mirror of
https://github.com/LCTT/TranslateProject.git
synced 2024-12-23 21:20:42 +08:00
275 lines
11 KiB
Markdown
275 lines
11 KiB
Markdown
[#]: collector: (lujun9972)
|
||
[#]: translator: (chunibyo-wly)
|
||
[#]: reviewer: (wxy)
|
||
[#]: publisher: (wxy)
|
||
[#]: url: (https://linux.cn/article-13744-1.html)
|
||
[#]: subject: (Deploy a deep learning model on Kubernetes)
|
||
[#]: via: (https://opensource.com/article/20/9/deep-learning-model-kubernetes)
|
||
[#]: author: (Chaimaa Zyani https://opensource.com/users/chaimaa)
|
||
|
||
在 Kubernetes 上部署一个深度学习模型
|
||
======
|
||
|
||
> 了解如何使用 Kubermatic Kubernetes 平台来部署、扩展与管理图像识别预测的深度学习模型。
|
||
|
||
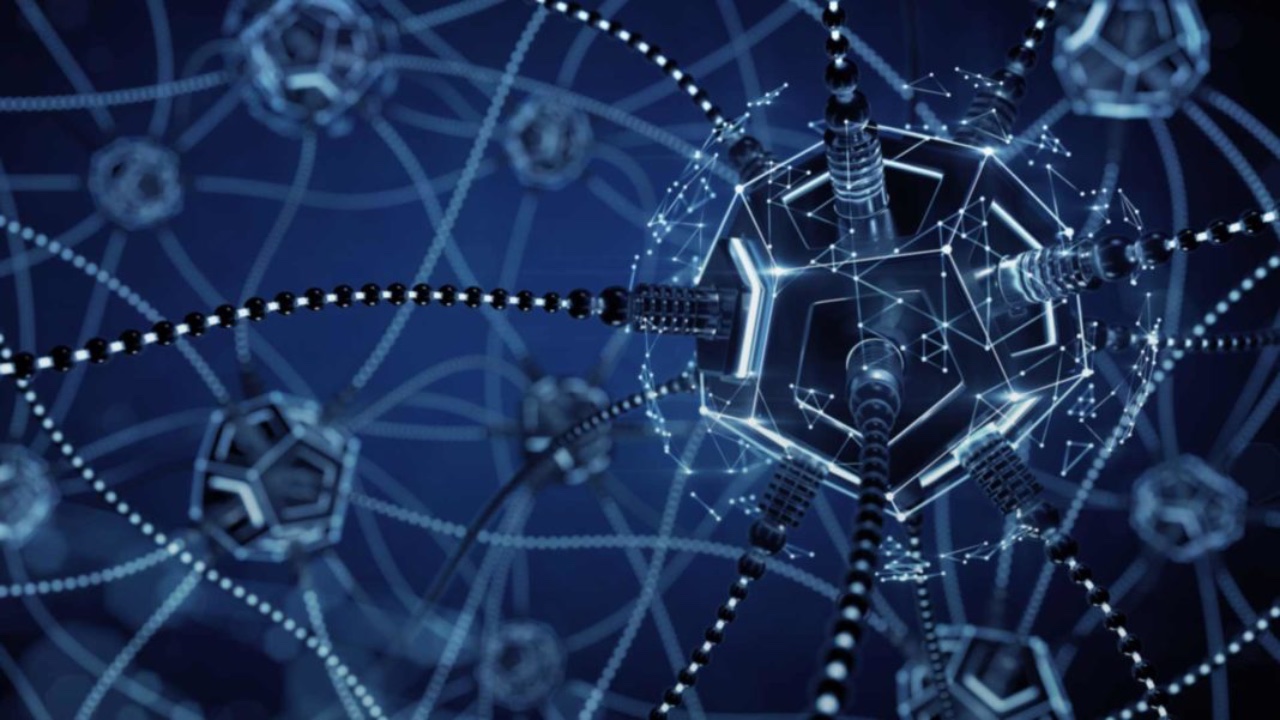
|
||
|
||
随着企业增加了对人工智能(AI)、机器学习(ML)与深度学习(DL)的使用,出现了一个关键问题:如何将机器学习的开发进行规模化与产业化?这些讨论经常聚焦于机器学习模型本身;然而,模型仅仅只是完整解决方案的其中一环。为了达到生产环境的应用和规模,模型的开发过程必须还包括一个可以说明开发前后关键活动以及可公用部署的可重复过程。
|
||
|
||
本文演示了如何使用 [Kubermatic Kubernetes 平台][2] 对图像识别预测的深度学习模型进行部署、扩展与管理。
|
||
|
||
Kubermatic Kubernetes 平台是一个生产级的开源 Kubernetes 集群管理工具,提供灵活性和自动化,与机器学习/深度学习工作流程整合,具有完整的集群生命周期管理。
|
||
|
||
### 开始
|
||
|
||
这个例子部署了一个用于图像识别的深度学习模型。它使用了 [CIFAR-10][3] 数据集,包含了 60,000 张分属 10 个类别的 32x32 彩色图,同时使用了 [Apache MXNet][5] 的 [Gluon][4] 与 NVIDIA GPU 进行加速计算。如果你希望使用 CIFAR-10 数据集的预训练模型,可以查阅其 [入门指南][6]。
|
||
|
||
使用训练集中的样本对模型训练 200 次,只要训练误差保持缓慢减少,就可以保证模型不会过拟合。下方图展示了训练的过程:
|
||
|
||
![深度学习模型训练 loss 图][7]
|
||
|
||
训练结束后,必须保存模型训练所得到的参数,以便稍后可以加载它们:
|
||
|
||
```
|
||
file_name = "net.params"
|
||
net.save_parameters(file_name)
|
||
```
|
||
|
||
一旦你的模型训练好了,就可以用 Flask 服务器来封装它。下方的程序演示了如何接收请求中的一张图片作为参数,并在响应中返回模型的预测结果:
|
||
|
||
```
|
||
from gluoncv.model_zoo import get_model
|
||
import matplotlib.pyplot as plt
|
||
from mxnet import gluon, nd, image
|
||
from mxnet.gluon.data.vision import transforms
|
||
from gluoncv import utils
|
||
from PIL import Image
|
||
import io
|
||
import flask
|
||
app = flask.Flask(__name__)
|
||
|
||
@app.route("/predict",methods=["POST"])
|
||
def predict():
|
||
if flask.request.method == "POST":
|
||
if flask.request.files.get("img"):
|
||
img = Image.open(io.BytesIO(flask.request.files["img"].read()))
|
||
transform_fn = transforms.Compose([
|
||
transforms.Resize(32),
|
||
transforms.CenterCrop(32),
|
||
transforms.ToTensor(),
|
||
transforms.Normalize([0.4914, 0.4822, 0.4465], [0.2023, 0.1994, 0.2010])])
|
||
img = transform_fn(nd.array(img))
|
||
net = get_model('cifar_resnet20_v1', classes=10)
|
||
net.load_parameters('net.params')
|
||
pred = net(img.expand_dims(axis=0))
|
||
class_names = ['airplane', 'automobile', 'bird', 'cat', 'deer',
|
||
'dog', 'frog', 'horse', 'ship', 'truck']
|
||
ind = nd.argmax(pred, axis=1).astype('int')
|
||
prediction = 'The input picture is classified as [%s], with probability %.3f.'%
|
||
(class_names[ind.asscalar()], nd.softmax(pred)[0][ind].asscalar())
|
||
return prediction
|
||
|
||
if __name__ == '__main__':
|
||
app.run(host='0.0.0.0')
|
||
```
|
||
|
||
### 容器化模型
|
||
|
||
在将模型部署到 Kubernetes 前,你需要先安装 Docker 并使用你的模型创建一个镜像。
|
||
|
||
1. 下载、安装并启动 Docker:
|
||
|
||
```
|
||
sudo yum install -y yum-utils device-mapper-persistent-data lvm2
|
||
sudo yum-config-manager --add-repo <https://download.docker.com/linux/centos/docker-ce.repo>
|
||
sudo yum install docker-ce
|
||
sudo systemctl start docker
|
||
```
|
||
|
||
2. 创建一个你用来管理代码与依赖的文件夹:
|
||
|
||
```
|
||
mkdir kubermatic-dl
|
||
cd kubermatic-dl
|
||
```
|
||
|
||
3. 创建 `requirements.txt` 文件管理代码运行时需要的所有依赖:
|
||
|
||
```
|
||
flask
|
||
gluoncv
|
||
matplotlib
|
||
mxnet
|
||
requests
|
||
Pillow
|
||
```
|
||
|
||
4. 创建 `Dockerfile`,Docker 将根据这个文件创建镜像:
|
||
|
||
```
|
||
FROM python:3.6
|
||
WORKDIR /app
|
||
COPY requirements.txt /app
|
||
RUN pip install -r ./requirements.txt
|
||
COPY app.py /app
|
||
CMD ["python", "app.py"]
|
||
```
|
||
|
||
这个 `Dockerfile` 主要可以分为三个部分。首先,Docker 会下载 Python 的基础镜像。然后,Docker 会使用 Python 的包管理工具 `pip` 安装 `requirements.txt` 记录的包。最后,Docker 会通过执行 `python app.py` 来运行你的脚本。
|
||
|
||
5. 构建 Docker 容器:
|
||
|
||
```
|
||
sudo docker build -t kubermatic-dl:latest .
|
||
```
|
||
这条命令使用 `kubermatic-dl` 镜像为你当前工作目录的代码创建了一个容器。
|
||
|
||
6. 使用
|
||
|
||
```
|
||
sudo docker run -d -p 5000:5000 kubermatic-dl
|
||
```
|
||
|
||
命令检查你的容器可以在你的主机上正常运行。
|
||
|
||
7. 使用
|
||
|
||
```
|
||
sudo docker ps -a
|
||
```
|
||
命令查看你本地容器的运行状态:
|
||
|
||
![查看容器的运行状态][9]
|
||
|
||
### 将你的模型上传到 Docker Hub
|
||
|
||
在向 Kubernetes 上部署模型前,你的镜像首先需要是公开可用的。你可以通过将你的模型上传到 [Docker Hub][10] 来将它公开。(如果你没有 Docker Hub 的账号,你需要先创建一个)
|
||
|
||
1. 在终端中登录 Docker Hub 账号:
|
||
|
||
```
|
||
sudo docker login
|
||
```
|
||
|
||
2. 给你的镜像打上标签,这样你的模型上传到 Docker Hub 后也能拥有版本信息:
|
||
|
||
```
|
||
sudo docker tag <your-image-id> <your-docker-hub-name>/<your-app-name>
|
||
|
||
sudo docker push <your-docker-hub-name>/<your-app-name>
|
||
```
|
||
|
||
![给镜像打上 tag][11]
|
||
|
||
3. 使用
|
||
|
||
```
|
||
sudo docker images
|
||
```
|
||
|
||
命令检查你的镜像的 ID。
|
||
|
||
### 部署你的模型到 Kubernetes 集群
|
||
|
||
1. 首先在 Kubermatic Kubernetes 平台创建一个项目, 然后根据 [快速开始][12] 创建一个 Kubernetes 集群。
|
||
|
||
![创建一个 Kubernetes 集群][13]
|
||
|
||
2. 下载用于访问你的集群的 `kubeconfig`,将它放置在下载目录中,并记得设置合适的环境变量,使得你的环境能找到它:
|
||
|
||
![Kubernetes 集群示例][14]
|
||
|
||
3. 使用 `kubectl` 命令检查集群信息,例如,需要检查 `kube-system` 是否在你的集群正常启动了就可以使用命令 `kubectl cluster-info`:
|
||
|
||
![查看集群信息][15]
|
||
|
||
4. 为了在集群中运行容器,你需要创建一个部署用的配置文件(`deployment.yaml`),再运行 `apply` 命令将其应用于集群中:
|
||
|
||
```
|
||
apiVersion: apps/v1
|
||
kind: Deployment
|
||
metadata:
|
||
name: kubermatic-dl-deployment
|
||
spec:
|
||
selector:
|
||
matchLabels:
|
||
app: kubermatic-dl
|
||
replicas: 3
|
||
template:
|
||
metadata:
|
||
labels:
|
||
app: kubermatic-dl
|
||
spec:
|
||
containers:
|
||
- name: kubermatic-dl
|
||
image: kubermatic00/kubermatic-dl:latest
|
||
imagePullPolicy: Always
|
||
ports:
|
||
- containerPort: 8080
|
||
|
||
```
|
||
|
||
```
|
||
kubectl apply -f deployment.yaml`
|
||
```
|
||
|
||
5. 为了将你的部署开放到公网环境,你需要一个能够给你的容器创建外部可达 IP 地址的服务:
|
||
|
||
```
|
||
kubectl expose deployment kubermatic-dl-deployment --type=LoadBalancer --port 80 --target-port 5000`
|
||
```
|
||
|
||
6. 就快大功告成了!首先检查你布署的服务的状态,然后通过 IP 请求的你图像识别 API:
|
||
|
||
```
|
||
kubectl get service
|
||
```
|
||
|
||
![获取请求图像识别 API 的 IP 地址][16]
|
||
|
||
7. 最后根据你的外部 IP 使用以下两张图片对你的图像识别服务进行测试:
|
||
|
||
![马][17]
|
||
|
||
![狗][18]
|
||
|
||
![测试 API][19]
|
||
|
||
### 总结
|
||
|
||
在这篇教程中,你可以创建一个深度学习模型,并且使用 Flask 提供 [REST API][20] 服务。它介绍了如何将应用放在 Docker 容器中,如何将这个镜像上传到 Docker Hub 中,以及如何使用 Kubernetes 部署你的服务。只需几个简单的命令,你就可以使用 Kubermatic Kubernetes 平台部署该应用程序,并且开放服务给别人使用。
|
||
|
||
--------------------------------------------------------------------------------
|
||
|
||
via: https://opensource.com/article/20/9/deep-learning-model-kubernetes
|
||
|
||
作者:[Chaimaa Zyani][a]
|
||
选题:[lujun9972][b]
|
||
译者:[chunibyo-wly](https://github.com/chunibyo-wly)
|
||
校对:[wxy](https://github.com/wxy)
|
||
|
||
本文由 [LCTT](https://github.com/LCTT/TranslateProject) 原创编译,[Linux中国](https://linux.cn/) 荣誉推出
|
||
|
||
[a]: https://opensource.com/users/chaimaa
|
||
[b]: https://github.com/lujun9972
|
||
[1]: https://opensource.com/sites/default/files/styles/image-full-size/public/lead-images/brain_computer_solve_fix_tool.png?itok=okq8joti (Brain on a computer screen)
|
||
[2]: https://www.loodse.com/products/kubermatic/
|
||
[3]: https://www.cs.toronto.edu/~kriz/cifar.html
|
||
[4]: https://gluon.mxnet.io/
|
||
[5]: https://mxnet.apache.org/
|
||
[6]: https://gluon-cv.mxnet.io/build/examples_classification/demo_cifar10.html
|
||
[7]: https://opensource.com/sites/default/files/uploads/trainingplot.png (Deep learning model training plot)
|
||
[8]: https://creativecommons.org/licenses/by-sa/4.0/
|
||
[9]: https://opensource.com/sites/default/files/uploads/containerstatus.png (Checking the container's status)
|
||
[10]: https://hub.docker.com/
|
||
[11]: https://opensource.com/sites/default/files/uploads/tagimage.png (Tagging the image)
|
||
[12]: https://docs.kubermatic.com/kubermatic/v2.13/installation/install_kubermatic/_installer/
|
||
[13]: https://opensource.com/sites/default/files/uploads/kubernetesclusterempty.png (Create a Kubernetes cluster)
|
||
[14]: https://opensource.com/sites/default/files/uploads/kubernetesexamplecluster.png (Kubernetes cluster example)
|
||
[15]: https://opensource.com/sites/default/files/uploads/clusterinfo.png (Checking the cluster info)
|
||
[16]: https://opensource.com/sites/default/files/uploads/getservice.png (Get the IP address to call your image recognition API)
|
||
[17]: https://opensource.com/sites/default/files/uploads/horse.jpg (Horse)
|
||
[18]: https://opensource.com/sites/default/files/uploads/dog.jpg (Dog)
|
||
[19]: https://opensource.com/sites/default/files/uploads/testapi.png (Testing the API)
|
||
[20]: https://www.redhat.com/en/topics/api/what-is-a-rest-api
|