cn/) 荣誉推出
|
||
|
||
[a]:https://www.codementor.io/likegeeks
|
||
[1]:https://likegeeks.com/python-list-functions/
|
||
[2]:https://likegeeks.com/import-create-install-reload-alias-python-modules/#Install-Python-Modules-Using-pip
|