mirror of
https://github.com/LCTT/TranslateProject.git
synced 2025-02-03 23:40:14 +08:00
commit
fd9c0aff4e
@ -1,59 +1,59 @@
|
|||||||
用户报告:Steam Machines 与 SteamOS 发布一周年记
|
回顾 Steam Machines 与 SteamOS
|
||||||
====
|
====
|
||||||
|
|
||||||
去年今日,在非常符合 Valve 风格的跳票之后大众迎来了 [Steam Machine 的发布][2]。即使是在 Linux 桌面环境对于游戏的支持大步进步的今天,Steam Machines 作为一个平台依然没有飞跃,而 SteamOS 似乎也止步不前。这些由 Valve 发起的项目究竟怎么了?这些项目为何被发起,又是如何失败的?一些改进又是否曾有机会挽救这些项目的成败?
|
去年今日(LCTT 译注:本文发表于 2016 年),在非常符合 Valve 风格的跳票之后,大众迎来了 [Steam Machines 的发布][2]。即使是在 Linux 桌面环境对于游戏的支持大步进步的今天,Steam Machines 作为一个平台依然没有飞跃,而 SteamOS 似乎也止步不前。这些由 Valve 发起的项目究竟怎么了?这些项目为何被发起,又是如何失败的?一些改进又是否曾有机会挽救这些项目的成败?
|
||||||
|
|
||||||
**行业环境**
|
### 行业环境
|
||||||
|
|
||||||
在 2012 年 Windows 8 发布的时候,微软像 iOS 与 Android 那样,为 Windows 集成了一个应用商店。在微软试图推广对触摸体验友好的界面时,为了更好的提供 “Metro” UI 语言指导下的沉浸式触摸体验,他们同时推出了一系列叫做 “WinRT” 的 API。然而为了能够使用这套 API,应用开发者们必须把应用程序通过 Windows 应用商城发布,并且正如其他应用商城那样,微软从中抽成30%。对于 Valve 的 CEO,Gabe Newell (G胖) 而言,这种限制发布平台和抽成行为是让人无法接受的,而且他前瞻地看到了微软利用行业龙头地位来推广 Windows 商店和 Metro 应用对于 Valve 潜在的危险,正如当年微软用 IE 浏览器击垮 Netscape 浏览器一样。
|
在 2012 年 Windows 8 发布的时候,微软像 iOS 与 Android 那样,为 Windows 集成了一个应用商店。在微软试图推广对触摸体验友好的界面时,为了更好的提供 “Metro” UI 语言指导下的沉浸式触摸体验,他们同时推出了一系列叫做 “WinRT” 的 API。然而为了能够使用这套 API,应用开发者们必须把应用程序通过 Windows 应用商城发布,并且正如其它应用商城那样,微软从中抽成 30%。对于 Valve 的 CEO,Gabe Newell (G 胖) 而言,这种限制发布平台和抽成行为是让人无法接受的,而且他前瞻地看到了微软利用行业龙头地位来推广 Windows 商店和 Metro 应用对于 Valve 潜在的危险,正如当年微软用 IE 浏览器击垮 Netscape 浏览器一样。
|
||||||
|
|
||||||
对于 Valve 来说,运行 Windows 的 PC 的优势在于任何人都可以不受操作系统和硬件方的限制运行各种软件。当像 Windows 这样的专有平台对像 Steam 这样的第三方软件限制越来越严格时,应用开发者们自然会想要寻找一个对任何人都更开放和自由的替代品,他们很自然的会想到 Linux 。Linux 本质上只是一套内核,但你可以轻易地使用 GNU 组件,Gnnome 等软件在这套内核上开发出一个操作系统,比如 Ubuntu 就是这么来的。推行 Ubuntu 或者其他 Linux 发行版自然可以为 Valve 提供一个无拘无束的平台,以防止微软或者苹果变成 Valve 作为第三方平台之路上的的敌人,但 Linux 甚至给了 Valve 一个创造新的操作系统平台的机会。
|
对于 Valve 来说,运行 Windows 的 PC 的优势在于任何人都可以不受操作系统和硬件方的限制运行各种软件。当像 Windows 这样的专有平台对像 Steam 这样的第三方软件限制越来越严格时,应用开发者们自然会想要寻找一个对任何人都更开放和自由的替代品,他们很自然的会想到 Linux 。Linux 本质上只是一套内核,但你可以轻易地使用 GNU 组件、Gnome 等软件在这套内核上开发出一个操作系统,比如 Ubuntu 就是这么来的。推行 Ubuntu 或者其他 Linux 发行版自然可以为 Valve 提供一个无拘无束的平台,以防止微软或者苹果变成 Valve 作为第三方平台之路上的的敌人,但 Linux 甚至给了 Valve 一个创造新的操作系统平台的机会。
|
||||||
|
|
||||||
**概念化**
|
### 概念化
|
||||||
|
|
||||||
如果我们把 Steam Machines 叫做主机的话,Valve 当时似乎认定了主机平台是一个机会。为了迎合用户对于电视主机平台用户界面的审美期待,同时也为了让玩家更好地从稍远的距离上在电视上玩游戏,Valve 为 Steam 推出了 Big Picture 模式。Steam Machines 的核心要点是开放性;比方说所有的软件都被设计成可以脱离 Windows 工作,又比如说 Steam Machines 手柄的 CAD 图纸也被公布出来以便支持玩家二次创作。
|
如果我们把 Steam Machines 叫做主机的话,Valve 当时似乎认定了主机平台是一个机会。为了迎合用户对于电视主机平台用户界面的审美期待,同时也为了让玩家更好地从稍远的距离上在电视上玩游戏,Valve 为 Steam 推出了 Big Picture 模式。Steam Machines 的核心要点是开放性;比方说所有的软件都被设计成可以脱离 Windows 工作,又比如说 Steam Machines 手柄的 CAD 图纸也被公布出来以便支持玩家二次创作。
|
||||||
|
|
||||||
原初计划中,Valve 打算设计一款官方的 Steam Machine 作为旗舰机型。但最终,这些机型只在 2013 年的时候作为原型机给与了部分测试者用于测试。Valve 后来也允许像戴尔这样的 OEM 厂商们制造 Steam Machines,并且也赋予了他们定制价格和配置规格的权利。有一家叫做 “Xi3” 的公司展示了他们设计的 Steam Machine 小型机型,那款机型小到可以放在手掌上,这一新闻创造了围绕 Steam Machines 的更多热烈讨论。最终,Valve 决定不自己设计知道 Steam Machines,而全权交给 OEM 合作厂商们。
|
原初计划中,Valve 打算设计一款官方的 Steam Machine 作为旗舰机型。但最终,这些机型只在 2013 年的时候作为原型机给与了部分测试者用于测试。Valve 后来也允许像戴尔这样的 OEM 厂商们制造 Steam Machines,并且也赋予了他们制定价格和配置规格的权利。有一家叫做 “Xi3” 的公司展示了他们设计的 Steam Machine 小型机型,那款机型小到可以放在手掌上,这一新闻创造了围绕 Steam Machines 的更多热烈讨论。最终,Valve 决定不自己设计制造 Steam Machines,而全权交给 OEM 合作厂商们。
|
||||||
|
|
||||||
这一过程中还有很多天马行空的创意被列入考量,比如在手柄上加入生物识别技术,眼球追踪以及动作控制等。在这些最初的想法里,陀螺仪被加入了 Steam Controller 手柄,HTC Vive 的手柄也有各种动作追踪仪器;这些想法可能最初都来源于 Steam 手柄的设计过程中。手柄最初还有些更激进的设计,比如在中心放置一块可定制化并且会随着游戏内容变化的触摸屏。但最后的最后,发布会上的手柄偏向保守了许多,但也有诸如双触摸板和内置软件等黑科技。Valve 也考虑过制作面向笔记本类型硬件的 Steam Machines 和 SteamOS。这个企划最终没有任何成果,但也许 “Smach Z” 手持游戏机会是发展的方向之一。
|
这一过程中还有很多天马行空的创意被列入考量,比如在手柄上加入生物识别技术、眼球追踪以及动作控制等。在这些最初的想法里,陀螺仪被加入了 Steam Controller 手柄,HTC Vive 的手柄也有各种动作追踪仪器;这些想法可能最初都来源于 Steam 手柄的设计过程中。手柄最初还有些更激进的设计,比如在中心放置一块可定制化并且会随着游戏内容变化的触摸屏。但最后的最后,发布会上的手柄偏向保守了许多,但也有诸如双触摸板和内置软件等黑科技。Valve 也考虑过制作面向笔记本类型硬件的 Steam Machines 和 SteamOS。这个企划最终没有任何成果,但也许 “Smach Z” 手持游戏机会是发展的方向之一。
|
||||||
|
|
||||||
在 [2013年九月][3],Valve 对外界宣布了 Steam Machines 和 SteamOS, 并且预告会在 2014 年中发布。前述的 300 台原型机在当年 12 月被分发给了测试者们,随后次年 1 月,2000 台原型机又被分发给了开发者们。SteamOS 也在那段时间被分发给有 Linux 经验的测试者们试用。根据当时的测试反馈,Valve 最终决定把产品发布延期到 2015 年 11 月。
|
在 [2013 年九月][3],Valve 对外界宣布了 Steam Machines 和 SteamOS, 并且预告会在 2014 年中发布。前述的 300 台原型机在当年 12 月分发给了测试者们,随后次年 1 月,又分发给了开发者们 2000 台原型机。SteamOS 也在那段时间分发给有 Linux 经验的测试者们试用。根据当时的测试反馈,Valve 最终决定把产品发布延期到 2015 年 11 月。
|
||||||
|
|
||||||
SteamOS 的延期跳票给合作伙伴带来了问题;戴尔的 Steam Machine 由于早发售了一年结果不得不改为搭配了额外软件甚至运行着 Windows 操作系统的 Alienware Alpha。
|
SteamOS 的延期跳票给合作伙伴带来了问题;戴尔的 Steam Machine 由于早发售了一年,结果不得不改为搭配了额外软件、甚至运行着 Windows 操作系统的 Alienware Alpha。
|
||||||
|
|
||||||
**正式发布**
|
### 正式发布
|
||||||
|
|
||||||
在最终的正式发布会上,Valve 和 OEM 合作商们发布了 Steam Machines,同时 Valve 还推出了 Steam Controller 手柄和 Steam Link 串流游戏设备。Valve 也在线下零售行业比如 GameStop 里开辟了货架空间。在发布会前,有几家 OEM 合作商退出了与 Valve 的合作;比如 Origin PC 和 Falcon Northwest 这两家高端精品主机设计商。他们宣称 Steam 生态的性能问题和一些限制迫使他们决定弃用 SteamOS。
|
在最终的正式发布会上,Valve 和 OEM 合作商们发布了 Steam Machines,同时 Valve 还推出了 Steam Controller 手柄和 Steam Link 串流游戏设备。Valve 也在线下零售行业比如 GameStop 里开辟了货架空间。在发布会前,有几家 OEM 合作商退出了与 Valve 的合作;比如 Origin PC 和 Falcon Northwest 这两家高端精品主机设计商。他们宣称 Steam 生态的性能问题和一些限制迫使他们决定弃用 SteamOS。
|
||||||
|
|
||||||
Steam Machines 在发布后收到了褒贬不一的评价。另一方面 Steam Link 则普遍受到好评,很多人表示愿意在客厅电视旁为他们已有的 PC 系统购买 Steam Link, 而不是购置一台全新的 Steam Machine。Steam Controller 手柄则受到其丰富功能伴随而来的陡峭学习曲线影响,评价一败涂地。然而针对 Steam Machines 的批评则是最猛烈的。诸如 LinusTechTips 这样的评测团体 (译者:YouTube硬件界老大,个人也经常看他们节目) 注意到了主机的明显的不足,其中甚至不乏性能为题。很多厂商的 Machines 都被批评为性价比极低,特别是经过和玩家们自己组装的同配置机器或者电视主机做对比之后。SteamOS 而被批评为兼容性有问题,Bugs 太多,以及性能不及 Windows。在所有 Machines 里,戴尔的 Alienware Alpha 被评价为最有意思的一款,主要是由于品牌价值和机型外观极小的缘故。
|
Steam Machines 在发布后收到了褒贬不一的评价。另一方面 Steam Link 则普遍受到好评,很多人表示愿意在客厅电视旁为他们已有的 PC 系统购买 Steam Link, 而不是购置一台全新的 Steam Machine。Steam Controller 手柄则受到其丰富功能伴随而来的陡峭学习曲线影响,评价一败涂地。然而针对 Steam Machines 的批评则是最猛烈的。诸如 LinusTechTips 这样的评测团体 (LCTT 译注:YouTube 硬件界老大,个人也经常看他们节目)注意到了主机的明显的不足,其中甚至不乏性能为题。很多厂商的 Machines 都被批评为性价比极低,特别是经过和玩家们自己组装的同配置机器或者电视主机做对比之后。SteamOS 而被批评为兼容性有问题,Bug 太多,以及性能不及 Windows。在所有 Machines 里,戴尔的 Alienware Alpha 被评价为最有意思的一款,主要是由于品牌价值和机型外观极小的缘故。
|
||||||
|
|
||||||
通过把 Debian Linux 操作系统作为开发基础,Valve 得以为 SteamOS 平台找到很多原本就存在与 Steam 平台上的 Linux 兼容游戏来作为“首发游戏”。所以起初大家认为在“首发游戏”上 Steam Machines 对比其他新发布的主机优势明显。然而,很多宣称会在新平台上发布的游戏要么跳票要么被中断了。Rocket League 和 Mad Max 在宣布支持新平台整整一年后才真正发布,而 巫师3 和蝙蝠侠:阿克汉姆骑士 甚至从来没有发布在新平台上。就 巫师3 的情况而言,他们的开发者 CD Projekt Red 拒绝承认他们曾经说过要支持新平台;然而他们的游戏曾在宣布支持 Linux 和 SteamOS 的游戏列表里赫然醒目。雪上加霜的是,很多 AAA 级的大作甚至没被宣布移植,虽然最近这种情况稍有所好转了。
|
通过把 Debian Linux 操作系统作为开发基础,Valve 得以为 SteamOS 平台找到很多原本就存在与 Steam 平台上的 Linux 兼容游戏来作为“首发游戏”。所以起初大家认为在“首发游戏”上 Steam Machines 对比其他新发布的主机优势明显。然而,很多宣称会在新平台上发布的游戏要么跳票要么被中断了。Rocket League 和 Mad Max 在宣布支持新平台整整一年后才真正发布,而《巫师 3》和《蝙蝠侠:阿克汉姆骑士》甚至从来没有发布在新平台上。就《巫师 3》的情况而言,他们的开发者 CD Projekt Red 拒绝承认他们曾经说过要支持新平台;然而他们的游戏曾在宣布支持 Linux 和 SteamOS 的游戏列表里赫然醒目。雪上加霜的是,很多 AAA 级的大作甚至没宣布移植,虽然最近这种情况稍有所好转了。
|
||||||
|
|
||||||
**被忽视的**
|
### 被忽视的
|
||||||
|
|
||||||
在 Stame Machines 发售后,Valve 的开发者们很快转移到了其他项目的工作中去了。在当时,VR 项目最为被内部所重视,6 月份的时候大约有 1/3 的员工都在相关项目上工作。Valve 把 VR 视为亟待开发的一片领域,而他们的 Steam 则应该作为分发 VR 内容的生态环境。通过与 HTC 合作生产,Valve 设计并制造出了他们自己的 VR 头戴和手柄,并计划在将来更新换代。然而与此同时,Linux 和 Steam Machines 都渐渐淡出了视野。SteamVR 甚至直到最近才刚刚支持 Linux (其实还没对普通消费者开放使用,只在 SteamDevDays 上展示过对 Linux 的支持),而这一点则让我们怀疑 Valve 在 Stame Machines 和 Linux 的开发上是否下定了足够的决心。
|
在 Stame Machines 发售后,Valve 的开发者们很快转移到了其他项目的工作中去了。在当时,VR 项目最为内部所重视,6 月份的时候大约有 1/3 的员工都在相关项目上工作。Valve 把 VR 视为亟待开发的一片领域,而他们的 Steam 则应该作为分发 VR 内容的生态环境。通过与 HTC 合作生产,Valve 设计并制造出了他们自己的 VR 头戴和手柄,并计划在将来更新换代。然而与此同时,Linux 和 Steam Machines 都渐渐淡出了视野。SteamVR 甚至直到最近才刚刚支持 Linux (其实还没对普通消费者开放使用,只在 SteamDevDays 上展示过对 Linux 的支持),而这一点则让我们怀疑 Valve 在 Stame Machines 和 Linux 的开发上是否下定了足够的决心。
|
||||||
|
|
||||||
SteamOS 自发布以来几乎止步不前。SteamOS 2.0 作为上一个大版本号更新,几乎只是同步了 Debian 上游的变化,而且还需要用户重新安装整个系统,而之后的小补丁也只是在做些上游更新的配合。当 Valve 在其他事关性能和用户体验的项目,例如 Mesa,上进步匪浅的时候,针对 Steam Machines 的相关项目则少有顾及。
|
SteamOS 自发布以来几乎止步不前。SteamOS 2.0 作为上一个大版本号更新,几乎只是同步了 Debian 上游的变化,而且还需要用户重新安装整个系统,而之后的小补丁也只是在做些上游更新的配合。当 Valve 在其他事关性能和用户体验的项目(例如 Mesa)上进步匪浅的时候,针对 Steam Machines 的相关项目则少有顾及。
|
||||||
|
|
||||||
很多原本应有的功能都从未完成。Steam 的内置功能,例如聊天和直播,都依然处于较弱的状态,而且这种落后会影响所有平台上的 Steam 用户体验。更具体来说,Steam 没有像其他主流主机平台一样把诸如 Netflix,Twitch 和 Spotify 之类的服务集成到客户端里,而通过 Steam 内置的浏览器使用这些服务则体验极差,甚至无法使用;而如果要使用第三方软件则需要开启 Terminal,而且很多软件甚至无法支持控制手柄 —— 无论从哪方面讲这样的用户界面体验都糟糕透顶。
|
很多原本应有的功能都从未完成。Steam 的内置功能,例如聊天和直播,都依然处于较弱的状态,而且这种落后会影响所有平台上的 Steam 用户体验。更具体来说,Steam 没有像其他主流主机平台一样把诸如 Netflix、Twitch 和 Spotify 之类的服务集成到客户端里,而通过 Steam 内置的浏览器使用这些服务则体验极差,甚至无法使用;而如果要使用第三方软件则需要开启 Terminal,而且很多软件甚至无法支持控制手柄 —— 无论从哪方面讲这样的用户界面体验都糟糕透顶。
|
||||||
|
|
||||||
Valve 同时也几乎没有花任何力气去推广他们的新平台而选择把一切都交由 OEM 厂商们去做。然而,几乎所有 OEM 合作商们要么是高端主机定制商,要么是电脑生产商,要么是廉价电脑公司(译者:简而言之没有一家有大型宣传渠道)。在所有 OEM 中,只有戴尔是 PC 市场的大碗,也只有他们真正给 Steam Machines 做了广告宣传。
|
Valve 同时也几乎没有花任何力气去推广他们的新平台,而选择把一切都交由 OEM 厂商们去做。然而,几乎所有 OEM 合作商们要么是高端主机定制商,要么是电脑生产商,要么是廉价电脑公司(LCTT 译注:简而言之没有一家有大型宣传渠道)。在所有 OEM 中,只有戴尔是 PC 市场的大碗,也只有他们真正给 Steam Machines 做了广告宣传。
|
||||||
|
|
||||||
最终销量也不尽人意。截至 2016 年 6 月,7 个月间 Steam Controller 手柄的销量在包括捆绑销售的情况下仅销售 500,000 件。这让 Steam Machines 的零售情况差到只能被归类到十万俱乐部的最底部。对比已经存在的巨大 PC 和主机游戏平台,可以说销量极低。
|
最终销量也不尽人意。截至 2016 年 6 月,7 个月间 Steam Controller 手柄的销量在包括捆绑销售的情况下仅销售 500,000 件。这让 Steam Machines 的零售情况差到只能被归类到十万俱乐部的最底部。对比已经存在的巨大 PC 和主机游戏平台,可以说销量极低。
|
||||||
|
|
||||||
**事后诸葛亮**
|
### 事后诸葛亮
|
||||||
|
|
||||||
既然知道了 Steam Machines 的历史,我们又能否总结出失败的原因以及可能存在的翻身改进呢?
|
既然知道了 Steam Machines 的历史,我们又能否总结出失败的原因以及可能存在的翻身改进呢?
|
||||||
|
|
||||||
_视野与目标_
|
#### 视野与目标
|
||||||
|
|
||||||
Steam Machines 从来没搞清楚他们在市场里的定位究竟是什么,也从来没说清楚他们具体有何优势。从 PC 市场的角度来说,自己搭建台式机已经非常普及并且往往让电脑的可以匹配玩家自己的目标,同时升级性也非常好。从主机平台的角度来说,Steam Machines 又被主机本身的相对廉价所打败,虽然算上游戏可能稍微便宜一些,但主机上的用户体验也直白很多。
|
Steam Machines 从来没搞清楚他们在市场里的定位究竟是什么,也从来没说清楚他们具体有何优势。从 PC 市场的角度来说,自己搭建台式机已经非常普及,并且往往可以让电脑可以匹配玩家自己的目标,同时升级性也非常好。从主机平台的角度来说,Steam Machines 又被主机本身的相对廉价所打败,虽然算上游戏可能稍微便宜一些,但主机上的用户体验也直白很多。
|
||||||
|
|
||||||
PC 用户会把多功能性看得很重,他们不仅能用电脑打游戏,也一样能办公和做各种各样的事情。即使 Steam Machines 也是跑着的 SteamOS 操作系统的自由的 Linux 电脑,但操作系统和市场宣传加固了 PC 玩家们对 Steam Machines 是不可定制硬件,低价的更接近主机的印象。即使这些 PC 用户能接受在客厅里购置一台 Steam Machines,他们也有 Steam Link 可以选择,而且很多更小型机比如 NUC 和 Mini-ITX 主板定制机可以让他们搭建更适合放在客厅里的电脑。SteamOS 软件也允许把这些硬件转变为 Steam Machines,但寻求灵活性和兼容性的用户通常都会使用一般 Linux 发行版或者 Windows。二矿最近的 Windows 和 Linux 桌面环境都让维护一般用户的操作系统变得自动化和简单了。
|
PC 用户会把多功能性看得很重,他们不仅能用电脑打游戏,也一样能办公和做各种各样的事情。即使 Steam Machines 也是跑着的 SteamOS 操作系统的自由的 Linux 电脑,但操作系统和市场宣传加深了 PC 玩家们对 Steam Machines 是不可定制的硬件、低价的、更接近主机的印象。即使这些 PC 用户能接受在客厅里购置一台 Steam Machines,他们也有 Steam Link 可以选择,而且很多更小型机比如 NUC 和 Mini-ITX 主板定制机可以让他们搭建更适合放在客厅里的电脑。SteamOS 软件也允许把这些硬件转变为 Steam Machines,但寻求灵活性和兼容性的用户通常都会使用一般 Linux 发行版或者 Windows。何况最近的 Windows 和 Linux 桌面环境都让维护一般用户的操作系统变得自动化和简单了。
|
||||||
|
|
||||||
电视主机用户们则把易用性放在第一。虽然近年来主机的功能也逐渐扩展,比如可以播放视频或者串流,但总体而言用户还是把即插即用即玩,不用担心兼容性和性能问题和低门槛放在第一。主机的使用寿命也往往较常,一般在 4-7 年左右,而统一固定的硬件也让游戏开发者们能针对其更好的优化和调试软件。现在刚刚新起的中代升级,例如天蝎和 PS 4 Pro 则可能会打破这样统一的游戏体验,但无论如何厂商还是会要求开发者们需要保证游戏在原机型上的体验。为了提高用户粘性,主机也会有自己的社交系统和独占游戏。而主机上的游戏也有实体版,以便将来重用或者二手转卖,这对零售商和用户都是好事儿。Steam Machines 则完全没有这方面的保证;即使长的像一台客厅主机,他们却有 PC 高昂的价格和复杂的硬件情况。
|
电视主机用户们则把易用性放在第一。虽然近年来主机的功能也逐渐扩展,比如可以播放视频或者串流,但总体而言用户还是把即插即用即玩、不用担心兼容性和性能问题和低门槛放在第一。主机的使用寿命也往往较长,一般在 4-7 年左右,而统一固定的硬件也让游戏开发者们能针对其更好的优化和调试软件。现在刚刚兴起的中生代升级,例如天蝎和 PS 4 Pro 则可能会打破这样统一的游戏体验,但无论如何厂商还是会要求开发者们需要保证游戏在原机型上的体验。为了提高用户粘性,主机也会有自己的社交系统和独占游戏。而主机上的游戏也有实体版,以便将来重用或者二手转卖,这对零售商和用户都是好事儿。Steam Machines 则完全没有这方面的保证;即使长的像一台客厅主机,他们却有 PC 高昂的价格和复杂的硬件情况。
|
||||||
|
|
||||||
_妥协_
|
#### 妥协
|
||||||
|
|
||||||
综上所述,Steam Machines 可以说是“集大成者”,吸取了两边的缺点,又没有自己明确的定位。更糟糕的是 Steam Machines 还展现了 PC 和主机都没有的毛病,比如没有 AAA 大作,又没有 Netflix 这样的客户端。抛开这些不说,Valve 在提高他们产品这件事上几乎没有出力,甚至没有尝试着解决 PC 和主机两头定位矛盾这一点。
|
综上所述,Steam Machines 可以说是“集大成者”,吸取了两边的缺点,又没有自己明确的定位。更糟糕的是 Steam Machines 还展现了 PC 和主机都没有的毛病,比如没有 AAA 大作,又没有 Netflix 这样的客户端。抛开这些不说,Valve 在提高他们产品这件事上几乎没有出力,甚至没有尝试着解决 PC 和主机两头定位矛盾这一点。
|
||||||
|
|
||||||
@ -61,35 +61,35 @@ _妥协_
|
|||||||
|
|
||||||
而最复杂的是 Steam Machines 多变的硬件情况,这使得用户不仅要考虑价格还要考虑配置,还要考虑这个价格下和别的系统(PC 和主机)比起来划算与否。更关键的是,Valve 无论如何也应该做出某种自动硬件检测机制,这样玩家才能知道是否能玩某个游戏,而且这个测试既得简单明了,又要能支持 Steam 上几乎所有游戏。同时,Valve 还要操心未来游戏对配置需求的变化,比如2016 年的 "A" 等主机三年后该给什么评分呢?
|
而最复杂的是 Steam Machines 多变的硬件情况,这使得用户不仅要考虑价格还要考虑配置,还要考虑这个价格下和别的系统(PC 和主机)比起来划算与否。更关键的是,Valve 无论如何也应该做出某种自动硬件检测机制,这样玩家才能知道是否能玩某个游戏,而且这个测试既得简单明了,又要能支持 Steam 上几乎所有游戏。同时,Valve 还要操心未来游戏对配置需求的变化,比如2016 年的 "A" 等主机三年后该给什么评分呢?
|
||||||
|
|
||||||
_Valve, 个人努力与公司结构_
|
#### Valve, 个人努力与公司结构
|
||||||
|
|
||||||
尽管 Valve 在 Steam 上创造了辉煌,但其公司的内部结构可能对于开发一个像 Steam Machines 一样的平台是有害的。他们几乎没有领导的自由办公结构,以及所有人都可以自由移动到想要工作的项目组里决定了他们具有极大的创新,研发,甚至开发能力。据说 Valve 只愿意招他们眼中的的 "顶尖人才",通过极其严格的筛选标准,并通过让他们在自己认为“有意义”的项目里工作以保持热情。然而这种思路很可能是错误的;拉帮结派总是存在,而 G胖 的话或许比公司手册上写的还管用,而又有人时不时会由于特殊原因被雇佣或解雇。
|
尽管 Valve 在 Steam 上创造了辉煌,但其公司的内部结构可能对于开发一个像 Steam Machines 一样的平台是有害的。他们几乎没有领导的自由办公结构,以及所有人都可以自由移动到想要工作的项目组里决定了他们具有极大的创新,研发,甚至开发能力。据说 Valve 只愿意招他们眼中的的 “顶尖人才”,通过极其严格的筛选标准,并通过让他们在自己认为“有意义”的项目里工作以保持热情。然而这种思路很可能是错误的;拉帮结派总是存在,而 G胖的话或许比公司手册上写的还管用,而又有人时不时会由于特殊原因被雇佣或解雇。
|
||||||
|
|
||||||
正因为如此,很多虽不闪闪发光甚至维护起来有些无聊但又需要大量时间的项目很容易枯萎。Valve 的客服已是被人诟病已久的毛病,玩家经常觉得被无视了,而 Valve 则经常不到万不得已法律要求的情况下绝不行动:例如自动退款系统,就是在澳大利亚和欧盟法律的要求下才被加入的;更有目前还没结案的华盛顿州 CS:GO 物品在线赌博网站一案。
|
正因为如此,很多虽不闪闪发光甚至维护起来有些无聊但又需要大量时间的项目很容易枯萎。Valve 的客服已是被人诟病已久的毛病,玩家经常觉得被无视了,而 Valve 则经常不到万不得已、法律要求的情况下绝不行动:例如自动退款系统,就是在澳大利亚和欧盟法律的要求下才被加入的;更有目前还没结案的华盛顿州 CS:GO 物品在线赌博网站一案。
|
||||||
|
|
||||||
各种因素最后也反映在 Steam Machines 这一项目上。Valve 方面的跳票迫使一些合作方做出了尴尬的决定,比如戴尔提前一年发布了 Alienware Alpha 外观的 Steam Machine 就在一年后的正式发布时显得硬件状况落后了。跳票很可能也导致了游戏数量上的问题。开发者和硬件合作商的对跳票和最终毫无轰动的发布也不明朗。Valve 的 VR 平台干脆直接不支持 Linux,而直到最近,SteamVR 都风风火火迭代了好几次之后,SteamOS 和 Linux 依然不支持 VR。
|
各种因素最后也反映在 Steam Machines 这一项目上。Valve 方面的跳票迫使一些合作方做出了尴尬的决定,比如戴尔提前一年发布了 Alienware Alpha 外观的 Steam Machine 就在一年后的正式发布时显得硬件状况落后了。跳票很可能也导致了游戏数量上的问题。开发者和硬件合作商的对跳票和最终毫无轰动的发布也不明朗。Valve 的 VR 平台干脆直接不支持 Linux,而直到最近,SteamVR 都风风火火迭代了好几次之后,SteamOS 和 Linux 依然不支持 VR。
|
||||||
|
|
||||||
_“长线钓鱼”_
|
#### “长线钓鱼”
|
||||||
|
|
||||||
尽管 Valve 方面对未来的规划毫无透露,有些人依然认为 Valve 在 Steam Machine 和 SteamOS 上是放长线钓大鱼。他们论点是 Steam 本身也是这样的项目 —— 一开始作为游戏补丁平台出现,到现在无敌的游戏零售和玩家社交网络。虽然 Valve 的独占游戏比如 Half-Life 2 和 CS 也帮助了 Steam 平台的传播。但现今我们完全无法看到 Valve 像当初对 Steam 那样上心 Steam Machines。同时现在 Steam Machines 也面临着 Steam 从没碰到过的激烈竞争。而这些竞争里自然也包含 Valve 自己的那些把 Windows 作为平台的 Steam 客户端。
|
尽管 Valve 方面对未来的规划毫无透露,有些人依然认为 Valve 在 Steam Machine 和 SteamOS 上是放长线钓大鱼。他们论点是 Steam 本身也是这样的项目 —— 一开始作为游戏补丁平台出现,到现在无敌的游戏零售和玩家社交网络。虽然 Valve 的独占游戏比如《半条命 2》和 《CS》 也帮助了 Steam 平台的传播。但现今我们完全无法看到 Valve 像当初对 Steam 那样上心 Steam Machines。同时现在 Steam Machines 也面临着 Steam 从没碰到过的激烈竞争。而这些竞争里自然也包含 Valve 自己的那些把 Windows 作为平台的 Steam 客户端。
|
||||||
|
|
||||||
_真正目的_
|
#### 真正目的
|
||||||
|
|
||||||
介于投入在 Steam Machines 上的努力如此之少,有些人怀疑整个产品平台是不是仅仅作为某种博弈的筹码才被开发出来。原初 Steam Machines 就发家于担心微软和苹果通过自己的应用市场垄断游戏的反制手段当中,Valve 寄希望于 Steam Machines 可以在不备之时脱离那些操作系统的支持而运行,同时也是提醒开发者们,也许有一日整个 Steam 平台会独立出来。而当微软和苹果等方面的风口没有继续收紧的情况下,Valve 自然就放慢了开发进度。然而我不这样认为;Valve 其实已经花了不少精力与硬件商和游戏开发者们共同推行这件事,不可能仅仅是为了吓吓他人就终止项目。你可以把这件事想成,微软和 Valve 都在吓唬对方 —— 微软推出了突然收紧的 Windows 8 而 Valve 则展示了一下可以独立门户的能力。
|
鉴于投入在 Steam Machines 上的努力如此之少,有些人怀疑整个产品平台是不是仅仅作为某种博弈的筹码才被开发出来。原初 Steam Machines 就发家于担心微软和苹果通过自己的应用市场垄断游戏的反制手段当中,Valve 寄希望于 Steam Machines 可以在不备之时脱离那些操作系统的支持而运行,同时也是提醒开发者们,也许有一日整个 Steam 平台会独立出来。而当微软和苹果等方面的风口没有继续收紧的情况下,Valve 自然就放慢了开发进度。然而我不这样认为;Valve 其实已经花了不少精力与硬件商和游戏开发者们共同推行这件事,不可能仅仅是为了吓吓他人就终止项目。你可以把这件事想成,微软和 Valve 都在吓唬对方 —— 微软推出了突然收紧的 Windows 8 ,而 Valve 则展示了一下可以独立门户的能力。
|
||||||
|
|
||||||
但即使如此,谁能保证开发者不会愿意跟着微软的封闭环境跑了呢?万一微软最后能提供更好的待遇和用户群体呢?更何况,微软现在正大力推行 Xbox 和 Windows 的交叉和整合,甚至 Xbox 独占游戏也出现在 Windows 上,这一切都没有损害 Windows 原本的平台性定位 —— 谁还能说微软方面不是 Steam 的直接竞争对手呢?
|
但即使如此,谁能保证开发者不会愿意跟着微软的封闭环境跑了呢?万一微软最后能提供更好的待遇和用户群体呢?更何况,微软现在正大力推行 Xbox 和 Windows 的交叉和整合,甚至 Xbox 独占游戏也出现在 Windows 上,这一切都没有损害 Windows 原本的平台性定位 —— 谁还能说微软方面不是 Steam 的直接竞争对手呢?
|
||||||
|
|
||||||
还会有人说这一切一切都是为了推进 Linux 生态环境尽快接纳 PC 游戏,而 Steam Machines 只是想为此大力推一把。但如果是这样,那这个目的实在是性价比极低,因为本愿意支持 Linux 的自然会开发,而 Steam Machines 这一出甚至会让开发者对平台期待额落空从而伤害到他们。
|
还会有人说这一切一切都是为了推进 Linux 生态环境尽快接纳 PC 游戏,而 Steam Machines 只是想为此大力推一把。但如果是这样,那这个目的实在是性价比极低,因为本愿意支持 Linux 的自然会开发,而 Steam Machines 这一出甚至会让开发者对平台期待额落空从而伤害到他们。
|
||||||
|
|
||||||
**大家眼中 Valve 曾经的机会**
|
### 大家眼中 Valve 曾经的机会
|
||||||
|
|
||||||
我认为 Steam Machines 的创意还是很有趣的,而也有一个与之匹配的市场,但就结果而言 Valve 投入的创意和努力还不够多,而定位模糊也伤害了这个产品。我认为 Steam Machines 的优势在于能砍掉 PC 游戏传统的复杂性,比如硬件问题,整机寿命和维护等;但又能拥有游戏便宜,可以打 Mod 等好处,而且也可以做各种定制化以满足用户需求。但他们必须要让产品的核心内容:价格,市场营销,机型产品线还有软件的质量有所保证才行。
|
我认为 Steam Machines 的创意还是很有趣的,而也有一个与之匹配的市场,但就结果而言 Valve 投入的创意和努力还不够多,而定位模糊也伤害了这个产品。我认为 Steam Machines 的优势在于能砍掉 PC 游戏传统的复杂性,比如硬件问题、整机寿命和维护等;但又能拥有游戏便宜,可以打 Mod 等好处,而且也可以做各种定制化以满足用户需求。但他们必须要让产品的核心内容:价格、市场营销、机型产品线还有软件的质量有所保证才行。
|
||||||
|
|
||||||
我认为 Steam Machines 可以做出一点妥协,比如硬件升级性(尽管这一点还是有可能被保留下来的 —— 但也要极为小心整个过程对用户体验的影响)和产品选择性,来减少摩擦成本。PC 一直会是一个并列的选项。想给用户产品可选性带来的只有一个困境,成吨的质量低下的 Steam Machines 根本不能解决。Valve 得自己造一台旗舰机型来指明 Steam Machines 的方向。毫无疑问,Alienware 的产品是最接近理想目标的,但他说到底也不是 Valve 的官方之作。Valve 内部不乏优秀的工业设计人才,如果他们愿意投入足够多的重视,我认为结果也许会值得他们努力。而像戴尔和 HTC 这样的公司则可以用他们丰富的经验帮 Valve 制造成品。直接钦定 Steam Machines 的硬件周期,并且在期间只推出 1-2 台机型也有助于帮助解决问题,更不用说他们还可以依次和开发商们确立性能的基准线。我不知道 OEM 合作商们该怎么办;如果 Valve 专注于自己的几台设备里,OEM 们很可能会变得多余甚至拖平台后腿。
|
我认为 Steam Machines 可以做出一点妥协,比如硬件升级性(尽管这一点还是有可能被保留下来的 —— 但也要极为小心整个过程对用户体验的影响)和产品选择性,来减少摩擦成本。PC 一直会是一个并列的选项。想给用户产品可选性带来的只有一个困境,成吨的质量低下的 Steam Machines 根本不能解决。Valve 得自己造一台旗舰机型来指明 Steam Machines 的方向。毫无疑问,Alienware 的产品是最接近理想目标的,但它说到底也不是 Valve 的官方之作。Valve 内部不乏优秀的工业设计人才,如果他们愿意投入足够多的重视,我认为结果也许会值得他们努力。而像戴尔和 HTC 这样的公司则可以用他们丰富的经验帮 Valve 制造成品。直接钦定 Steam Machines 的硬件周期,并且在期间只推出 1-2 台机型也有助于帮助解决问题,更不用说他们还可以依次和开发商们确立性能的基准线。我不知道 OEM 合作商们该怎么办;如果 Valve 专注于自己的几台设备里,OEM 们很可能会变得多余甚至拖平台后腿。
|
||||||
|
|
||||||
我觉得修复软件问题是最关键的。很多问题在严重拖着 Steam Machines 的后退,比如缺少主机上遍地都是,又能轻易安装在 PC 上的的 Netflix 和 Twitch,即使做好了客厅体验问题依然是严重的败笔。即使 Valve 已经在逐步购买电影的版权以便在 Steam 上发售,我觉得用户还是会倾向于去使用已经在市场上建立口碑的一些串流服务。这些问题需要被严肃地对待,因为玩家日益倾向于把主机作为家庭影院系统的一部分。同时,修复 Steam 客户端和平台的问题也很重要,和更多第三方服务商合作增加内容应该会是个好主意。性能问题和 Linux 下的显卡问题也很严重,不过好在他们最近在慢慢进步。移植游戏也是个问题。类似 Feral Interactive 或者 Aspyr Media 这样的游戏移植商可以帮助扩展 Steam 的商店游戏数量,但联系开发者和出版社可能会有问题,而且这两家移植商经常在移植的容器上搞自己的花样。Valve 已经在帮助游戏工作室自己移植游戏了,比如 Rocket League,不过这种情况很少见,而且就算 Valve 去帮忙了,也是非常符合 Valve 风格的拖拉。而 AAA 大作这一块内容也绝不应该被忽略 —— 近来这方面的情况已经有极大好转了,虽然 Linux 平台的支持好了很多,但在玩家数量不够以及 Valve 为 Steam Machines 提供的开发帮助甚少的情况下,Bethesda 这样的开发商依然不愿意移植游戏;同时,也有像 Denuvo 一样缺乏数字版权管理的公司难以向 Steam Machines 移植游戏。
|
我觉得修复软件问题是最关键的。很多问题在严重拖着 Steam Machines 的后腿,比如缺少主机上遍地都是、又能轻易安装在 PC 上的的 Netflix 和 Twitch,那么即使做好了客厅体验问题依然是严重的败笔。即使 Valve 已经在逐步购买电影的版权以便在 Steam 上发售,我觉得用户还是会倾向于去使用已经在市场上建立口碑的一些流媒体服务。这些问题需要被严肃地对待,因为玩家日益倾向于把主机作为家庭影院系统的一部分。同时,修复 Steam 客户端和平台的问题也很重要,和更多第三方服务商合作增加内容应该会是个好主意。性能问题和 Linux 下的显卡问题也很严重,不过好在他们最近在慢慢进步。移植游戏也是个问题。类似 Feral Interactive 或者 Aspyr Media 这样的游戏移植商可以帮助扩展 Steam 的商店游戏数量,但联系开发者和出版社可能会有问题,而且这两家移植商经常在移植的容器上搞自己的花样。Valve 已经在帮助游戏工作室自己移植游戏了,比如 Rocket League,不过这种情况很少见,而且就算 Valve 去帮忙了,也是非常符合 Valve 拖拉的风格。而 AAA 大作这一块内容也绝不应该被忽略 —— 近来这方面的情况已经有极大好转了,虽然 Linux 平台的支持好了很多,但在玩家数量不够以及 Valve 为 Steam Machines 提供的开发帮助甚少的情况下,Bethesda 这样的开发商依然不愿意移植游戏;同时,也有像 Denuvo 一样缺乏数字版权管理的公司难以向 Steam Machines 移植游戏。
|
||||||
|
|
||||||
在我看来 Valve 需要在除了软件和硬件的地方也多花些功夫。如果他们只有一个机型的话,他们可以很方便的在硬件生产上贴点钱。这样 Steam Machines 的价格就能跻身主机的行列,而且还能比自己组装 PC 要便宜。针对正确的市场群体做营销也很关键,即便我们还不知道目标玩家应该是谁(我个人会对这样的 Steam Machines 感兴趣,而且我有一整堆已经在 Steam 上以相当便宜的价格买好的游戏)。最后,我觉得零售商们其实不会对 Valve 的计划很感冒,毕竟他们要靠卖和倒卖实体游戏赚钱。
|
在我看来 Valve 需要在除了软件和硬件的地方也多花些功夫。如果他们只有一个机型的话,他们可以很方便的在硬件生产上贴点钱。这样 Steam Machines 的价格就能跻身主机的行列,而且还能比自己组装 PC 要便宜。针对正确的市场群体做营销也很关键,即便我们还不知道目标玩家应该是谁(我个人会对这样的 Steam Machines 感兴趣,而且我有一整堆已经在 Steam 上以相当便宜的价格买下的游戏)。最后,我觉得零售商们其实不会对 Valve 的计划很感冒,毕竟他们要靠卖和倒卖实体游戏赚钱。
|
||||||
|
|
||||||
就算 Valve 在产品和平台上采纳过这些改进,我也不知道怎样才能激活 Steam Machines 的全市场潜力。总的来说,Valve 不仅得学习自己的经验教训,还应该参考曾经有过类似尝试的厂商们,比如尝试依靠开放平台的 3DO 和 Pippin;又或者那些从台式机体验的竞争力退赛的那些公司,其实 Valve 如今的情况和他们也有几分相似。亦或者他们也可以观察一下任天堂 Switch —— 毕竟任天堂也在尝试跨界的创新。
|
就算 Valve 在产品和平台上采纳过这些改进,我也不知道怎样才能激活 Steam Machines 的全市场潜力。总的来说,Valve 不仅得学习自己的经验教训,还应该参考曾经有过类似尝试的厂商们,比如尝试依靠开放平台的 3DO 和 Pippin;又或者那些从台式机体验的竞争力退赛的那些公司,其实 Valve 如今的情况和他们也有几分相似。亦或者他们也可以观察一下任天堂 Switch —— 毕竟任天堂也在尝试跨界的创新。
|
||||||
|
|
||||||
@ -103,7 +103,7 @@ via: https://www.gamingonlinux.com/articles/user-editorial-steam-machines-steamo
|
|||||||
|
|
||||||
作者:[calvin][a]
|
作者:[calvin][a]
|
||||||
译者:[Moelf](https://github.com/Moelf)
|
译者:[Moelf](https://github.com/Moelf)
|
||||||
校对:[校对者ID](https://github.com/校对者ID)
|
校对:[wxy](https://github.com/wxy)
|
||||||
|
|
||||||
本文由 [LCTT](https://github.com/LCTT/TranslateProject) 原创编译,[Linux中国](https://linux.cn/) 荣誉推出
|
本文由 [LCTT](https://github.com/LCTT/TranslateProject) 原创编译,[Linux中国](https://linux.cn/) 荣誉推出
|
||||||
|
|
@ -1,77 +1,59 @@
|
|||||||
JavaScript 函数式编程介绍
|
JavaScript 函数式编程介绍
|
||||||
============================================================
|
============================================================
|
||||||
|
|
||||||
### 探索函数式编程和通过它让你的程序更具有可读性和易于调试
|
> 探索函数式编程,通过它让你的程序更具有可读性和易于调试
|
||||||
|
|
||||||
|
|
||||||

|

|
||||||
|
|
||||||
>Image credits : Steve Jurvetson via [Flickr][80] (CC-BY-2.0)
|
>Image credits : Steve Jurvetson via [Flickr][80] (CC-BY-2.0)
|
||||||
|
|
||||||
当 Brendan Eich 在 1995 年创造了 JavaScript,他打算[将 Scheme 移植到浏览器里][81] 。Scheme 作为 Lisp 的方言,是一种函数式编程语言。而当 Eich 被告知新的语言应该是一种可以与 Java 相比的脚本语言后,他最终确立了一种拥有 C 风格语法的语言(也和 Java 一样),但将函数视作一等公民。Java 直到版本 8 才从技术上将函数视为一等公民,虽然你可以用匿名类来模拟它。这个特性允许 JavaScript 通过函数式范式编程。
|
当 Brendan Eich 在 1995 年创造 JavaScript 时,他原本打算[将 Scheme 移植到浏览器里][81] 。Scheme 作为 Lisp 的方言,是一种函数式编程语言。而当 Eich 被告知新的语言应该是一种可以与 Java 相比的脚本语言后,他最终确立了一种拥有 C 风格语法的语言(也和 Java 一样),但将函数视作一等公民。而 Java 直到版本 8 才从技术上将函数视为一等公民,虽然你可以用匿名类来模拟它。这个特性允许 JavaScript 通过函数式范式编程。
|
||||||
|
|
||||||
JavaScript 是一个多范式语言,允许你自由地混合和使用面向对象式,过程式和函数式的范式。最近,函数式编程越来越火热。在诸如 [Angular][82] 和 [React ][83]这样的框架中,通过使用不可变数据结构实际上可以提高性能。不可变是函数式编程的核心原则,纯函数和它使得编写和调试程序变得更加容易。使用函数来代替程序的循环可以提高程序的可读性并使它更加优雅。总之,函数式编程拥有很多优点。
|
JavaScript 是一个多范式语言,允许你自由地混合和使用面向对象式、过程式和函数式的编程范式。最近,函数式编程越来越火热。在诸如 [Angular][82] 和 [React][83] 这样的框架中,通过使用不可变数据结构可以切实提高性能。不可变是函数式编程的核心原则,它以及纯函数使得编写和调试程序变得更加容易。使用函数来代替程序的循环可以提高程序的可读性并使它更加优雅。总之,函数式编程拥有很多优点。
|
||||||
|
|
||||||
### 什么不是函数式编程
|
### 什么不是函数式编程
|
||||||
|
|
||||||
在讨论什么是函数式编程前,让我们先排除那些不属于函数式编程的东西。实际上它们是你需要丢弃的语言组件(再见,老朋友):
|
在讨论什么是函数式编程前,让我们先排除那些不属于函数式编程的东西。实际上它们是你需要丢弃的语言组件(再见,老朋友):
|
||||||
|
|
||||||
|
* 循环:
|
||||||
|
* `while`
|
||||||
* 循环
|
* `do...while`
|
||||||
* **while**
|
* `for`
|
||||||
|
* `for...of`
|
||||||
* **do...while**
|
* `for...in`
|
||||||
|
* 用 `var` 或者 `let` 来声明变量
|
||||||
* **for**
|
|
||||||
|
|
||||||
* **for...of**
|
|
||||||
|
|
||||||
* **for...in**
|
|
||||||
* 用 **var** 或者 **let** 来声明变量
|
|
||||||
* 没有返回值的函数
|
* 没有返回值的函数
|
||||||
* 改变对象的属性 (比如: **o.x = 5;**)
|
* 改变对象的属性 (比如: `o.x = 5;`)
|
||||||
* 改变 Array 本身的方法
|
* 改变数组本身的方法:
|
||||||
* **copyWithin**
|
* `copyWithin`
|
||||||
|
* `fill`
|
||||||
* **fill**
|
* `pop`
|
||||||
|
* `push`
|
||||||
* **pop**
|
* `reverse`
|
||||||
|
* `shift`
|
||||||
* **push**
|
* `sort`
|
||||||
|
* `splice`
|
||||||
* **reverse**
|
* `unshift`
|
||||||
|
* 改变映射本身的方法:
|
||||||
* **shift**
|
* `clear`
|
||||||
|
* `delete`
|
||||||
* **sort**
|
* `set`
|
||||||
|
* 改变集合本身的方法:
|
||||||
* **splice**
|
* `add`
|
||||||
|
* `clear`
|
||||||
* **unshift**
|
* `delete`
|
||||||
* 改变 Map 本身的方法
|
|
||||||
* **clear**
|
|
||||||
|
|
||||||
* **delete**
|
|
||||||
|
|
||||||
* **set**
|
|
||||||
* 改变 Set 本身的方法
|
|
||||||
* **add**
|
|
||||||
|
|
||||||
* **clear**
|
|
||||||
|
|
||||||
* **delete**
|
|
||||||
|
|
||||||
脱离这些特性应该如何编写程序呢?这是我们将在后面探索的问题。
|
脱离这些特性应该如何编写程序呢?这是我们将在后面探索的问题。
|
||||||
|
|
||||||
### 纯函数
|
### 纯函数
|
||||||
|
|
||||||
你的程序中包含函数不一定意味着你正在进行函数式编程。函数式范式将纯函数和非纯函数区分开。鼓励你编写纯函数。纯函数必须满足下面的两个属性:
|
你的程序中包含函数不一定意味着你正在进行函数式编程。函数式范式将<ruby>纯函数<rt>pure function</rt></ruby>和<ruby>非纯函数<rt>impure function</rt></ruby>区分开。鼓励你编写纯函数。纯函数必须满足下面的两个属性:
|
||||||
|
|
||||||
* **引用透明:**函数在传入相同的参数后永远返回相同的返回值。这意味着该函数不依赖于任何可变状态。
|
* 引用透明:函数在传入相同的参数后永远返回相同的返回值。这意味着该函数不依赖于任何可变状态。
|
||||||
* **Side-effect free无函数副作用:**函数不能导致任何副作用。副作用可能包括 I/O(比如 向终端或者日志文件写入),改变一个不可变的对象,对变量重新赋值等等。
|
* 无副作用:函数不能导致任何副作用。副作用可能包括 I/O(比如向终端或者日志文件写入),改变一个不可变的对象,对变量重新赋值等等。
|
||||||
|
|
||||||
我们来看一些例子。首先,**multiply** 就是一个纯函数的例子,它在传入相同的参数后永远返回相同的返回值,并且不会导致副作用。
|
|
||||||
|
|
||||||
|
我们来看一些例子。首先,`multiply` 就是一个纯函数的例子,它在传入相同的参数后永远返回相同的返回值,并且不会导致副作用。
|
||||||
|
|
||||||
```
|
```
|
||||||
function multiply(a, b) {
|
function multiply(a, b) {
|
||||||
@ -79,9 +61,7 @@ function multiply(a, b) {
|
|||||||
}
|
}
|
||||||
```
|
```
|
||||||
|
|
||||||
[view raw][5][pure-function-example.js][6] hosted with ❤ by [GitHub][7]
|
下面是非纯函数的例子。`canRide` 函数依赖捕获的 `heightRequirement` 变量。被捕获的变量不一定导致一个函数是非纯函数,除非它是一个可变的变量(或者可以被重新赋值)。这种情况下使用 `let` 来声明这个变量,意味着可以对它重新赋值。`multiply` 函数是非纯函数,因为它会导致在 console 上输出。
|
||||||
|
|
||||||
下面是非纯函数的例子。**canRide** 函数依赖捕获的 **heightRequirement** 变量。被捕获的变量不一定导致一个函数是非纯函数,除非它是一个可变的变量(或者可以被重新赋值)。这种情况下使用 **let** 来声明这个变量,意味着可以对它重新赋值。**multiply** 函数是非纯函数,因为它会导致在 console 上输出。
|
|
||||||
|
|
||||||
```
|
```
|
||||||
let heightRequirement = 46;
|
let heightRequirement = 46;
|
||||||
@ -98,36 +78,27 @@ function multiply(a, b) {
|
|||||||
}
|
}
|
||||||
```
|
```
|
||||||
|
|
||||||
|
|
||||||
[view raw][8][impure-functions.js][9] hosted with ❤ by [GitHub][10]
|
|
||||||
|
|
||||||
下面的列表包含着 JavaScript 内置的非纯函数。你可以指出它们不满足两个属性中的哪个吗?
|
下面的列表包含着 JavaScript 内置的非纯函数。你可以指出它们不满足两个属性中的哪个吗?
|
||||||
|
|
||||||
* **console.log**
|
* `console.log`
|
||||||
|
* `element.addEventListener`
|
||||||
|
* `Math.random`
|
||||||
|
* `Date.now`
|
||||||
|
* `$.ajax` (这里 `$` 代表你使用的 Ajax 库)
|
||||||
|
|
||||||
* **element.addEventListener**
|
理想的程序中所有的函数都是纯函数,但是从上面的函数列表可以看出,任何有意义的程序都将包含非纯函数。大多时候我们需要进行 AJAX 调用,检查当前日期或者获取一个随机数。一个好的经验法则是遵循 80/20 规则:函数中有 80% 应该是纯函数,剩下的 20% 的必要性将不可避免地是非纯函数。
|
||||||
|
|
||||||
* **Math.random**
|
|
||||||
|
|
||||||
* **Date.now**
|
|
||||||
|
|
||||||
* **$.ajax** (**$** 代表你使用的 Ajax 库)
|
|
||||||
|
|
||||||
理想的程序中所有的函数都是纯函数,但是从上面的函数列表可以看出,任何有意义的程序都将包含非纯函数。大多时候我们需要进行 AJAX 调用,检查当前日期或者获取一个随机数。一个好的经验法则是遵循80/20规则:函数中有80%应该是纯函数,剩下的20%的必要性将不可避免地是非纯函数。
|
|
||||||
|
|
||||||
使用纯函数有几个优点:
|
使用纯函数有几个优点:
|
||||||
|
|
||||||
* 它们很容易导出和调试,因为它们不依赖于可变的状态。
|
* 它们很容易导出和调试,因为它们不依赖于可变的状态。
|
||||||
|
|
||||||
* 返回值可以被缓存或者“记忆”来避免以后重复计算。
|
* 返回值可以被缓存或者“记忆”来避免以后重复计算。
|
||||||
|
|
||||||
* 它们很容易测试,因为没有需要模拟(mock)的依赖(比如日志,AJAX,数据库等等)。
|
* 它们很容易测试,因为没有需要模拟(mock)的依赖(比如日志,AJAX,数据库等等)。
|
||||||
|
|
||||||
你编写或者使用的函数返回空(换句话说它没有返回值),那代表它是非纯函数。
|
你编写或者使用的函数返回空(换句话说它没有返回值),那代表它是非纯函数。
|
||||||
|
|
||||||
### 不变性
|
### 不变性
|
||||||
|
|
||||||
让我们回到捕获变量的概念上。来看看 **canRide** 函数。我们认为它是一个非纯函数,因为 **heightRequirement** 变量可以被重新赋值。下面是一个构造出来的例子来说明如何用不可预测的值来对它重新赋值。
|
让我们回到捕获变量的概念上。来看看 `canRide` 函数。我们认为它是一个非纯函数,因为 `heightRequirement` 变量可以被重新赋值。下面是一个构造出来的例子来说明如何用不可预测的值来对它重新赋值。
|
||||||
|
|
||||||
```
|
```
|
||||||
let heightRequirement = 46;
|
let heightRequirement = 46;
|
||||||
@ -146,10 +117,7 @@ const mySonsHeight = 47;
|
|||||||
setInterval(() => console.log(canRide(mySonsHeight)), 500);
|
setInterval(() => console.log(canRide(mySonsHeight)), 500);
|
||||||
```
|
```
|
||||||
|
|
||||||
|
我要再次强调被捕获的变量不一定会使函数成为非纯函数。我们可以通过只是简单地改变 `heightRequirement` 的声明方式来使 `canRide` 函数成为纯函数。
|
||||||
[view raw][11][mutable-state.js][12] hosted with ❤ by [GitHub][13]
|
|
||||||
|
|
||||||
我要再次强调被捕获的变量不一定会使函数成为非纯函数。我们可以通过只是简单地改变 **heightRequirement** 的声明方式来使 **canRide** 函数成为纯函数。
|
|
||||||
|
|
||||||
```
|
```
|
||||||
const heightRequirement = 46;
|
const heightRequirement = 46;
|
||||||
@ -159,10 +127,7 @@ function canRide(height) {
|
|||||||
}
|
}
|
||||||
```
|
```
|
||||||
|
|
||||||
|
通过用 `const` 来声明变量意味着它不能被再次赋值。如果尝试对它重新赋值,运行时引擎将抛出错误;那么,如果用对象来代替数字来存储所有的“常量”怎么样?
|
||||||
[view raw][14][immutable-state.js][15] hosted with ❤ by [GitHub][16]
|
|
||||||
|
|
||||||
通过用 **const** 来声明变量意味着它不能被再次赋值。如果尝试对它重新赋值,运行时引擎将抛出错误;那么,如果用对象来代替数字来存储所有的“常量”怎么样?
|
|
||||||
|
|
||||||
```
|
```
|
||||||
const constants = {
|
const constants = {
|
||||||
@ -175,58 +140,53 @@ function canRide(height) {
|
|||||||
}
|
}
|
||||||
```
|
```
|
||||||
|
|
||||||
|
我们用了 `const` ,所以这个变量不能被重新赋值,但是还有一个问题:这个对象可以被改变。下面的代码展示了,为了真正使其不可变,你不仅需要防止它被重新赋值,你也需要不可变的数据结构。JavaScript 语言提供了 `Object.freeze` 方法来阻止对象被改变。
|
||||||
[view raw][17][captured-mutable-object.js][18] hosted with ❤ by [GitHub][19]
|
|
||||||
|
|
||||||
我们用了 **const** ,所以这个变量不能被重新赋值,但是还有一个问题:这个对象可以被改变。下面的代码展示了,为了真正使其不可变,你不仅需要防止它被重新赋值,你也需要不可变的数据结构。JavaScript 语言提供了 **Object.freeze** 方法来阻止对象被改变。
|
|
||||||
|
|
||||||
```
|
```
|
||||||
'use strict';
|
'use strict';
|
||||||
|
|
||||||
// CASE 1: The object is mutable and the variable can be reassigned.对象的属性是可变的,并且变量可以被再次赋值。
|
// CASE 1: 对象的属性是可变的,并且变量可以被再次赋值。
|
||||||
let o1 = { foo: 'bar' };
|
let o1 = { foo: 'bar' };
|
||||||
|
|
||||||
// Mutate the object改变对象的属性
|
// 改变对象的属性
|
||||||
o1.foo = 'something different';
|
o1.foo = 'something different';
|
||||||
|
|
||||||
// Reassign the variable对变量再次赋值
|
// 对变量再次赋值
|
||||||
o1 = { message: "I'm a completely new object" };
|
o1 = { message: "I'm a completely new object" };
|
||||||
|
|
||||||
|
|
||||||
// CASE 2: The object is still mutable but the variable cannot be reassigned.对象的属性还是可变的,但是变量不能被再次赋值。
|
// CASE 2: 对象的属性还是可变的,但是变量不能被再次赋值。
|
||||||
const o2 = { foo: 'baz' };
|
const o2 = { foo: 'baz' };
|
||||||
|
|
||||||
// Can still mutate the object
|
// 仍然能改变对象
|
||||||
o2.foo = 'Something different, yet again';
|
o2.foo = 'Something different, yet again';
|
||||||
|
|
||||||
// Cannot reassign the variable不能对变量再次赋值
|
// 不能对变量再次赋值
|
||||||
// o2 = { message: 'I will cause an error if you uncomment me' }; // Error!
|
// o2 = { message: 'I will cause an error if you uncomment me' }; // Error!
|
||||||
|
|
||||||
|
|
||||||
// CASE 3: The object is immutable but the variable can be reassigned.对象的属性是不可变的,但是变量可以被再次赋值。
|
// CASE 3: 对象的属性是不可变的,但是变量可以被再次赋值。
|
||||||
let o3 = Object.freeze({ foo: "Can't mutate me" });
|
let o3 = Object.freeze({ foo: "Can't mutate me" });
|
||||||
|
|
||||||
// Cannot mutate the object不能改变对象的属性
|
// 不能改变对象的属性
|
||||||
// o3.foo = 'Come on, uncomment me. I dare ya!'; // Error!
|
// o3.foo = 'Come on, uncomment me. I dare ya!'; // Error!
|
||||||
|
|
||||||
// Can still reassign the variable还是可以对变量再次赋值
|
// 还是可以对变量再次赋值
|
||||||
o3 = { message: "I'm some other object, and I'm even mutable -- so take that!" };
|
o3 = { message: "I'm some other object, and I'm even mutable -- so take that!" };
|
||||||
|
|
||||||
|
|
||||||
// CASE 4: The object is immutable and the variable cannot be reassigned. This is what we want!!!!!!!!对象的属性是不可变的,并且变量不能被再次赋值。这是我们想要的!!!!!!!!
|
// CASE 4: 对象的属性是不可变的,并且变量不能被再次赋值。这是我们想要的!!!!!!!!
|
||||||
const o4 = Object.freeze({ foo: 'never going to change me' });
|
const o4 = Object.freeze({ foo: 'never going to change me' });
|
||||||
|
|
||||||
// Cannot mutate the object不能改变对象的属性
|
// 不能改变对象的属性
|
||||||
// o4.foo = 'talk to the hand' // Error!
|
// o4.foo = 'talk to the hand' // Error!
|
||||||
|
|
||||||
// Cannot reassign the variable不能对变量再次赋值
|
// 不能对变量再次赋值
|
||||||
// o4 = { message: "ain't gonna happen, sorry" }; // Error
|
// o4 = { message: "ain't gonna happen, sorry" }; // Error
|
||||||
```
|
```
|
||||||
|
|
||||||
|
|
||||||
[view raw][20][immutability-vs-reassignment.js][21] hosted with ❤ by [GitHub][22]
|
不变性适用于所有的数据结构,包括数组、映射和集合。它意味着不能调用例如 `Array.prototype.push` 等会导致本身改变的方法,因为它会改变已经存在的数组。可以通过创建一个含有原来元素和新加元素的新数组,而不是将新元素加入一个已经存在的数组。其实所有会导致数组本身被修改的方法都可以通过一个返回修改好的新数组的函数代替。
|
||||||
|
|
||||||
不变性适用于所有的数据结构,包括数组,映射和集合。它意味着不能调用例如 **Array.prototype.push** 等会导致本身改变的方法,因为它会改变已经存在的数组。可以通过创建一个含有原来元素和新加元素的新数组,代替将新元素加入一个已经存在的数组。其实所有会导致数组本身被修改的方法都可以通过一个返回修改好的新数组的函数代替。**这里一段mutator的翻译大概有些啰嗦。**
|
|
||||||
|
|
||||||
```
|
```
|
||||||
'use strict';
|
'use strict';
|
||||||
@ -251,16 +211,13 @@ const f = R.sort(myCompareFunction, a); // R = Ramda
|
|||||||
// Instead of: a.reverse();
|
// Instead of: a.reverse();
|
||||||
const g = R.reverse(a); // R = Ramda
|
const g = R.reverse(a); // R = Ramda
|
||||||
|
|
||||||
// Exercise for the reader留给读者的练习:
|
// 留给读者的练习:
|
||||||
// copyWithin
|
// copyWithin
|
||||||
// fill
|
// fill
|
||||||
// splice
|
// splice
|
||||||
```
|
```
|
||||||
|
|
||||||
|
[映射][84] 和 [集合][85] 也很相似。可以通过返回一个新的修改好的映射或者集合来代替使用会修改其本身的函数。
|
||||||
[view raw][23][array-mutator-method-replacement.js][24] hosted with ❤ by [GitHub][25]
|
|
||||||
|
|
||||||
[**Map**][84] 和 [**Set**][85] 也很相似。可以通过返回一个新的修改好的 Map 或者 Set 来代替使用会修改其本身的函数。
|
|
||||||
|
|
||||||
```
|
```
|
||||||
const map = new Map([
|
const map = new Map([
|
||||||
@ -279,9 +236,6 @@ const map3 = new Map([...map].filter(([key]) => key !== 1));
|
|||||||
const map4 = new Map();
|
const map4 = new Map();
|
||||||
```
|
```
|
||||||
|
|
||||||
|
|
||||||
[view raw][26][map-mutator-method-replacement.js][27] hosted with ❤ by [GitHub][28]
|
|
||||||
|
|
||||||
```
|
```
|
||||||
const set = new Set(['A', 'B', 'C']);
|
const set = new Set(['A', 'B', 'C']);
|
||||||
|
|
||||||
@ -295,19 +249,15 @@ const set3 = new Set([...set].filter(key => key !== 'B'));
|
|||||||
const set4 = new Set();
|
const set4 = new Set();
|
||||||
```
|
```
|
||||||
|
|
||||||
|
我想提一句如果你在使用 TypeScript(我非常喜欢 TypeScript),你可以用 `Readonly<T>`、`ReadonlyArray<T>`、`ReadonlyMap<K, V>` 和 `ReadonlySet<T>` 接口来在编译期检查你是否尝试更改这些对象,有则抛出编译错误。如果在对一个对象字面量或者数组调用 `Object.freeze`,编译器会自动推断它是只读的。由于映射和集合在其内部表达,所以在这些数据结构上调用 `Object.freeze` 不起作用。但是你可以轻松地告诉编译器它们是只读的变量。
|
||||||
|
|
||||||
[view raw][29][set-mutator-method-replacement.js][30] hosted with ❤ by [GitHub][31]
|
|
||||||
|
|
||||||
我想提一句如果你在使用 TypeScript(我非常喜欢TypeScript),你可以用 **Readonly<T>**, **ReadonlyArray<T>**, **ReadonlyMap<K, V>**, 和 **ReadonlySet<T>** 接口来在编译期检查你是否尝试更改这些对象,有则抛出编译错误。如果在对一个对象字面量或者数组调用 **Object.freeze**,编译器会自动推断它是只读的。由于映射和集合在其内部表达,所以在这些数据结构上调用 **Object.freeze** 不起作用。但是你可以轻松地告诉编译器它们是只读的变量。
|
|
||||||
|
|
||||||
### [typescript-readonly.png][32]
|
|
||||||
|
|
||||||

|

|
||||||
|
|
||||||
|
|
||||||
TypeScript read-only interfaces
|
*TypeScript 只读接口*
|
||||||
|
|
||||||
好,所以我们可以通过创建新的对象来代替修改原来的对象,但是这样不会导致性能损失吗?当然会。确保在你自己的应用中做了性能测试。如果你需要提高性能,可以考虑使用 [Immutable.js][86]。Immutable.js 用[持久的数据结构][91] 实现了[链表][87],,[堆栈][88], [映射][89],[集合][90]和其他数据结构。使用了和比如 Clojure 和 Scala 的函数式语言中相同的技术。
|
好,所以我们可以通过创建新的对象来代替修改原来的对象,但是这样不会导致性能损失吗?当然会。确保在你自己的应用中做了性能测试。如果你需要提高性能,可以考虑使用 [Immutable.js][86]。Immutable.js 用[持久的数据结构][91] 实现了[链表][87]、[堆栈][88]、[映射][89]、[集合][90]和其他数据结构。使用了如同 Clojure 和 Scala 这样的函数式语言中相同的技术。
|
||||||
|
|
||||||
```
|
```
|
||||||
// Use in place of `[]`.
|
// Use in place of `[]`.
|
||||||
@ -338,17 +288,14 @@ console.log([...set1]); // [1, 2, 3, 4]
|
|||||||
console.log([...set2]); // [1, 2, 3, 4, 5]
|
console.log([...set2]); // [1, 2, 3, 4, 5]
|
||||||
```
|
```
|
||||||
|
|
||||||
[view raw][33][immutable-js-demo.js][34] hosted with ❤ by [GitHub][35]
|
### 函数组合
|
||||||
|
|
||||||
### 函数组合(不确定是这个叫法
|
记不记得在中学时我们学过一些像 `(f ∘ g)(x)` 的东西?你那时可能想,“我什么时候会用到这些?”,好了,现在就用到了。你准备好了吗?`f ∘ g`读作 “函数 f 和函数 g 组合”。对它的理解有两种等价的方式,如等式所示: `(f ∘ g)(x) = f(g(x))`。你可以认为 `f ∘ g` 是一个单独的函数,或者视作将调用函数 `g` 的结果作为参数传给函数 `f`。注意这些函数是从右向左依次调用的,先执行 `g`,接下来执行 `f`。
|
||||||
|
|
||||||
好,你准备好听解答了吗?**f ∘ g **读作 **“函数 f 和函数 g 组合”(不确定 没搜到中文资料**。它的定义是 **(f ∘ g)(x) = f(g(x))**,你也可以认为 **f ∘ g** 是一个单独的函数,它将调用函数 **g** 的结果作为参数传给函数 **f**。注意这些函数是从右向左以此调用的,先执行 **g**,接下来执行 **f**。
|
|
||||||
|
|
||||||
关于函数组合的几个要点:
|
关于函数组合的几个要点:
|
||||||
|
|
||||||
1. 我们可以组合任意数量的函数(不仅限于 2 个)。
|
1. 我们可以组合任意数量的函数(不仅限于 2 个)。
|
||||||
|
2. 组合函数的一个方式是简单地把一个函数的输出作为下一个函数的输入(比如 `f(g(x))`)。
|
||||||
2. 组合函数的一个方式是简单地把一个函数的输出作为下一个函数的输入。(比如 **f(g(x))**))
|
|
||||||
|
|
||||||
```
|
```
|
||||||
// h(x) = x + 1
|
// h(x) = x + 1
|
||||||
@ -374,9 +321,7 @@ const y = f(g(h(1)));
|
|||||||
console.log(y); // '4'
|
console.log(y); // '4'
|
||||||
```
|
```
|
||||||
|
|
||||||
[view raw][36][function-composition-basic.js][37] hosted with ❤ by [GitHub][38]
|
[Ramda][92] 和 [lodash][93] 之类的库提供了更优雅的方式来组合函数。我们可以在更多的在数学意义上处理函数组合,而不是简单地将一个函数的返回值传递给下一个函数。我们可以创建一个由这些函数组成的单一复合函数(就是 `(f ∘ g)(x)`)。
|
||||||
|
|
||||||
[Ramda][92] 和 [lodash][93] 之类的库提供了更优雅的方式来组合函数。我们可以在更多的在数学意义上处理函数组合,而不是简单地将一个函数的返回值传递给下一个函数。我们可以创建一个由这些函数组成的单一复合函数(就是,**(f ∘ g)(x)**)。
|
|
||||||
|
|
||||||
```
|
```
|
||||||
// h(x) = x + 1
|
// h(x) = x + 1
|
||||||
@ -406,17 +351,15 @@ const y = composite(1);
|
|||||||
console.log(y); // '4'
|
console.log(y); // '4'
|
||||||
```
|
```
|
||||||
|
|
||||||
[view raw][39][function-composition-elegant.js][40] hosted with ❤ by [GitHub][41]
|
好了,我们可以在 JavaScript 中组合函数了。接下来呢?好,如果你已经入门了函数式编程,理想中你的程序将只有函数的组合。代码里没有循环(`for`, `for...of`, `for...in`, `while`, `do`),基本没有。你可能觉得那是不可能的。并不是这样。我们下面的两个话题是:递归和高阶函数。
|
||||||
|
|
||||||
我们可以在 JavaScript 中组合函数。接下来呢?好,如果你已经入门了函数式编程,理想中你的程序将只有函数的组合。代码里没有循环(**for**, **for...of**, **for...in**, **while**, **do**),基本没有。你说但是那不可能。并不是这样。我们下面的两个话题是:递归和高阶函数。
|
|
||||||
|
|
||||||
### 递归
|
### 递归
|
||||||
|
|
||||||
假设你想实现一个计算数字的阶乘的函数。 让我们回顾一下数学中阶乘的定义:
|
假设你想实现一个计算数字的阶乘的函数。 让我们回顾一下数学中阶乘的定义:
|
||||||
|
|
||||||
**n! = n * (n-1) * (n-2) * ... * 1**.
|
`n! = n * (n-1) * (n-2) * ... * 1`.
|
||||||
|
|
||||||
**n!** 是从 **n** 到 **1** 的所有整数的乘积。我们可以编写一个循环轻松地计算出结果。
|
`n!` 是从 `n` 到 `1` 的所有整数的乘积。我们可以编写一个循环轻松地计算出结果。
|
||||||
|
|
||||||
```
|
```
|
||||||
function iterativeFactorial(n) {
|
function iterativeFactorial(n) {
|
||||||
@ -428,16 +371,13 @@ function iterativeFactorial(n) {
|
|||||||
}
|
}
|
||||||
```
|
```
|
||||||
|
|
||||||
|
注意 `product` 和 `i` 都在循环中被反复重新赋值。这是解决这个问题的标准过程式方法。如何用函数式的方法解决这个问题呢?我们需要消除循环,确保没有变量被重新赋值。递归是函数式程序员的最有力的工具之一。递归需要我们将整体问题分解为类似整体问题的子问题。
|
||||||
|
|
||||||
[view raw][42][iterative-factorial.js][43] hosted with ❤ by [GitHub][44]
|
计算阶乘是一个很好的例子,为了计算 `n!` 我们需要将 n 乘以所有比它小的正整数。它的意思就相当于:
|
||||||
|
|
||||||
注意 **product** 和 **i** 都在循环中被反复重新赋值。这是解决这个问题的标准过程式方法。如何用函数式的方法解决这个问题呢?我们需要消除循环,确保没有变量被重新赋值。递归是函数式程序员的最有力的工具之一。递归需要我们将整体问题分解为类似整体问题的子问题。
|
`n! = n * (n-1)!`
|
||||||
|
|
||||||
计算阶乘是一个很好的例子,为了计算 **n!** 我们需要将 n 乘以所有比它小的正整数。它的意思就相当于:
|
啊哈!我们发现了一个解决 `(n-1)!` 的子问题,它类似于整个问题 `n!`。还有一个需要注意的地方就是基础条件。基础条件告诉我们何时停止递归。 如果我们没有基础条件,那么递归将永远持续。 实际上,如果有太多的递归调用,程序会抛出一个堆栈溢出错误。啊哈!
|
||||||
|
|
||||||
**n! = n * (n-1)!**
|
|
||||||
|
|
||||||
A-ha!我们发现了一个类似于整个问题 **n!** 的子问题来解决 **(n-1)!**。还有一个需要注意的地方就是基础条件。基础条件告诉我们何时停止递归。 如果我们没有基础条件,那么递归将永远持续。 实际上,如果有太多的递归调用,程序会抛出一个堆栈溢出错误。A-HA!
|
|
||||||
|
|
||||||
```
|
```
|
||||||
function recursiveFactorial(n) {
|
function recursiveFactorial(n) {
|
||||||
@ -449,28 +389,21 @@ function recursiveFactorial(n) {
|
|||||||
}
|
}
|
||||||
```
|
```
|
||||||
|
|
||||||
|
然后我们来计算 `recursiveFactorial(20000)` 因为……,为什么不呢?当我们这样做的时候,我们得到了这个结果:
|
||||||
[view raw][45][recursive-factorial.js][46] hosted with ❤ by [GitHub][47]
|
|
||||||
|
|
||||||
然后我们来计算 **recursiveFactorial(20000)** 因为...,为什么不呢?当我们这样做的时候,我们得到了这个结果:
|
|
||||||
|
|
||||||
### [stack-overflow.png][48]
|
|
||||||
|
|
||||||

|

|
||||||
|
|
||||||
堆栈溢出错误
|
*堆栈溢出错误*
|
||||||
|
|
||||||
这里发生了什么?我们得到一个堆栈溢出错误!这不是无穷的递归导致的。我们已经处理了基础条件(**n === 0** 的情况)。那是因为浏览器的堆栈大小是有限的,而我们的代码使用了越过了这个大小的堆栈。每次对 **recursiveFactorial** 的调用导致了新的帧被压入堆栈中,就像一个盒子压在另一个盒子上。每当 **recursiveFactorial** 被调用,一个新的盒子被放在最上面。下图展示了在计算 **recursiveFactorial(3)** 时堆栈的样子。注意在真实的堆栈中,堆栈顶部的帧将存储在执行完成后应该返回的内存地址,但是我选择用变量 **r** 来表示返回值,因为 JavaScript 开发者一般不需要考虑内存地址。
|
这里发生了什么?我们得到一个堆栈溢出错误!这不是无穷的递归导致的。我们已经处理了基础条件(`n === 0` 的情况)。那是因为浏览器的堆栈大小是有限的,而我们的代码使用了越过了这个大小的堆栈。每次对 `recursiveFactorial` 的调用导致了新的帧被压入堆栈中,就像一个盒子压在另一个盒子上。每当 `recursiveFactorial` 被调用,一个新的盒子被放在最上面。下图展示了在计算 `recursiveFactorial(3)` 时堆栈的样子。注意在真实的堆栈中,堆栈顶部的帧将存储在执行完成后应该返回的内存地址,但是我选择用变量 `r` 来表示返回值,因为 JavaScript 开发者一般不需要考虑内存地址。
|
||||||
|
|
||||||
### [stack-frames.png][49]
|
|
||||||
|
|
||||||
")
|
")
|
||||||
|
|
||||||
递归计算 3! 的堆栈(三次乘法)
|
*递归计算 3! 的堆栈(三次乘法)*
|
||||||
|
|
||||||
你可能会想象当计算 **n = 20000** 时堆栈会更高。我们可以做些什么优化它吗?当然可以。作为 **ES2015** (又名 **ES6**) 标准的一部分,有一个优化用来解决这个问题。它被称作_尾调用优化_。它使得浏览器删除或者忽略堆栈帧,当递归函数做的最后一件事是调用自己并返回结果的时候。实际上,这个优化对于相互递归函数也是有效的,但是为了简单起见,我们还是来看单递归函数。
|
你可能会想象当计算 `n = 20000` 时堆栈会更高。我们可以做些什么优化它吗?当然可以。作为 ES2015 (又名 ES6) 标准的一部分,有一个优化用来解决这个问题。它被称作<ruby>尾调用优化<rt>proper tail calls optimization</rt></ruby>(PTC)。当递归函数做的最后一件事是调用自己并返回结果的时候,它使得浏览器删除或者忽略堆栈帧。实际上,这个优化对于相互递归函数也是有效的,但是为了简单起见,我们还是来看单一递归函数。
|
||||||
|
|
||||||
你可能会注意到,在递归函数调用之后,还要进行一次额外的计算(**n * r**)。那意味着浏览器不能通过 PTC 来优化递归;然而,我们可以通过重写函数使最后一步变成递归调用以便优化。一个窍门是将中间结果(在这里是**乘积**)作为参数传递给函数。
|
你可能会注意到,在递归函数调用之后,还要进行一次额外的计算(`n * r`)。那意味着浏览器不能通过 PTC 来优化递归;然而,我们可以通过重写函数使最后一步变成递归调用以便优化。一个窍门是将中间结果(在这里是 `product`)作为参数传递给函数。
|
||||||
|
|
||||||
```
|
```
|
||||||
'use strict';
|
'use strict';
|
||||||
@ -484,30 +417,23 @@ function factorial(n, product = 1) {
|
|||||||
}
|
}
|
||||||
```
|
```
|
||||||
|
|
||||||
|
让我们来看看优化后的计算 `factorial(3)` 时的堆栈。如下图所示,堆栈不会增长到超过两层。原因是我们把必要的信息都传到了递归函数中(比如 `product`)。所以,在 `product` 被更新后,浏览器可以丢弃掉堆栈中原先的帧。你可以在图中看到每次最上面的帧下沉变成了底部的帧,原先底部的帧被丢弃,因为不再需要它了。
|
||||||
[view raw][50][factorial-tail-recursion.js][51] hosted with ❤ by [GitHub][52]
|
|
||||||
|
|
||||||
让我们来看看优化后的计算 **factorial(3)** 时的堆栈。如下图所示,堆栈不会增长到超过两层。原因是我们把必要的信息都传到了递归函数中(比如**乘积**)。所以,在**乘积**被更新后,浏览器可以丢弃掉堆栈中原先的帧。你可以在图中看到每次最上面的帧下沉变成了底部的帧,原先底部的帧被丢弃,因为不再需要它了。
|
|
||||||
|
|
||||||
### [optimized-stack-frames.png][53]
|
|
||||||
|
|
||||||
 using PTC")
|
 using PTC")
|
||||||
|
|
||||||
递归计算 3! 的堆栈(三次乘法)使用 PTC
|
*递归计算 3! 的堆栈(三次乘法)使用 PTC*
|
||||||
|
|
||||||
现在选一个浏览器运行吧,假设你在使用 Safari,你会得到**无穷大**(它是比在 JavaScript 中能表达的最大值更大的数)。但是我们没有得到堆栈溢出错误,那很不错!现在在其他的浏览器中呢怎么样呢?Safari 可能现在乃至将来是实现 PTC 的唯一一个浏览器。看看下面的兼容性表格:
|
现在选一个浏览器运行吧,假设你在使用 Safari,你会得到 `Infinity`(它是比在 JavaScript 中能表达的最大值更大的数)。但是我们没有得到堆栈溢出错误,那很不错!现在在其他的浏览器中呢怎么样呢?Safari 可能现在乃至将来是实现 PTC 的唯一一个浏览器。看看下面的兼容性表格:
|
||||||
|
|
||||||
### [ptc-compatibility.png][54]
|
|
||||||
|
|
||||||

|

|
||||||
|
|
||||||
PTC 兼容性
|
*PTC 兼容性*
|
||||||
|
|
||||||
其他浏览器提出了一种被称作[语法级尾调用][95](STC)的竞争标准。"Syntactic"意味着你需要用新的语法来标识你想要执行尾递归优化的函数。,即使浏览器还没有广泛支持,但是把你的递归函数写成支持尾递归优化的样子还是一个好主意。
|
其他浏览器提出了一种被称作<ruby>[语法级尾调用][95]<rt>syntactic tail calls</rt></ruby>(STC)的竞争标准。“语法级”意味着你需要用新的语法来标识你想要执行尾递归优化的函数。即使浏览器还没有广泛支持,但是把你的递归函数写成支持尾递归优化的样子还是一个好主意。
|
||||||
|
|
||||||
### 高阶函数
|
### 高阶函数
|
||||||
|
|
||||||
我们已经知道 JavaScript 将函数视作一等公民,可以把函数像其他值一样传递。所以,把一个函数传给另一个函数也很常见。我们也可以让函数返回一个函数。就是它!我们有高阶函数。你可能已经很熟悉几个在 **Array.prototype** 中的高阶函数。比如 **filter**,**map**,和 **reduce** 就在其中。对高阶函数的一种理解是:它是接受(一般会调用)一个回调函数参数的函数。让我们来看看一些内置的高阶函数的例子:
|
我们已经知道 JavaScript 将函数视作一等公民,可以把函数像其他值一样传递。所以,把一个函数传给另一个函数也很常见。我们也可以让函数返回一个函数。就是它!我们有高阶函数。你可能已经很熟悉几个在 `Array.prototype` 中的高阶函数。比如 `filter`、`map` 和 `reduce` 就在其中。对高阶函数的一种理解是:它是接受(一般会调用)一个回调函数参数的函数。让我们来看看一些内置的高阶函数的例子:
|
||||||
|
|
||||||
```
|
```
|
||||||
const vehicles = [
|
const vehicles = [
|
||||||
@ -531,10 +457,7 @@ const averageSUVPrice = vehicles
|
|||||||
console.log(averageSUVPrice); // 33399
|
console.log(averageSUVPrice); // 33399
|
||||||
```
|
```
|
||||||
|
|
||||||
|
注意我们在一个数组对象上调用其方法,这是面向对象编程的特性。如果我们想要更函数式一些,我们可以用 Rmmda 或者 lodash/fp 提供的函数。注意如果我们使用 `R.compose` 的话,需要倒转函数的顺序,因为它从右向左依次调用函数(从底向上);然而,如果我们想从左向右调用函数就像上面的例子,我们可以用 `R.pipe`。下面两个例子用了 Rmmda。注意 Rmmda 有一个 `mean` 函数用来代替 `reduce` 。
|
||||||
[view raw][55][built-in-higher-order-functions.js][56] hosted with ❤ by [GitHub][57]
|
|
||||||
|
|
||||||
注意我们在一个数组对象上调用其方法,这是面向对象编程的特性。如果我们想要更函数式一些,我们可以用 Rmmda 或者 lodash/fp 提供的函数。注意如果我们使用 **R.compose** 的话,需要倒转函数的顺序,因为它从右向左依次调用函数(从底向上);然而,如果我们想从左向右调用函数就像上面的例子,我们可以用 **R.pipe**。下面两个例子用了 Rmmda。注意 Rmmda 有一个 **mean** 函数用来代替 **reduce** 。
|
|
||||||
|
|
||||||
```
|
```
|
||||||
const vehicles = [
|
const vehicles = [
|
||||||
@ -569,14 +492,11 @@ const averageSUVPrice2 = R.compose(
|
|||||||
console.log(averageSUVPrice2); // 33399
|
console.log(averageSUVPrice2); // 33399
|
||||||
```
|
```
|
||||||
|
|
||||||
|
使用函数式方法的优点是清楚地分开了数据(`vehicles`)和逻辑(函数 `filter`,`map` 和 `reduce`)。面向对象的代码相比之下把数据和函数用以方法的对象的形式混合在了一起。
|
||||||
[view raw][58][composing-higher-order-functions.js][59] hosted with ❤ by [GitHub][60]
|
|
||||||
|
|
||||||
使用函数式方法的优点是清楚地分开了数据(**车辆**)和逻辑(函数 **filter**,**map** 和 **reduce**)面向对象的代码相比之下把数据和函数用以方法的对象的形式混合在了一起。
|
|
||||||
|
|
||||||
### 柯里化
|
### 柯里化
|
||||||
|
|
||||||
不规范地说,柯里化是把一个接受 **n** 个参数的函数变成 **n** 个每个接受单个参数的函数的过程。函数的 **arity** 是它接受参数的个数。接受一个参数的函数是 **unary**,两个的是 **binary**,三个的是 **ternary**,**n**个的是 **n-ary**。那么,我们可以把柯里化定义成将一个 **n-ary** 函数转换成 **n** 个 unary 函数的过程。让我们通过简单的例子开始,一个计算两个向量点积的函数。回忆一下线性代数,两个向量 **[a, b, c]** 和 **[x, y, z]** 的点积是 **ax + by + cz**。
|
不规范地说,<ruby>柯里化<rt>currying</rt></ruby>是把一个接受 `n` 个参数的函数变成 `n` 个每个接受单个参数的函数的过程。函数的 `arity` 是它接受参数的个数。接受一个参数的函数是 `unary`,两个的是 `binary`,三个的是 `ternary`,`n` 个的是 `n-ary`。那么,我们可以把柯里化定义成将一个 `n-ary` 函数转换成 `n` 个 `unary` 函数的过程。让我们通过简单的例子开始,一个计算两个向量点积的函数。回忆一下线性代数,两个向量 `[a, b, c]` 和 `[x, y, z]` 的点积是 `ax + by + cz`。
|
||||||
|
|
||||||
```
|
```
|
||||||
function dot(vector1, vector2) {
|
function dot(vector1, vector2) {
|
||||||
@ -589,10 +509,7 @@ const v2 = [4, -2, -1];
|
|||||||
console.log(dot(v1, v2)); // 1(4) + 3(-2) + (-5)(-1) = 4 - 6 + 5 = 3
|
console.log(dot(v1, v2)); // 1(4) + 3(-2) + (-5)(-1) = 4 - 6 + 5 = 3
|
||||||
```
|
```
|
||||||
|
|
||||||
|
`dot` 函数是 binary,因为它接受两个参数;然而我们可以将它手动转换成两个 unary 函数,就像下面的例子。注意 `curriedDot` 是一个 unary 函数,它接受一个向量并返回另一个接受第二个向量的 unary 函数。
|
||||||
[view raw][61][dot-product.js][62] hosted with ❤ by [GitHub][63]
|
|
||||||
|
|
||||||
**dot** 函数是 binary 因为它接受两个参数;然而我们可以将它手动转换成两个 unary 函数,就像下面的例子。注意 **curriedDot** 是一个 unary 函数,它接受一个向量并返回另一个接受第二个向量的 unary 函数。
|
|
||||||
|
|
||||||
```
|
```
|
||||||
function curriedDot(vector1) {
|
function curriedDot(vector1) {
|
||||||
@ -609,9 +526,6 @@ console.log(sumElements([1, 3, -5])); // -1
|
|||||||
console.log(sumElements([4, -2, -1])); // 1
|
console.log(sumElements([4, -2, -1])); // 1
|
||||||
```
|
```
|
||||||
|
|
||||||
|
|
||||||
[view raw][64][manual-currying.js][65] hosted with ❤ by [GitHub][66]
|
|
||||||
|
|
||||||
很幸运,我们不需要把每一个函数都手动转换成柯里化以后的形式。[Ramda][96] 和 [lodash][97] 等库可以为我们做这些工作。实际上,它们是柯里化的混合形式。你既可以每次传递一个参数,也可以像原来一样一次传递所有参数。
|
很幸运,我们不需要把每一个函数都手动转换成柯里化以后的形式。[Ramda][96] 和 [lodash][97] 等库可以为我们做这些工作。实际上,它们是柯里化的混合形式。你既可以每次传递一个参数,也可以像原来一样一次传递所有参数。
|
||||||
|
|
||||||
```
|
```
|
||||||
@ -634,8 +548,6 @@ console.log(sumElements(v2)); // 1
|
|||||||
console.log(curriedDot(v1, v2)); // 3
|
console.log(curriedDot(v1, v2)); // 3
|
||||||
```
|
```
|
||||||
|
|
||||||
[view raw][67][fancy-currying.js][68] hosted with ❤ by [GitHub][69]
|
|
||||||
|
|
||||||
Ramda 和 lodash 都允许你“跳过”一些变量之后再指定它们。它们使用置位符来做这些工作。因为点积的计算可以交换两项。传入向量的顺序不影响结果。让我们换一个例子来阐述如何使用一个置位符。Ramda 使用双下划线作为其置位符。
|
Ramda 和 lodash 都允许你“跳过”一些变量之后再指定它们。它们使用置位符来做这些工作。因为点积的计算可以交换两项。传入向量的顺序不影响结果。让我们换一个例子来阐述如何使用一个置位符。Ramda 使用双下划线作为其置位符。
|
||||||
|
|
||||||
```
|
```
|
||||||
@ -657,11 +569,9 @@ console.log(result);
|
|||||||
// 3: French Hens
|
// 3: French Hens
|
||||||
```
|
```
|
||||||
|
|
||||||
[view raw][70][currying-placeholder.js][71] hosted with ❤ by [GitHub][72]
|
在我们结束探讨柯里化之前最后的议题是<ruby>偏函数应用<rt>partial application</rt></ruby>。偏函数应用和柯里化经常同时出场,尽管它们实际上是不同的概念。一个柯里化的函数还是柯里化的函数,即使没有给它任何参数。偏函数应用,另一方面是仅仅给一个函数传递部分参数而不是所有参数。柯里化是偏函数应用常用的方法之一,但是不是唯一的。
|
||||||
|
|
||||||
在我们结束探讨柯里化之前最后的议题是偏函数应用。偏函数应用和柯里化经常同时出场,尽管它们实际上是不同的概念。一个柯里化的函数还是柯里化的函数,即使没有给它任何参数。偏函数应用,另一方面是仅仅给一个函数传递部分而不是所有参数。柯里化是偏函数应用常用的方法之一,但是不是唯一的。
|
JavaScript 拥有一个内置机制可以不依靠柯里化来做偏函数应用。那就是 [function.prototype.bind][98] 方法。这个方法的一个特殊之处在于,它要求你将 `this` 作为第一个参数传入。 如果你不进行面向对象编程,那么你可以通过传入 `null` 来忽略 `this`。
|
||||||
|
|
||||||
JavaScript 拥有一个内置机制可以不依靠柯里化来偏函数应用。那就是 [**function.prototype.bind**][98] 方法。这个方法的一个特殊之处在于,它要求你将 **this** 作为第一个参数传入。 如果你不进行面向对象编程,那么你可以通过传入 **null** 来忽略 **this**。
|
|
||||||
|
|
||||||
```
|
```
|
||||||
1function giveMe3(item1, item2, item3) {
|
1function giveMe3(item1, item2, item3) {
|
||||||
@ -682,30 +592,25 @@ console.log(result);
|
|||||||
// 3: scissors
|
// 3: scissors
|
||||||
```
|
```
|
||||||
|
|
||||||
|
|
||||||
[view raw][73][partial-application-using-bind.js][74] hosted with ❤ by [GitHub][75]
|
|
||||||
|
|
||||||
### 总结
|
### 总结
|
||||||
|
|
||||||
我希望你享受探索 JavaScript 中函数式编程的过程。对一些人来说,它可能是一个全新的编程范式,但我希望你能尝试它。你会发现你的程序更易于阅读和调试。不变性还将允许你优化 Angular 和 React 的性能。
|
我希望你享受探索 JavaScript 中函数式编程的过程。对一些人来说,它可能是一个全新的编程范式,但我希望你能尝试它。你会发现你的程序更易于阅读和调试。不变性还将允许你优化 Angular 和 React 的性能。
|
||||||
|
|
||||||
_这篇文章基于 Matt 在 OpenWest 的演讲 [JavaScript the Good-er Parts][77]. [OpenWest][78] ~~将~~在 6/12-15 ,2017 在 Salt Lake City, Utah 举行。_
|
_这篇文章基于 Matt 在 OpenWest 的演讲 [JavaScript the Good-er Parts][77]. [OpenWest][78] ~~将~~在 6/12-15 ,2017 在 Salt Lake City, Utah 举行。_
|
||||||
|
|
||||||
--------------------------------------------------------------------------------
|
--------------------------------------------------------------------------------
|
||||||
|
|
||||||
作者简介:
|
作者简介:
|
||||||
|
|
||||||
Matt Banz - Matt graduated from the University of Utah with a degree in mathematics in May of 2008. One month later he landed a job as a web developer, and he's been loving it ever since! In 2013, he earned a Master of Computer Science degree from North Carolina State University. He has taught courses in web development for LDS Business College and the Davis School District Community Education program. He is currently a Senior Front-End Developer for Motorola Solutions.
|
|
||||||
|
|
||||||
Matt Banz - Matt 于 2008 年五月在犹他大学获得了数学学位毕业。一个月后他得到了一份 web 开发者的工作,他从那时起就爱上了它!在 2013 年,他在北卡罗莱纳州立大学获得了计算机科学硕士学位。他在 LDS 商学院和戴维斯学区社区教育计划教授 Web 课程。他现在是就职于 Motorola Solutions 公司的高级前端开发者。
|
Matt Banz - Matt 于 2008 年五月在犹他大学获得了数学学位毕业。一个月后他得到了一份 web 开发者的工作,他从那时起就爱上了它!在 2013 年,他在北卡罗莱纳州立大学获得了计算机科学硕士学位。他在 LDS 商学院和戴维斯学区社区教育计划教授 Web 课程。他现在是就职于 Motorola Solutions 公司的高级前端开发者。
|
||||||
|
|
||||||
--------------
|
--------------
|
||||||
|
|
||||||
via: https://opensource.com/article/17/6/functional-javascript
|
via: https://opensource.com/article/17/6/functional-javascript
|
||||||
|
|
||||||
作者:[Matt Banz ][a]
|
作者:[Matt Banz][a]
|
||||||
译者:[trnhoe](https://github.com/trnhoe)
|
译者:[trnhoe](https://github.com/trnhoe)
|
||||||
校对:[校对者ID](https://github.com/校对者ID)
|
校对:[wxy](https://github.com/wxy)
|
||||||
|
|
||||||
本文由 [LCTT](https://github.com/LCTT/TranslateProject) 原创编译,[Linux中国](https://linux.cn/) 荣誉推出
|
本文由 [LCTT](https://github.com/LCTT/TranslateProject) 原创编译,[Linux中国](https://linux.cn/) 荣誉推出
|
||||||
|
|
@ -1,4 +1,4 @@
|
|||||||
[并发服务器: 第一节 —— 简介][18]
|
并发服务器(一):简介
|
||||||
============================================================
|
============================================================
|
||||||
|
|
||||||
这是关于并发网络服务器编程的第一篇教程。我计划测试几个主流的、可以同时处理多个客户端请求的服务器并发模型,基于可扩展性和易实现性对这些模型进行评判。所有的服务器都会监听套接字连接,并且实现一些简单的协议用于与客户端进行通讯。
|
这是关于并发网络服务器编程的第一篇教程。我计划测试几个主流的、可以同时处理多个客户端请求的服务器并发模型,基于可扩展性和易实现性对这些模型进行评判。所有的服务器都会监听套接字连接,并且实现一些简单的协议用于与客户端进行通讯。
|
||||||
@ -6,34 +6,32 @@
|
|||||||
该系列的所有文章:
|
该系列的所有文章:
|
||||||
|
|
||||||
* [第一节 - 简介][7]
|
* [第一节 - 简介][7]
|
||||||
|
|
||||||
* [第二节 - 线程][8]
|
* [第二节 - 线程][8]
|
||||||
|
|
||||||
* [第三节 - 事件驱动][9]
|
* [第三节 - 事件驱动][9]
|
||||||
|
|
||||||
### 协议
|
### 协议
|
||||||
|
|
||||||
该系列教程所用的协议都非常简单,但足以展示并发服务器设计的许多有趣层面。而且这个协议是 _有状态的_ —— 服务器根据客户端发送的数据改变内部状态,然后根据内部状态产生相应的行为。并非所有的协议都是有状态的 —— 实际上,基于 HTTP 的许多协议是无状态的,但是有状态的协议对于保证重要会议的可靠很常见。
|
该系列教程所用的协议都非常简单,但足以展示并发服务器设计的许多有趣层面。而且这个协议是 _有状态的_ —— 服务器根据客户端发送的数据改变内部状态,然后根据内部状态产生相应的行为。并非所有的协议都是有状态的 —— 实际上,基于 HTTP 的许多协议是无状态的,但是有状态的协议也是很常见,值得认真讨论。
|
||||||
|
|
||||||
在服务器端看来,这个协议的视图是这样的:
|
在服务器端看来,这个协议的视图是这样的:
|
||||||
|
|
||||||
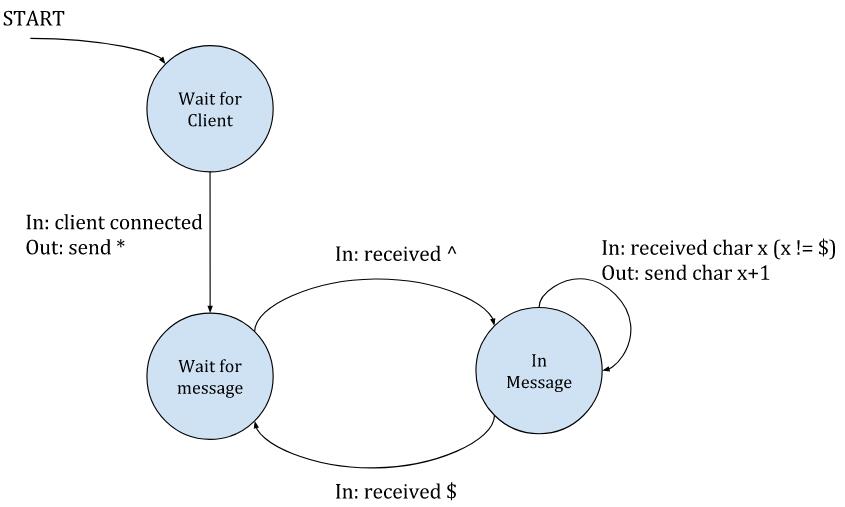
|
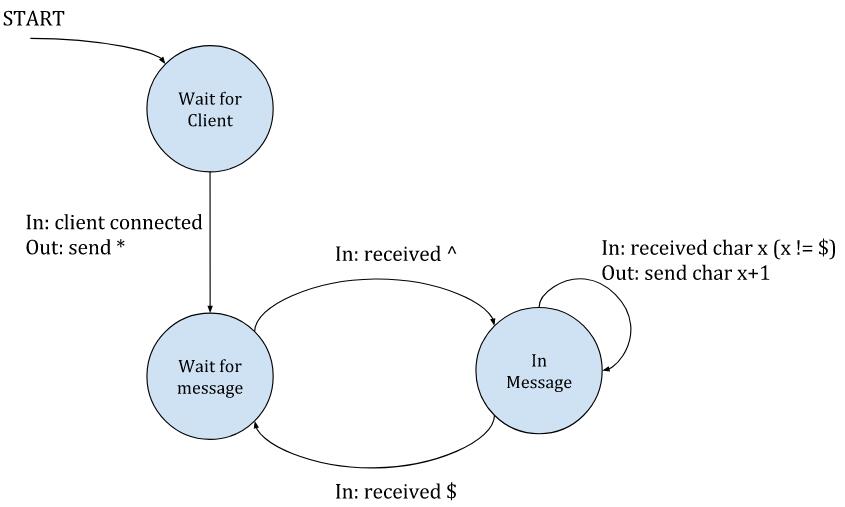
|
||||||
|
|
||||||
总之:服务器等待新客户端的连接;当一个客户端连接的时候,服务器会向该客户端发送一个 `*` 字符,进入“等待消息”的状态。在该状态下,服务器会忽略客户端发送的所有字符,除非它看到了一个 `^` 字符,这表示一个新消息的开始。这个时候服务器就会转变为“正在通信”的状态,这时它会向客户端回送数据,把收到的所有字符的每个字节加 1 回送给客户端 [ [1][10] ]。当客户端发送了 `$`字符,服务器就会退回到等待新消息的状态。`^` 和 `$` 字符仅仅用于分隔消息 —— 它们不会被服务器回送。
|
总之:服务器等待新客户端的连接;当一个客户端连接的时候,服务器会向该客户端发送一个 `*` 字符,进入“等待消息”的状态。在该状态下,服务器会忽略客户端发送的所有字符,除非它看到了一个 `^` 字符,这表示一个新消息的开始。这个时候服务器就会转变为“正在通信”的状态,这时它会向客户端回送数据,把收到的所有字符的每个字节加 1 回送给客户端^注1 。当客户端发送了 `$` 字符,服务器就会退回到等待新消息的状态。`^` 和 `$` 字符仅仅用于分隔消息 —— 它们不会被服务器回送。
|
||||||
|
|
||||||
每个状态之后都有个隐藏的箭头指向 “等待客户端” 状态,用来防止客户端断开连接。因此,客户端要表示“我已经结束”的方法很简单,关掉它那一端的连接就好。
|
每个状态之后都有个隐藏的箭头指向 “等待客户端” 状态,用来客户端断开连接。因此,客户端要表示“我已经结束”的方法很简单,关掉它那一端的连接就好。
|
||||||
|
|
||||||
显然,这个协议是真实协议的简化版,真实使用的协议一般包含复杂的报文头,转义字符序列(例如让消息体中可以出现 `$` 符号),额外的状态变化。但是我们这个协议足以完成期望。
|
显然,这个协议是真实协议的简化版,真实使用的协议一般包含复杂的报文头、转义字符序列(例如让消息体中可以出现 `$` 符号),额外的状态变化。但是我们这个协议足以完成期望。
|
||||||
|
|
||||||
另一点:这个系列是引导性的,并假设客户端都工作的很好(虽然可能运行很慢);因此没有设置超时,也没有设置特殊的规则来确保服务器不会因为客户端的恶意行为(或是故障)而出现阻塞,导致不能正常结束。
|
另一点:这个系列是介绍性的,并假设客户端都工作的很好(虽然可能运行很慢);因此没有设置超时,也没有设置特殊的规则来确保服务器不会因为客户端的恶意行为(或是故障)而出现阻塞,导致不能正常结束。
|
||||||
|
|
||||||
### 有序服务器
|
### 顺序服务器
|
||||||
|
|
||||||
这个系列中我们的第一个服务端程序是一个简单的“有序”服务器,用 C 进行编写,除了标准的 POSIX 中用于套接字的内容以外没有使用其它库。服务器程序是有序的,因为它一次只能处理一个客户端的请求;当有客户端连接时,像之前所说的那样,服务器会进入到状态机中,并且不再监听套接字接受新的客户端连接,直到当前的客户端结束连接。显然这不是并发的,而且即便在很少的负载下也不能服务多个客户端,但它对于我们的讨论很有用,因为我们需要的是一个易于理解的基础。
|
这个系列中我们的第一个服务端程序是一个简单的“顺序”服务器,用 C 进行编写,除了标准的 POSIX 中用于套接字的内容以外没有使用其它库。服务器程序是顺序,因为它一次只能处理一个客户端的请求;当有客户端连接时,像之前所说的那样,服务器会进入到状态机中,并且不再监听套接字接受新的客户端连接,直到当前的客户端结束连接。显然这不是并发的,而且即便在很少的负载下也不能服务多个客户端,但它对于我们的讨论很有用,因为我们需要的是一个易于理解的基础。
|
||||||
|
|
||||||
这个服务器的完整代码在 [这里][11];接下来,我会着重于高亮的部分。`main` 函数里面的外层循环用于监听套接字,以便接受新客户端的连接。一旦有客户端进行连接,就会调用 `serve_connection`,这个函数中的代码会一直运行,直到客户端断开连接。
|
这个服务器的完整代码在[这里][11];接下来,我会着重于一些重点的部分。`main` 函数里面的外层循环用于监听套接字,以便接受新客户端的连接。一旦有客户端进行连接,就会调用 `serve_connection`,这个函数中的代码会一直运行,直到客户端断开连接。
|
||||||
|
|
||||||
有序服务器在循环里调用 `accept` 用来监听套接字,并接受新连接:
|
顺序服务器在循环里调用 `accept` 用来监听套接字,并接受新连接:
|
||||||
|
|
||||||
```
|
```
|
||||||
while (1) {
|
while (1) {
|
||||||
@ -105,25 +103,23 @@ void serve_connection(int sockfd) {
|
|||||||
|
|
||||||
它完全是按照状态机协议进行编写的。每次循环的时候,服务器尝试接收客户端的数据。收到 0 字节意味着客户端断开连接,然后循环就会退出。否则,会逐字节检查接收缓存,每一个字节都可能会触发一个状态。
|
它完全是按照状态机协议进行编写的。每次循环的时候,服务器尝试接收客户端的数据。收到 0 字节意味着客户端断开连接,然后循环就会退出。否则,会逐字节检查接收缓存,每一个字节都可能会触发一个状态。
|
||||||
|
|
||||||
`recv` 函数返回接收到的字节数与客户端发送消息的数量完全无关(`^...$` 闭合序列的字节)。因此,在保持状态的循环中,遍历整个缓冲区很重要。而且,每一个接收到的缓冲中可能包含多条信息,但也有可能开始了一个新消息,却没有显式的结束字符;而这个结束字符可能在下一个缓冲中才能收到,这就是处理状态在循环迭代中进行维护的原因。
|
`recv` 函数返回接收到的字节数与客户端发送消息的数量完全无关(`^...$` 闭合序列的字节)。因此,在保持状态的循环中遍历整个缓冲区很重要。而且,每一个接收到的缓冲中可能包含多条信息,但也有可能开始了一个新消息,却没有显式的结束字符;而这个结束字符可能在下一个缓冲中才能收到,这就是处理状态在循环迭代中进行维护的原因。
|
||||||
|
|
||||||
例如,试想主循环中的 `recv` 函数在某次连接中返回了三个非空的缓冲:
|
例如,试想主循环中的 `recv` 函数在某次连接中返回了三个非空的缓冲:
|
||||||
|
|
||||||
1. `^abc$de^abte$f`
|
1. `^abc$de^abte$f`
|
||||||
|
|
||||||
2. `xyz^123`
|
2. `xyz^123`
|
||||||
|
|
||||||
3. `25$^ab$abab`
|
3. `25$^ab$abab`
|
||||||
|
|
||||||
服务端返回的是哪些数据?追踪代码对于理解状态转变很有用。(答案见 [ [2][12] ])
|
服务端返回的是哪些数据?追踪代码对于理解状态转变很有用。(答案见^注[2] )
|
||||||
|
|
||||||
### 多个并发客户端
|
### 多个并发客户端
|
||||||
|
|
||||||
如果多个客户端在同一时刻向有序服务器发起连接会发生什么事情?
|
如果多个客户端在同一时刻向顺序服务器发起连接会发生什么事情?
|
||||||
|
|
||||||
服务器端的代码(以及它的名字 `有序的服务器`)已经说的很清楚了,一次只能处理 _一个_ 客户端的请求。只要服务器在 `serve_connection` 函数中忙于处理客户端的请求,就不会接受别的客户端的连接。只有当前的客户端断开了连接,`serve_connection` 才会返回,然后最外层的循环才能继续执行接受其他客户端的连接。
|
服务器端的代码(以及它的名字 “顺序服务器”)已经说的很清楚了,一次只能处理 _一个_ 客户端的请求。只要服务器在 `serve_connection` 函数中忙于处理客户端的请求,就不会接受别的客户端的连接。只有当前的客户端断开了连接,`serve_connection` 才会返回,然后最外层的循环才能继续执行接受其他客户端的连接。
|
||||||
|
|
||||||
为了演示这个行为,[该系列教程的示例代码][13] 包含了一个 Python 脚本,用于模拟几个想要同时连接服务器的客户端。每一个客户端发送类似之前那样的三个数据缓冲 [ [3][14] ],不过每次发送数据之间会有一定延迟。
|
为了演示这个行为,[该系列教程的示例代码][13] 包含了一个 Python 脚本,用于模拟几个想要同时连接服务器的客户端。每一个客户端发送类似之前那样的三个数据缓冲 ^注3 ,不过每次发送数据之间会有一定延迟。
|
||||||
|
|
||||||
客户端脚本在不同的线程中并发地模拟客户端行为。这是我们的序列化服务器与客户端交互的信息记录:
|
客户端脚本在不同的线程中并发地模拟客户端行为。这是我们的序列化服务器与客户端交互的信息记录:
|
||||||
|
|
||||||
@ -158,30 +154,26 @@ INFO:2017-09-16 14:14:24,176:conn0 received b'36bc1111'
|
|||||||
INFO:2017-09-16 14:14:24,376:conn0 disconnecting
|
INFO:2017-09-16 14:14:24,376:conn0 disconnecting
|
||||||
```
|
```
|
||||||
|
|
||||||
这里要注意连接名:`conn1` 是第一个连接到服务器的,先跟服务器交互了一段时间。接下来的连接 `conn2` —— 在第一个断开连接后,连接到了服务器,然后第三个连接也是一样。就像日志显示的那样,每一个连接让服务器变得繁忙,保持大约 2.2 秒的时间(这实际上是人为地在客户端代码中加入的延迟),在这段时间里别的客户端都不能连接。
|
这里要注意连接名:`conn1` 是第一个连接到服务器的,先跟服务器交互了一段时间。接下来的连接 `conn2` —— 在第一个断开连接后,连接到了服务器,然后第三个连接也是一样。就像日志显示的那样,每一个连接让服务器变得繁忙,持续了大约 2.2 秒的时间(这实际上是人为地在客户端代码中加入的延迟),在这段时间里别的客户端都不能连接。
|
||||||
|
|
||||||
显然,这不是一个可扩展的策略。这个例子中,客户端中加入了延迟,让服务器不能处理别的交互动作。一个智能服务器应该能处理一堆客户端的请求,而这个原始的服务器在结束连接之前一直繁忙(我们将会在之后的章节中看到如何实现智能的服务器)。尽管服务端有延迟,但这不会过度占用CPU;例如,从数据库中查找信息(时间基本上是花在连接到数据库服务器上,或者是花在硬盘中的本地数据库)。
|
显然,这不是一个可扩展的策略。这个例子中,客户端中加入了延迟,让服务器不能处理别的交互动作。一个智能服务器应该能处理一堆客户端的请求,而这个原始的服务器在结束连接之前一直繁忙(我们将会在之后的章节中看到如何实现智能的服务器)。尽管服务端有延迟,但这不会过度占用 CPU;例如,从数据库中查找信息(时间基本上是花在连接到数据库服务器上,或者是花在硬盘中的本地数据库)。
|
||||||
|
|
||||||
### 总结及期望
|
### 总结及期望
|
||||||
|
|
||||||
这个示例服务器达成了两个预期目标:
|
这个示例服务器达成了两个预期目标:
|
||||||
|
|
||||||
1. 首先是介绍了问题范畴和贯彻该系列文章的套接字编程基础。
|
1. 首先是介绍了问题范畴和贯彻该系列文章的套接字编程基础。
|
||||||
|
2. 对于并发服务器编程的抛砖引玉 —— 就像之前的部分所说,顺序服务器还不能在非常轻微的负载下进行扩展,而且没有高效的利用资源。
|
||||||
|
|
||||||
2. 对于并发服务器编程的抛砖引玉 —— 就像之前的部分所说,有序服务器还不能在几个轻微的负载下进行扩展,而且没有高效的利用资源。
|
在看下一篇文章前,确保你已经理解了这里所讲的服务器/客户端协议,还有顺序服务器的代码。我之前介绍过了这个简单的协议;例如 [串行通信分帧][15] 和 [用协程来替代状态机][16]。要学习套接字网络编程的基础,[Beej 的教程][17] 用来入门很不错,但是要深入理解我推荐你还是看本书。
|
||||||
|
|
||||||
在看下一篇文章前,确保你已经理解了这里所讲的服务器/客户端协议,还有有序服务器的代码。我之前介绍过了这个简单的协议;例如 [串行通信分帧][15] 和 [协同运行,作为状态机的替代][16]。要学习套接字网络编程的基础,[Beej's 教程][17] 用来入门很不错,但是要深入理解我推荐你还是看本书。
|
|
||||||
|
|
||||||
如果有什么不清楚的,请在评论区下进行评论或者向我发送邮件。深入理解并发服务器!
|
如果有什么不清楚的,请在评论区下进行评论或者向我发送邮件。深入理解并发服务器!
|
||||||
|
|
||||||
***
|
***
|
||||||
|
|
||||||
|
- 注1:状态转变中的 In/Out 记号是指 [Mealy machine][2]。
|
||||||
[ [1][1] ] 状态转变中的 In/Out 记号是指 [Mealy machine][2]。
|
- 注2:回应的是 `bcdbcuf23436bc`。
|
||||||
|
- 注3:这里在结尾处有一点小区别,加了字符串 `0000` —— 服务器回应这个序列,告诉客户端让其断开连接;这是一个简单的握手协议,确保客户端有足够的时间接收到服务器发送的所有回复。
|
||||||
[ [2][3] ] 回应的是 `bcdbcuf23436bc`。
|
|
||||||
|
|
||||||
[ [3][4] ] 这里在结尾处有一点小区别,加了字符串 `0000` —— 服务器回应这个序列,告诉客户端让其断开连接;这是一个简单的握手协议,确保客户端有足够的时间接收到服务器发送的所有回复。
|
|
||||||
|
|
||||||
--------------------------------------------------------------------------------
|
--------------------------------------------------------------------------------
|
||||||
|
|
||||||
@ -189,7 +181,7 @@ via: https://eli.thegreenplace.net/2017/concurrent-servers-part-1-introduction/
|
|||||||
|
|
||||||
作者:[Eli Bendersky][a]
|
作者:[Eli Bendersky][a]
|
||||||
译者:[GitFuture](https://github.com/GitFuture)
|
译者:[GitFuture](https://github.com/GitFuture)
|
||||||
校对:[校对者ID](https://github.com/校对者ID)
|
校对:[wxy](https://github.com/wxy)
|
||||||
|
|
||||||
本文由 [LCTT](https://github.com/LCTT/TranslateProject) 原创编译,[Linux中国](https://linux.cn/) 荣誉推出
|
本文由 [LCTT](https://github.com/LCTT/TranslateProject) 原创编译,[Linux中国](https://linux.cn/) 荣誉推出
|
||||||
|
|
294
published/20171004 Concurrent Servers Part 2 - Threads.md
Normal file
294
published/20171004 Concurrent Servers Part 2 - Threads.md
Normal file
@ -0,0 +1,294 @@
|
|||||||
|
并发服务器(二):线程
|
||||||
|
============================================================
|
||||||
|
|
||||||
|
这是并发网络服务器系列的第二节。[第一节][20] 提出了服务端实现的协议,还有简单的顺序服务器的代码,是这整个系列的基础。
|
||||||
|
|
||||||
|
这一节里,我们来看看怎么用多线程来实现并发,用 C 实现一个最简单的多线程服务器,和用 Python 实现的线程池。
|
||||||
|
|
||||||
|
该系列的所有文章:
|
||||||
|
|
||||||
|
* [第一节 - 简介][8]
|
||||||
|
* [第二节 - 线程][9]
|
||||||
|
* [第三节 - 事件驱动][10]
|
||||||
|
|
||||||
|
### 多线程的方法设计并发服务器
|
||||||
|
|
||||||
|
说起第一节里的顺序服务器的性能,最显而易见的,是在服务器处理客户端连接时,计算机的很多资源都被浪费掉了。尽管假定客户端快速发送完消息,不做任何等待,仍然需要考虑网络通信的开销;网络要比现在的 CPU 慢上百万倍还不止,因此 CPU 运行服务器时会等待接收套接字的流量,而大量的时间都花在完全不必要的等待中。
|
||||||
|
|
||||||
|
这里是一份示意图,表明顺序时客户端的运行过程:
|
||||||
|
|
||||||
|
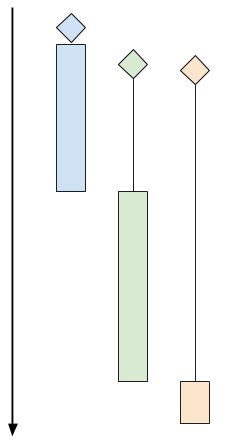
|
||||||
|
|
||||||
|
这个图片上有 3 个客户端程序。棱形表示客户端的“到达时间”(即客户端尝试连接服务器的时间)。黑色线条表示“等待时间”(客户端等待服务器真正接受连接所用的时间),有色矩形表示“处理时间”(服务器和客户端使用协议进行交互所用的时间)。有色矩形的末端表示客户端断开连接。
|
||||||
|
|
||||||
|
上图中,绿色和橘色的客户端尽管紧跟在蓝色客户端之后到达服务器,也要等到服务器处理完蓝色客户端的请求。这时绿色客户端得到响应,橘色的还要等待一段时间。
|
||||||
|
|
||||||
|
多线程服务器会开启多个控制线程,让操作系统管理 CPU 的并发(使用多个 CPU 核心)。当客户端连接的时候,创建一个线程与之交互,而在主线程中,服务器能够接受其他的客户端连接。下图是该模式的时间轴:
|
||||||
|
|
||||||
|
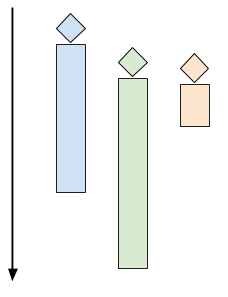
|
||||||
|
|
||||||
|
### 每个客户端一个线程,在 C 语言里要用 pthread
|
||||||
|
|
||||||
|
这篇文章的 [第一个示例代码][11] 是一个简单的 “每个客户端一个线程” 的服务器,用 C 语言编写,使用了 [phtreads API][12] 用于实现多线程。这里是主循环代码:
|
||||||
|
|
||||||
|
```
|
||||||
|
while (1) {
|
||||||
|
struct sockaddr_in peer_addr;
|
||||||
|
socklen_t peer_addr_len = sizeof(peer_addr);
|
||||||
|
|
||||||
|
int newsockfd =
|
||||||
|
accept(sockfd, (struct sockaddr*)&peer_addr, &peer_addr_len);
|
||||||
|
|
||||||
|
if (newsockfd < 0) {
|
||||||
|
perror_die("ERROR on accept");
|
||||||
|
}
|
||||||
|
|
||||||
|
report_peer_connected(&peer_addr, peer_addr_len);
|
||||||
|
pthread_t the_thread;
|
||||||
|
|
||||||
|
thread_config_t* config = (thread_config_t*)malloc(sizeof(*config));
|
||||||
|
if (!config) {
|
||||||
|
die("OOM");
|
||||||
|
}
|
||||||
|
config->sockfd = newsockfd;
|
||||||
|
pthread_create(&the_thread, NULL, server_thread, config);
|
||||||
|
|
||||||
|
// 回收线程 —— 在线程结束的时候,它占用的资源会被回收
|
||||||
|
// 因为主线程在一直运行,所以它比服务线程存活更久。
|
||||||
|
pthread_detach(the_thread);
|
||||||
|
}
|
||||||
|
```
|
||||||
|
|
||||||
|
这是 `server_thread` 函数:
|
||||||
|
|
||||||
|
```
|
||||||
|
void* server_thread(void* arg) {
|
||||||
|
thread_config_t* config = (thread_config_t*)arg;
|
||||||
|
int sockfd = config->sockfd;
|
||||||
|
free(config);
|
||||||
|
|
||||||
|
// This cast will work for Linux, but in general casting pthread_id to an 这个类型转换在 Linux 中可以正常运行,但是一般来说将 pthread_id 类型转换成整形不便于移植代码
|
||||||
|
// integral type isn't portable.
|
||||||
|
unsigned long id = (unsigned long)pthread_self();
|
||||||
|
printf("Thread %lu created to handle connection with socket %d\n", id,
|
||||||
|
sockfd);
|
||||||
|
serve_connection(sockfd);
|
||||||
|
printf("Thread %lu done\n", id);
|
||||||
|
return 0;
|
||||||
|
}
|
||||||
|
```
|
||||||
|
|
||||||
|
线程 “configuration” 是作为 `thread_config_t` 结构体进行传递的:
|
||||||
|
|
||||||
|
```
|
||||||
|
typedef struct { int sockfd; } thread_config_t;
|
||||||
|
```
|
||||||
|
|
||||||
|
主循环中调用的 `pthread_create` 产生一个新线程,然后运行 `server_thread` 函数。这个线程会在 `server_thread` 返回的时候结束。而在 `serve_connection` 返回的时候 `server_thread` 才会返回。`serve_connection` 和第一节完全一样。
|
||||||
|
|
||||||
|
第一节中我们用脚本生成了多个并发访问的客户端,观察服务器是怎么处理的。现在来看看多线程服务器的处理结果:
|
||||||
|
|
||||||
|
```
|
||||||
|
$ python3.6 simple-client.py -n 3 localhost 9090
|
||||||
|
INFO:2017-09-20 06:31:56,632:conn1 connected...
|
||||||
|
INFO:2017-09-20 06:31:56,632:conn2 connected...
|
||||||
|
INFO:2017-09-20 06:31:56,632:conn0 connected...
|
||||||
|
INFO:2017-09-20 06:31:56,632:conn1 sending b'^abc$de^abte$f'
|
||||||
|
INFO:2017-09-20 06:31:56,632:conn2 sending b'^abc$de^abte$f'
|
||||||
|
INFO:2017-09-20 06:31:56,632:conn0 sending b'^abc$de^abte$f'
|
||||||
|
INFO:2017-09-20 06:31:56,633:conn1 received b'b'
|
||||||
|
INFO:2017-09-20 06:31:56,633:conn2 received b'b'
|
||||||
|
INFO:2017-09-20 06:31:56,633:conn0 received b'b'
|
||||||
|
INFO:2017-09-20 06:31:56,670:conn1 received b'cdbcuf'
|
||||||
|
INFO:2017-09-20 06:31:56,671:conn0 received b'cdbcuf'
|
||||||
|
INFO:2017-09-20 06:31:56,671:conn2 received b'cdbcuf'
|
||||||
|
INFO:2017-09-20 06:31:57,634:conn1 sending b'xyz^123'
|
||||||
|
INFO:2017-09-20 06:31:57,634:conn2 sending b'xyz^123'
|
||||||
|
INFO:2017-09-20 06:31:57,634:conn1 received b'234'
|
||||||
|
INFO:2017-09-20 06:31:57,634:conn0 sending b'xyz^123'
|
||||||
|
INFO:2017-09-20 06:31:57,634:conn2 received b'234'
|
||||||
|
INFO:2017-09-20 06:31:57,634:conn0 received b'234'
|
||||||
|
INFO:2017-09-20 06:31:58,635:conn1 sending b'25$^ab0000$abab'
|
||||||
|
INFO:2017-09-20 06:31:58,635:conn2 sending b'25$^ab0000$abab'
|
||||||
|
INFO:2017-09-20 06:31:58,636:conn1 received b'36bc1111'
|
||||||
|
INFO:2017-09-20 06:31:58,636:conn2 received b'36bc1111'
|
||||||
|
INFO:2017-09-20 06:31:58,637:conn0 sending b'25$^ab0000$abab'
|
||||||
|
INFO:2017-09-20 06:31:58,637:conn0 received b'36bc1111'
|
||||||
|
INFO:2017-09-20 06:31:58,836:conn2 disconnecting
|
||||||
|
INFO:2017-09-20 06:31:58,836:conn1 disconnecting
|
||||||
|
INFO:2017-09-20 06:31:58,837:conn0 disconnecting
|
||||||
|
```
|
||||||
|
|
||||||
|
实际上,所有客户端同时连接,它们与服务器的通信是同时发生的。
|
||||||
|
|
||||||
|
### 每个客户端一个线程的难点
|
||||||
|
|
||||||
|
尽管在现代操作系统中就资源利用率方面来看,线程相当的高效,但前一节中讲到的方法在高负载时却会出现纰漏。
|
||||||
|
|
||||||
|
想象一下这样的情景:很多客户端同时进行连接,某些会话持续的时间长。这意味着某个时刻服务器上有很多活跃的线程。太多的线程会消耗掉大量的内存和 CPU 资源,而仅仅是用于上下文切换^注1 。另外其也可视为安全问题:因为这样的设计容易让服务器成为 [DoS 攻击][14] 的目标 —— 上百万个客户端同时连接,并且客户端都处于闲置状态,这样耗尽了所有资源就可能让服务器宕机。
|
||||||
|
|
||||||
|
当服务器要与每个客户端通信,CPU 进行大量计算时,就会出现更严重的问题。这种情况下,容易想到的方法是减少服务器的响应能力 —— 只有其中一些客户端能得到服务器的响应。
|
||||||
|
|
||||||
|
因此,对多线程服务器所能够处理的并发客户端数做一些 _速率限制_ 就是个明智的选择。有很多方法可以实现。最容易想到的是计数当前已经连接上的客户端,把连接数限制在某个范围内(需要通过仔细的测试后决定)。另一种流行的多线程应用设计是使用 _线程池_。
|
||||||
|
|
||||||
|
### 线程池
|
||||||
|
|
||||||
|
[线程池][15] 很简单,也很有用。服务器创建几个任务线程,这些线程从某些队列中获取任务。这就是“池”。然后每一个客户端的连接被当成任务分发到池中。只要池中有空闲的线程,它就会去处理任务。如果当前池中所有线程都是繁忙状态,那么服务器就会阻塞,直到线程池可以接受任务(某个繁忙状态的线程处理完当前任务后,变回空闲的状态)。
|
||||||
|
|
||||||
|
这里有个 4 线程的线程池处理任务的图。任务(这里就是客户端的连接)要等到线程池中的某个线程可以接受新任务。
|
||||||
|
|
||||||
|
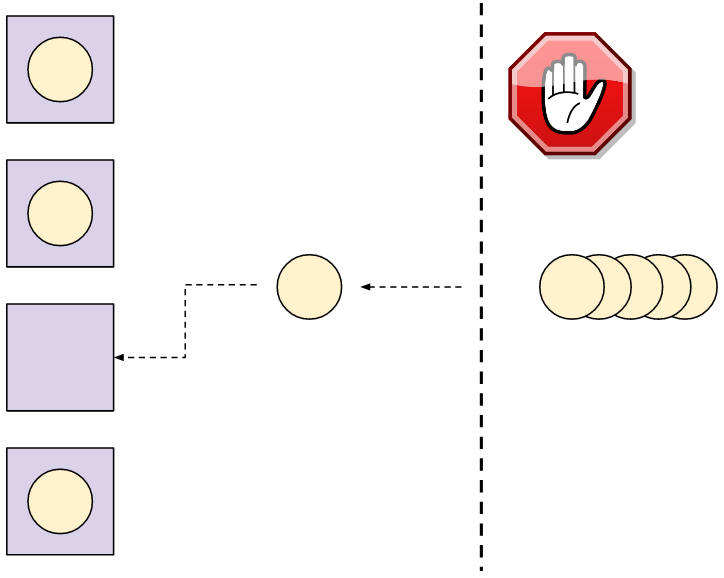
|
||||||
|
|
||||||
|
非常明显,线程池的定义就是一种按比例限制的机制。我们可以提前设定服务器所能拥有的线程数。那么这就是并发连接的最多的客户端数 —— 其它的客户端就要等到线程空闲。如果我们的池中有 8 个线程,那么 8 就是服务器可以处理的最多的客户端并发连接数,哪怕上千个客户端想要同时连接。
|
||||||
|
|
||||||
|
那么怎么确定池中需要有多少个线程呢?通过对问题范畴进行细致的分析、评估、实验以及根据我们拥有的硬件配置。如果是单核的云服务器,答案只有一个;如果是 100 核心的多套接字的服务器,那么答案就有很多种。也可以在运行时根据负载动态选择池的大小 —— 我会在这个系列之后的文章中谈到这个东西。
|
||||||
|
|
||||||
|
使用线程池的服务器在高负载情况下表现出 _性能退化_ —— 客户端能够以稳定的速率进行连接,可能会比其它时刻得到响应的用时稍微久一点;也就是说,无论多少个客户端同时进行连接,服务器总能保持响应,尽最大能力响应等待的客户端。与之相反,每个客户端一个线程的服务器,会接收多个客户端的连接直到过载,这时它更容易崩溃或者因为要处理_所有_客户端而变得缓慢,因为资源都被耗尽了(比如虚拟内存的占用)。
|
||||||
|
|
||||||
|
### 在服务器上使用线程池
|
||||||
|
|
||||||
|
为了[改变服务器的实现][16],我用了 Python,在 Python 的标准库中带有一个已经实现好的稳定的线程池。(`concurrent.futures` 模块里的 `ThreadPoolExecutor`) ^注2 。
|
||||||
|
|
||||||
|
服务器创建一个线程池,然后进入循环,监听套接字接收客户端的连接。用 `submit` 把每一个连接的客户端分配到池中:
|
||||||
|
|
||||||
|
```
|
||||||
|
pool = ThreadPoolExecutor(args.n)
|
||||||
|
sockobj = socket.socket(socket.AF_INET, socket.SOCK_STREAM)
|
||||||
|
sockobj.setsockopt(socket.SOL_SOCKET, socket.SO_REUSEADDR, 1)
|
||||||
|
sockobj.bind(('localhost', args.port))
|
||||||
|
sockobj.listen(15)
|
||||||
|
|
||||||
|
try:
|
||||||
|
while True:
|
||||||
|
client_socket, client_address = sockobj.accept()
|
||||||
|
pool.submit(serve_connection, client_socket, client_address)
|
||||||
|
except KeyboardInterrupt as e:
|
||||||
|
print(e)
|
||||||
|
sockobj.close()
|
||||||
|
```
|
||||||
|
|
||||||
|
`serve_connection` 函数和 C 的那部分很像,与一个客户端交互,直到其断开连接,并且遵循我们的协议:
|
||||||
|
|
||||||
|
```
|
||||||
|
ProcessingState = Enum('ProcessingState', 'WAIT_FOR_MSG IN_MSG')
|
||||||
|
|
||||||
|
def serve_connection(sockobj, client_address):
|
||||||
|
print('{0} connected'.format(client_address))
|
||||||
|
sockobj.sendall(b'*')
|
||||||
|
state = ProcessingState.WAIT_FOR_MSG
|
||||||
|
|
||||||
|
while True:
|
||||||
|
try:
|
||||||
|
buf = sockobj.recv(1024)
|
||||||
|
if not buf:
|
||||||
|
break
|
||||||
|
except IOError as e:
|
||||||
|
break
|
||||||
|
for b in buf:
|
||||||
|
if state == ProcessingState.WAIT_FOR_MSG:
|
||||||
|
if b == ord(b'^'):
|
||||||
|
state = ProcessingState.IN_MSG
|
||||||
|
elif state == ProcessingState.IN_MSG:
|
||||||
|
if b == ord(b'$'):
|
||||||
|
state = ProcessingState.WAIT_FOR_MSG
|
||||||
|
else:
|
||||||
|
sockobj.send(bytes([b + 1]))
|
||||||
|
else:
|
||||||
|
assert False
|
||||||
|
|
||||||
|
print('{0} done'.format(client_address))
|
||||||
|
sys.stdout.flush()
|
||||||
|
sockobj.close()
|
||||||
|
```
|
||||||
|
|
||||||
|
来看看线程池的大小对并行访问的客户端的阻塞行为有什么样的影响。为了演示,我会运行一个池大小为 2 的线程池服务器(只生成两个线程用于响应客户端)。
|
||||||
|
|
||||||
|
```
|
||||||
|
$ python3.6 threadpool-server.py -n 2
|
||||||
|
```
|
||||||
|
|
||||||
|
在另外一个终端里,运行客户端模拟器,产生 3 个并发访问的客户端:
|
||||||
|
|
||||||
|
```
|
||||||
|
$ python3.6 simple-client.py -n 3 localhost 9090
|
||||||
|
INFO:2017-09-22 05:58:52,815:conn1 connected...
|
||||||
|
INFO:2017-09-22 05:58:52,827:conn0 connected...
|
||||||
|
INFO:2017-09-22 05:58:52,828:conn1 sending b'^abc$de^abte$f'
|
||||||
|
INFO:2017-09-22 05:58:52,828:conn0 sending b'^abc$de^abte$f'
|
||||||
|
INFO:2017-09-22 05:58:52,828:conn1 received b'b'
|
||||||
|
INFO:2017-09-22 05:58:52,828:conn0 received b'b'
|
||||||
|
INFO:2017-09-22 05:58:52,867:conn1 received b'cdbcuf'
|
||||||
|
INFO:2017-09-22 05:58:52,867:conn0 received b'cdbcuf'
|
||||||
|
INFO:2017-09-22 05:58:53,829:conn1 sending b'xyz^123'
|
||||||
|
INFO:2017-09-22 05:58:53,829:conn0 sending b'xyz^123'
|
||||||
|
INFO:2017-09-22 05:58:53,830:conn1 received b'234'
|
||||||
|
INFO:2017-09-22 05:58:53,831:conn0 received b'2'
|
||||||
|
INFO:2017-09-22 05:58:53,831:conn0 received b'34'
|
||||||
|
INFO:2017-09-22 05:58:54,831:conn1 sending b'25$^ab0000$abab'
|
||||||
|
INFO:2017-09-22 05:58:54,832:conn1 received b'36bc1111'
|
||||||
|
INFO:2017-09-22 05:58:54,832:conn0 sending b'25$^ab0000$abab'
|
||||||
|
INFO:2017-09-22 05:58:54,833:conn0 received b'36bc1111'
|
||||||
|
INFO:2017-09-22 05:58:55,032:conn1 disconnecting
|
||||||
|
INFO:2017-09-22 05:58:55,032:conn2 connected...
|
||||||
|
INFO:2017-09-22 05:58:55,033:conn2 sending b'^abc$de^abte$f'
|
||||||
|
INFO:2017-09-22 05:58:55,033:conn0 disconnecting
|
||||||
|
INFO:2017-09-22 05:58:55,034:conn2 received b'b'
|
||||||
|
INFO:2017-09-22 05:58:55,071:conn2 received b'cdbcuf'
|
||||||
|
INFO:2017-09-22 05:58:56,036:conn2 sending b'xyz^123'
|
||||||
|
INFO:2017-09-22 05:58:56,036:conn2 received b'234'
|
||||||
|
INFO:2017-09-22 05:58:57,037:conn2 sending b'25$^ab0000$abab'
|
||||||
|
INFO:2017-09-22 05:58:57,038:conn2 received b'36bc1111'
|
||||||
|
INFO:2017-09-22 05:58:57,238:conn2 disconnecting
|
||||||
|
```
|
||||||
|
|
||||||
|
回顾之前讨论的服务器行为:
|
||||||
|
|
||||||
|
1. 在顺序服务器中,所有的连接都是串行的。一个连接结束后,下一个连接才能开始。
|
||||||
|
2. 前面讲到的每个客户端一个线程的服务器中,所有连接都被同时接受并得到服务。
|
||||||
|
|
||||||
|
这里可以看到一种可能的情况:两个连接同时得到服务,只有其中一个结束连接后第三个才能连接上。这就是把线程池大小设置成 2 的结果。真实用例中我们会把线程池设置的更大些,取决于机器和实际的协议。线程池的缓冲机制就能很好理解了 —— 我 [几个月前][18] 更详细的介绍过这种机制,关于 Clojure 的 `core.async` 模块。
|
||||||
|
|
||||||
|
### 总结与展望
|
||||||
|
|
||||||
|
这篇文章讨论了在服务器中,用多线程作并发的方法。每个客户端一个线程的方法最早提出来,但是实际上却不常用,因为它并不安全。
|
||||||
|
|
||||||
|
线程池就常见多了,最受欢迎的几个编程语言有良好的实现(某些编程语言,像 Python,就是在标准库中实现)。这里说的使用线程池的服务器,不会受到每个客户端一个线程的弊端。
|
||||||
|
|
||||||
|
然而,线程不是处理多个客户端并行访问的唯一方法。下一节中我们会看看其它的解决方案,可以使用_异步处理_,或者_事件驱动_的编程。
|
||||||
|
|
||||||
|
* * *
|
||||||
|
|
||||||
|
- 注1:老实说,现代 Linux 内核可以承受足够多的并发线程 —— 只要这些线程主要在 I/O 上被阻塞。[这里有个示例程序][2],它产生可配置数量的线程,线程在循环体中是休眠的,每 50 ms 唤醒一次。我在 4 核的 Linux 机器上可以轻松的产生 10000 个线程;哪怕这些线程大多数时间都在睡眠,它们仍然消耗一到两个核心,以便实现上下文切换。而且,它们占用了 80 GB 的虚拟内存(Linux 上每个线程的栈大小默认是 8MB)。实际使用中,线程会使用内存并且不会在循环体中休眠,因此它可以非常快的占用完一个机器的内存。
|
||||||
|
- 注2:自己动手实现一个线程池是个有意思的练习,但我现在还不想做。我曾写过用来练手的 [针对特殊任务的线程池][4]。是用 Python 写的;用 C 重写的话有些难度,但对于经验丰富的程序员,几个小时就够了。
|
||||||
|
|
||||||
|
--------------------------------------------------------------------------------
|
||||||
|
|
||||||
|
via: https://eli.thegreenplace.net/2017/concurrent-servers-part-2-threads/
|
||||||
|
|
||||||
|
作者:[Eli Bendersky][a]
|
||||||
|
译者:[GitFuture](https://github.com/GitFuture)
|
||||||
|
校对:[wxy](https://github.com/wxy)
|
||||||
|
|
||||||
|
本文由 [LCTT](https://github.com/LCTT/TranslateProject) 原创编译,[Linux中国](https://linux.cn/) 荣誉推出
|
||||||
|
|
||||||
|
[a]:https://eli.thegreenplace.net/pages/about
|
||||||
|
[1]:https://eli.thegreenplace.net/2017/concurrent-servers-part-2-threads/#id1
|
||||||
|
[2]:https://github.com/eliben/code-for-blog/blob/master/2017/async-socket-server/threadspammer.c
|
||||||
|
[3]:https://eli.thegreenplace.net/2017/concurrent-servers-part-2-threads/#id2
|
||||||
|
[4]:http://eli.thegreenplace.net/2011/12/27/python-threads-communication-and-stopping
|
||||||
|
[5]:https://eli.thegreenplace.net/tag/concurrency
|
||||||
|
[6]:https://eli.thegreenplace.net/tag/c-c
|
||||||
|
[7]:https://eli.thegreenplace.net/tag/python
|
||||||
|
[8]:https://linux.cn/article-8993-1.html
|
||||||
|
[9]:http://eli.thegreenplace.net/2017/concurrent-servers-part-2-threads/
|
||||||
|
[10]:http://eli.thegreenplace.net/2017/concurrent-servers-part-3-event-driven/
|
||||||
|
[11]:https://github.com/eliben/code-for-blog/blob/master/2017/async-socket-server/threaded-server.c
|
||||||
|
[12]:http://eli.thegreenplace.net/2010/04/05/pthreads-as-a-case-study-of-good-api-design
|
||||||
|
[13]:https://eli.thegreenplace.net/2017/concurrent-servers-part-2-threads/#id3
|
||||||
|
[14]:https://en.wikipedia.org/wiki/Denial-of-service_attack
|
||||||
|
[15]:https://en.wikipedia.org/wiki/Thread_pool
|
||||||
|
[16]:https://github.com/eliben/code-for-blog/blob/master/2017/async-socket-server/threadpool-server.py
|
||||||
|
[17]:https://eli.thegreenplace.net/2017/concurrent-servers-part-2-threads/#id4
|
||||||
|
[18]:http://eli.thegreenplace.net/2017/clojure-concurrency-and-blocking-with-coreasync/
|
||||||
|
[19]:https://eli.thegreenplace.net/2017/concurrent-servers-part-2-threads/
|
||||||
|
[20]:https://linux.cn/article-8993-1.html
|
@ -0,0 +1,53 @@
|
|||||||
|
如何成规模地部署多云的无服务器程序和 Cloud Foundry API
|
||||||
|
============================================================
|
||||||
|
|
||||||
|
> IBM 的 Ken Parmelee 说:“微服务和 API 是产品,我们需要以这种方式思考。”
|
||||||
|
|
||||||
|
领导 IBM 的 API 网关和 Big Blue 开源项目的的 Ken Parmelee 对以开源方式 “进攻” API 以及如何创建微服务和使其伸缩有一些思考。
|
||||||
|
|
||||||
|
Parmelee 说:“微服务和 API 是产品,我们需要以这种方式思考这些问题。当你开始这么做,人们依赖它作为它们业务的一部分。这是你在这个领域所做的关键方面。”
|
||||||
|
|
||||||
|

|
||||||
|
|
||||||
|
他在最近的[北欧 APIs 2017 平台峰会][3]登上讲台,并挑战了一些流行的观念。
|
||||||
|
|
||||||
|
“快速失败不是一个很好的概念。你想在第一场比赛中获得一些非常棒的东西。这并不意味着你需要花费大量的时间,而是应该让它变得非常棒,然后不断的发展和改进。如果一开始真的很糟糕,人们就不会想要用你。”
|
||||||
|
|
||||||
|
他谈及包括 [OpenWhisk][4] 在内的 IBM 现代无服务器架构,这是一个 IBM 和 Apache 之间的开源伙伴关系。 云优先的基于分布式事件的编程服务是这两年多来重点关注这个领域的成果;IBM 是该领域领先的贡献者,它是 IBM 云服务的基础。它提供基础设施即服务(IaaS)、自动缩放、为多种语言提供支持、用户只需支付实际使用费用即可。这次旅程充满了挑战,因为他们发现服务器操作需要安全、并且需要轻松 —— 匿名访问、缺少使用路径、固定的 URL 格式等。
|
||||||
|
|
||||||
|
任何人都可以在 30 秒内在 [https://console.bluemix.net/openwhisk/][5] 上尝试这些无服务器 API。“这听起来很有噱头,但这是很容易做到的。我们正在结合 [Cloud Foundry 中完成的工作][6],并在 OpenWhisk 下的 Bluemix 中发布了它们,以提供安全性和可扩展性。”
|
||||||
|
|
||||||
|
他说:“灵活性对于微服务也是非常重要的。 当你使用 API 在现实世界中工作时,你开始需要跨云进行扩展。”这意味着从你的内部云走向公共云,并且“对你要怎么做有一个实在的概念很重要”。
|
||||||
|
|
||||||
|

|
||||||
|
|
||||||
|
在思考“任何云概念”的时候,他警告说,不是“将其放入一个 Docker 容器,并到处运行。这很棒,但需要在这些环境中有效运行。Docker 和 Kubernetes 有提供了很多帮助,但是你想要你的操作方式付诸实施。” 提前考虑 API 的使用,无论是在内部运行还是扩展到公有云并可以公开调用 - 你需要有这样的“架构观”,他补充道。
|
||||||
|
|
||||||
|
Parmelee 说:“我们都希望我们所创造的有价值,并被广泛使用。” API 越成功,将其提升到更高水平的挑战就越大。
|
||||||
|
|
||||||
|

|
||||||
|
|
||||||
|
*API 是微服务或“服务间”的组成部分。*
|
||||||
|
|
||||||
|
他说,API 的未来是原生云的 - 无论你从哪里开始。关键因素是可扩展性,简化后端管理,降低成本,避免厂商锁定。
|
||||||
|
|
||||||
|
你可以在下面或在 [YouTube][7] 观看他整整 23 分钟的演讲。
|
||||||
|
|
||||||
|
--------------------------------------------------------------------------------
|
||||||
|
|
||||||
|
via: http://superuser.openstack.org/articles/deploy-multi-cloud-serverless-cloud-foundry-apis-scale/
|
||||||
|
|
||||||
|
作者:[Superuser][a]
|
||||||
|
译者:[geekpi](https://github.com/geekpi)
|
||||||
|
校对:[wxy](https://github.com/wxy)
|
||||||
|
|
||||||
|
本文由 [LCTT](https://github.com/LCTT/TranslateProject) 原创编译,[Linux中国](https://linux.cn/) 荣誉推出
|
||||||
|
|
||||||
|
[a]:http://superuser.openstack.org/articles/author/superuser/
|
||||||
|
[1]:http://superuser.openstack.org/articles/author/superuser/
|
||||||
|
[2]:http://superuser.openstack.org/articles/deploy-multi-cloud-serverless-cloud-foundry-apis-scale/
|
||||||
|
[3]:https://nordicapis.com/events/the-2017-api-platform-summit/
|
||||||
|
[4]:https://developer.ibm.com/openwhisk/
|
||||||
|
[5]:https://console.bluemix.net/openwhisk/
|
||||||
|
[6]:https://cloudfoundry.org/the-foundry/ibm-cloud/
|
||||||
|
[7]:https://www.youtube.com/jA25Kmxr6fU
|
@ -3,21 +3,21 @@ Linus Torvalds 说针对性的模糊测试正提升 Linux 安全性
|
|||||||
|
|
||||||
Linux 4.14 发布候选第五版已经出来。Linus Torvalds 说:“可以去测试了。”
|
Linux 4.14 发布候选第五版已经出来。Linus Torvalds 说:“可以去测试了。”
|
||||||
|
|
||||||

|

|
||||||
|
|
||||||
随着宣布推出 Linux 内核 4.14 的第五个候选版本,Linus Torvalds 表示模糊测试正产生一系列稳定的安全更新
|
随着宣布推出 Linux 内核 4.14 的第五个候选版本,Linus Torvalds 表示<ruby>模糊测试<rt>fuzzing</rt></ruby>正产生一系列稳定的安全更新。
|
||||||
|
|
||||||
模糊测试通过产生随机代码来引发错误来对系统进行压力测试,从而有助于识别潜在的安全漏洞。模糊测试正在帮助软件开发人员在向用户发布软件之前捕获错误。
|
模糊测试通过产生随机代码来引发错误来对系统进行压力测试,从而有助于识别潜在的安全漏洞。模糊测试可以帮助软件开发人员在向用户发布软件之前捕获错误。
|
||||||
|
|
||||||
Google 使用各种模糊测试工具来查找其他供应商软件中的错误。微软推出了 [Project Springfield][1] 模糊测试服务,它能让企业客户测试自己的软件。
|
Google 使用各种模糊测试工具来查找它及其它供应商软件中的错误。微软推出了 [Project Springfield][1] 模糊测试服务,它能让企业客户测试自己的软件。
|
||||||
|
|
||||||
正如 Torvalds 指出的那样,Linux 内核开发人员从一开始就一直在使用模糊测试程序,例如 1991 年发布的工具 “crashme”,它在近 20 年后被[ Google 安全研究员 Tavis Ormandy ][2] 用来测试在虚拟机中处理不受信任的数据时,宿主机是否受到良好保护。
|
正如 Torvalds 指出的那样,Linux 内核开发人员从一开始就一直在使用模糊测试流程,例如 1991 年发布的工具 “crashme”,它在近 20 年后被 [Google 安全研究员 Tavis Ormandy ][2] 用来测试在虚拟机中处理不受信任的数据时,宿主机是否受到良好保护。
|
||||||
|
|
||||||
Torvalds 说:“另外值得一提的是人们有做多少随机化模糊测试,而且这正在发现东西。”
|
Torvalds [说][3]:“另外值得一提的是人们做了多少随机化模糊测试,而且这正在发现东西。”
|
||||||
|
|
||||||
“我们一直在做模糊测试(谁记得只能生成随机代码,并跳转过去的老 “crashme” 程序?我们过去很早就这样做),但是人们一直在做一些很好的针对性的驱动子系统等等,而且已经有了各种各样的修复(不仅仅是上周的这些)。很高兴可以看到。
|
“我们一直在做模糊测试(谁还记得只是生成随机代码,并跳转过去的老 “crashme” 程序?我们过去很早就这样做),人们在驱动子系统等方面做了一些很好的针对性模糊测试,而且已经有了各种各样的修复(不仅仅是上周的这些)。很高兴可以看到。”
|
||||||
|
|
||||||
Torvalds 提到,到目前为止,4.14 的发展“比预想的要麻烦一些”,但现在已经好了,并且在这个版本有一些 x86 系统以及带 AMD 芯片的系统的修复。还有几个驱动程序、核心内核组件和工具的更新。
|
Torvalds 提到,到目前为止,4.14 的发展“比预想的要麻烦一些”,但现在已经好了,并且在这个版本已经跑通了一些针对 x86 系统以及 AMD 芯片系统的修复。还有几个驱动程序、核心内核组件和工具的更新。
|
||||||
|
|
||||||
如前[所述][4],Linux 4.14 是 2017 年的长期稳定版本,迄今为止,它引入了核心内存管理功能、设备驱动程序更新以及文档、架构、文件系统、网络和工具的修改。
|
如前[所述][4],Linux 4.14 是 2017 年的长期稳定版本,迄今为止,它引入了核心内存管理功能、设备驱动程序更新以及文档、架构、文件系统、网络和工具的修改。
|
||||||
|
|
||||||
@ -25,9 +25,9 @@ Torvalds 提到,到目前为止,4.14 的发展“比预想的要麻烦一些
|
|||||||
|
|
||||||
via: http://www.zdnet.com/article/linus-torvalds-says-targeted-fuzzing-is-improving-linux-security/
|
via: http://www.zdnet.com/article/linus-torvalds-says-targeted-fuzzing-is-improving-linux-security/
|
||||||
|
|
||||||
作者:[Liam Tung ][a]
|
作者:[Liam Tung][a]
|
||||||
译者:[geekpi](https://github.com/geekpi)
|
译者:[geekpi](https://github.com/geekpi)
|
||||||
校对:[校对者ID](https://github.com/校对者ID)
|
校对:[wxy](https://github.com/wxy)
|
||||||
|
|
||||||
本文由 [LCTT](https://github.com/LCTT/TranslateProject) 原创编译,[Linux中国](https://linux.cn/) 荣誉推出
|
本文由 [LCTT](https://github.com/LCTT/TranslateProject) 原创编译,[Linux中国](https://linux.cn/) 荣誉推出
|
||||||
|
|
73
published/20171017 PingCAP Launches TiDB 1.0.md
Normal file
73
published/20171017 PingCAP Launches TiDB 1.0.md
Normal file
@ -0,0 +1,73 @@
|
|||||||
|
PingCAP 推出 TiDB 1.0
|
||||||
|
============================================================
|
||||||
|
|
||||||
|
> PingCAP 推出了 TiDB 1.0,一个可扩展的混合数据库解决方案
|
||||||
|
|
||||||
|
2017 年 10 月 16 日, 一家尖端的分布式数据库技术公司 PingCAP Inc. 正式宣布发布 [TiDB][4] 1.0。TiDB 是一个开源的分布式混合事务/分析处理 (HTAP) 数据库,它使企业能够使用单个数据库来满足这两个负载。
|
||||||
|
|
||||||
|
在当前的数据库环境中,基础架构工程师通常要使用一个数据库进行在线事务处理(OLTP),另一个用于在线分析处理(OLAP)。TiDB 旨在通过构建一个基于实时事务数据的实时业务分析的 HTAP 数据库来打破这种分离。有了 TiDB,工程师现在可以花更少的时间来管理多个数据库解决方案,并有更多的时间为他们的公司提供业务价值。TiDB 的一个金融证券公司的用户正在利用这项技术为财富管理和用户角色的应用提供支持。借助 TiDB,该公司可以轻松处理 web 量级的计费记录,并进行关键任务时间敏感的数据分析。
|
||||||
|
|
||||||
|
PingCAP 联合创始人兼 CEO 刘奇(Max Liu)说:
|
||||||
|
|
||||||
|
> “两年半前,Edward、Dylan 和我开始这个旅程,为长期困扰基础设施软件业的老问题建立一个新的数据库。今天,我们很自豪地宣布,这个数据库 TiDB 可以面向生产环境了。亚伯拉罕·林肯曾经说过,‘预测未来的最好办法就是创造’,我们在 771 天前预测的未来,现在我们已经创造了,这不仅是我们团队的每一个成员,也是我们的开源社区的每个贡献者、用户和合作伙伴的努力工作和奉献。今天,我们庆祝和感谢开源精神的力量。明天,我们将继续创造我们相信的未来。”
|
||||||
|
|
||||||
|
TiDB 已经在亚太地区 30 多家公司投入生产环境,其中包括 [摩拜][5]、[Gaea][6] 和 [YOUZU][7] 等快速增长的互联网公司。使用案例涵盖从在线市场和游戏到金融科技、媒体和旅游的多个行业。
|
||||||
|
|
||||||
|
### TiDB 功能
|
||||||
|
|
||||||
|
**水平可扩展性**
|
||||||
|
|
||||||
|
TiDB 随着你的业务发展而增长。你可以通过添加更多机器来增加存储和计算能力。
|
||||||
|
|
||||||
|
**兼容 MySQL 协议**
|
||||||
|
|
||||||
|
像用 MySQL 一样使用 TiDB。你可以用 TiDB 替换 MySQL 来增强你的应用,且在大多数情况下不用更改一行代码,也几乎没有迁移成本。
|
||||||
|
|
||||||
|
**自动故障切换和高可用性**
|
||||||
|
|
||||||
|
你的数据和程序始终处于在线状态。TiDB 自动处理故障并保护你的应用免受整个数据中心的机器故障甚至停机。
|
||||||
|
|
||||||
|
**一致的分布式事务**
|
||||||
|
|
||||||
|
TiDB 类似于单机关系型数据库系统(RDBMS)。你可以启动跨多台机器的事务,而不用担心一致性。TiDB 使你的应用程序代码简单而强大。
|
||||||
|
|
||||||
|
**在线 DDL**
|
||||||
|
|
||||||
|
根据你的要求更改 TiDB 模式。你可以添加新的列和索引,而不会停止或影响你正在进行的操作。
|
||||||
|
|
||||||
|
[现在尝试TiDB!][8]
|
||||||
|
|
||||||
|
### 使用案例
|
||||||
|
|
||||||
|
- [yuanfudao.com 中 TiDB 如何处理快速的数据增长和复杂查询] [9]
|
||||||
|
- [从 MySQL 迁移到 TiDB 以每天处理数千万行数据] [10]
|
||||||
|
|
||||||
|
### 更多信息:
|
||||||
|
|
||||||
|
TiDB 内部:
|
||||||
|
|
||||||
|
* [数据存储][1]
|
||||||
|
* [计算][2]
|
||||||
|
* [调度][3]
|
||||||
|
|
||||||
|
--------------------------------------------------------------------------------
|
||||||
|
|
||||||
|
via: https://pingcap.github.io/blog/2017/10/17/announcement/
|
||||||
|
|
||||||
|
作者:[PingCAP][a]
|
||||||
|
译者:[geekpi](https://github.com/geekpi)
|
||||||
|
校对:[wxy](https://github.com/wxy)
|
||||||
|
|
||||||
|
本文由 [LCTT](https://github.com/LCTT/TranslateProject) 原创编译,[Linux中国](https://linux.cn/) 荣誉推出
|
||||||
|
|
||||||
|
[a]:https://pingcap.github.io/blog/
|
||||||
|
[1]:https://pingcap.github.io/blog/2017/07/11/tidbinternal1/
|
||||||
|
[2]:https://pingcap.github.io/blog/2017/07/11/tidbinternal2/
|
||||||
|
[3]:https://pingcap.github.io/blog/2017/07/20/tidbinternal3/
|
||||||
|
[4]:https://github.com/pingcap/tidb
|
||||||
|
[5]:https://en.wikipedia.org/wiki/Mobike
|
||||||
|
[6]:http://www.gaea.com/en/
|
||||||
|
[7]:http://www.yoozoo.com/aboutEn
|
||||||
|
[8]:https://pingcap.com/doc-QUICKSTART
|
||||||
|
[9]:https://pingcap.github.io/blog/2017/08/08/tidbforyuanfudao/
|
||||||
|
[10]:https://pingcap.github.io/blog/2017/05/22/Comparison-between-MySQL-and-TiDB-with-tens-of-millions-of-data-per-day/
|
75
published/20171020 Why and how you should switch to Linux.md
Normal file
75
published/20171020 Why and how you should switch to Linux.md
Normal file
@ -0,0 +1,75 @@
|
|||||||
|
为什么要切换到 Linux 系统?我该怎么做?
|
||||||
|
============================================================
|
||||||
|
|
||||||
|
> 是时候做出改变了。
|
||||||
|
|
||||||
|

|
||||||
|
|
||||||
|
*让 Ubuntu,一个简单易用的 Linux 版本,来掌管你的电脑。*
|
||||||
|
|
||||||
|
当你在选购电脑的时候,你可能会在 [Windows][1] 和 [macOS][2] 之间犹豫,但是可能基本不会想到 Linux。尽管如此,这个名气没那么大的操作系统仍然拥有庞大而忠诚的粉丝。因为它相对于它的竞争者,有很大的优势。
|
||||||
|
|
||||||
|
不管你是完全不了解 Linux,或是已经尝试过一两次,我们希望你考虑一下在你的下一台笔记本或台式机上运行 Linux,或者可以和现存系统做个双启动。请继续阅读下去,看是不是时候该切换了。
|
||||||
|
|
||||||
|
### 什么是 Linux?
|
||||||
|
|
||||||
|
如果你已经非常熟悉 Linux,可以跳过这个部分。对于不熟悉的其他人,Linux 是一个免费的开源操作系统,所有人都可以去探索它的代码。技术上来说,术语“Linux”说的只是内核,或者核心代码。不过,人们一般用这个名字统称整个操作系统,包括界面和集成的应用。
|
||||||
|
|
||||||
|
因为所有人都可以修改它,Linux 有非常自由的可定制性,这鼓舞了很多程序员制作并发布了自己的系统——常称为<ruby>发行版<rt>distro</rt></ruby>。这些不同口味的系统,每一个都有自己特色的软件和界面。一些比较有名的发行版,模仿了熟悉的 Windows 或 macOS 操作系统,比如 [Ubuntu][3]、 [Linux Mint][4] 和 [Zorin OS][5]。当你准备选择一个发行版时,可以去它们官网看一下,试试看是不是适合自己。
|
||||||
|
|
||||||
|
为了制作和维护这些 Linux 发行版,大量的开发者无偿地贡献了自己的时间。有时候,利润主导的公司为了拓展自己的软件销售领域,也会主导开发带有独特特性的 Linux 版本。比如 Android(它虽然不能当作一个完整的 Linux 操作系统)就是以基于 Linux 内核的,这也是为什么它有很多[不同变种][6]的原因。另外,很多服务器和数据中心也运行了 Linux,所以很有可能这个操作系统托管着你正在看的网页。
|
||||||
|
|
||||||
|
### 有什么好处?
|
||||||
|
|
||||||
|
首先,Linux 是免费而且开源的,意味着你可以将它安装到你现有的电脑或笔记本上,或者你自己组装的机器,而不用支付任何费用。系统会自带一些常用软件,包括网页浏览器、媒体播放器、[图像编辑器][7]和[办公软件][8],所以你也不用为了查看图片或处理文档再支付其他额外费用。而且,以后还可以免费升级。
|
||||||
|
|
||||||
|
Linux 比其他系统能更好的防御恶意软件,强大到你都不需要运行杀毒软件。开发者们在最早构建时就考虑了安全性,比如说,操作系统只运行可信的软件。而且,很少有恶意软件针对这个系统,对于黑客来说,这样做没有价值。Linux 也并不是完全没有任何漏洞,只不过对于一般只运行已验证软件的家庭用户来说,并不用太担心安全性。
|
||||||
|
|
||||||
|
这个操作系统对硬件资源的要求比起数据臃肿的 Windows 或 macOS 来说也更少。一些发行版不像它们名气更大的表兄弟,默认集成了更少的组件,开发者特别开发了一些,比如 [Puppy Linux][9] 和 [Linux Lite][10],让系统尽可能地轻量。这让 Linux 非常适合那些家里有很老的电脑的人。如果你的远古笔记本正在原装操作系统的重压下喘息,试试装一个 Linux,应该会快很多。如果不愿意的话,你也不用抛弃旧系统,我们会在后面的部分里解释怎么做。
|
||||||
|
|
||||||
|
尽管你可能会需要一点时间来适应新系统,不过不用太久,你就会发现 Linux 界面很容易使用。任何年龄和任何技术水平的人都可以掌握这个软件。而且在线的 Linux 社区提供了大量的帮助和支持。说到社区,下载 Linux 也是对开源软件运动的支持:这些开发者一起工作,并不收取任何费用,为全球用户开发更优秀的软件。
|
||||||
|
|
||||||
|
### 我该从哪儿开始?
|
||||||
|
|
||||||
|
Linux 据说只有专家才能安装。不过比起前几年,现在安装并运行操作系统已经非常简单了。
|
||||||
|
|
||||||
|
首先,打开你喜欢的发行版的网站,按照上面的安装指南操作。一般会需要烧录一张 DVD 或者制作一个带有必要程序的 U 盘,然后重启你的电脑,执行这段程序。实际上,这个操作系统的一个好处是,你可以将它直接安装在可插拔的 U 盘上,我们有一个[如何把电脑装在 U 盘里][11]的完整指南。
|
||||||
|
|
||||||
|
如果你想在不影响原来旧系统的情况下运行 Linux,你可以选择从 DVD 或 U 盘或者电脑硬盘的某个分区(分成不同的区来独立运行不同的操作系统)单独启动。有些 Linux 发行版在安装过程中会帮你自动处理磁盘分区。或者你可以用[磁盘管理器][12] (Windows)或者[磁盘工具][13] (macOS)自己调整分区。
|
||||||
|
|
||||||
|
这些安装说明可能看上去很模糊,但是不要担心:每个发行版都会提供详细的安装指引,虽然大多数情况下过程都差不多。比如,如果你想安装 Ubuntu(最流行的家用 Linux 发行版中的一个),可以[参考这里的指引][14]。(在安装之前,你也可以[尝试运行一下][15])你需要下载最新的版本,烧录到 DVD 或是 U 盘里,然后再用光盘或 U 盘引导开机,然后跟随安装向导里的指引操作。安装完成时提示安装额外软件时,Ubuntu 会引导你打开合适的工具。
|
||||||
|
|
||||||
|
如果你要在一台全新的电脑上安装 Linux,那没什么需要特别留意的。不过如果你要保留旧系统的情况下安装新系统,我们建议你首先[备份自己的数据][16]。在安装过程中,也要注意选择双启动选项,避免擦除现有的系统和文件。你选好的发行版的介绍里会有更详细的说明:你可以在[这里][17]查看 Zorin OS 的完整介绍,[这里][18]有 Linux Mint的,其他发行版的介绍在他们各自的网站上也都会有。
|
||||||
|
|
||||||
|
就这些了!那么,你准备好试试 Linux 了吗?
|
||||||
|
|
||||||
|
--------------------------------------------------------------------------------
|
||||||
|
|
||||||
|
via: https://www.popsci.com/switch-to-linux-operating-system
|
||||||
|
|
||||||
|
作者:[David Nield][a]
|
||||||
|
译者:[zpl1025](https://github.com/zpl1025)
|
||||||
|
校对:[wxy](https://github.com/wxy)
|
||||||
|
|
||||||
|
本文由 [LCTT](https://github.com/LCTT/TranslateProject) 原创编译,[Linux中国](https://linux.cn/) 荣誉推出
|
||||||
|
|
||||||
|
[a]:https://www.popsci.com/authors/david-nield
|
||||||
|
[1]:https://www.popsci.com/windows-tweaks-improve-performance
|
||||||
|
[2]:https://www.popsci.com/macos-tweaks-improve-performance
|
||||||
|
[3]:https://www.ubuntu.com/
|
||||||
|
[4]:https://linuxmint.com/
|
||||||
|
[5]:https://zorinos.com/
|
||||||
|
[6]:https://lineageos.org/
|
||||||
|
[7]:https://www.gimp.org/
|
||||||
|
[8]:https://www.libreoffice.org/
|
||||||
|
[9]:http://puppylinux.org/main/Overview%20and%20Getting%20Started.htm
|
||||||
|
[10]:https://www.linuxliteos.com/
|
||||||
|
[11]:https://www.popsci.com/portable-computer-usb-stick
|
||||||
|
[12]:https://www.disk-partition.com/windows-10/windows-10-disk-management-0528.html
|
||||||
|
[13]:https://support.apple.com/kb/PH22240?locale=en_US
|
||||||
|
[14]:https://tutorials.ubuntu.com/tutorial/tutorial-install-ubuntu-desktop?backURL=%2F#0
|
||||||
|
[15]:https://tutorials.ubuntu.com/tutorial/try-ubuntu-before-you-install?backURL=%2F#0
|
||||||
|
[16]:https://www.popsci.com/back-up-and-protect-your-data
|
||||||
|
[17]:https://zorinos.com/help/install-zorin-os/
|
||||||
|
[18]:https://linuxmint.com/documentation.php
|
||||||
|
[19]:https://www.popsci.com/authors/david-nield
|
@ -1,7 +1,6 @@
|
|||||||
# Kprobes Event Tracing on ARMv8
|
# Kprobes Event Tracing on ARMv8
|
||||||
|
|
||||||
|

|
||||||

|
|
||||||
|
|
||||||
### Introduction
|
### Introduction
|
||||||
|
|
||||||
|
@ -1,287 +0,0 @@
|
|||||||
Server-side I/O Performance: Node vs. PHP vs. Java vs. Go
|
|
||||||
============
|
|
||||||
|
|
||||||
Understanding the Input/Output (I/O) model of your application can mean the difference between an application that deals with the load it is subjected to, and one that crumples in the face of real-world use cases. Perhaps while your application is small and does not serve high loads, it may matter far less. But as your application’s traffic load increases, working with the wrong I/O model can get you into a world of hurt.
|
|
||||||
|
|
||||||
And like most any situation where multiple approaches are possible, it’s not just a matter of which one is better, it’s a matter of understanding the tradeoffs. Let’s take a walk across the I/O landscape and see what we can spy.
|
|
||||||
|
|
||||||
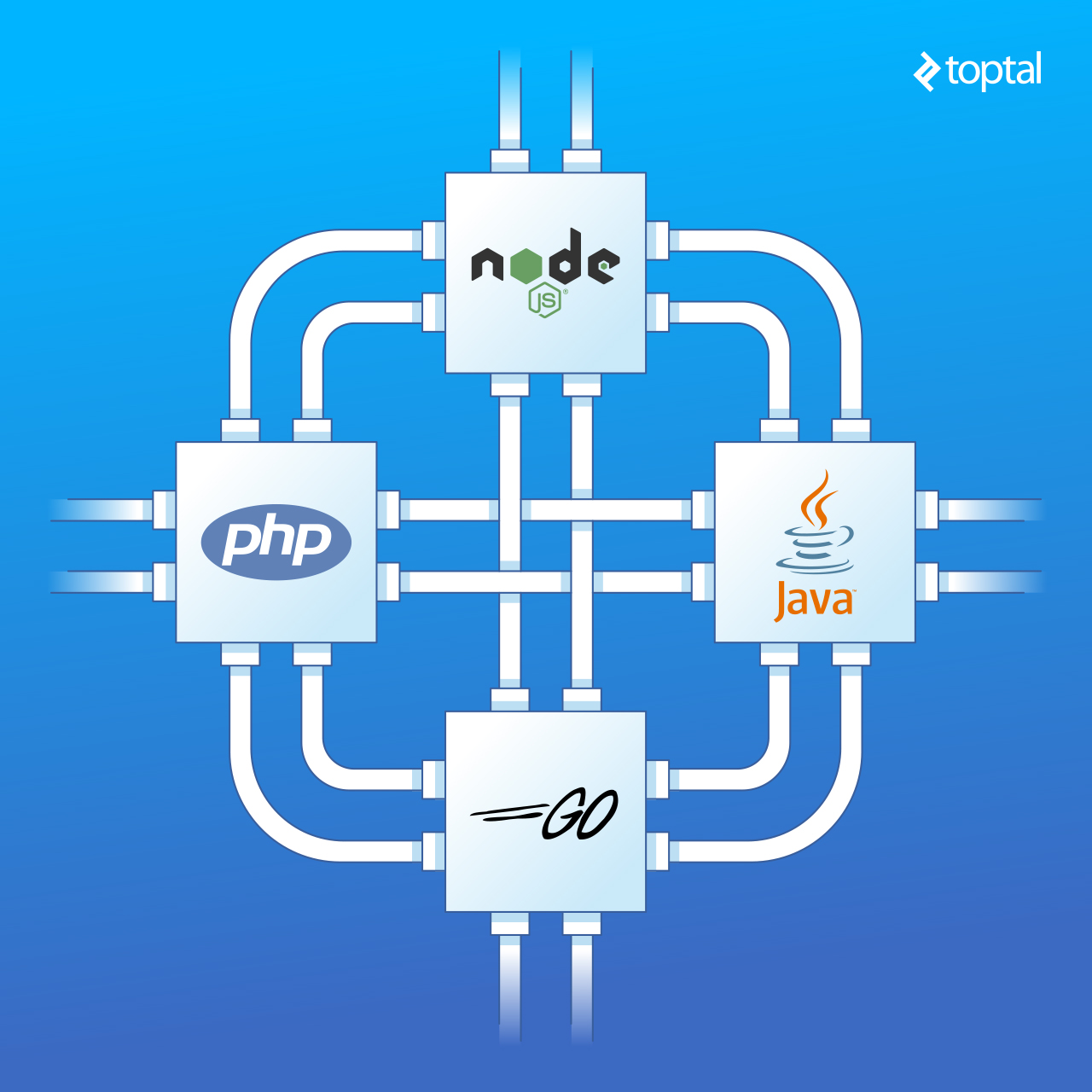
|
|
||||||
|
|
||||||
In this article, we’ll be comparing Node, Java, Go, and PHP with Apache, discussing how the different languages model their I/O, the advantages and disadvantages of each model, and conclude with some rudimentary benchmarks. If you’re concerned about the I/O performance of your next web application, this article is for you.
|
|
||||||
|
|
||||||
### I/O Basics: A Quick Refresher
|
|
||||||
|
|
||||||
To understand the factors involved with I/O, we must first review the concepts down at the operating system level. While it is unlikely that will have to deal with many of these concepts directly, you deal with them indirectly through your application’s runtime environment all the time. And the details matter.
|
|
||||||
|
|
||||||
### System Calls
|
|
||||||
|
|
||||||
Firstly, we have system calls, which can be described as follows:
|
|
||||||
|
|
||||||
* Your program (in “user land,” as they say) must ask the operating system kernel to perform an I/O operation on its behalf.
|
|
||||||
|
|
||||||
* A “syscall” is the means by which your program asks the kernel do something. The specifics of how this is implemented vary between OSes but the basic concept is the same. There is going to be some specific instruction that transfers control from your program over to the kernel (like a function call but with some special sauce specifically for dealing with this situation). Generally speaking, syscalls are blocking, meaning your program waits for the kernel to return back to your code.
|
|
||||||
|
|
||||||
* The kernel performs the underlying I/O operation on the physical device in question (disk, network card, etc.) and replies to the syscall. In the real world, the kernel might have to do a number of things to fulfill your request including waiting for the device to be ready, updating its internal state, etc., but as an application developer, you don’t care about that. That’s the kernel’s job.
|
|
||||||
|
|
||||||
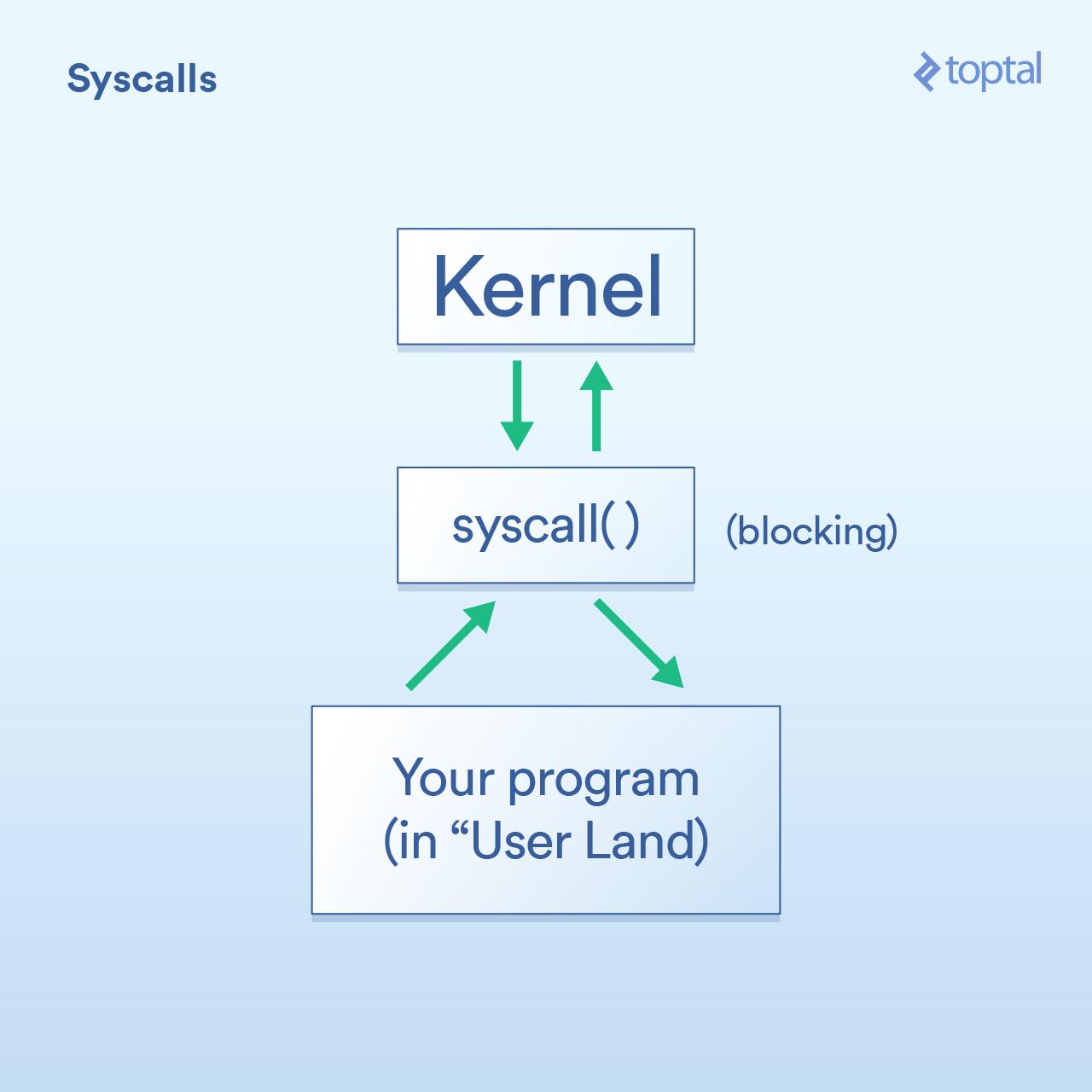
|
|
||||||
|
|
||||||
### Blocking vs. Non-blocking Calls
|
|
||||||
|
|
||||||
Now, I just said above that syscalls are blocking, and that is true in a general sense. However, some calls are categorized as “non-blocking,” which means that the kernel takes your request, puts it in queue or buffer somewhere, and then immediately returns without waiting for the actual I/O to occur. So it “blocks” for only a very brief time period, just long enough to enqueue your request.
|
|
||||||
|
|
||||||
Some examples (of Linux syscalls) might help clarify: - `read()` is a blocking call - you pass it a handle saying which file and a buffer of where to deliver the data it reads, and the call returns when the data is there. Note that this has the advantage of being nice and simple. - `epoll_create()`, `epoll_ctl()` and `epoll_wait()` are calls that, respectively, let you create a group of handles to listen on, add/remove handlers from that group and then block until there is any activity. This allows you to efficiently control a large number of I/O operations with a single thread, but I’m getting ahead of myself. This is great if you need the functionality, but as you can see it’s certainly more complex to use.
|
|
||||||
|
|
||||||
It’s important to understand the order of magnitude of difference in timing here. If a CPU core is running at 3GHz, without getting into optimizations the CPU can do, it’s performing 3 billion cycles per second (or 3 cycles per nanosecond). A non-blocking system call might take on the order of 10s of cycles to complete - or “a relatively few nanoseconds”. A call that blocks for information being received over the network might take a much longer time - let’s say for example 200 milliseconds (1/5 of a second). And let’s say, for example, the non-blocking call took 20 nanoseconds, and the blocking call took 200,000,000 nanoseconds. Your process just waited 10 million times longer for the blocking call.
|
|
||||||
|
|
||||||
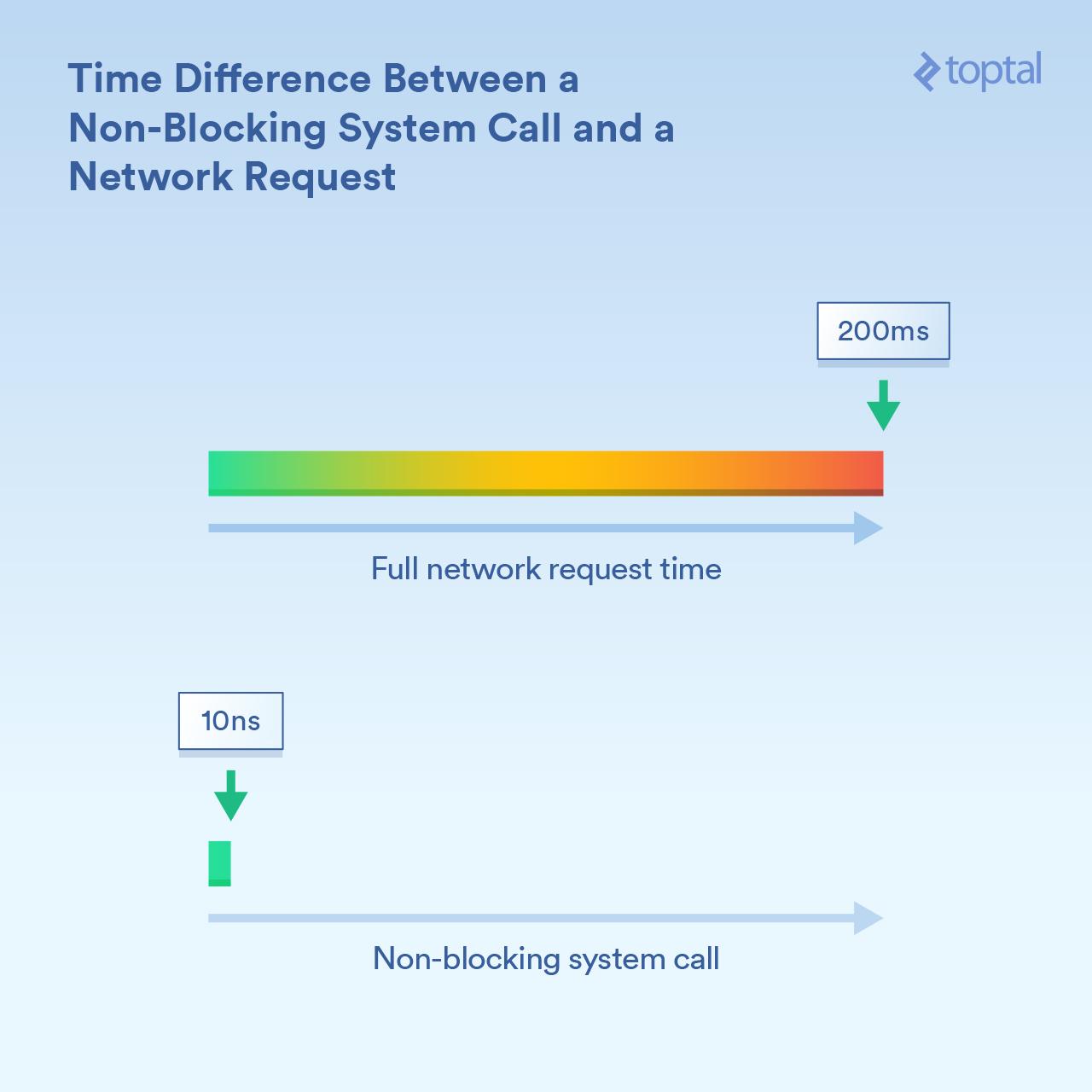
|
|
||||||
The kernel provides the means to do both blocking I/O (“read from this network connection and give me the data”) and non-blocking I/O (“tell me when any of these network connections have new data”). And which mechanism is used will block the calling process for dramatically different lengths of time.
|
|
||||||
|
|
||||||
### Scheduling
|
|
||||||
|
|
||||||
The third thing that’s critical to follow is what happens when you have a lot of threads or processes that start blocking.
|
|
||||||
|
|
||||||
For our purposes, there is not a huge difference between a thread and process. In real life, the most noticeable performance-related difference is that since threads share the same memory, and processes each have their own memory space, making separate processes tends to take up a lot more memory. But when we’re talking about scheduling, what it really boils down to is a list of things (threads and processes alike) that each need to get a slice of execution time on the available CPU cores. If you have 300 threads running and 8 cores to run them on, you have to divide the time up so each one gets its share, with each core running for a short period of time and then moving onto the next thread. This is done through a “context switch,” making the CPU switch from running one thread/process to the next.
|
|
||||||
|
|
||||||
These context switches have a cost associated with them - they take some time. In some fast cases, it may be less than 100 nanoseconds, but it is not uncommon for it to take 1000 nanoseconds or longer depending on the implementation details, processor speed/architecture, CPU cache, etc.
|
|
||||||
|
|
||||||
And the more threads (or processes), the more context switching. When we’re talking about thousands of threads, and hundreds of nanoseconds for each, things can get very slow.
|
|
||||||
|
|
||||||
However, non-blocking calls in essence tell the kernel “only call me when you have some new data or event on one of any of these connections.” These non-blocking calls are designed to efficiently handle large I/O loads and reduce context switching.
|
|
||||||
|
|
||||||
With me so far? Because now comes the fun part: Let’s look at what some popular languages do with these tools and draw some conclusions about the tradeoffs between ease of use and performance… and other interesting tidbits.
|
|
||||||
|
|
||||||
As a note, while the examples shown in this article are trivial (and partial, with only the relevant bits shown); database access, external caching systems (memcache, et. all) and anything that requires I/O is going to end up performing some sort of I/O call under the hood which will have the same effect as the simple examples shown. Also, for the scenarios where the I/O is described as “blocking” (PHP, Java), the HTTP request and response reads and writes are themselves blocking calls: Again, more I/O hidden in the system with its attendant performance issues to take into account.
|
|
||||||
|
|
||||||
There are a lot of factors that go into choosing a programming language for a project. There are even a lot factors when you only consider performance. But, if you are concerned that your program will be constrained primarily by I/O, if I/O performance is make or break for your project, these are things you need to know. ## The “Keep It Simple” Approach: PHP
|
|
||||||
|
|
||||||
Back in the 90’s, a lot of people were wearing [Converse][1] shoes and writing CGI scripts in Perl. Then PHP came along and, as much as some people like to rag on it, it made making dynamic web pages much easier.
|
|
||||||
|
|
||||||
The model PHP uses is fairly simple. There are some variations to it but your average PHP server looks like:
|
|
||||||
|
|
||||||
An HTTP request comes in from a user’s browser and hits your Apache web server. Apache creates a separate process for each request, with some optimizations to re-use them in order to minimize how many it has to do (creating processes is, relatively speaking, slow). Apache calls PHP and tells it to run the appropriate `.php` file on the disk. PHP code executes and does blocking I/O calls. You call `file_get_contents()` in PHP and under the hood it makes `read()` syscalls and waits for the results.
|
|
||||||
|
|
||||||
And of course the actual code is simply embedded right into your page, and operations are blocking:
|
|
||||||
|
|
||||||
```
|
|
||||||
<?php
|
|
||||||
|
|
||||||
// blocking file I/O
|
|
||||||
$file_data = file_get_contents(‘/path/to/file.dat’);
|
|
||||||
|
|
||||||
// blocking network I/O
|
|
||||||
$curl = curl_init('http://example.com/example-microservice');
|
|
||||||
$result = curl_exec($curl);
|
|
||||||
|
|
||||||
// some more blocking network I/O
|
|
||||||
$result = $db->query('SELECT id, data FROM examples ORDER BY id DESC limit 100');
|
|
||||||
|
|
||||||
?>
|
|
||||||
|
|
||||||
```
|
|
||||||
|
|
||||||
In terms of how this integrates with system, it’s like this:
|
|
||||||
|
|
||||||
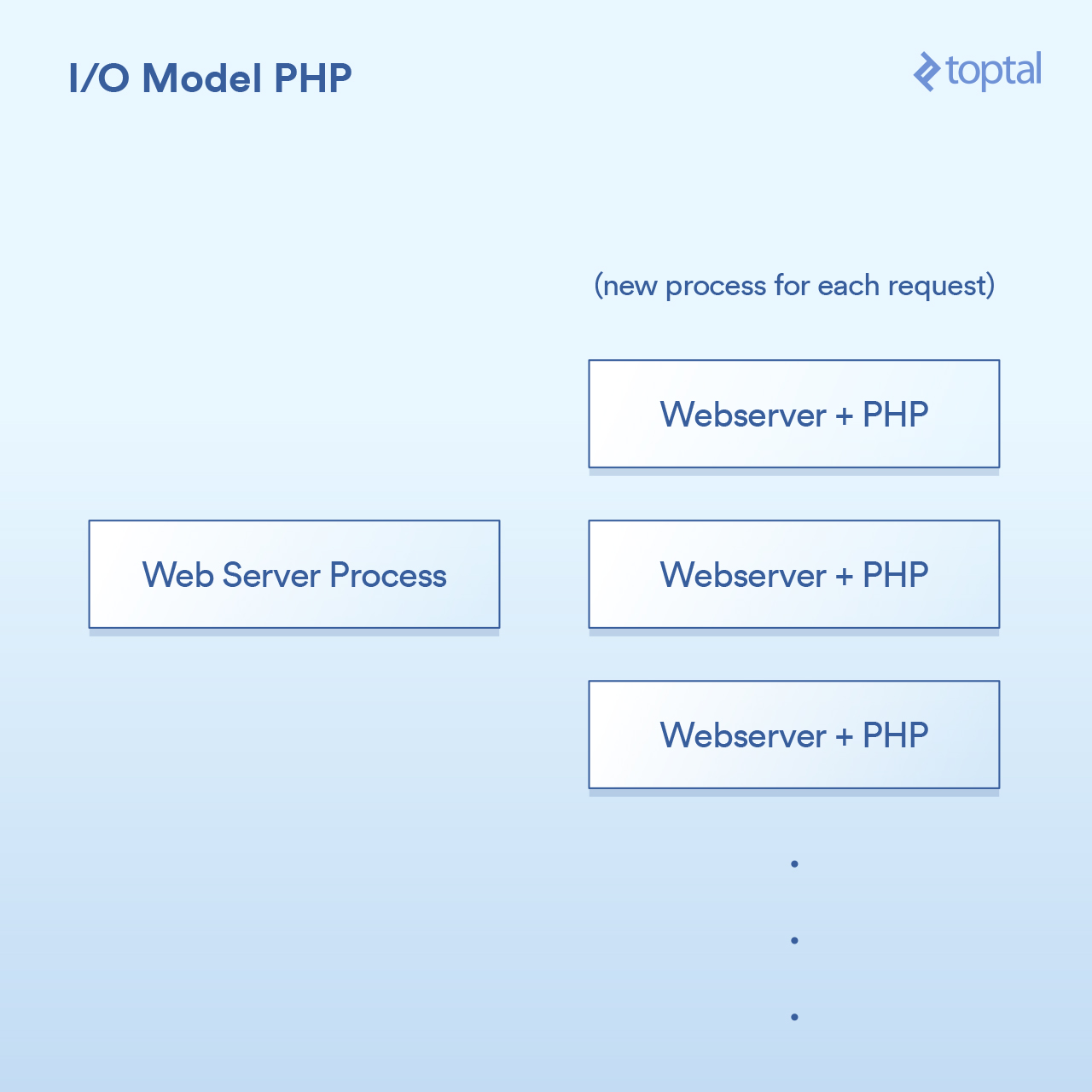
|
|
||||||
|
|
||||||
Pretty simple: one process per request. I/O calls just block. Advantage? It’s simple and it works. Disadvantage? Hit it with 20,000 clients concurrently and your server will burst into flames. This approach does not scale well because the tools provided by the kernel for dealing with high volume I/O (epoll, etc.) are not being used. And to add insult to injury, running a separate process for each request tends to use a lot of system resources, especially memory, which is often the first thing you run out of in a scenario like this.
|
|
||||||
|
|
||||||
_Note: The approach used for Ruby is very similar to that of PHP, and in a broad, general, hand-wavy way they can be considered the same for our purposes._
|
|
||||||
|
|
||||||
### The Multithreaded Approach: Java
|
|
||||||
|
|
||||||
So Java comes along, right about the time you bought your first domain name and it was cool to just randomly say “dot com” after a sentence. And Java has multithreading built into the language, which (especially for when it was created) is pretty awesome.
|
|
||||||
|
|
||||||
Most Java web servers work by starting a new thread of execution for each request that comes in and then in this thread eventually calling the function that you, as the application developer, wrote.
|
|
||||||
|
|
||||||
Doing I/O in a Java Servlet tends to look something like:
|
|
||||||
|
|
||||||
```
|
|
||||||
public void doGet(HttpServletRequest request,
|
|
||||||
HttpServletResponse response) throws ServletException, IOException
|
|
||||||
{
|
|
||||||
|
|
||||||
// blocking file I/O
|
|
||||||
InputStream fileIs = new FileInputStream("/path/to/file");
|
|
||||||
|
|
||||||
// blocking network I/O
|
|
||||||
URLConnection urlConnection = (new URL("http://example.com/example-microservice")).openConnection();
|
|
||||||
InputStream netIs = urlConnection.getInputStream();
|
|
||||||
|
|
||||||
// some more blocking network I/O
|
|
||||||
out.println("...");
|
|
||||||
}
|
|
||||||
|
|
||||||
```
|
|
||||||
|
|
||||||
Since our `doGet` method above corresponds to one request and is run in its own thread, instead of a separate process for each request which requires its own memory, we have a separate thread. This has some nice perks, like being able to share state, cached data, etc. between threads because they can access each other’s memory, but the impact on how it interacts with the schedule it still almost identical to what is being done in the PHP example previously. Each request gets a new thread and the various I/O operations block inside that thread until the request is fully handled. Threads are pooled to minimize the cost of creating and destroying them, but still, thousands of connections means thousands of threads which is bad for the scheduler.
|
|
||||||
|
|
||||||
An important milestone is that in version 1.4 Java (and a significant upgrade again in 1.7) gained the ability to do non-blocking I/O calls. Most applications, web and otherwise, don’t use it, but at least it’s available. Some Java web servers try to take advantage of this in various ways; however, the vast majority of deployed Java applications still work as described above.
|
|
||||||
|
|
||||||
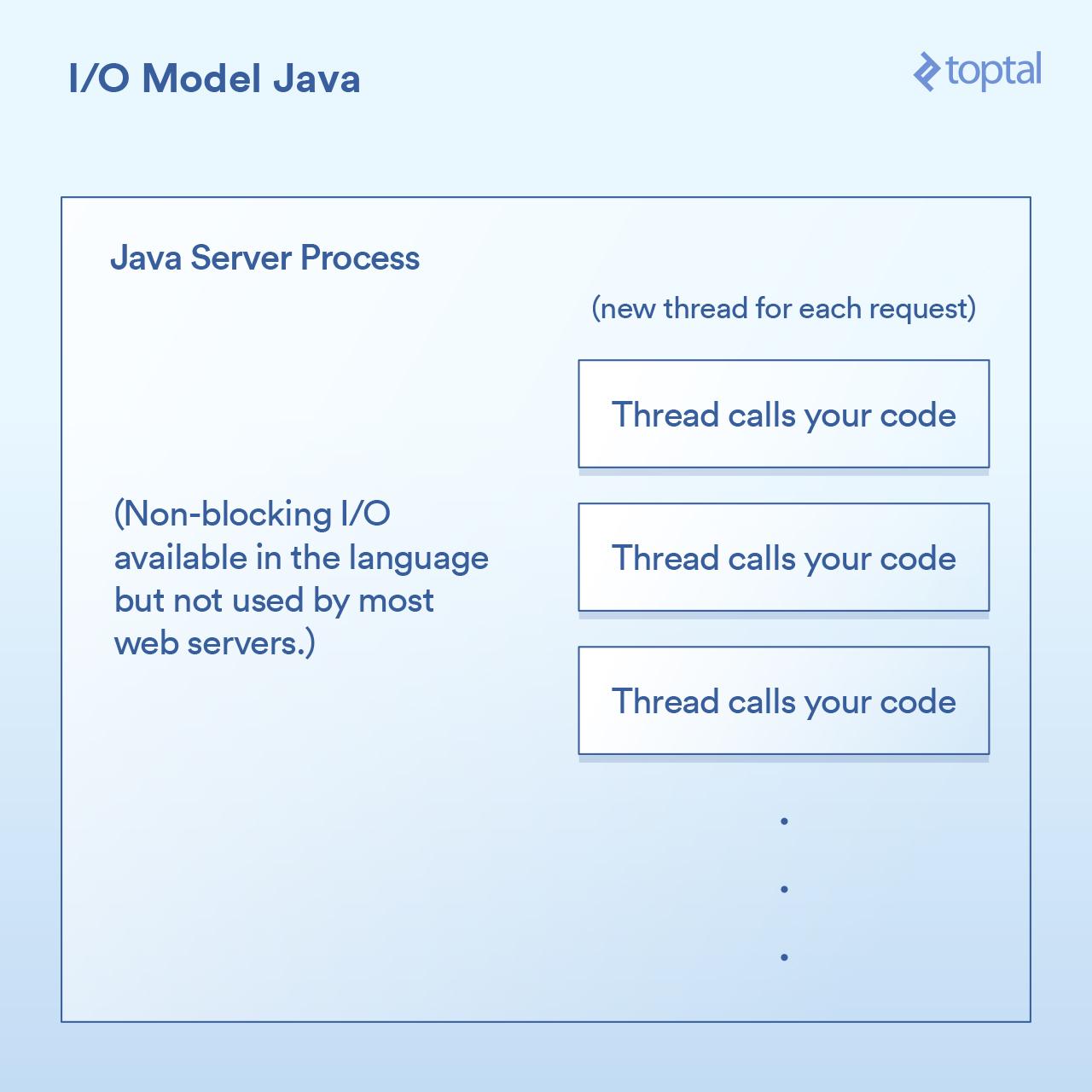
|
|
||||||
|
|
||||||
Java gets us closer and certainly has some good out-of-the-box functionality for I/O, but it still doesn’t really solve the problem of what happens when you have a heavily I/O bound application that is getting pounded into the ground with many thousands of blocking threads.
|
|
||||||
|
|
||||||
<form action="https://www.toptal.com/blog/subscription" class="embeddable_form" data-entity="blog_subscription" data-remote="" data-view="form#form" method="post" style="border: 0px; vertical-align: baseline; min-height: 0px; min-width: 0px;">Like what you're reading?Get the latest updates first.<input autocomplete="off" class="input is-medium" data-role="email" name="blog_subscription[email]" placeholder="Enter your email address..." type="text" style="-webkit-appearance: none; background: rgb(250, 250, 250); border-radius: 4px; border-width: 1px; border-style: solid; border-color: rgb(238, 238, 238); color: rgb(60, 60, 60); font-family: proxima-nova, Arial, sans-serif; font-size: 14px; padding: 15px 12px; transition: all 0.2s; width: 799.36px;"><input class="button is-green_candy is-default is-full_width" data-loader-text="Subscribing..." data-role="submit" type="submit" value="Get Exclusive Updates" style="-webkit-appearance: none; font-weight: 600; border-radius: 4px; transition: background 150ms; background: linear-gradient(rgb(67, 198, 146), rgb(57, 184, 133)); border-width: 1px; border-style: solid; border-color: rgb(31, 124, 87); box-shadow: rgb(79, 211, 170) 0px 1px inset; color: rgb(255, 255, 255); position: relative; text-shadow: rgb(28, 143, 61) 0px 1px 0px; font-size: 14px; padding: 15px 20px; width: 549.32px;">No spam. Just great engineering posts.</form>
|
|
||||||
|
|
||||||
### Non-blocking I/O as a First Class Citizen: Node
|
|
||||||
|
|
||||||
The popular kid on the block when it comes to better I/O is Node.js. Anyone who has had even the briefest introduction to Node has been told that it’s “non-blocking” and that it handles I/O efficiently. And this is true in a general sense. But the devil is in the details and the means by which this witchcraft was achieved matter when it comes to performance.
|
|
||||||
|
|
||||||
Essentially the paradigm shift that Node implements is that instead of essentially saying “write your code here to handle the request”, they instead say “write code here to start handling the request.” Each time you need to do something that involves I/O, you make the request and give a callback function which Node will call when it’s done.
|
|
||||||
|
|
||||||
Typical Node code for doing an I/O operation in a request goes like this:
|
|
||||||
|
|
||||||
```
|
|
||||||
http.createServer(function(request, response) {
|
|
||||||
fs.readFile('/path/to/file', 'utf8', function(err, data) {
|
|
||||||
response.end(data);
|
|
||||||
});
|
|
||||||
});
|
|
||||||
|
|
||||||
```
|
|
||||||
|
|
||||||
As you can see, there are two callback functions here. The first gets called when a request starts, and the second gets called when the file data is available.
|
|
||||||
|
|
||||||
What this does is basically give Node an opportunity to efficiently handle the I/O in between these callbacks. A scenario where it would be even more relevant is where you are doing a database call in Node, but I won’t bother with the example because it’s the exact same principle: You start the database call, and give Node a callback function, it performs the I/O operations separately using non-blocking calls and then invokes your callback function when the data you asked for is available. This mechanism of queuing up I/O calls and letting Node handle it and then getting a callback is called the “Event Loop.” And it works pretty well.
|
|
||||||
|
|
||||||
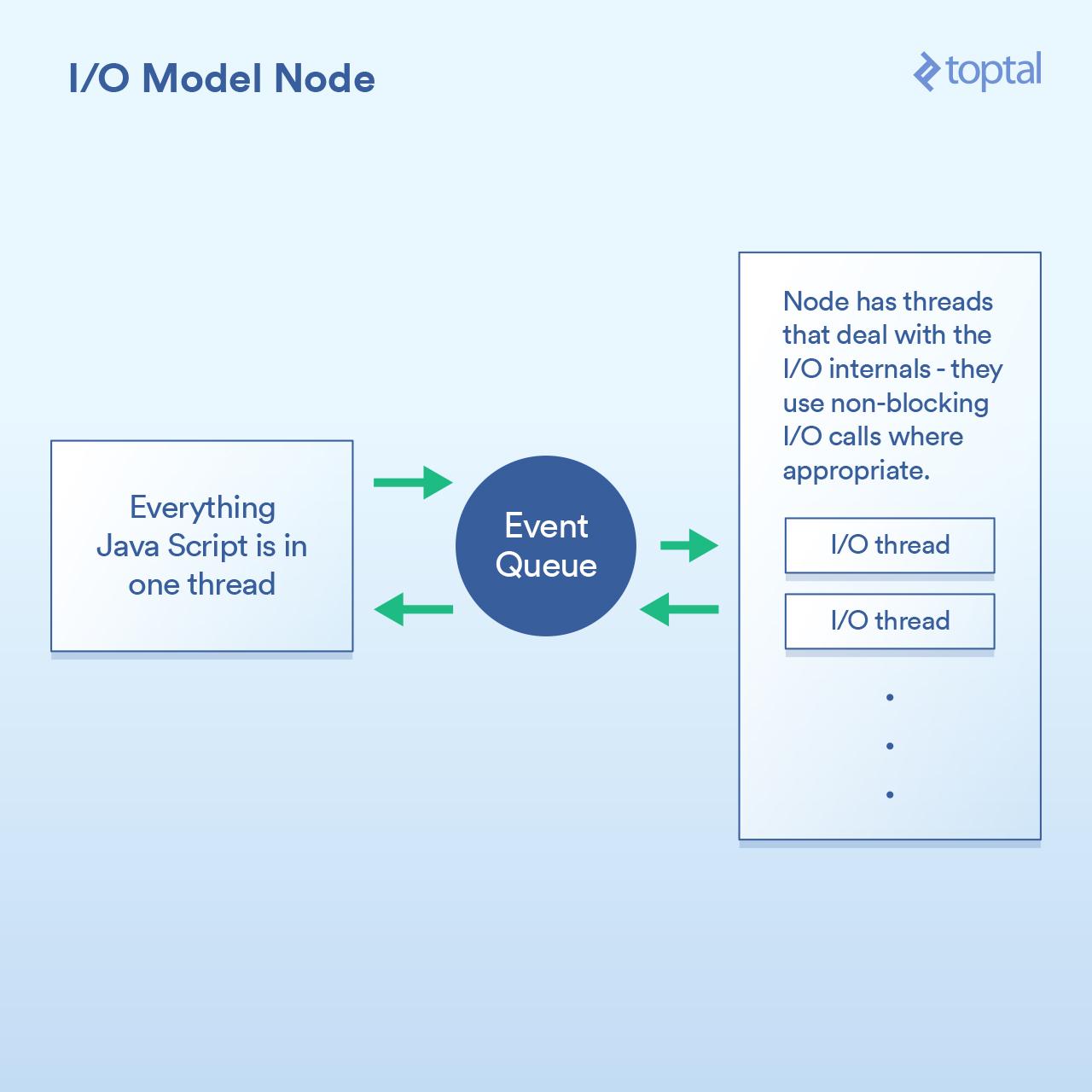
|
|
||||||
|
|
||||||
There is however a catch to this model. Under the hood, the reason for it has a lot more to do with how the V8 JavaScript engine (Chrome’s JS engine that is used by Node) is implemented [<sup style="border: 0px; vertical-align: super; min-height: 0px; min-width: 0px;">1</sup>][2] than anything else. The JS code that you write all runs in a single thread. Think about that for a moment. It means that while I/O is performed using efficient non-blocking techniques, your JS can that is doing CPU-bound operations runs in a single thread, each chunk of code blocking the next. A common example of where this might come up is looping over database records to process them in some way before outputting them to the client. Here’s an example that shows how that works:
|
|
||||||
|
|
||||||
```
|
|
||||||
var handler = function(request, response) {
|
|
||||||
|
|
||||||
connection.query('SELECT ...', function (err, rows) {
|
|
||||||
|
|
||||||
if (err) { throw err };
|
|
||||||
|
|
||||||
for (var i = 0; i < rows.length; i++) {
|
|
||||||
// do processing on each row
|
|
||||||
}
|
|
||||||
|
|
||||||
response.end(...); // write out the results
|
|
||||||
|
|
||||||
})
|
|
||||||
|
|
||||||
};
|
|
||||||
|
|
||||||
```
|
|
||||||
|
|
||||||
While Node does handle the I/O efficiently, that `for` loop in the example above is using CPU cycles inside your one and only main thread. This means that if you have 10,000 connections, that loop could bring your entire application to a crawl, depending on how long it takes. Each request must share a slice of time, one at a time, in your main thread.
|
|
||||||
|
|
||||||
The premise this whole concept is based on is that the I/O operations are the slowest part, thus it is most important to handle those efficiently, even if it means doing other processing serially. This is true in some cases, but not in all.
|
|
||||||
|
|
||||||
The other point is that, and while this is only an opinion, it can be quite tiresome writing a bunch of nested callbacks and some argue that it makes the code significantly harder to follow. It’s not uncommon to see callbacks nested four, five, or even more levels deep inside Node code.
|
|
||||||
|
|
||||||
We’re back again to the trade-offs. The Node model works well if your main performance problem is I/O. However, its achilles heel is that you can go into a function that is handling an HTTP request and put in CPU-intensive code and bring every connection to a crawl if you’re not careful.
|
|
||||||
|
|
||||||
### Naturally Non-blocking: Go
|
|
||||||
|
|
||||||
Before I get into the section for Go, it’s appropriate for me to disclose that I am a Go fanboy. I’ve used it for many projects and I’m openly a proponent of its productivity advantages, and I see them in my work when I use it.
|
|
||||||
|
|
||||||
That said, let’s look at how it deals with I/O. One key feature of the Go language is that it contains its own scheduler. Instead of each thread of execution corresponding to a single OS thread, it works with the concept of “goroutines.” And the Go runtime can assign a goroutine to an OS thread and have it execute, or suspend it and have it not be associated with an OS thread, based on what that goroutine is doing. Each request that comes in from Go’s HTTP server is handled in a separate Goroutine.
|
|
||||||
|
|
||||||
The diagram of how the scheduler works looks like this:
|
|
||||||
|
|
||||||
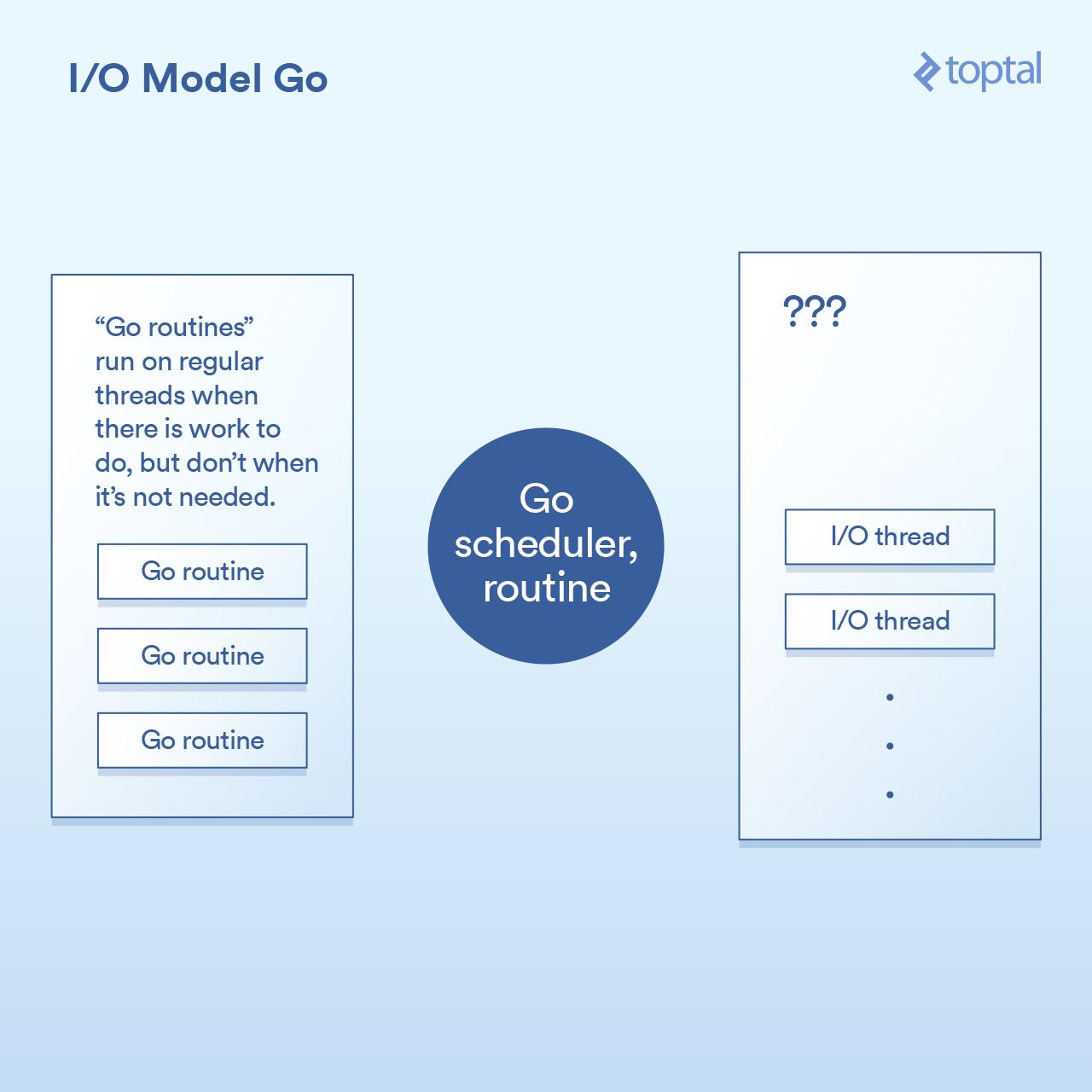
|
|
||||||
|
|
||||||
Under the hood, this is implemented by various points in the Go runtime that implement the I/O call by making the request to write/read/connect/etc., put the current goroutine to sleep, with the information to wake the goroutine back up when further action can be taken.
|
|
||||||
|
|
||||||
In effect, the Go runtime is doing something not terribly dissimilar to what Node is doing, except that the callback mechanism is built into the implementation of the I/O call and interacts with the scheduler automatically. It also does not suffer from the restriction of having to have all of your handler code run in the same thread, Go will automatically map your Goroutines to as many OS threads it deems appropriate based on the logic in its scheduler. The result is code like this:
|
|
||||||
|
|
||||||
```
|
|
||||||
func ServeHTTP(w http.ResponseWriter, r *http.Request) {
|
|
||||||
|
|
||||||
// the underlying network call here is non-blocking
|
|
||||||
rows, err := db.Query("SELECT ...")
|
|
||||||
|
|
||||||
for _, row := range rows {
|
|
||||||
// do something with the rows,
|
|
||||||
// each request in its own goroutine
|
|
||||||
}
|
|
||||||
|
|
||||||
w.Write(...) // write the response, also non-blocking
|
|
||||||
|
|
||||||
}
|
|
||||||
|
|
||||||
```
|
|
||||||
|
|
||||||
As you can see above, the basic code structure of what we are doing resembles that of the more simplistic approaches, and yet achieves non-blocking I/O under the hood.
|
|
||||||
|
|
||||||
In most cases, this ends up being “the best of both worlds.” Non-blocking I/O is used for all of the important things, but your code looks like it is blocking and thus tends to be simpler to understand and maintain. The interaction between the Go scheduler and the OS scheduler handles the rest. It’s not complete magic, and if you build a large system, it’s worth putting in the time to understand more detail about how it works; but at the same time, the environment you get “out-of-the-box” works and scales quite well.
|
|
||||||
|
|
||||||
Go may have its faults, but generally speaking, the way it handles I/O is not among them.
|
|
||||||
|
|
||||||
### Lies, Damned Lies and Benchmarks
|
|
||||||
|
|
||||||
It is difficult to give exact timings on the context switching involved with these various models. I could also argue that it’s less useful to you. So instead, I’ll give you some basic benchmarks that compare overall HTTP server performance of these server environments. Bear in mind that a lot of factors are involved in the performance of the entire end-to-end HTTP request/response path, and the numbers presented here are just some samples I put together to give a basic comparison.
|
|
||||||
|
|
||||||
For each of these environments, I wrote the appropriate code to read in a 64k file with random bytes, ran a SHA-256 hash on it N number of times (N being specified in the URL’s query string, e.g., `.../test.php?n=100`) and print the resulting hash in hex. I chose this because it’s a very simple way to run the same benchmarks with some consistent I/O and a controlled way to increase CPU usage.
|
|
||||||
|
|
||||||
See [these benchmark notes][3] for a bit more detail on the environments used.
|
|
||||||
|
|
||||||
First, let’s look at some low concurrency examples. Running 2000 iterations with 300 concurrent requests and only one hash per request (N=1) gives us this:
|
|
||||||
|
|
||||||
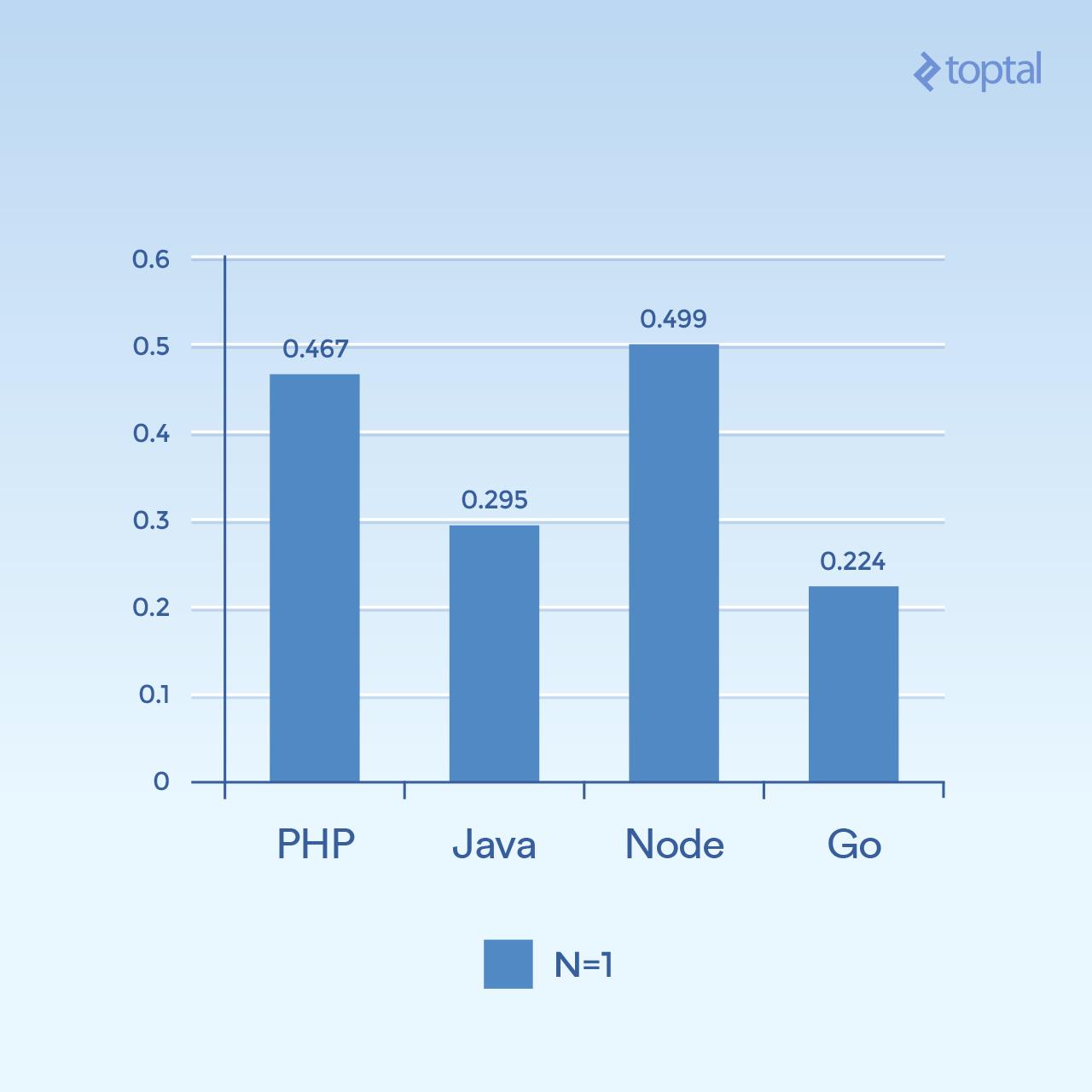
|
|
||||||
|
|
||||||
Times are the mean number of milliseconds to complete a request across all concurrent requests. Lower is better.
|
|
||||||
|
|
||||||
It’s hard to draw a conclusion from just this one graph, but this to me seems that, at this volume of connection and computation, we’re seeing times that more to do with the general execution of the languages themselves, much more so that the I/O. Note that the languages which are considered “scripting languages” (loose typing, dynamic interpretation) perform the slowest.
|
|
||||||
|
|
||||||
But what happens if we increase N to 1000, still with 300 concurrent requests - the same load but 100x more hash iterations (significantly more CPU load):
|
|
||||||
|
|
||||||
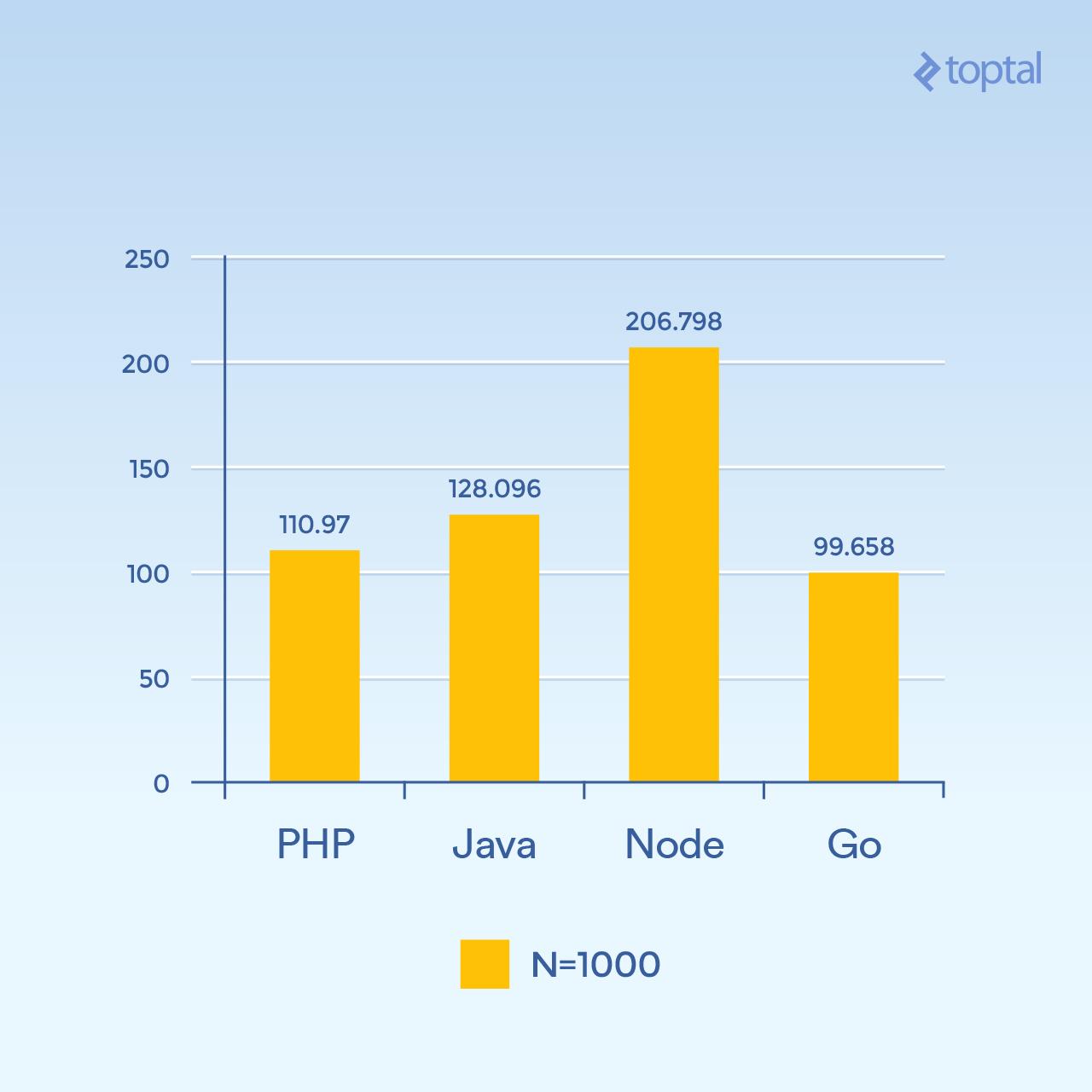
|
|
||||||
|
|
||||||
Times are the mean number of milliseconds to complete a request across all concurrent requests. Lower is better.
|
|
||||||
|
|
||||||
All of a sudden, Node performance drops significantly, because the CPU-intensive operations in each request are blocking each other. And interestingly enough, PHP’s performance gets much better (relative to the others) and beats Java in this test. (It’s worth noting that in PHP the SHA-256 implementation is written in C and the execution path is spending a lot more time in that loop, since we’re doing 1000 hash iterations now).
|
|
||||||
|
|
||||||
Now let’s try 5000 concurrent connections (with N=1) - or as close to that as I could come. Unfortunately, for most of these environments, the failure rate was not insignificant. For this chart, we’ll look at the total number of requests per second. _The higher the better_ :
|
|
||||||
|
|
||||||
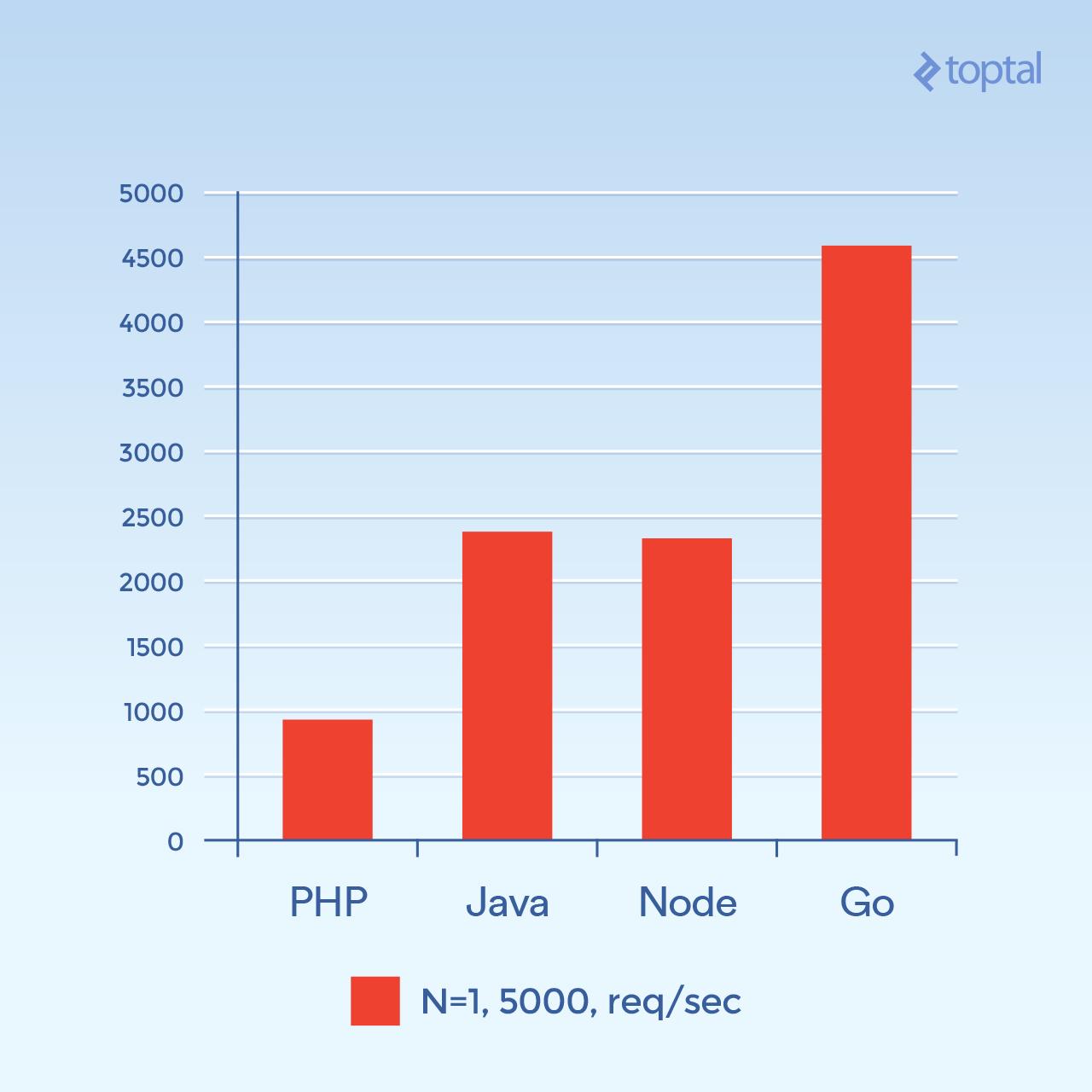
|
|
||||||
|
|
||||||
Total number of requests per second. Higher is better.
|
|
||||||
|
|
||||||
And the picture looks quite different. It’s a guess, but it looks like at high connection volume the per-connection overhead involved with spawning new processes and the additional memory associated with it in PHP+Apache seems to become a dominant factor and tanks PHP’s performance. Clearly, Go is the winner here, followed by Java, Node and finally PHP.
|
|
||||||
|
|
||||||
While the factors involved with your overall throughput are many and also vary widely from application to application, the more you understand about the guts of what is going on under the hood and the tradeoffs involved, the better off you’ll be.
|
|
||||||
|
|
||||||
### In Summary
|
|
||||||
|
|
||||||
With all of the above, it’s pretty clear that as languages have evolved, the solutions to dealing with large-scale applications that do lots of I/O have evolved with it.
|
|
||||||
|
|
||||||
To be fair, both PHP and Java, despite the descriptions in this article, do have [implementations][4] of [non-blocking I/O][5] [available for use][6] in [web applications][7]. But these are not as common as the approaches described above, and the attendant operational overhead of maintaining servers using such approaches would need to be taken into account. Not to mention that your code must be structured in a way that works with such environments; your “normal” PHP or Java web application usually will not run without significant modifications in such an environment.
|
|
||||||
|
|
||||||
As a comparison, if we consider a few significant factors that affect performance as well as ease of use, we get this:
|
|
||||||
|
|
||||||
| Language | Threads vs. Processes | Non-blocking I/O | Ease of Use |
|
|
||||||
| --- | --- | --- | --- |
|
|
||||||
| PHP | Processes | No | |
|
|
||||||
| Java | Threads | Available | Requires Callbacks |
|
|
||||||
| Node.js | Threads | Yes | Requires Callbacks |
|
|
||||||
| Go | Threads (Goroutines) | Yes | No Callbacks Needed |
|
|
||||||
|
|
||||||
Threads are generally going to be much more memory efficient than processes, since they share the same memory space whereas processes don’t. Combining that with the factors related to non-blocking I/O, we can see that at least with the factors considered above, as we move down the list the general setup as it related to I/O improves. So if I had to pick a winner in the above contest, it would certainly be Go.
|
|
||||||
|
|
||||||
Even so, in practice, choosing an environment in which to build your application is closely connected to the familiarity your team has with said environment, and the overall productivity you can achieve with it. So it may not make sense for every team to just dive in and start developing web applications and services in Node or Go. Indeed, finding developers or the familiarity of your in-house team is often cited as the main reason to not use a different language and/or environment. That said, times have changed over the past fifteen years or so, a lot.
|
|
||||||
|
|
||||||
Hopefully the above helps paint a clearer picture of what is happening under the hood and gives you some ideas of how to deal with real-world scalability for your application. Happy inputting and outputting!
|
|
||||||
|
|
||||||
--------------------------------------------------------------------------------
|
|
||||||
|
|
||||||
via: https://www.toptal.com/back-end/server-side-io-performance-node-php-java-go
|
|
||||||
|
|
||||||
作者:[ BRAD PEABODY][a]
|
|
||||||
译者:[译者ID](https://github.com/译者ID)
|
|
||||||
校对:[校对者ID](https://github.com/校对者ID)
|
|
||||||
|
|
||||||
本文由 [LCTT](https://github.com/LCTT/TranslateProject) 原创编译,[Linux中国](https://linux.cn/) 荣誉推出
|
|
||||||
|
|
||||||
[a]:https://www.toptal.com/resume/brad-peabody
|
|
||||||
[1]:https://www.pinterest.com/pin/414401603185852181/
|
|
||||||
[2]:http://www.journaldev.com/7462/node-js-architecture-single-threaded-event-loop
|
|
||||||
[3]:https://peabody.io/post/server-env-benchmarks/
|
|
||||||
[4]:http://reactphp.org/
|
|
||||||
[5]:http://amphp.org/
|
|
||||||
[6]:http://undertow.io/
|
|
||||||
[7]:https://netty.io/
|
|
@ -1,5 +1,3 @@
|
|||||||
翻译中 by zionfuo
|
|
||||||
|
|
||||||
How to take screenshots on Linux using Scrot
|
How to take screenshots on Linux using Scrot
|
||||||
============================================================
|
============================================================
|
||||||
|
|
||||||
|
@ -1,420 +0,0 @@
|
|||||||
【翻译中 @haoqixu】Your Serverless Raspberry Pi cluster with Docker
|
|
||||||
============================================================
|
|
||||||
|
|
||||||
|
|
||||||
This blog post will show you how to create your own Serverless Raspberry Pi cluster with Docker and the [OpenFaaS][33] framework. People often ask me what they should do with their cluster and this application is perfect for the credit-card sized device - want more compute power? Scale by adding more RPis.
|
|
||||||
|
|
||||||
> "Serverless" is a design pattern for event-driven architectures just like "bridge", "facade", "factory" and "cloud" are also abstract concepts - [so is "serverless"][21].
|
|
||||||
|
|
||||||
Here's my cluster for the blog post - with brass stand-offs used to separate each device.
|
|
||||||
|
|
||||||
|
|
||||||
|
|
||||||
### What is Serverless and why does it matter to you?
|
|
||||||
|
|
||||||
> As an industry we have some explaining to do regarding what the term "serverless" means. For the sake of this blog post let us assume that it is a new architectural pattern for event-driven architectures and that it lets you write tiny, reusable functions in whatever language you like. [Read more on Serverless here][22].
|
|
||||||
|
|
||||||
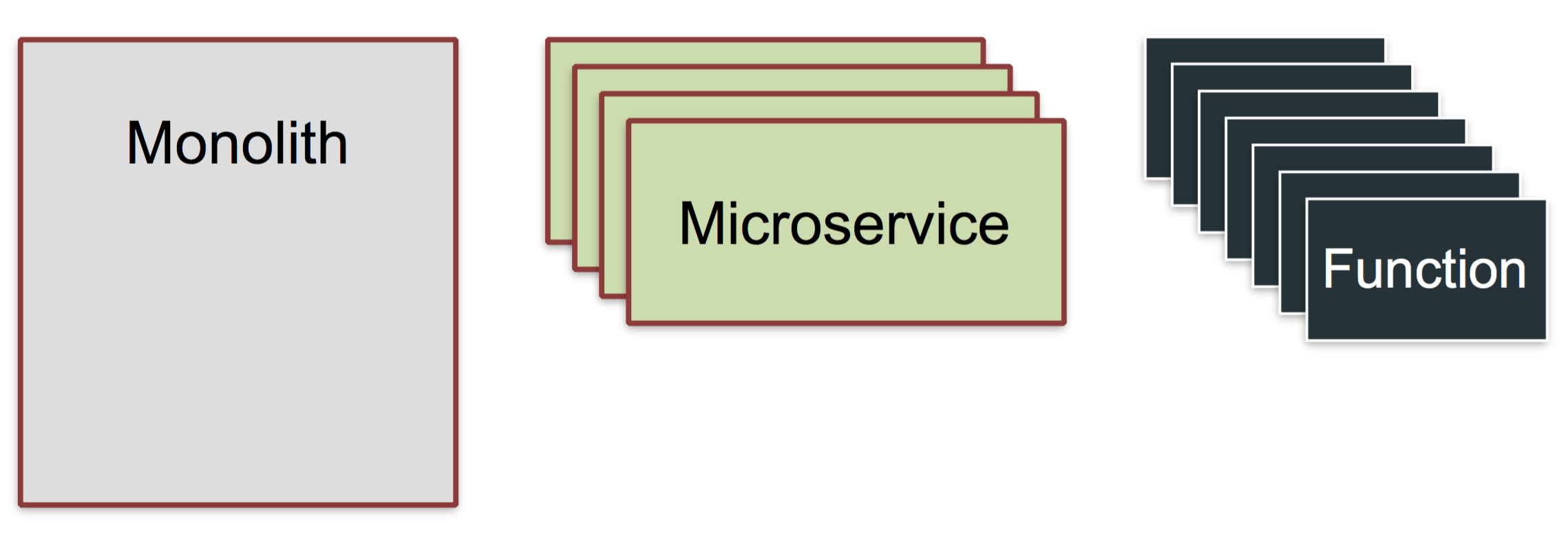
|
|
||||||
_Serverless is an architectural pattern resulting in: Functions as a Service, or FaaS_
|
|
||||||
|
|
||||||
Serverless functions can do anything, but usually work on a given input - such as an event from GitHub, Twitter, PayPal, Slack, your Jenkins CI pipeline - or in the case of a Raspberry Pi - maybe a real-world sensor input such as a PIR motion sensor, laser tripwire or even a temperature gauge.
|
|
||||||
|
|
||||||

|
|
||||||
|
|
||||||
Let's also assume that serverless functions tend to make use of third-party back-end services to become greater than the sum of their parts.
|
|
||||||
|
|
||||||
For more background information checkout my latest blog post - [Introducing Functions as a Service (FaaS)][34]
|
|
||||||
|
|
||||||
### Overview
|
|
||||||
|
|
||||||
We'll be using [OpenFaaS][35] which lets you turn any single host or cluster into a back-end to run serverless functions. Any binary, script or programming language that can be deployed with Docker will work on [OpenFaaS][36] and you can chose on a scale between speed and flexibility. The good news is a UI and metrics are also built-in.
|
|
||||||
|
|
||||||
Here's what we'll do:
|
|
||||||
|
|
||||||
* Set up Docker on one or more hosts (Raspberry Pi 2/3)
|
|
||||||
|
|
||||||
* Join them together in a Docker Swarm
|
|
||||||
|
|
||||||
* Deploy [OpenFaaS][23]
|
|
||||||
|
|
||||||
* Write our first function in Python
|
|
||||||
|
|
||||||
### Docker Swarm
|
|
||||||
|
|
||||||
Docker is a technology for packaging and deploying applications, it also has clustering built-in which is secure by default and only takes one line to set up. OpenFaaS uses Docker and Swarm to spread your serverless functions across all your available RPis.
|
|
||||||
|
|
||||||
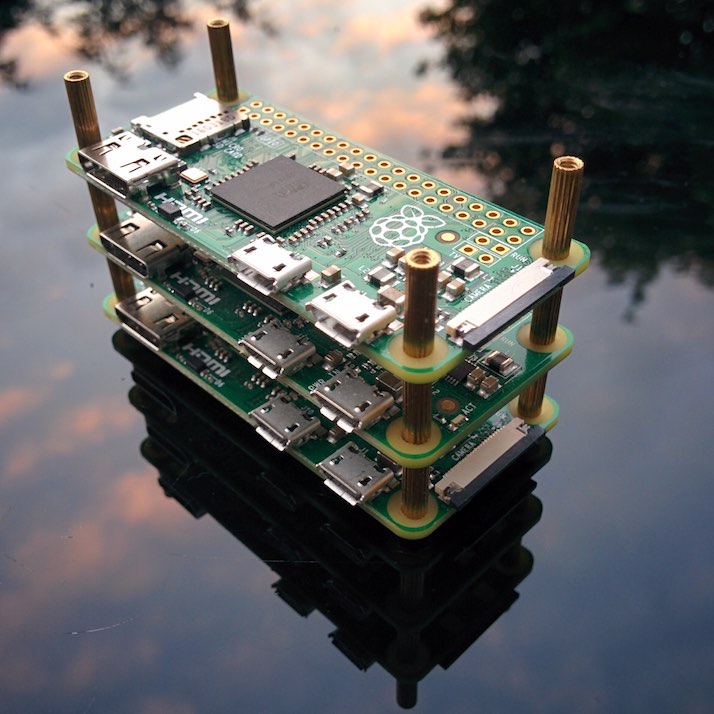
|
|
||||||
_Pictured: 3x Raspberry Pi Zero_
|
|
||||||
|
|
||||||
I recommend using Raspberry Pi 2 or 3 for this project along with an Ethernet switch and a [powerful USB multi-adapter][37].
|
|
||||||
|
|
||||||
### Prepare Raspbian
|
|
||||||
|
|
||||||
Flash [Raspbian Jessie Lite][38] to an SD card, 8GB will do but 16GB is recommended.
|
|
||||||
|
|
||||||
_Note: do not download Raspbian Stretch_
|
|
||||||
|
|
||||||
> The community is helping the Docker team to ready support for Raspbian Stretch, but it's not yet seamless. Please download Jessie Lite from the [RPi foundation's archive here][24]
|
|
||||||
|
|
||||||
I recommend using [Etcher.io][39] to flash the image.
|
|
||||||
|
|
||||||
> Before booting the RPi you'll need to create a file in the boot partition called "ssh". Just keep the file blank. This enables remote logins.
|
|
||||||
|
|
||||||
* Power up and change the hostname
|
|
||||||
|
|
||||||
Now power up the RPi and connect with `ssh`
|
|
||||||
|
|
||||||
```
|
|
||||||
$ ssh pi@raspberrypi.local
|
|
||||||
|
|
||||||
```
|
|
||||||
|
|
||||||
> The password is `raspberry`.
|
|
||||||
|
|
||||||
Use the `raspi-config` utility to change the hostname to `swarm-1` or similar and then reboot.
|
|
||||||
|
|
||||||
While you're here you can also change the memory split between the GPU (graphics) and the system to 16mb.
|
|
||||||
|
|
||||||
* Now install Docker
|
|
||||||
|
|
||||||
We can use a utility script for this:
|
|
||||||
|
|
||||||
```
|
|
||||||
$ curl -sSL https://get.docker.com | sh
|
|
||||||
|
|
||||||
```
|
|
||||||
|
|
||||||
> This installation method may change in the future. As noted above you need to be running Jessie so we have a known configuration.
|
|
||||||
|
|
||||||
You may see a warning like this, but you can ignore it and you should end up with Docker CE 17.05:
|
|
||||||
|
|
||||||
```
|
|
||||||
WARNING: raspbian is no longer updated @ https://get.docker.com/
|
|
||||||
Installing the legacy docker-engine package...
|
|
||||||
|
|
||||||
```
|
|
||||||
|
|
||||||
After, make sure your user account can access the Docker client with this command:
|
|
||||||
|
|
||||||
```
|
|
||||||
$ usermod pi -aG docker
|
|
||||||
|
|
||||||
```
|
|
||||||
|
|
||||||
> If your username isn't `pi` then replace `pi` with `alex` for instance.
|
|
||||||
|
|
||||||
* Change the default password
|
|
||||||
|
|
||||||
Type in `$sudo passwd pi` and enter a new password, please don't skip this step!
|
|
||||||
|
|
||||||
* Repeat
|
|
||||||
|
|
||||||
Now repeat the above for each of the RPis.
|
|
||||||
|
|
||||||
### Create your Swarm cluster
|
|
||||||
|
|
||||||
Log into the first RPi and type in the following:
|
|
||||||
|
|
||||||
```
|
|
||||||
$ docker swarm init
|
|
||||||
Swarm initialized: current node (3ra7i5ldijsffjnmubmsfh767) is now a manager.
|
|
||||||
|
|
||||||
To add a worker to this swarm, run the following command:
|
|
||||||
|
|
||||||
docker swarm join \
|
|
||||||
--token SWMTKN-1-496mv9itb7584pzcddzj4zvzzfltgud8k75rvujopw15n3ehzu-af445b08359golnzhncbdj9o3 \
|
|
||||||
192.168.0.79:2377
|
|
||||||
|
|
||||||
```
|
|
||||||
|
|
||||||
You'll see the output with your join token and the command to type into the other RPis. So log into each one with `ssh` and paste in the command.
|
|
||||||
|
|
||||||
Give this a few seconds to connect then on the first RPi check all your nodes are listed:
|
|
||||||
|
|
||||||
```
|
|
||||||
$ docker node ls
|
|
||||||
ID HOSTNAME STATUS AVAILABILITY MANAGER STATUS
|
|
||||||
3ra7i5ldijsffjnmubmsfh767 * swarm1 Ready Active Leader
|
|
||||||
k9mom28s2kqxocfq1fo6ywu63 swarm3 Ready Active
|
|
||||||
y2p089bs174vmrlx30gc77h4o swarm4 Ready Active
|
|
||||||
|
|
||||||
```
|
|
||||||
|
|
||||||
Congratulations! You have a Raspberry Pi cluster!
|
|
||||||
|
|
||||||
_*More on clusters_
|
|
||||||
|
|
||||||
You can see my three hosts up and running. Only one is a manager at this point. If our manager were to go _down_ then we'd be in an unrecoverable situation. The way around this is to add redundancy by promoting more of the nodes to managers - they will still run workloads, unless you specifically set up your services to only be placed on workers.
|
|
||||||
|
|
||||||
To upgrade a worker to a manager, just type in `docker node promote <node_name>`from one of your managers.
|
|
||||||
|
|
||||||
> Note: Swarm commands such as `docker service ls` or `docker node ls` can only be done on the manager.
|
|
||||||
|
|
||||||
For a deeper dive into how managers and workers keep "quorum" head over to the [Docker Swarm admin guide][40].
|
|
||||||
|
|
||||||
### OpenFaaS
|
|
||||||
|
|
||||||
Now let's move on to deploying a real application to enable Serverless functions to run on our cluster. [OpenFaaS][41] is a framework for Docker that lets any process or container become a serverless function - at scale and on any hardware or cloud. Thanks to Docker and Golang's portability it also runs very well on a Raspberry Pi.
|
|
||||||
|
|
||||||
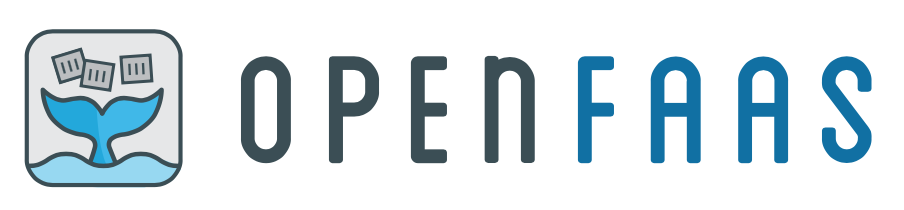
|
|
||||||
|
|
||||||
> Please show your support and **star** the [OpenFaaS][25] repository on GitHub.
|
|
||||||
|
|
||||||
Log into the first RPi (where we ran `docker swarm init`) and clone/deploy the project:
|
|
||||||
|
|
||||||
```
|
|
||||||
$ git clone https://github.com/alexellis/faas/
|
|
||||||
$ cd faas
|
|
||||||
$ ./deploy_stack.armhf.sh
|
|
||||||
Creating network func_functions
|
|
||||||
Creating service func_gateway
|
|
||||||
Creating service func_prometheus
|
|
||||||
Creating service func_alertmanager
|
|
||||||
Creating service func_nodeinfo
|
|
||||||
Creating service func_markdown
|
|
||||||
Creating service func_wordcount
|
|
||||||
Creating service func_echoit
|
|
||||||
|
|
||||||
```
|
|
||||||
|
|
||||||
Your other RPis will now be instructed by Docker Swarm to start pulling the Docker images from the internet and extracting them to the SD card. The work will be spread across all the RPis so that none of them are overworked.
|
|
||||||
|
|
||||||
This could take a couple of minutes, so you can check when it's done by typing in:
|
|
||||||
|
|
||||||
```
|
|
||||||
$ watch 'docker service ls'
|
|
||||||
ID NAME MODE REPLICAS IMAGE PORTS
|
|
||||||
57ine9c10xhp func_wordcount replicated 1/1 functions/alpine:latest-armhf
|
|
||||||
d979zipx1gld func_prometheus replicated 1/1 alexellis2/prometheus-armhf:1.5.2 *:9090->9090/tcp
|
|
||||||
f9yvm0dddn47 func_echoit replicated 1/1 functions/alpine:latest-armhf
|
|
||||||
lhbk1fc2lobq func_markdown replicated 1/1 functions/markdownrender:latest-armhf
|
|
||||||
pj814yluzyyo func_alertmanager replicated 1/1 alexellis2/alertmanager-armhf:0.5.1 *:9093->9093/tcp
|
|
||||||
q4bet4xs10pk func_gateway replicated 1/1 functions/gateway-armhf:0.6.0 *:8080->8080/tcp
|
|
||||||
v9vsvx73pszz func_nodeinfo replicated 1/1 functions/nodeinfo:latest-armhf
|
|
||||||
|
|
||||||
```
|
|
||||||
|
|
||||||
We want to see 1/1 listed on all of our services.
|
|
||||||
|
|
||||||
Given any service name you can type in the following to see which RPi it was scheduled to:
|
|
||||||
|
|
||||||
```
|
|
||||||
$ docker service ps func_markdown
|
|
||||||
ID IMAGE NODE STATE
|
|
||||||
func_markdown.1 functions/markdownrender:latest-armhf swarm4 Running
|
|
||||||
|
|
||||||
```
|
|
||||||
|
|
||||||
The state should be `Running` - if it says `Pending` then the image could still be on its way down from the internet.
|
|
||||||
|
|
||||||
At that point, find the IP address of your RPi and open that in a web-browser on port 8080:
|
|
||||||
|
|
||||||
```
|
|
||||||
$ ifconfig
|
|
||||||
|
|
||||||
```
|
|
||||||
|
|
||||||
For example if your IP was: 192.168.0.100 - then go to [http://192.168.0.100:8080][42]
|
|
||||||
|
|
||||||
At this point you should see the FaaS UI also called the API Gateway. This is where you can define, test and invoke your functions.
|
|
||||||
|
|
||||||
Click on the Markdown conversion function called func_markdown and type in some Markdown (this is what Wikipedia uses to write its content).
|
|
||||||
|
|
||||||
Then hit invoke. You'll see the invocation count go up and the bottom half of the screen shows the result of your function:
|
|
||||||
|
|
||||||
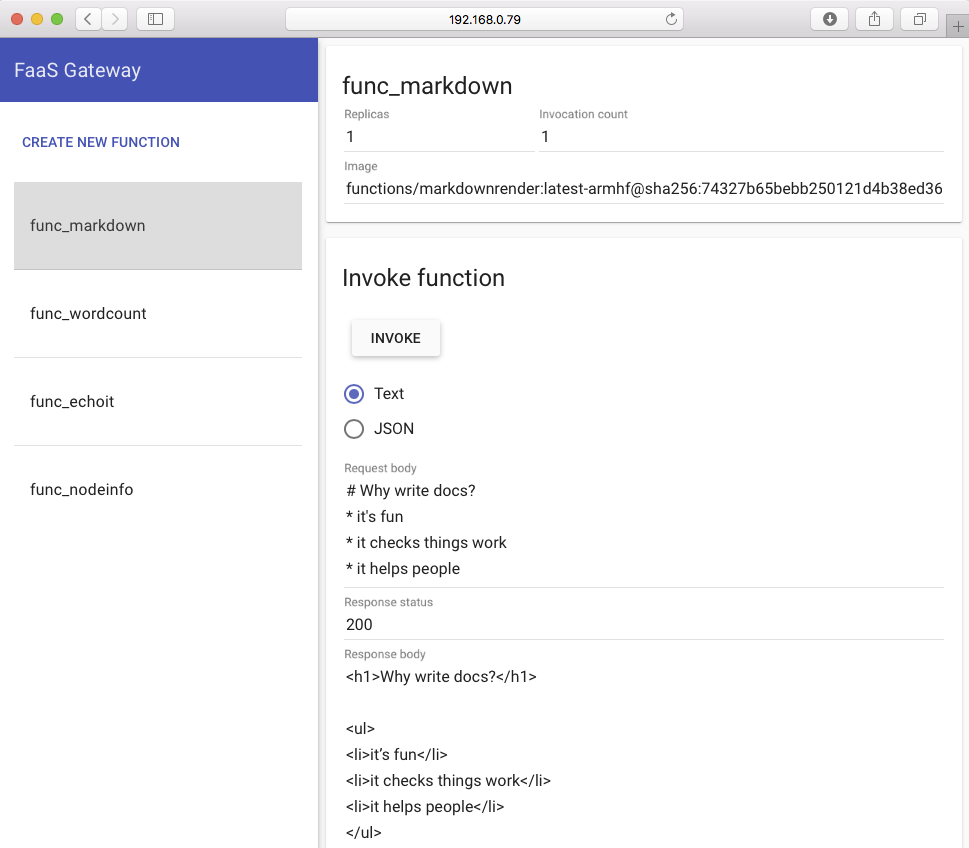
|
|
||||||
|
|
||||||
### Deploy your first serverless function:
|
|
||||||
|
|
||||||
There is already a tutorial written for this section, but we'll need to get the RPi set up with a couple of custom steps first.
|
|
||||||
|
|
||||||
* Get the FaaS-CLI
|
|
||||||
|
|
||||||
```
|
|
||||||
$ curl -sSL cli.openfaas.com | sudo sh
|
|
||||||
armv7l
|
|
||||||
Getting package https://github.com/alexellis/faas-cli/releases/download/0.4.5-b/faas-cli-armhf
|
|
||||||
|
|
||||||
```
|
|
||||||
|
|
||||||
* Clone the samples:
|
|
||||||
|
|
||||||
```
|
|
||||||
$ git clone https://github.com/alexellis/faas-cli
|
|
||||||
$ cd faas-cli
|
|
||||||
|
|
||||||
```
|
|
||||||
|
|
||||||
* Patch the samples for Raspberry Pi
|
|
||||||
|
|
||||||
We'll temporarily update our templates so they work with the Raspberry Pi:
|
|
||||||
|
|
||||||
```
|
|
||||||
$ cp template/node-armhf/Dockerfile template/node/
|
|
||||||
$ cp template/python-armhf/Dockerfile template/python/
|
|
||||||
|
|
||||||
```
|
|
||||||
|
|
||||||
The reason for doing this is that the Raspberry Pi has a different processor to most computers we interact with on a daily basis.
|
|
||||||
|
|
||||||
> Get up to speed on Docker on the Raspberry Pi - read: [5 Things you need to know][26]
|
|
||||||
|
|
||||||
Now you can follow the same tutorial written for PC, Laptop and Cloud available below, but we are going to run a couple of commands first for the Raspberry Pi.
|
|
||||||
|
|
||||||
* [Your first serverless Python function with OpenFaaS][27]
|
|
||||||
|
|
||||||
Pick it up at step 3:
|
|
||||||
|
|
||||||
* Instead of placing your functions in `~/functions/hello-python` - place them inside the `faas-cli` folder we just cloned from GitHub.
|
|
||||||
|
|
||||||
* Also replace "localhost" for the IP address of your first RPi in the `stack.yml`file.
|
|
||||||
|
|
||||||
Note that the Raspberry Pi may take a few minutes to download your serverless function to the relevant RPi. You can check on your services to make sure you have 1/1 replicas showing up with this command:
|
|
||||||
|
|
||||||
```
|
|
||||||
$ watch 'docker service ls'
|
|
||||||
pv27thj5lftz hello-python replicated 1/1 alexellis2/faas-hello-python-armhf:latest
|
|
||||||
|
|
||||||
```
|
|
||||||
|
|
||||||
**Continue the tutorial:** [Your first serverless Python function with OpenFaaS][43]
|
|
||||||
|
|
||||||
For more information on working with Node.js or other languages head over to the main [FaaS repo][44]
|
|
||||||
|
|
||||||
### Check your function metrics
|
|
||||||
|
|
||||||
With a Serverless experience, you don't want to spend all your time managing your functions. Fortunately [Prometheus][45] metrics are built into OpenFaaS meaning you can keep track of how long each functions takes to run and how often it's being called.
|
|
||||||
|
|
||||||
_Metrics drive auto-scaling_
|
|
||||||
|
|
||||||
If you generate enough load on any of of the functions then OpenFaaS will auto-scale your function and when the demand eases off you'll get back to a single replica again.
|
|
||||||
|
|
||||||
Here is a sample query you can paste into Safari, Chrome etc:
|
|
||||||
|
|
||||||
Just change the IP address to your own.
|
|
||||||
|
|
||||||
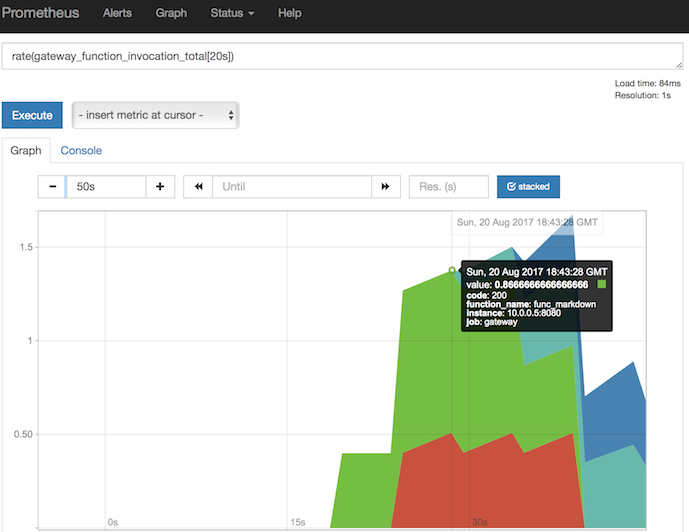
|
|
||||||
|
|
||||||
```
|
|
||||||
http://192.168.0.25:9090/graph?g0.range_input=15m&g0.stacked=1&g0.expr=rate(gateway_function_invocation_total%5B20s%5D)&g0.tab=0&g1.range_input=1h&g1.expr=gateway_service_count&g1.tab=0
|
|
||||||
|
|
||||||
```
|
|
||||||
|
|
||||||
The queries are written in PromQL - Prometheus query language. The first one shows us how often the function is being called:
|
|
||||||
|
|
||||||
```
|
|
||||||
rate(gateway_function_invocation_total[20s])
|
|
||||||
|
|
||||||
```
|
|
||||||
|
|
||||||
The second query shows us how many replicas we have of each function, there should be only one of each at the start:
|
|
||||||
|
|
||||||
```
|
|
||||||
gateway_service_count
|
|
||||||
|
|
||||||
```
|
|
||||||
|
|
||||||
If you want to trigger auto-scaling you could try the following on the RPi:
|
|
||||||
|
|
||||||
```
|
|
||||||
$ while [ true ]; do curl -4 localhost:8080/function/func_echoit --data "hello world" ; done
|
|
||||||
|
|
||||||
```
|
|
||||||
|
|
||||||
Check the Prometheus "alerts" page, and see if you are generating enough load for the auto-scaling to trigger, if you're not then run the command in a few additional Terminal windows too.
|
|
||||||
|
|
||||||
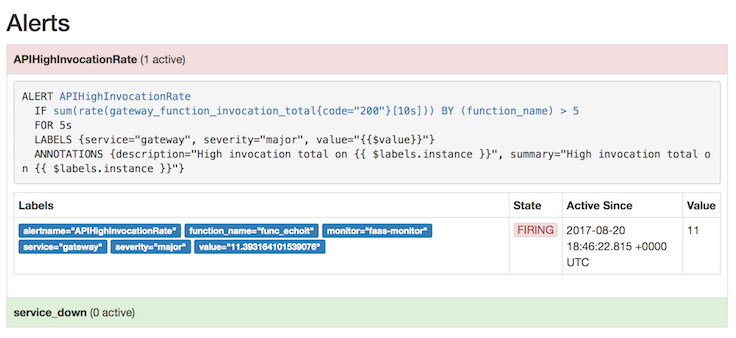
|
|
||||||
|
|
||||||
After you reduce the load, the replica count shown in your second graph and the `gateway_service_count` metric will go back to 1 again.
|
|
||||||
|
|
||||||
### Wrapping up
|
|
||||||
|
|
||||||
We've now set up Docker, Swarm and run OpenFaaS - which let us treat our Raspberry Pi like one giant computer - ready to crunch through code.
|
|
||||||
|
|
||||||
> Please show support for the project and **Star** the [FaaS GitHub repository][28]
|
|
||||||
|
|
||||||
How did you find setting up your Docker Swarm first cluster and running OpenFaaS? Please share a picture or a Tweet on Twitter [@alexellisuk][46]
|
|
||||||
|
|
||||||
**Watch my Dockercon video of OpenFaaS**
|
|
||||||
|
|
||||||
I presented OpenFaaS (then called FaaS) [at Dockercon in Austin][47] - watch this video for a high-level introduction and some really interactive demos Alexa and GitHub.
|
|
||||||
|
|
||||||
** 此处有iframe,请手动处理 **
|
|
||||||
|
|
||||||
Got questions? Ask in the comments below - or send your email over to me for an invite to my Raspberry Pi, Docker and Serverless Slack channel where you can chat with like-minded people about what you're working on.
|
|
||||||
|
|
||||||
**Want to learn more about Docker on the Raspberry Pi?**
|
|
||||||
|
|
||||||
I'd suggest starting with [5 Things you need to know][48] which covers things like security and and the subtle differences between RPi and a regular PC.
|
|
||||||
|
|
||||||
* [Dockercon tips: Docker & Raspberry Pi][18]
|
|
||||||
|
|
||||||
* [Control GPIO with Docker Swarm][19]
|
|
||||||
|
|
||||||
* [Is that a Docker Engine in your pocket??][20]
|
|
||||||
|
|
||||||
_Share on Twitter_
|
|
||||||
|
|
||||||
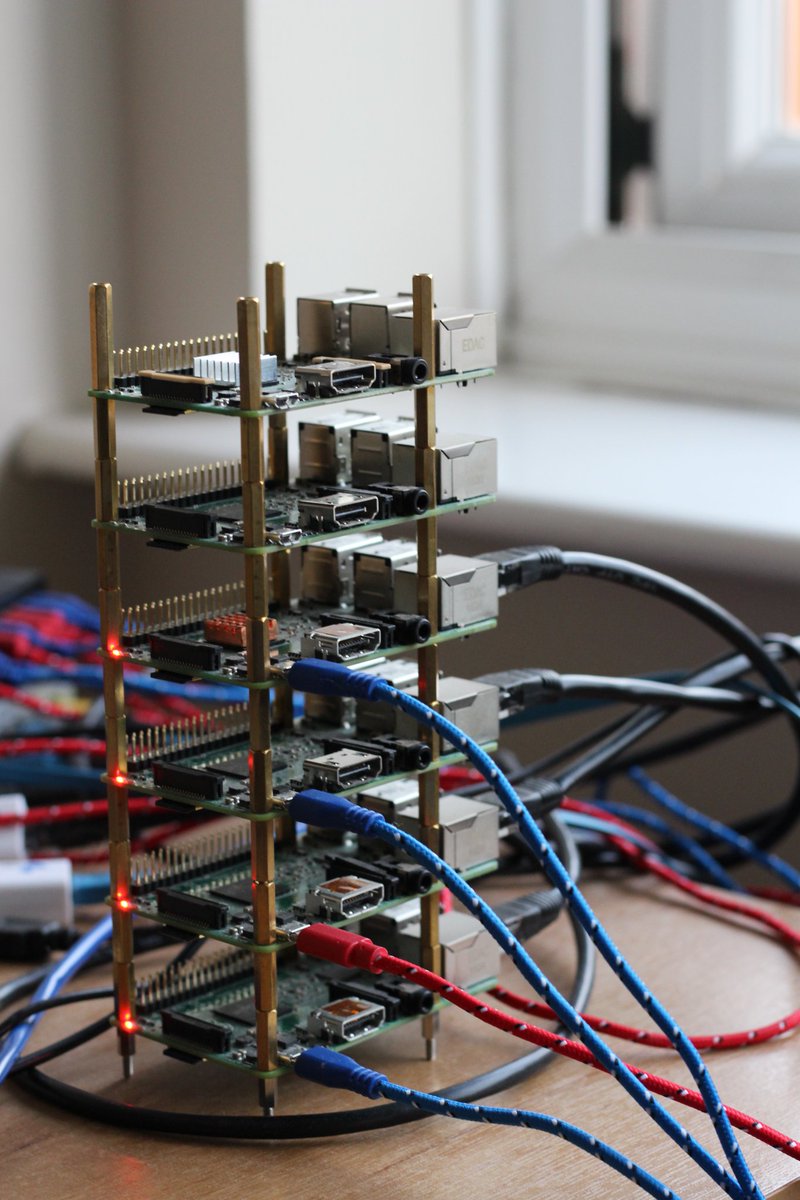
|
|
||||||
|
|
||||||
|
|
||||||
|
|
||||||
--------------------------------------------------------------------------------
|
|
||||||
|
|
||||||
via: https://blog.alexellis.io/your-serverless-raspberry-pi-cluster/
|
|
||||||
|
|
||||||
作者:[Alex Ellis ][a]
|
|
||||||
译者:[译者ID](https://github.com/译者ID)
|
|
||||||
校对:[校对者ID](https://github.com/校对者ID)
|
|
||||||
|
|
||||||
本文由 [LCTT](https://github.com/LCTT/TranslateProject) 原创编译,[Linux中国](https://linux.cn/) 荣誉推出
|
|
||||||
|
|
||||||
[a]:https://twitter.com/alexellisuk
|
|
||||||
[1]:https://twitter.com/alexellisuk
|
|
||||||
[2]:https://twitter.com/intent/tweet?in_reply_to=898978596773138436
|
|
||||||
[3]:https://twitter.com/intent/retweet?tweet_id=898978596773138436
|
|
||||||
[4]:https://twitter.com/intent/like?tweet_id=898978596773138436
|
|
||||||
[5]:https://twitter.com/alexellisuk
|
|
||||||
[6]:https://twitter.com/alexellisuk
|
|
||||||
[7]:https://twitter.com/Docker
|
|
||||||
[8]:https://twitter.com/Raspberry_Pi
|
|
||||||
[9]:https://twitter.com/alexellisuk/status/898978596773138436
|
|
||||||
[10]:https://twitter.com/alexellisuk/status/899545370916728832/photo/1
|
|
||||||
[11]:https://twitter.com/alexellisuk
|
|
||||||
[12]:https://twitter.com/alexellisuk/status/898978596773138436/photo/1
|
|
||||||
[13]:https://twitter.com/alexellisuk/status/898978596773138436/photo/1
|
|
||||||
[14]:https://twitter.com/alexellisuk/status/898978596773138436/photo/1
|
|
||||||
[15]:https://twitter.com/alexellisuk/status/898978596773138436/photo/1
|
|
||||||
[16]:https://twitter.com/alexellisuk/status/899545370916728832/photo/1
|
|
||||||
[17]:https://support.twitter.com/articles/20175256
|
|
||||||
[18]:https://blog.alexellis.io/dockercon-tips-docker-raspberry-pi/
|
|
||||||
[19]:https://blog.alexellis.io/gpio-on-swarm/
|
|
||||||
[20]:https://blog.alexellis.io/docker-engine-in-your-pocket/
|
|
||||||
[21]:https://news.ycombinator.com/item?id=15052192
|
|
||||||
[22]:https://blog.alexellis.io/introducing-functions-as-a-service/
|
|
||||||
[23]:https://github.com/alexellis/faas
|
|
||||||
[24]:http://downloads.raspberrypi.org/raspbian_lite/images/raspbian_lite-2017-07-05/
|
|
||||||
[25]:https://github.com/alexellis/faas
|
|
||||||
[26]:https://blog.alexellis.io/5-things-docker-rpi/
|
|
||||||
[27]:https://blog.alexellis.io/first-faas-python-function
|
|
||||||
[28]:https://github.com/alexellis/faas
|
|
||||||
[29]:https://blog.alexellis.io/tag/docker/
|
|
||||||
[30]:https://blog.alexellis.io/tag/raspberry-pi/
|
|
||||||
[31]:https://blog.alexellis.io/tag/openfaas/
|
|
||||||
[32]:https://blog.alexellis.io/tag/faas/
|
|
||||||
[33]:https://github.com/alexellis/faas
|
|
||||||
[34]:https://blog.alexellis.io/introducing-functions-as-a-service/
|
|
||||||
[35]:https://github.com/alexellis/faas
|
|
||||||
[36]:https://github.com/alexellis/faas
|
|
||||||
[37]:https://www.amazon.co.uk/Anker-PowerPort-Family-Sized-Technology-Smartphones/dp/B00PK1IIJY
|
|
||||||
[38]:http://downloads.raspberrypi.org/raspbian/images/raspbian-2017-07-05/
|
|
||||||
[39]:https://etcher.io/
|
|
||||||
[40]:https://docs.docker.com/engine/swarm/admin_guide/
|
|
||||||
[41]:https://github.com/alexellis/faas
|
|
||||||
[42]:http://192.168.0.100:8080/
|
|
||||||
[43]:https://blog.alexellis.io/first-faas-python-function
|
|
||||||
[44]:https://github.com/alexellis/faas
|
|
||||||
[45]:https://prometheus.io/
|
|
||||||
[46]:https://twitter.com/alexellisuk
|
|
||||||
[47]:https://blog.alexellis.io/dockercon-2017-captains-log/
|
|
||||||
[48]:https://blog.alexellis.io/5-things-docker-rpi/
|
|
@ -1,3 +1,4 @@
|
|||||||
|
zpl1025 translating
|
||||||
12 Practices every Android Development Beginner should know — Part 1
|
12 Practices every Android Development Beginner should know — Part 1
|
||||||
============================================================
|
============================================================
|
||||||
|
|
||||||
|
@ -1,47 +0,0 @@
|
|||||||
polebug is translating
|
|
||||||
|
|
||||||
PostgreSQL's Hash Indexes Are Now Cool
|
|
||||||
=======
|
|
||||||
Since I just committed the last pending patch to improve hash indexes to PostgreSQL 11, and since most of the improvements to hash indexes were committed to PostgreSQL 10 which is expected to be released next week, it seems like a good time for a brief review of all the work that has been done over the last 18 months or so. Prior to version 10, hash indexes didn't perform well under concurrency, lacked write-ahead logging and thus were not safe in the face either of crashes or of replication, and were in various other ways second-class citizens. In PostgreSQL 10, this is largely fixed.
|
|
||||||
|
|
||||||
While I was involved in some of the design, credit for the hash index improvements goes first and foremost to my colleague Amit Kapila, whose [blog entry on this topic is worth reading][1]. The problem with hash indexes wasn't simply that nobody had bothered to write the code for write-ahead logging, but that the code was not structured in a way that made it possible to add write-ahead logging that would actually work correctly. To split a bucket, the system would lock the existing bucket (using a fairly inefficient locking mechanism), move half the tuples to the new bucket, compact the existing bucket, and release the lock. Even if the individual changes had been logged, a crash at the wrong time could have left the index in a corrupted state. So, Amit's first step was to redesign the locking mechanism. The [new mechanism][2] allows scans and splits to proceed in parallel to some degree, and allows a split interrupted by an error or crash to be completed at a later time. Once that was done a bunch of resulting bugs fixed, and some refactoring work completed, another patch from Amit added [write-ahead logging support for hash indexes][3].
|
|
||||||
|
|
||||||
In the meantime, it was discovered that hash indexes had missed out on many fairly obvious performance improvements which had been applied to btree over the years. Because hash indexes had no support for write-ahead logging, and because the old locking mechanism was so ponderous, there wasn't much motivation to make other performance improvements either. However, this meant that if hash indexes were to become a really useful technology, there was more to do than just add write-ahead logging. PostgreSQL's index access method abstraction layer allows indexes to retain a backend-private cache of information about the index so that the index itself need not be repeatedly consulted to obtain relevant metadata. btree and spgist indexes were using this mechanism, but hash indexes were not, so my colleague Mithun Cy wrote a patch to [cache the hash index's metapage using this mechanism][4]. Similarly, btree indexes have an optimization called "single page vacuum" which opportunistically removes dead index pointers from index pages, preventing a huge amount of index bloat which would otherwise occur. My colleague Ashutosh Sharma wrote a patch to [port this logic over to hash indexes][5], dramatically reducing index bloat there as well. Finally, btree indexes have since 2006 had a feature to avoid locking and unlocking the same index page repeatedly -- instead, all tuples are slurped from the page in one shot and then returned one at a time. Ashutosh Sharma also [ported this logic to hash indexes][6], but that optimization didn't make it into v10 for lack of time. Of everything mentioned in this blog entry, this is the only improvement that won't show up until v11.
|
|
||||||
|
|
||||||
One of the more interesting aspects of the hash index work was the difficulty of determining whether the behavior was in fact correct. Changes to locking behavior may fail only under heavy concurrency, while a bug in write-ahead logging will probably only manifest in the case of crash recovery. Furthermore, in each case, the problems may be subtle. It's not enough that things run without crashing; they must also produce the right answer in all cases, and this can be difficult to verify. To assist in that task, my colleague Kuntal Ghosh followed up on work initially begun by Heikki Linnakangas and Michael Paquier and produced a WAL consistency checker that could be used not only as a private patch for developer testing but actually [committed to PostgreSQL][7]. The write-ahead logging code for hash indexes was extensively tested using this tool prior to commit, and it was very successful at finding bugs. The tool is not limited to hash indexes, though: it can be used to validate the write-ahead logging code for other modules as well, including the heap, all index AMs we have today, and other things that are developed in the future. In fact, it already succeeded in [finding a bug in BRIN][8].
|
|
||||||
|
|
||||||
While wal_consistency_checking is primarily a developer tool -- though it is suitable for use by users as well if a bug is suspected -- upgrades were also made to several tools intended for DBAs. Jesper Pedersen wrote a patch to [upgrade the pageinspect contrib module with support for hash indexes][9], on which Ashutosh Sharma did further work and to which Peter Eisentraut contributed test cases (which was a really good idea, since those test cases promptly failed, provoking several rounds of bug-fixing). The pgstattuple contrib module also [got support for hash indexes][10], due to work by Ashutosh Sharma.
|
|
||||||
|
|
||||||
Along the way, there were a few other performance improvements as well. One thing that I had not realized at the outset is that when a hash index begins a new round of bucket splits, the size on disk tended to abruptly double, which is not really a problem for a 1MB index but is a bit unfortunate if you happen to have a 64GB index. Mithun Cy addressed this problem to a degree by writing a patch to allow the doubling to be [divided into four stages][11], meaning that we'll go from 64GB to 80GB to 96GB to 112GB to 128GB instead of going from 64GB to 128GB in one shot. This could be improved further, but it would require deeper restructuring of the on-disk format and some careful thinking about the effects on lookup performance.
|
|
||||||
|
|
||||||
A report from [a tester who goes by "AP"][12] in July tipped us off to the need for a few further tweaks. AP found that trying to insert 2 billion rows into a newly-created hash index was causing an error. To address that problem, Amit modified the bucket split code to [attempt a cleanup of the old bucket just after each split][13], which greatly reduced the accumulation of overflow pages. Just to be sure, Amit and I also [increased the maximum number of bitmap pages][14], which are used to track overflow page allocations, by a factor of four.
|
|
||||||
|
|
||||||
While there's [always more that can be done][15], I feel that my colleagues and I -- with help from others in the PostgreSQL community -- have accomplished our goal of making hash indexes into a first-class feature rather than a badly neglected half-feature. One may well ask, however, what the use case for that feature may be. The blog entry from Amit to which I referred (and linked) at the beginning of this post shows that even for a pgbench workload, it's possible for a hash index to outperform btree at both low and high levels of concurrency. However, in some sense, that's really a worst case. One of the selling points of hash indexes is that the index stores the hash value, not the actual indexed value - so I expect that the improvements will be larger for wide keys, such as UUIDs or long strings. They will likely to do better on read-heavy workloads, as we have not optimized writes to the same degree as reads, but I would encourage anyone who is interested in this technology to try it out and post results to the mailing list (or send private email), because the real key for a feature like this is not what some developer thinks will happen in the lab but what actually does happen in the field.
|
|
||||||
|
|
||||||
In closing, I'd like to thank Jeff Janes and Jesper Pedersen for their invaluable testing work, both related to this project and in general. Getting a project of this magnitude correct is not simple, and having persistent testers who are determined to break whatever can be broken is a great help. Others not already mentioned who deserve credit for testing, review, and general help of various sorts include Andreas Seltenreich, Dilip Kumar, Tushar Ahuja, Álvaro Herrera, Michael Paquier, Mark Kirkwood, Tom Lane, and Kyotaro Horiguchi. Thank you, and thanks as well to anyone whose work should have been mentioned here but which I have inadvertently omitted.
|
|
||||||
|
|
||||||
---
|
|
||||||
via:https://rhaas.blogspot.jp/2017/09/postgresqls-hash-indexes-are-now-cool.html?showComment=1507079869582#c6521238465677174123
|
|
||||||
|
|
||||||
作者:[作者名][a]
|
|
||||||
译者:[译者ID](https://github.com/id)
|
|
||||||
校对:[校对者ID](https://github.com/id)
|
|
||||||
本文由[LCTT](https://github.com/LCTT/TranslateProject)原创编译,[Linux中国](https://linux.cn/)荣誉推出
|
|
||||||
|
|
||||||
|
|
||||||
[a]:http://rhaas.blogspot.jp
|
|
||||||
[1]:http://amitkapila16.blogspot.jp/2017/03/hash-indexes-are-faster-than-btree.html
|
|
||||||
[2]:https://git.postgresql.org/gitweb/?p=postgresql.git;a=commitdiff;h=6d46f4783efe457f74816a75173eb23ed8930020
|
|
||||||
[3]:https://git.postgresql.org/gitweb/?p=postgresql.git;a=commitdiff;h=c11453ce0aeaa377cbbcc9a3fc418acb94629330
|
|
||||||
[4]:https://git.postgresql.org/gitweb/?p=postgresql.git;a=commitdiff;h=293e24e507838733aba4748b514536af2d39d7f2
|
|
||||||
[5]:https://git.postgresql.org/gitweb/?p=postgresql.git;a=commitdiff;h=6977b8b7f4dfb40896ff5e2175cad7fdbda862eb
|
|
||||||
[6]:https://git.postgresql.org/gitweb/?p=postgresql.git;a=commitdiff;h=7c75ef571579a3ad7a1d3ee909f11dba5e0b9440
|
|
||||||
[7]:https://git.postgresql.org/gitweb/?p=postgresql.git;a=commitdiff;h=a507b86900f695aacc8d52b7d2cfcb65f58862a2
|
|
||||||
[8]:https://git.postgresql.org/gitweb/?p=postgresql.git;a=commitdiff;h=7403561c0f6a8c62b79b6ddf0364ae6c01719068
|
|
||||||
[9]:https://git.postgresql.org/gitweb/?p=postgresql.git;a=commitdiff;h=08bf6e529587e1e9075d013d859af2649c32a511
|
|
||||||
[10]:https://git.postgresql.org/gitweb/?p=postgresql.git;a=commitdiff;h=e759854a09d49725a9519c48a0d71a32bab05a01
|
|
||||||
[11]:https://git.postgresql.org/gitweb/?p=postgresql.git;a=commitdiff;h=ea69a0dead5128c421140dc53fac165ba4af8520
|
|
||||||
[12]:https://www.postgresql.org/message-id/20170704105728.mwb72jebfmok2nm2@zip.com.au
|
|
||||||
[13]:https://git.postgresql.org/gitweb/?p=postgresql.git;a=commitdiff;h=ff98a5e1e49de061600feb6b4de5ce0a22d386af
|
|
||||||
[14]:https://git.postgresql.org/gitweb/?p=postgresql.git;a=commitdiff;h=ff98a5e1e49de061600feb6b4de5ce0a22d386af
|
|
||||||
[15]:https://www.postgresql.org/message-id/CA%2BTgmoax6DhnKsuE_gzY5qkvmPEok77JAP1h8wOTbf%2Bdg2Ycrw%40mail.gmail.com
|
|
@ -1,300 +0,0 @@
|
|||||||
[Concurrent Servers: Part 2 - Threads][19]
|
|
||||||
============================================================
|
|
||||||
|
|
||||||
GitFuture is Translating
|
|
||||||
|
|
||||||
This is part 2 of a series on writing concurrent network servers. [Part 1][20] presented the protocol implemented by the server, as well as the code for a simple sequential server, as a baseline for the series.
|
|
||||||
|
|
||||||
In this part, we're going to look at multi-threading as one approach to concurrency, with a bare-bones threaded server implementation in C, as well as a thread pool based implementation in Python.
|
|
||||||
|
|
||||||
All posts in the series:
|
|
||||||
|
|
||||||
* [Part 1 - Introduction][8]
|
|
||||||
|
|
||||||
* [Part 2 - Threads][9]
|
|
||||||
|
|
||||||
* [Part 3 - Event-driven][10]
|
|
||||||
|
|
||||||
### The multi-threaded approach to concurrent server design
|
|
||||||
|
|
||||||
When discussing the performance of the sequential server in part 1, it was immediately obvious that a lot of compute resources are wasted while the server processes a client connection. Even assuming a client that sends messages immediately and doesn't do any waiting, network communication is still involved; networks tend to be millions (or more) times slower than a modern CPU, so the CPU running the sequential server will spend the vast majority of time in gloriuos boredom waiting for new socket traffic to arrive.
|
|
||||||
|
|
||||||
Here's a chart showing how sequential client processing happens over time:
|
|
||||||
|
|
||||||
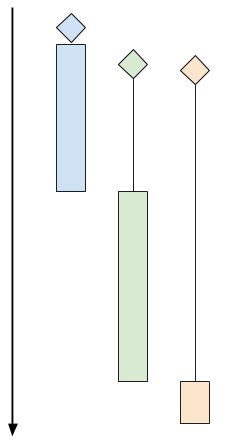
|
|
||||||
|
|
||||||
The diagrams shows 3 clients. The diamond shapes denote the client's "arrival time" (the time at which the client attempted to connect to the server). The black lines denote "wait time" (the time clients spent waiting for the server to actually accept their connection), and the colored bars denote actual "processing time" (the time server and client are interacting using the protocol). At the end of the colored bar, the client disconnects.
|
|
||||||
|
|
||||||
In the diagram above, even though the green and orange clients arrived shortly after the blue one, they have to wait for a while until the server is done with the blue client. At this point the green client is accepted, while the orange one has to wait even longer.
|
|
||||||
|
|
||||||
A multi-threaded server would launch multiple control threads, letting the OS manage concurrency on the CPU (and across multiple CPU cores). When a client connects, a thread is created to serve it, while the server is ready to accept more clients in the main thread. The time chart for this mode looks like the following:
|
|
||||||
|
|
||||||
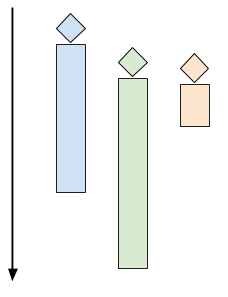
|
|
||||||
|
|
||||||
### One thread per client, in C using pthreads
|
|
||||||
|
|
||||||
Our [first code sample][11] in this post is a simple "one thread per client" server, written in C using the foundational [pthreads API][12] for multi-threading. Here's the main loop:
|
|
||||||
|
|
||||||
```
|
|
||||||
while (1) {
|
|
||||||
struct sockaddr_in peer_addr;
|
|
||||||
socklen_t peer_addr_len = sizeof(peer_addr);
|
|
||||||
|
|
||||||
int newsockfd =
|
|
||||||
accept(sockfd, (struct sockaddr*)&peer_addr, &peer_addr_len);
|
|
||||||
|
|
||||||
if (newsockfd < 0) {
|
|
||||||
perror_die("ERROR on accept");
|
|
||||||
}
|
|
||||||
|
|
||||||
report_peer_connected(&peer_addr, peer_addr_len);
|
|
||||||
pthread_t the_thread;
|
|
||||||
|
|
||||||
thread_config_t* config = (thread_config_t*)malloc(sizeof(*config));
|
|
||||||
if (!config) {
|
|
||||||
die("OOM");
|
|
||||||
}
|
|
||||||
config->sockfd = newsockfd;
|
|
||||||
pthread_create(&the_thread, NULL, server_thread, config);
|
|
||||||
|
|
||||||
// Detach the thread - when it's done, its resources will be cleaned up.
|
|
||||||
// Since the main thread lives forever, it will outlive the serving threads.
|
|
||||||
pthread_detach(the_thread);
|
|
||||||
}
|
|
||||||
```
|
|
||||||
|
|
||||||
And this is the `server_thread` function:
|
|
||||||
|
|
||||||
```
|
|
||||||
void* server_thread(void* arg) {
|
|
||||||
thread_config_t* config = (thread_config_t*)arg;
|
|
||||||
int sockfd = config->sockfd;
|
|
||||||
free(config);
|
|
||||||
|
|
||||||
// This cast will work for Linux, but in general casting pthread_id to an
|
|
||||||
// integral type isn't portable.
|
|
||||||
unsigned long id = (unsigned long)pthread_self();
|
|
||||||
printf("Thread %lu created to handle connection with socket %d\n", id,
|
|
||||||
sockfd);
|
|
||||||
serve_connection(sockfd);
|
|
||||||
printf("Thread %lu done\n", id);
|
|
||||||
return 0;
|
|
||||||
}
|
|
||||||
```
|
|
||||||
|
|
||||||
The thread "configuration" is passed as a `thread_config_t` structure:
|
|
||||||
|
|
||||||
```
|
|
||||||
typedef struct { int sockfd; } thread_config_t;
|
|
||||||
```
|
|
||||||
|
|
||||||
The `pthread_create` call in the main loop launches a new thread that runs the `server_thread` function. This thread terminates when `server_thread` returns. In turn, `server_thread` returns when `serve_connection` returns.`serve_connection` is exactly the same function from part 1.
|
|
||||||
|
|
||||||
In part 1 we used a script to launch multiple clients concurrently and observe how the server handles them. Let's do the same with the multithreaded server:
|
|
||||||
|
|
||||||
```
|
|
||||||
$ python3.6 simple-client.py -n 3 localhost 9090
|
|
||||||
INFO:2017-09-20 06:31:56,632:conn1 connected...
|
|
||||||
INFO:2017-09-20 06:31:56,632:conn2 connected...
|
|
||||||
INFO:2017-09-20 06:31:56,632:conn0 connected...
|
|
||||||
INFO:2017-09-20 06:31:56,632:conn1 sending b'^abc$de^abte$f'
|
|
||||||
INFO:2017-09-20 06:31:56,632:conn2 sending b'^abc$de^abte$f'
|
|
||||||
INFO:2017-09-20 06:31:56,632:conn0 sending b'^abc$de^abte$f'
|
|
||||||
INFO:2017-09-20 06:31:56,633:conn1 received b'b'
|
|
||||||
INFO:2017-09-20 06:31:56,633:conn2 received b'b'
|
|
||||||
INFO:2017-09-20 06:31:56,633:conn0 received b'b'
|
|
||||||
INFO:2017-09-20 06:31:56,670:conn1 received b'cdbcuf'
|
|
||||||
INFO:2017-09-20 06:31:56,671:conn0 received b'cdbcuf'
|
|
||||||
INFO:2017-09-20 06:31:56,671:conn2 received b'cdbcuf'
|
|
||||||
INFO:2017-09-20 06:31:57,634:conn1 sending b'xyz^123'
|
|
||||||
INFO:2017-09-20 06:31:57,634:conn2 sending b'xyz^123'
|
|
||||||
INFO:2017-09-20 06:31:57,634:conn1 received b'234'
|
|
||||||
INFO:2017-09-20 06:31:57,634:conn0 sending b'xyz^123'
|
|
||||||
INFO:2017-09-20 06:31:57,634:conn2 received b'234'
|
|
||||||
INFO:2017-09-20 06:31:57,634:conn0 received b'234'
|
|
||||||
INFO:2017-09-20 06:31:58,635:conn1 sending b'25$^ab0000$abab'
|
|
||||||
INFO:2017-09-20 06:31:58,635:conn2 sending b'25$^ab0000$abab'
|
|
||||||
INFO:2017-09-20 06:31:58,636:conn1 received b'36bc1111'
|
|
||||||
INFO:2017-09-20 06:31:58,636:conn2 received b'36bc1111'
|
|
||||||
INFO:2017-09-20 06:31:58,637:conn0 sending b'25$^ab0000$abab'
|
|
||||||
INFO:2017-09-20 06:31:58,637:conn0 received b'36bc1111'
|
|
||||||
INFO:2017-09-20 06:31:58,836:conn2 disconnecting
|
|
||||||
INFO:2017-09-20 06:31:58,836:conn1 disconnecting
|
|
||||||
INFO:2017-09-20 06:31:58,837:conn0 disconnecting
|
|
||||||
```
|
|
||||||
|
|
||||||
Indeed, all clients connected at the same time, and their communication with the server occurs concurrently.
|
|
||||||
|
|
||||||
### Challenges with one thread per client
|
|
||||||
|
|
||||||
Even though threads are fairly efficient in terms of resource usage on modern OSes, the approach outlined in the previous section can still present challenges with some workloads.
|
|
||||||
|
|
||||||
Imagine a scenario where many clients are connecting simultaneously, and some of the sessions are long-lived. This means that many threads may be active at the same time in the server. Too many threads can consume a large amount of memory and CPU time just for the context switching [[1]][13]. An alternative way to look at it is as a security problem: this design makes it the server an easy target for a [DoS attack][14] - connect a few 100,000s of clients at the same time and let them all sit idle - this will likely kill the server due to excessive resource usage.
|
|
||||||
|
|
||||||
A larger problem occurs when there's a non-trivial amount of CPU-bound computation the server has to do for each client. In this case, swamping the server is considerably easier - just a few dozen clients can bring a server to its knees.
|
|
||||||
|
|
||||||
For these reasons, it's prudent the do some _rate-limiting_ on the number of concurrent clients handled by a multi-threaded server. There's a number of ways to do this. The simplest that comes to mind is simply count the number of clients currently connected and restrict that number to some quantity (that was determined by careful benchmarking, hopefully). A variation on this approach that's very popular in concurrent application design is using a _thread pool_ .
|
|
||||||
|
|
||||||
### Thread pools
|
|
||||||
|
|
||||||
The idea of a [thread pool][15] is simple, yet powerful. The server creates a number of working threads that all expect to get tasks from some queue. This is the "pool". Then, each client connection is dispatched as a task to the pool. As long as there's an idle thread in the pool, it's handed the task. If all the threads in the pool are currently busy, the server blocks until the pool accepts the task (which happens after one of the busy threads finished processing its current task and went back to an idle state).
|
|
||||||
|
|
||||||
Here's a diagram showing a pool of 4 threads, each processing a task. Tasks (client connections in our case) are waiting until one of the threads in the pool is ready to accept new tasks.
|
|
||||||
|
|
||||||
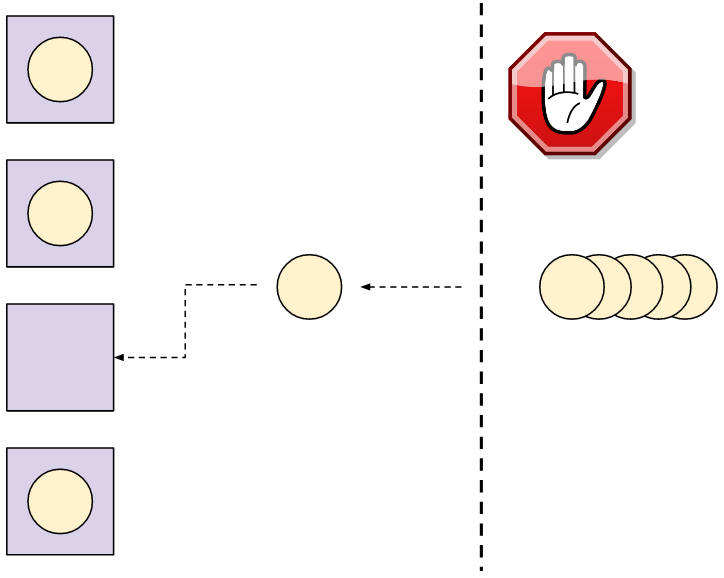
|
|
||||||
|
|
||||||
It should be fairly obvious that the thread pool approach provides a rate-limiting mechanism in its very definition. We can decide ahead of time how many threads we want our server to have. Then, this is the maximal number of clients processed concurrently - the rest are waiting until one of the threads becomes free. If we have 8 threads in the pool, 8 is the maximal number of concurrent clients the server handles - even if thousands are attempting to connect simultaneously.
|
|
||||||
|
|
||||||
How do we decide how many threads should be in the pool? By a careful analysis of the problem domain, benchmarking, experimentation and also by the HW we have. If we have a single-core cloud instance that's one answer, if we have a 100-core dual socket server available, the answer is different. Picking the thread pool size can also be done dynamically at runtime based on load - I'll touch upon this topic in future posts in this series.
|
|
||||||
|
|
||||||
Servers that use thread pools manifest _graceful degradation_ in the face of high load - clients are accepted at some steady rate, potentially slower than their rate of arrival for some periods of time; that said, no matter how many clients are trying to connect simultaneously, the server will remain responsive and will just churn through the backlog of clients to its best ability. Contrast this with the one-thread-per-client server which can merrily accept a large number of clients until it gets overloaded, at which point it's likely to either crash or start working very slowly for _all_ processed clients due to resource exhaustion (such as virtual memory thrashing).
|
|
||||||
|
|
||||||
### Using a thread pool for our network server
|
|
||||||
|
|
||||||
For [this variation of the server][16] I've switched to Python, which comes with a robust implementation of a thread pool in the standard library (`ThreadPoolExecutor` from the `concurrent.futures` module) [[2]][17].
|
|
||||||
|
|
||||||
This server creates a thread pool, then loops to accept new clients on the main listening socket. Each connected client is dispatched into the pool with `submit`:
|
|
||||||
|
|
||||||
```
|
|
||||||
pool = ThreadPoolExecutor(args.n)
|
|
||||||
sockobj = socket.socket(socket.AF_INET, socket.SOCK_STREAM)
|
|
||||||
sockobj.setsockopt(socket.SOL_SOCKET, socket.SO_REUSEADDR, 1)
|
|
||||||
sockobj.bind(('localhost', args.port))
|
|
||||||
sockobj.listen(15)
|
|
||||||
|
|
||||||
try:
|
|
||||||
while True:
|
|
||||||
client_socket, client_address = sockobj.accept()
|
|
||||||
pool.submit(serve_connection, client_socket, client_address)
|
|
||||||
except KeyboardInterrupt as e:
|
|
||||||
print(e)
|
|
||||||
sockobj.close()
|
|
||||||
```
|
|
||||||
|
|
||||||
The `serve_connection` function is very similar to its C counterpart, serving a single client until the client disconnects, while following our protocol:
|
|
||||||
|
|
||||||
```
|
|
||||||
ProcessingState = Enum('ProcessingState', 'WAIT_FOR_MSG IN_MSG')
|
|
||||||
|
|
||||||
def serve_connection(sockobj, client_address):
|
|
||||||
print('{0} connected'.format(client_address))
|
|
||||||
sockobj.sendall(b'*')
|
|
||||||
state = ProcessingState.WAIT_FOR_MSG
|
|
||||||
|
|
||||||
while True:
|
|
||||||
try:
|
|
||||||
buf = sockobj.recv(1024)
|
|
||||||
if not buf:
|
|
||||||
break
|
|
||||||
except IOError as e:
|
|
||||||
break
|
|
||||||
for b in buf:
|
|
||||||
if state == ProcessingState.WAIT_FOR_MSG:
|
|
||||||
if b == ord(b'^'):
|
|
||||||
state = ProcessingState.IN_MSG
|
|
||||||
elif state == ProcessingState.IN_MSG:
|
|
||||||
if b == ord(b'$'):
|
|
||||||
state = ProcessingState.WAIT_FOR_MSG
|
|
||||||
else:
|
|
||||||
sockobj.send(bytes([b + 1]))
|
|
||||||
else:
|
|
||||||
assert False
|
|
||||||
|
|
||||||
print('{0} done'.format(client_address))
|
|
||||||
sys.stdout.flush()
|
|
||||||
sockobj.close()
|
|
||||||
```
|
|
||||||
|
|
||||||
Let's see how the thread pool size affects the blocking behavior for multiple concurrent clients. For demonstration purposes, I'll run the threadpool server with a pool size of 2 (only two threads are created to service clients):
|
|
||||||
|
|
||||||
```
|
|
||||||
$ python3.6 threadpool-server.py -n 2
|
|
||||||
```
|
|
||||||
|
|
||||||
And in a separate terminal, let's run the client simulator again, with 3 concurrent clients:
|
|
||||||
|
|
||||||
```
|
|
||||||
$ python3.6 simple-client.py -n 3 localhost 9090
|
|
||||||
INFO:2017-09-22 05:58:52,815:conn1 connected...
|
|
||||||
INFO:2017-09-22 05:58:52,827:conn0 connected...
|
|
||||||
INFO:2017-09-22 05:58:52,828:conn1 sending b'^abc$de^abte$f'
|
|
||||||
INFO:2017-09-22 05:58:52,828:conn0 sending b'^abc$de^abte$f'
|
|
||||||
INFO:2017-09-22 05:58:52,828:conn1 received b'b'
|
|
||||||
INFO:2017-09-22 05:58:52,828:conn0 received b'b'
|
|
||||||
INFO:2017-09-22 05:58:52,867:conn1 received b'cdbcuf'
|
|
||||||
INFO:2017-09-22 05:58:52,867:conn0 received b'cdbcuf'
|
|
||||||
INFO:2017-09-22 05:58:53,829:conn1 sending b'xyz^123'
|
|
||||||
INFO:2017-09-22 05:58:53,829:conn0 sending b'xyz^123'
|
|
||||||
INFO:2017-09-22 05:58:53,830:conn1 received b'234'
|
|
||||||
INFO:2017-09-22 05:58:53,831:conn0 received b'2'
|
|
||||||
INFO:2017-09-22 05:58:53,831:conn0 received b'34'
|
|
||||||
INFO:2017-09-22 05:58:54,831:conn1 sending b'25$^ab0000$abab'
|
|
||||||
INFO:2017-09-22 05:58:54,832:conn1 received b'36bc1111'
|
|
||||||
INFO:2017-09-22 05:58:54,832:conn0 sending b'25$^ab0000$abab'
|
|
||||||
INFO:2017-09-22 05:58:54,833:conn0 received b'36bc1111'
|
|
||||||
INFO:2017-09-22 05:58:55,032:conn1 disconnecting
|
|
||||||
INFO:2017-09-22 05:58:55,032:conn2 connected...
|
|
||||||
INFO:2017-09-22 05:58:55,033:conn2 sending b'^abc$de^abte$f'
|
|
||||||
INFO:2017-09-22 05:58:55,033:conn0 disconnecting
|
|
||||||
INFO:2017-09-22 05:58:55,034:conn2 received b'b'
|
|
||||||
INFO:2017-09-22 05:58:55,071:conn2 received b'cdbcuf'
|
|
||||||
INFO:2017-09-22 05:58:56,036:conn2 sending b'xyz^123'
|
|
||||||
INFO:2017-09-22 05:58:56,036:conn2 received b'234'
|
|
||||||
INFO:2017-09-22 05:58:57,037:conn2 sending b'25$^ab0000$abab'
|
|
||||||
INFO:2017-09-22 05:58:57,038:conn2 received b'36bc1111'
|
|
||||||
INFO:2017-09-22 05:58:57,238:conn2 disconnecting
|
|
||||||
```
|
|
||||||
|
|
||||||
Recall the behavior of previously discussed servers:
|
|
||||||
|
|
||||||
1. In the sequential server, all connections were serialized. One finished, and only then the next started.
|
|
||||||
|
|
||||||
2. In the thread-per-client server earlier in this post, all connections wer accepted and serviced concurrently.
|
|
||||||
|
|
||||||
Here we see another possibility: two connections are serviced concurrently, and only when one of them is done the third is admitted. This is a direct result of the thread pool size set to 2\. For a more realistic use case we'd set the thread pool size to much higher, depending on the machine and the exact protocol. This buffering behavior of thread pools is well understood - I've written about it more in detail [just a few months ago][18] in the context of Clojure's `core.async` module.
|
|
||||||
|
|
||||||
### Summary and next steps
|
|
||||||
|
|
||||||
This post discusses multi-threading as a means of concurrency in network servers. The one-thread-per-client approach is presented for an initial discussion, but this method is not common in practice since it's a security hazard.
|
|
||||||
|
|
||||||
Thread pools are much more common, and most popular programming languages have solid implementations (for some, like Python, it's in the standard library). The thread pool server presented here doesn't suffer from the problems of one-thread-per-client.
|
|
||||||
|
|
||||||
However, threads are not the only way to handle multiple clients concurrently. In the next post we're going to look at some solutions using _asynchronous_ , or _event-driven_ programming.
|
|
||||||
|
|
||||||
* * *
|
|
||||||
|
|
||||||
[[1]][1] To be fair, modern Linux kernels can tolerate a significant number of concurrent threads - as long as these threads are mostly blocked on I/O, of course. [Here's a sample program][2] that launches a configurable number of threads that sleep in a loop, waking up every 50 ms. On my 4-core Linux machine I can easily launch 10000 threads; even though these threads sleep almost all the time, they still consume between one and two cores for the context switching. Also, they occupy 80 GB of virtual memory (8 MB is the default per-thread stack size for Linux). More realistic threads that actually use memory and not just sleep in a loop can therefore exhaust the physical memory of a machine fairly quickly.
|
|
||||||
|
|
||||||
[[2]][3] Implementing a thread pool from scratch is a fun exercise, but I'll leave it for another day. I've written about hand-rolled [thread pools for specific tasks][4] in the past. That's in Python; doing it in C would be more challenging, but shouldn't take more than a few of hours for an experienced programmer.
|
|
||||||
|
|
||||||
--------------------------------------------------------------------------------
|
|
||||||
|
|
||||||
via: https://eli.thegreenplace.net/2017/concurrent-servers-part-2-threads/
|
|
||||||
|
|
||||||
作者:[Eli Bendersky][a]
|
|
||||||
译者:[译者ID](https://github.com/译者ID)
|
|
||||||
校对:[校对者ID](https://github.com/校对者ID)
|
|
||||||
|
|
||||||
本文由 [LCTT](https://github.com/LCTT/TranslateProject) 原创编译,[Linux中国](https://linux.cn/) 荣誉推出
|
|
||||||
|
|
||||||
[a]:https://eli.thegreenplace.net/pages/about
|
|
||||||
[1]:https://eli.thegreenplace.net/2017/concurrent-servers-part-2-threads/#id1
|
|
||||||
[2]:https://github.com/eliben/code-for-blog/blob/master/2017/async-socket-server/threadspammer.c
|
|
||||||
[3]:https://eli.thegreenplace.net/2017/concurrent-servers-part-2-threads/#id2
|
|
||||||
[4]:http://eli.thegreenplace.net/2011/12/27/python-threads-communication-and-stopping
|
|
||||||
[5]:https://eli.thegreenplace.net/tag/concurrency
|
|
||||||
[6]:https://eli.thegreenplace.net/tag/c-c
|
|
||||||
[7]:https://eli.thegreenplace.net/tag/python
|
|
||||||
[8]:http://eli.thegreenplace.net/2017/concurrent-servers-part-1-introduction/
|
|
||||||
[9]:http://eli.thegreenplace.net/2017/concurrent-servers-part-2-threads/
|
|
||||||
[10]:http://eli.thegreenplace.net/2017/concurrent-servers-part-3-event-driven/
|
|
||||||
[11]:https://github.com/eliben/code-for-blog/blob/master/2017/async-socket-server/threaded-server.c
|
|
||||||
[12]:http://eli.thegreenplace.net/2010/04/05/pthreads-as-a-case-study-of-good-api-design
|
|
||||||
[13]:https://eli.thegreenplace.net/2017/concurrent-servers-part-2-threads/#id3
|
|
||||||
[14]:https://en.wikipedia.org/wiki/Denial-of-service_attack
|
|
||||||
[15]:https://en.wikipedia.org/wiki/Thread_pool
|
|
||||||
[16]:https://github.com/eliben/code-for-blog/blob/master/2017/async-socket-server/threadpool-server.py
|
|
||||||
[17]:https://eli.thegreenplace.net/2017/concurrent-servers-part-2-threads/#id4
|
|
||||||
[18]:http://eli.thegreenplace.net/2017/clojure-concurrency-and-blocking-with-coreasync/
|
|
||||||
[19]:https://eli.thegreenplace.net/2017/concurrent-servers-part-2-threads/
|
|
||||||
[20]:http://eli.thegreenplace.net/2017/concurrent-servers-part-1-introduction/
|
|
@ -1,94 +0,0 @@
|
|||||||
Why Linux Works
|
|
||||||
============================================================
|
|
||||||
translating by softpaopao
|
|
||||||
|
|
||||||
_Amid the big cash and fierce corporate jockeying around Linux, it’s the developers who truly give the operating system its vitality._
|
|
||||||
|
|
||||||
The [Linux community][7] works, it turns out, because the Linux community isn’t too concerned about work, per se. As much as Linux has come to dominate many areas of corporate computing – from HPC to mobile to cloud – the engineers who write the Linux kernel tend to focus on the code itself, rather than their corporate interests therein.
|
|
||||||
|
|
||||||
Such is one prominent conclusion that emerges from [Dawn Foster’s doctoral work][8], examining collaboration on the Linux kernel. Foster, a former community lead at Intel and Puppet Labs, notes, “Many people consider themselves a Linux kernel developer first, an employee second.”
|
|
||||||
|
|
||||||
With all the “foundation washing” corporations have inflicted upon various open source projects, hoping to hide corporate prerogatives behind a mask of supposed community, Linux has managed to keep itself pure. The question is how.
|
|
||||||
|
|
||||||
**Follow the Money**
|
|
||||||
|
|
||||||
After all, if any open source project should lend itself to corporate greed, it’s Linux. Back in 2008, [the Linux ecosystem was estimated to top $25 billion in value][9]. Nearly 10 years later, that number must be multiples bigger, with much of our current cloud, mobile, and big data infrastructure dependent on Linux. Even within a single company like Oracle, Linux delivers billions of dollars in value.
|
|
||||||
|
|
||||||
Small wonder, then, that there’s such a landgrab to influence the direction of Linux through code.
|
|
||||||
|
|
||||||
Take a look at the most active contributors to Linux over the last year and it’s enterprise “turtles” all the way down, as captured in the [Linux Foundation’s latest report][10]:
|
|
||||||
|
|
||||||

|
|
||||||
|
|
||||||
Each of these corporations spends significant quantities of cash to pay developers to contribute free software, and each is banking on a return on these investments. Because of the potential for undue corporate influence over Linux, [some have cried foul][11] on the supposed shepherd of Linux development, the Linux Foundation. This criticism has become more pronounced of late as erstwhile enemies of open source like Microsoft have bought their way into the Linux Foundation.
|
|
||||||
|
|
||||||
But this is a false foe and, frankly, an outdated one.
|
|
||||||
|
|
||||||
While it’s true that corporate interests line up to throw cash at the Linux Foundation, it’s just as true that this cash doesn’t buy them influence over code. In the best open source communities, cash helps to fund developers, but those developers in turn focus on code before corporation. As Linux Foundation executive director [Jim Zemlin has stressed][12]:
|
|
||||||
|
|
||||||
“The technical roles in our projects are separate from corporations. No one’s commits are tagged with their corporate identity: code talks loudest in Linux Foundation projects. Developers in our projects can move from one firm to another and their role in the projects will remain unchanged. Subsequent commercial or government adoption of that code creates value, which in turn can be reinvested in a project. This virtuous cycle benefits all, and is the goal of any of our projects.”
|
|
||||||
|
|
||||||
Anyone that has read [Linus Torvalds’][13] mailing list commentaries can’t possibly believe that he’s a dupe of this or that corporation. The same holds true for other prominent contributors. While they are almost universally employed by big corporations, it’s generally the case that the corporations pay developers for work they’re already predisposed to do and, in fact, are doing.
|
|
||||||
|
|
||||||
After all, few corporations would have the patience or risk profile necessary to fund a bunch of newbie Linux kernel hackers and wait around for years for some of them to _maybe_ contribute enough quality code to merit a position of influence on the kernel team. So they opt to hire existing, trusted developers. As noted in the [2016 Linux Foundation report][14], “The number of unpaid developers continue[d] its slow decline, as Linux kernel development proves an increasingly valuable skill sought by employers, ensuring experienced kernel developers do not stay unpaid for long.”
|
|
||||||
|
|
||||||
Such trust is bought with code, however, not corporate cash. So none of those Linux kernel developers is going to sell out the trust they’ve earned for a brief stint of cash that will quickly fade when an emerging conflict of interest compromises the quality of their code. It makes no sense.
|
|
||||||
|
|
||||||
**Not Kumbaya, but not Game of Thrones, Either**
|
|
||||||
|
|
||||||
Ultimately, Linux kernel development is about identity, something Foster’s research calls out.
|
|
||||||
|
|
||||||
Working for Google may be nice, and perhaps carries with it a decent title and free drycleaning. Being the maintainer for a key subsystem of the Linux kernel, however, is even harder to come by and carries with it the promise of assured, highly lucrative employment by any number of companies.
|
|
||||||
|
|
||||||
As Foster writes, “Even when they enjoy their current job and like their employer, most [Linux kernel developers] tend to look at the employment relationship as something temporary, whereas their identity as a kernel developer is viewed as more permanent and more important.”
|
|
||||||
|
|
||||||
Because of this identity as a Linux kernel developer first, and corporate citizen second, Linux kernel developers can comfortably collaborate even with their employer’s fiercest competitors. This works because the employers ultimately have limited ability to steer their developers’ work, for reasons noted above. Foster delves into this issue:
|
|
||||||
|
|
||||||
“Although companies do sometimes influence the areas where their employees contribute, individuals have quite a bit of freedom in how they do the work. Many receive little direction for their day-to-day work, with a high degree of trust from their employers to do useful work. However, occasionally they are asked to do some specific piece of work or to take an interest in a particular area that is important for the company.
|
|
||||||
|
|
||||||
Many kernel developers also collaborate with their competitors on a regular basis, where they interact with each other as individuals without focusing on the fact that their employers compete with each other. This was something I saw a lot of when I was working at Intel, because our kernel developers worked with almost all of our major competitors.”
|
|
||||||
|
|
||||||
The corporations may compete on chips that run Linux, or distributions of Linux, or other software enabled by a robust operating system, but the developers focus on just one thing: making the best Linux possible. Again, this works because their identity is tied to Linux, not the firewall they sit behind while they code.
|
|
||||||
|
|
||||||
Foster has illustrated this interaction for the USB subsystem mailing list (between 2013 and 2015), with darker lines portraying heavier email interaction between companies:
|
|
||||||
|
|
||||||

|
|
||||||
|
|
||||||
In pricing discussions the obvious interaction between a number of companies might raise suspicions among antitrust authorities, but in Linux land it’s simply business as usual. This results in a better OS for all the parties to go out and bludgeon each other with in free market competition.
|
|
||||||
|
|
||||||
**Finding the Right Balance**
|
|
||||||
|
|
||||||
Such “coopetition,” as Novell founder Ray Noorda might have styled it, exists among the best open source communities, but only works where true community emerges. It’s tough, for example, for a project dominated by a single vendor to achieve the right collaborative tension. [Kubernetes][15], launched by Google, suggests it’s possible, but other projects like Docker have struggled to reach the same goal, in large part because they have been unwilling to give up technical leadership over their projects.
|
|
||||||
|
|
||||||
Perhaps Kubernetes worked so well because Google didn’t feel the need to dominate and, in fact, _wants_ other companies to take on the mantle of development leadership. With a fantastic code base that solves a major industry need, a project like Kubernetes is well-positioned to succeed so long as Google both helps to foster it and then gets out of the way, which it has, encouraging significant contributions from Red Hat and others.
|
|
||||||
|
|
||||||
Kubernetes, however, is the exception, just as Linux was before it. To succeed _because of_ corporate greed, there has to be a lot of it, and balanced between competing interests. If a project is governed by just one company’s self-interest, generally reflected in its technical governance, no amount of open source licensing will be enough to shake it free of that corporate influence.
|
|
||||||
|
|
||||||
Linux works, in short, because so many companies want to control it and can’t, due to its industry importance, making it far more profitable for a developer to build her career as a _Linux developer_ rather than a Red Hat (or Intel or Oracle or…) engineer.
|
|
||||||
|
|
||||||
--------------------------------------------------------------------------------
|
|
||||||
|
|
||||||
via: https://www.datamation.com/open-source/why-linux-works.html
|
|
||||||
|
|
||||||
作者:[Matt Asay][a]
|
|
||||||
译者:[译者ID](https://github.com/译者ID)
|
|
||||||
校对:[校对者ID](https://github.com/校对者ID)
|
|
||||||
|
|
||||||
本文由 [LCTT](https://github.com/LCTT/TranslateProject) 原创编译,[Linux中国](https://linux.cn/) 荣誉推出
|
|
||||||
|
|
||||||
[a]:https://www.datamation.com/author/Matt-Asay-1133910.html
|
|
||||||
[1]:https://www.datamation.com/feedback/https://www.datamation.com/open-source/why-linux-works.html
|
|
||||||
[2]:https://www.datamation.com/author/Matt-Asay-1133910.html
|
|
||||||
[3]:https://www.datamation.com/e-mail/https://www.datamation.com/open-source/why-linux-works.html
|
|
||||||
[4]:https://www.datamation.com/print/https://www.datamation.com/open-source/why-linux-works.html
|
|
||||||
[5]:https://www.datamation.com/open-source/why-linux-works.html#comment_form
|
|
||||||
[6]:https://www.datamation.com/author/Matt-Asay-1133910.html
|
|
||||||
[7]:https://www.datamation.com/open-source/
|
|
||||||
[8]:https://opensource.com/article/17/10/collaboration-linux-kernel
|
|
||||||
[9]:http://www.osnews.com/story/20416/Linux_Ecosystem_Worth_25_Billion
|
|
||||||
[10]:https://www.linux.com/publications/linux-kernel-development-how-fast-it-going-who-doing-it-what-they-are-doing-and-who-5
|
|
||||||
[11]:https://www.datamation.com/open-source/the-linux-foundation-and-the-uneasy-alliance.html
|
|
||||||
[12]:https://thenewstack.io/linux-foundation-critics/
|
|
||||||
[13]:https://github.com/torvalds
|
|
||||||
[14]:https://www.linux.com/publications/linux-kernel-development-how-fast-it-going-who-doing-it-what-they-are-doing-and-who-5
|
|
||||||
[15]:https://kubernetes.io/
|
|
@ -1,3 +1,5 @@
|
|||||||
|
translating by 2ephaniah
|
||||||
|
|
||||||
Proxy Models in Container Environments
|
Proxy Models in Container Environments
|
||||||
============================================================
|
============================================================
|
||||||
|
|
||||||
|
@ -1,144 +0,0 @@
|
|||||||
translating---geekpi
|
|
||||||
|
|
||||||
Why Use Docker with R? A DevOps Perspective
|
|
||||||
============================================================
|
|
||||||
|
|
||||||
[][11]
|
|
||||||
|
|
||||||
There have been several blog posts going around about why one would use Docker with R.
|
|
||||||
In this post I’ll try to add a DevOps point of view and explain how containerizing
|
|
||||||
R is used in the context of the OpenCPU system for building and deploying R servers.
|
|
||||||
|
|
||||||
> Has anyone in the [#rstats][2] world written really well about the *why* of their use of Docker, as opposed to the the *how*?
|
|
||||||
>
|
|
||||||
> — Jenny Bryan (@JennyBryan) [September 29, 2017][3]
|
|
||||||
|
|
||||||
### 1: Easy Development
|
|
||||||
|
|
||||||
The flagship of the OpenCPU system is the [OpenCPU server][12]:
|
|
||||||
a mature and powerful Linux stack for embedding R in systems and applications.
|
|
||||||
Because OpenCPU is completely open source we can build and ship on DockerHub. A ready-to-go linux server with both OpenCPU and RStudio
|
|
||||||
can be started using the following (use port 8004 or 80):
|
|
||||||
|
|
||||||
```
|
|
||||||
docker run -t -p 8004:8004 opencpu/rstudio
|
|
||||||
|
|
||||||
```
|
|
||||||
|
|
||||||
Now simply open [http://localhost:8004/ocpu/][13] and
|
|
||||||
[http://localhost:8004/rstudio/][14] in your browser!
|
|
||||||
Login via rstudio with user: `opencpu` (passwd: `opencpu`) to build or install apps.
|
|
||||||
See the [readme][15] for more info.
|
|
||||||
|
|
||||||
Docker makes it easy to get started with OpenCPU. The container gives you the full
|
|
||||||
flexibility of a Linux box, without the need to install anything on your system.
|
|
||||||
You can install packages or apps via rstudio server, or use `docker exec` to a
|
|
||||||
root shell on the running server:
|
|
||||||
|
|
||||||
```
|
|
||||||
# Lookup the container ID
|
|
||||||
docker ps
|
|
||||||
|
|
||||||
# Drop a shell
|
|
||||||
docker exec -i -t eec1cdae3228 /bin/bash
|
|
||||||
|
|
||||||
```
|
|
||||||
|
|
||||||
From the shell you can install additional software in the server, customize the apache2 httpd
|
|
||||||
config (auth, proxies, etc), tweak R options, optimize performance by preloading data or
|
|
||||||
packages, etc.
|
|
||||||
|
|
||||||
### 2: Shipping and Deployment via DockerHub
|
|
||||||
|
|
||||||
The most powerful use if Docker is shipping and deploying applications via DockerHub. To create a fully standalone
|
|
||||||
application container, simply use a standard [opencpu image][16]
|
|
||||||
and add your app.
|
|
||||||
|
|
||||||
For the purpose of this blog post I have wrapped up some of the [example apps][17] as docker containers by adding a very simple `Dockerfile` to each repository. For example the [nabel][18] app has a [Dockerfile][19] that contains the following:
|
|
||||||
|
|
||||||
```
|
|
||||||
FROM opencpu/base
|
|
||||||
|
|
||||||
RUN R -e 'devtools::install_github("rwebapps/nabel")'
|
|
||||||
|
|
||||||
```
|
|
||||||
|
|
||||||
It takes the standard [opencpu/base][20]
|
|
||||||
image and then installs the nabel app from the Github [repository][21].
|
|
||||||
The result is a completeley isolated, standalone application. The application can be
|
|
||||||
started by anyone using e.g:
|
|
||||||
|
|
||||||
```
|
|
||||||
docker run -d 8004:8004 rwebapps/nabel
|
|
||||||
|
|
||||||
```
|
|
||||||
|
|
||||||
The `-d` daemonizes on port 8004.
|
|
||||||
Obviously you can tweak the `Dockerfile` to install whatever extra software or settings you need
|
|
||||||
for your application.
|
|
||||||
|
|
||||||
Containerized deployment shows the true power of docker: it allows for shipping fully
|
|
||||||
self contained appliations that work out of the box, without installing any software or
|
|
||||||
relying on paid hosting services. If you do prefer professional hosting, there are
|
|
||||||
many companies that will gladly host docker applications for you on scalable infrastructure.
|
|
||||||
|
|
||||||
### 3 Cross Platform Building
|
|
||||||
|
|
||||||
There is a third way Docker is used for OpenCPU. At each release we build
|
|
||||||
the `opencpu-server` installation package for half a dozen operating systems, which
|
|
||||||
get published on [https://archive.opencpu.org][22].
|
|
||||||
This process has been fully automated using DockerHub. The following images automatically
|
|
||||||
build the enitre stack from source:
|
|
||||||
|
|
||||||
* [opencpu/ubuntu-16.04][4]
|
|
||||||
|
|
||||||
* [opencpu/debian-9][5]
|
|
||||||
|
|
||||||
* [opencpu/fedora-25][6]
|
|
||||||
|
|
||||||
* [opencpu/fedora-26][7]
|
|
||||||
|
|
||||||
* [opencpu/centos-6][8]
|
|
||||||
|
|
||||||
* [opencpu/centos-7][9]
|
|
||||||
|
|
||||||
DockerHub automatically rebuilds this images when a new release is published on Github.
|
|
||||||
All that is left to do is run a [script][23]
|
|
||||||
which pull down the images and copies the `opencpu-server`binaries to the [archive server][24].
|
|
||||||
|
|
||||||
--------------------------------------------------------------------------------
|
|
||||||
|
|
||||||
via: https://www.r-bloggers.com/why-use-docker-with-r-a-devops-perspective/
|
|
||||||
|
|
||||||
作者:[Jeroen Ooms][a]
|
|
||||||
译者:[译者ID](https://github.com/译者ID)
|
|
||||||
校对:[校对者ID](https://github.com/校对者ID)
|
|
||||||
|
|
||||||
本文由 [LCTT](https://github.com/LCTT/TranslateProject) 原创编译,[Linux中国](https://linux.cn/) 荣誉推出
|
|
||||||
|
|
||||||
[a]:https://www.r-bloggers.com/author/jeroen-ooms/
|
|
||||||
[1]:https://www.opencpu.org/posts/opencpu-with-docker/
|
|
||||||
[2]:https://twitter.com/hashtag/rstats?src=hash&ref_src=twsrc%5Etfw
|
|
||||||
[3]:https://twitter.com/JennyBryan/status/913785731998289920?ref_src=twsrc%5Etfw
|
|
||||||
[4]:https://hub.docker.com/r/opencpu/ubuntu-16.04/
|
|
||||||
[5]:https://hub.docker.com/r/opencpu/debian-9/
|
|
||||||
[6]:https://hub.docker.com/r/opencpu/fedora-25/
|
|
||||||
[7]:https://hub.docker.com/r/opencpu/fedora-26/
|
|
||||||
[8]:https://hub.docker.com/r/opencpu/centos-6/
|
|
||||||
[9]:https://hub.docker.com/r/opencpu/centos-7/
|
|
||||||
[10]:https://www.r-bloggers.com/
|
|
||||||
[11]:https://www.opencpu.org/posts/opencpu-with-docker
|
|
||||||
[12]:https://www.opencpu.org/download.html
|
|
||||||
[13]:http://localhost:8004/ocpu/
|
|
||||||
[14]:http://localhost:8004/rstudio/
|
|
||||||
[15]:https://hub.docker.com/r/opencpu/rstudio/
|
|
||||||
[16]:https://hub.docker.com/u/opencpu/
|
|
||||||
[17]:https://www.opencpu.org/apps.html
|
|
||||||
[18]:https://rwebapps.ocpu.io/nabel/www/
|
|
||||||
[19]:https://github.com/rwebapps/nabel/blob/master/Dockerfile
|
|
||||||
[20]:https://hub.docker.com/r/opencpu/base/
|
|
||||||
[21]:https://github.com/rwebapps
|
|
||||||
[22]:https://archive.opencpu.org/
|
|
||||||
[23]:https://github.com/opencpu/archive/blob/gh-pages/update.sh
|
|
||||||
[24]:https://archive.opencpu.org/
|
|
||||||
[25]:https://www.r-bloggers.com/author/jeroen-ooms/
|
|
@ -1,3 +1,5 @@
|
|||||||
|
translating---geekpi
|
||||||
|
|
||||||
# Introducing CRI-O 1.0
|
# Introducing CRI-O 1.0
|
||||||
|
|
||||||
Last year, the Kubernetes project introduced its [Container Runtime Interface][11] (CRI) -- a plugin interface that gives kubelet (a cluster node agent used to create pods and start containers) the ability to use different OCI-compliant container runtimes, without needing to recompile Kubernetes. Building on that work, the [CRI-O][12] project ([originally known as OCID][13]) is ready to provide a lightweight runtime for Kubernetes.
|
Last year, the Kubernetes project introduced its [Container Runtime Interface][11] (CRI) -- a plugin interface that gives kubelet (a cluster node agent used to create pods and start containers) the ability to use different OCI-compliant container runtimes, without needing to recompile Kubernetes. Building on that work, the [CRI-O][12] project ([originally known as OCID][13]) is ready to provide a lightweight runtime for Kubernetes.
|
||||||
|
@ -1,55 +0,0 @@
|
|||||||
translating---geekpi
|
|
||||||
|
|
||||||
[How to deploy multi-cloud serverless and Cloud Foundry APIs at scale][2]
|
|
||||||
============================================================
|
|
||||||
|
|
||||||
“Micro-services and APIs are products and we need to think about them that way,” says IBM’s Ken Parmelee.
|
|
||||||
|
|
||||||

|
|
||||||
|
|
||||||
Ken Parmelee, who leads the API gateway for IBM and Big Blue’s open source projects, has a few ideas about open-source methods for “attacking” the API and how to create micro-services and make them scale.
|
|
||||||
|
|
||||||
“Micro-services and APIs are products and we need to be thinking about them that way,” Parmelee says. “As you start to put them up people rely on them as part of their business. That’s a key aspect of what you’re doing in this space.”
|
|
||||||
|
|
||||||

|
|
||||||
|
|
||||||
He took the stage at the recent [Nordic APIs 2017 Platform Summit][3] and challenged a few popular notions.
|
|
||||||
|
|
||||||
“Fail fast is not really a good concept. You want to get something out there that’s fantastic on the first run. That doesn’t mean you need to take tons of time, but make it fantastic then keep evolving and improving. If it’s really bad in the beginning, people aren’t going to want to stick with you.”
|
|
||||||
|
|
||||||
He runs through IBM’s modern serverless architectures including [OpenWhisk][4], an open source partnership between IBM and Apache. The cloud-first distributed event-based programming service is result of focusing on this space for over two years; IBM is a leading contributor and it serves as the foundation of IBM cloud functions. It offers infrastructure-as-a-service, automatically scales, offers support for multiple languages and users pay only for what they actually use. Challenges were also a part of this journey, as they discovered that servers actions need securing and require ease — anonymous access, missing usage trails, fixed URL schemes etc.
|
|
||||||
|
|
||||||
Anyone can try out these serverless APIs in just 30 seconds at [https://console.bluemix.net/openwhisk/][5] “This sounds very gimmicky, but it is that easy to do…We’re combining the [work we’ve done with Cloud Foundry ][6]and released them in Bluemix under the OpenWhisk to provide security and scalability.”
|
|
||||||
|
|
||||||
“Flexibility is also hugely important for micro-services,” he says. “When you’re working in the real world with APIs, you start to have to scale across clouds.” That means from your on-premise cloud to the public cloud and “having an honest concept of how you’re going to do that is important.
|
|
||||||
|
|
||||||

|
|
||||||
|
|
||||||
And when thinking about the “any cloud concept” he warns that it’s not “throw it into any Docker container and it runs anywhere. That’s great but it needs to run effectively in those environments. Docker and Kubernetes help a lot, but you want to operationalize how you’re going to do it.” Think ahead on the consumption of your API – whether it’s running only internally or will expand to a public cloud and be publicly consumable – you need have that “architectural view,” he adds.
|
|
||||||
|
|
||||||
“We all hope that what we create has value and gets consumed a lot,” Parmelee says. The more successful the API, the bigger the challenge of taking it to the next level.
|
|
||||||

|
|
||||||
APIs are building blocks for micro-services or “inter-services.”
|
|
||||||
|
|
||||||
The future of APIs is cloud native — regardless of where you start, he says. Key factors are scalability, simplifying back-end management, cost and avoiding vendor lock-in.
|
|
||||||
|
|
||||||
You can catch his entire 23-minute talk below or on [YouTube][7].
|
|
||||||
|
|
||||||
--------------------------------------------------------------------------------
|
|
||||||
|
|
||||||
via: http://superuser.openstack.org/articles/deploy-multi-cloud-serverless-cloud-foundry-apis-scale/
|
|
||||||
|
|
||||||
作者:[Superuser ][a]
|
|
||||||
译者:[译者ID](https://github.com/译者ID)
|
|
||||||
校对:[校对者ID](https://github.com/校对者ID)
|
|
||||||
|
|
||||||
本文由 [LCTT](https://github.com/LCTT/TranslateProject) 原创编译,[Linux中国](https://linux.cn/) 荣誉推出
|
|
||||||
|
|
||||||
[a]:http://superuser.openstack.org/articles/author/superuser/
|
|
||||||
[1]:http://superuser.openstack.org/articles/author/superuser/
|
|
||||||
[2]:http://superuser.openstack.org/articles/deploy-multi-cloud-serverless-cloud-foundry-apis-scale/
|
|
||||||
[3]:https://nordicapis.com/events/the-2017-api-platform-summit/
|
|
||||||
[4]:https://developer.ibm.com/openwhisk/
|
|
||||||
[5]:https://console.bluemix.net/openwhisk/
|
|
||||||
[6]:https://cloudfoundry.org/the-foundry/ibm-cloud/
|
|
||||||
[7]:https://www.youtube.com/jA25Kmxr6fU
|
|
@ -1,3 +1,4 @@
|
|||||||
|
XYenChi is translating
|
||||||
Image Processing on Linux
|
Image Processing on Linux
|
||||||
============================================================
|
============================================================
|
||||||
|
|
||||||
|
@ -1,74 +0,0 @@
|
|||||||
PingCAP Launches TiDB 1.0
|
|
||||||
============================================================
|
|
||||||
|
|
||||||
### PingCAP Launches TiDB 1.0, A Scalable Hybrid Database Solution
|
|
||||||
|
|
||||||
October 16, 2017 - PingCAP Inc., a cutting-edge distributed database technology company, officially announces the release of [TiDB][4] 1.0\. TiDB is an open source distributed Hybrid Transactional/Analytical Processing (HTAP) database that empowers businesses to meet both workloads with a single database.
|
|
||||||
|
|
||||||
In the current database landscape, infrastructure engineers often have to use one database for online transactional processing (OLTP) and another for online analytical processing (OLAP). TiDB aims to break down this separation by building a HTAP database that enables real-time business analysis based on live transactional data. With TiDB, engineers can now spend less time managing multiple database solutions, and more time delivering business value for their companies. One of TiDB’s many users, a financial securities firm, is leveraging this technology to power its application for wealth management and user personas. With TiDB, this firm can easily process web-scale volumes of billing records and conduct mission-critical time sensitive data analysis like never before.
|
|
||||||
|
|
||||||
_“Two and a half years ago, Edward, Dylan and I started this journey to build a new database for an old problem that has long plagued the infrastructure software industry. Today, we are proud to announce that this database, TiDB, is production ready,”_ said Max Liu, co-founder and CEO of PingCAP. _“Abraham Lincoln once said, ‘the best way to predict the future is to create it.’ The future we predicted 771 days ago we now have created, because of the hard work and dedication of not just every member of our team, but also every contributor, user, and partner in our open source community. Today, we celebrate and pay gratitude to the power of the open source spirit. Tomorrow, we will continue to create the future we believe in.”_
|
|
||||||
|
|
||||||
TiDB has already been deployed in production in more than 30 companies in the APAC region, including fast-growing Internet companies like [Mobike][5], [Gaea][6], and [YOUZU][7]. The use cases span multiple industries from online marketplace and gaming, to fintech, media, and travel.
|
|
||||||
|
|
||||||
### TiDB features
|
|
||||||
|
|
||||||
**Horizontal Scalability**
|
|
||||||
|
|
||||||
TiDB grows as your business grows. You can increase the capacity for storage and computation simply by adding more machines.
|
|
||||||
|
|
||||||
**Compatible with MySQL Protocol**
|
|
||||||
|
|
||||||
Use TiDB as MySQL. You can replace MySQL with TiDB to power your application without changing a single line of code in most cases and with nearly no migration cost.
|
|
||||||
|
|
||||||
**Automatic Failover and High Availability**
|
|
||||||
|
|
||||||
Your data and applications are always-on. TiDB automatically handles malfunctions and protects your applications from machine failures or even downtime of an entire data-center.
|
|
||||||
|
|
||||||
**Consistent Distributed Transactions**
|
|
||||||
|
|
||||||
TiDB is analogous to a single-machine RDBMS. You can start a transaction that crosses multiple machines without worrying about consistency. TiDB makes your application code simple and robust.
|
|
||||||
|
|
||||||
**Online DDL**
|
|
||||||
|
|
||||||
Evolve TiDB schemas as your requirement changes. You can add new columns and indexes without stopping or affecting your ongoing operations.
|
|
||||||
|
|
||||||
[Try TiDB Now!][8]
|
|
||||||
|
|
||||||
### Use cases
|
|
||||||
|
|
||||||
[How TiDB tackles fast data growth and complex queries for yuanfudao.com][9]
|
|
||||||
|
|
||||||
[Migration from MySQL to TiDB to handle tens of millions of rows of data per day][10]
|
|
||||||
|
|
||||||
### For more information:
|
|
||||||
|
|
||||||
TiDB internal:
|
|
||||||
|
|
||||||
* [Data Storage][1]
|
|
||||||
|
|
||||||
* [Computing][2]
|
|
||||||
|
|
||||||
* [Scheduling][3]
|
|
||||||
|
|
||||||
--------------------------------------------------------------------------------
|
|
||||||
|
|
||||||
via: https://pingcap.github.io/blog/2017/10/17/announcement/
|
|
||||||
|
|
||||||
作者:[PingCAP ][a]
|
|
||||||
译者:[译者ID](https://github.com/译者ID)
|
|
||||||
校对:[校对者ID](https://github.com/校对者ID)
|
|
||||||
|
|
||||||
本文由 [LCTT](https://github.com/LCTT/TranslateProject) 原创编译,[Linux中国](https://linux.cn/) 荣誉推出
|
|
||||||
|
|
||||||
[a]:https://pingcap.github.io/blog/
|
|
||||||
[1]:https://pingcap.github.io/blog/2017/07/11/tidbinternal1/
|
|
||||||
[2]:https://pingcap.github.io/blog/2017/07/11/tidbinternal2/
|
|
||||||
[3]:https://pingcap.github.io/blog/2017/07/20/tidbinternal3/
|
|
||||||
[4]:https://github.com/pingcap/tidb
|
|
||||||
[5]:https://en.wikipedia.org/wiki/Mobike
|
|
||||||
[6]:http://www.gaea.com/en/
|
|
||||||
[7]:http://www.yoozoo.com/aboutEn
|
|
||||||
[8]:https://pingcap.com/doc-QUICKSTART
|
|
||||||
[9]:https://pingcap.github.io/blog/2017/08/08/tidbforyuanfudao/
|
|
||||||
[10]:https://pingcap.github.io/blog/2017/05/22/Comparison-between-MySQL-and-TiDB-with-tens-of-millions-of-data-per-day/
|
|
@ -1,42 +0,0 @@
|
|||||||
# IoT Cybersecurity: What's Plan B?
|
|
||||||
|
|
||||||
In August, four US Senators introduced a bill designed to improve Internet of Things (IoT) security. The IoT Cybersecurity Improvement Act of 2017 is a modest piece of legislation. It doesn't regulate the IoT market. It doesn't single out any industries for particular attention, or force any companies to do anything. It doesn't even modify the liability laws for embedded software. Companies can continue to sell IoT devices with whatever lousy security they want.
|
|
||||||
|
|
||||||
What the bill does do is leverage the government's buying power to nudge the market: any IoT product that the government buys must meet minimum security standards. It requires vendors to ensure that devices can not only be patched, but are patched in an authenticated and timely manner; don't have unchangeable default passwords; and are free from known vulnerabilities. It's about as low a security bar as you can set, and that it will considerably improve security speaks volumes about the current state of IoT security. (Full disclosure: I helped draft some of the bill's security requirements.)
|
|
||||||
|
|
||||||
The bill would also modify the Computer Fraud and Abuse and the Digital Millennium Copyright Acts to allow security researchers to study the security of IoT devices purchased by the government. It's a far narrower exemption than our industry needs. But it's a good first step, which is probably the best thing you can say about this legislation.
|
|
||||||
|
|
||||||
However, it's unlikely this first step will even be taken. I am writing this column in August, and have no doubt that the bill will have gone nowhere by the time you read it in October or later. If hearings are held, they won't matter. The bill won't have been voted on by any committee, and it won't be on any legislative calendar. The odds of this bill becoming law are zero. And that's not just because of current politics -- I'd be equally pessimistic under the Obama administration.
|
|
||||||
|
|
||||||
But the situation is critical. The Internet is dangerous -- and the IoT gives it not just eyes and ears, but also hands and feet. Security vulnerabilities, exploits, and attacks that once affected only bits and bytes now affect flesh and blood.
|
|
||||||
|
|
||||||
Markets, as we've repeatedly learned over the past century, are terrible mechanisms for improving the safety of products and services. It was true for automobile, food, restaurant, airplane, fire, and financial-instrument safety. The reasons are complicated, but basically, sellers don't compete on safety features because buyers can't efficiently differentiate products based on safety considerations. The race-to-the-bottom mechanism that markets use to minimize prices also minimizes quality. Without government intervention, the IoT remains dangerously insecure.
|
|
||||||
|
|
||||||
The US government has no appetite for intervention, so we won't see serious safety and security regulations, a new federal agency, or better liability laws. We might have a better chance in the EU. Depending on how the General Data Protection Regulation on data privacy pans out, the EU might pass a similar security law in 5 years. No other country has a large enough market share to make a difference.
|
|
||||||
|
|
||||||
Sometimes we can opt out of the IoT, but that option is becoming increasingly rare. Last year, I tried and failed to purchase a new car without an Internet connection. In a few years, it's going to be nearly impossible to not be multiply connected to the IoT. And our biggest IoT security risks will stem not from devices we have a market relationship with, but from everyone else's cars, cameras, routers, drones, and so on.
|
|
||||||
|
|
||||||
We can try to shop our ideals and demand more security, but companies don't compete on IoT safety -- and we security experts aren't a large enough market force to make a difference.
|
|
||||||
|
|
||||||
We need a Plan B, although I'm not sure what that is. Comment if you have any ideas.
|
|
||||||
|
|
||||||
This essay previously appeared in the September/October issue of _IEEE Security & Privacy_ .
|
|
||||||
|
|
||||||
--------------------------------------------------------------------------------
|
|
||||||
|
|
||||||
作者简介:
|
|
||||||
|
|
||||||
I've been writing about security issues on my blog since 2004, and in my monthly newsletter since 1998. I write books, articles, and academic papers. Currently, I'm the Chief Technology Officer of IBM Resilient, a fellow at Harvard's Berkman Center, and a board member of EFF.
|
|
||||||
|
|
||||||
------------------
|
|
||||||
|
|
||||||
|
|
||||||
via: https://www.schneier.com/blog/archives/2017/10/iot_cybersecuri.html
|
|
||||||
|
|
||||||
作者:[Bruce Schneier][a]
|
|
||||||
译者:[译者ID](https://github.com/译者ID)
|
|
||||||
校对:[校对者ID](https://github.com/校对者ID)
|
|
||||||
|
|
||||||
本文由 [LCTT](https://github.com/LCTT/TranslateProject) 原创编译,[Linux中国](https://linux.cn/) 荣誉推出
|
|
||||||
|
|
||||||
[a]:https://www.schneier.com/blog/about/
|
|
@ -0,0 +1,147 @@
|
|||||||
|
3 Tools to Help You Remember Linux Commands
|
||||||
|
============================================================
|
||||||
|
|
||||||
|
|
||||||
|

|
||||||
|
The apropos tool, which is installed by default on nearly every Linux distribution, can help you find the command you need.[Used with permission][5]
|
||||||
|
|
||||||
|
The Linux desktop has come a very long way from its humble beginnings. Back in my early days of using Linux, knowledge of the command line was essential—even for the desktop. That’s no longer true. Many users might never touch the command line. For Linux system administrators, however, that’s not the case. In fact, for any Linux admin (be it server or desktop), the command line is a requirement. From managing networks, to security, to application and server settings—there’s nothing like the power of the good ol’ command line.
|
||||||
|
|
||||||
|
But, the thing is… there are a _lot_ of commands to be found on a Linux system. Consider _/usr/bin_ alone and you’ll find quite a lot of commands (you can issue _ls /usr/bin/ | wc -l_ to find out exactly how many you have). Of course, these aren’t all user-facing executables, but it gives you a good idea of the scope of Linux commands. On my Elementary OS system, there are 2029 executables within _/usr/bin_ . Even though I will use only a fraction of those commands, how am I supposed to remember even that amount?
|
||||||
|
|
||||||
|
Fortunately, there are various tricks and tools you can use, so that you’re not struggling on a daily basis to remember those commands. I want to offer up a few such tips that will go a long way to helping you work with the command line a bit more efficiently (and save a bit of brain power along the way).
|
||||||
|
|
||||||
|
We’ll start with a built-in tool and then illustrate two very handy applications that can be installed.
|
||||||
|
|
||||||
|
### Bash history
|
||||||
|
|
||||||
|
You may or may not know this, but Bash (the most popular Linux shell) retains a history of the commands you run. Want to see it in action? There are two ways. Open up a terminal window and tap the Up arrow key. You should see commands appear, one by one. Once you find the command you’re looking for, you can either use it as is, by hitting the Enter key, or modify it and then execute with the Enter key.
|
||||||
|
|
||||||
|
This is a great way to re-run (or modify and run) a command you’ve previously issued. I use this Linux feature regularly. It not only saves me from having to remember the minutiae of a command, it also saves me from having to type out the same command over and over.
|
||||||
|
|
||||||
|
Speaking of the Bash history, if you issue the command _history_ , you will be presented with a listing of commands you have run in the past (Figure 1).
|
||||||
|
|
||||||
|
|
||||||
|

|
||||||
|
Figure 1: Can you spot the mistake in one of my commands?[Used with permission][1]
|
||||||
|
|
||||||
|
The number of commands your Bash history holds is configured within the ~/.bashrc file. In that file, you’ll find two lines:
|
||||||
|
|
||||||
|
```
|
||||||
|
HISTSIZE=1000
|
||||||
|
|
||||||
|
HISTFILESIZE=2000
|
||||||
|
```
|
||||||
|
|
||||||
|
HISTSIZE is the maximum number of commands to remember on the history list, whereas HISTFILESIZE is the maximum number of lines contained in the history file.
|
||||||
|
|
||||||
|
Clearly, by default, Bash will retain 1000 commands in your history. That’s a lot. For some, this is considered an issue of security. If you’re concerned about that, you can shrink the number to whatever gives you the best ratio of security to practicality. If you don’t want Bash to remember your history, set HISTSIZE to 0.
|
||||||
|
|
||||||
|
If you make any changes to the ~/.bashrc file, make sure to log out and log back in (otherwise the changes won’t take effect).
|
||||||
|
|
||||||
|
### Apropos
|
||||||
|
|
||||||
|
This is the first of two tools that can be installed to assist you in recalling Linux commands. Apropos is able to search the Linux man pages to help you find the command you're looking for. Say, for instance, you don’t remember which firewall tool your distribution uses. You could type _apropos “firewall” _ and the tool would return any related command (Figure 2).
|
||||||
|
|
||||||
|
|
||||||
|

|
||||||
|
Figure 2: What is your firewall command?[Used with permission][2]
|
||||||
|
|
||||||
|
What if you needed a command to work with a directory, but had no idea what command was required? Type _apropos “directory” _ to see every command that contains the word “directory” in its man page (Figure 3).
|
||||||
|
|
||||||
|
|
||||||
|

|
||||||
|
Figure 3: What was that tool you used on a directory?[Used with permission][3]
|
||||||
|
|
||||||
|
The apropos tool is installed, by default, on nearly every Linux distribution.
|
||||||
|
|
||||||
|
### Fish
|
||||||
|
|
||||||
|
There’s another tool that does a great job of helping you recall commands. Fish is a command line shell for Linux, Unix, and Mac OS that has a few nifty tricks up its sleeve:
|
||||||
|
|
||||||
|
* Autosuggestions
|
||||||
|
|
||||||
|
* VGA Color
|
||||||
|
|
||||||
|
* Full scriptability
|
||||||
|
|
||||||
|
* Web Based configuration
|
||||||
|
|
||||||
|
* Man Page Completions
|
||||||
|
|
||||||
|
* Syntax highlighting
|
||||||
|
|
||||||
|
* And more
|
||||||
|
|
||||||
|
The autosuggestions make fish a really helpful tool (especially when you can’t recall those commands).
|
||||||
|
|
||||||
|
As you might expect, fish isn’t installed by default. For Ubuntu (and its derivatives), you can install fish with the following commands:
|
||||||
|
|
||||||
|
```
|
||||||
|
sudo apt-add-repository ppa:fish-shell/release-2
|
||||||
|
|
||||||
|
sudo apt update
|
||||||
|
|
||||||
|
sudo apt install fish
|
||||||
|
```
|
||||||
|
|
||||||
|
For the likes of CentOS, fish can be installed like so. Add the repository with the commands:
|
||||||
|
|
||||||
|
```
|
||||||
|
sudo -s
|
||||||
|
|
||||||
|
cd /etc/yum.repos.d/
|
||||||
|
|
||||||
|
wget http://download.opensuse.org/repositories/shells:fish:release:2/CentOS_7/shells:fish:release:2.repo
|
||||||
|
```
|
||||||
|
|
||||||
|
Update the repository list with the commands:
|
||||||
|
|
||||||
|
```
|
||||||
|
yum repolist
|
||||||
|
|
||||||
|
yum update
|
||||||
|
```
|
||||||
|
|
||||||
|
Install fish with the command:
|
||||||
|
|
||||||
|
```
|
||||||
|
yum install fish
|
||||||
|
```
|
||||||
|
|
||||||
|
Using fish isn’t quite as intuitive as you might expect. Remember, fish is a shell, so you have to enter the shell before using the command. From your terminal, issue the command fish and you will find yourself in the newly install shell (Figure 4).
|
||||||
|
|
||||||
|
|
||||||
|

|
||||||
|
Figure 4: The fish interactive shell.[Used with permission][4]
|
||||||
|
|
||||||
|
Start typing a command and fish will automatically complete the command. If the suggested command is not the one you’re looking for, hit the Tab key on your keyboard for more suggestions. If it is the command you want, type the right arrow key on your keyboard to complete the command and then hit Enter to execute. When you’re done using fish, type exit to leave that shell.
|
||||||
|
|
||||||
|
Fish does quite a bit more, but with regards to helping you remember your commands, the autosuggestions will go a very long way.
|
||||||
|
|
||||||
|
### Keep learning
|
||||||
|
|
||||||
|
There are so many commands to learn on Linux. But don’t think you have to commit every single one of them to memory. Thanks to the Bash history and tools like apropos and fish, you won’t have to strain your memory much to recall the commands you need to get your job done.
|
||||||
|
|
||||||
|
--------------------------------------------------------------------------------
|
||||||
|
|
||||||
|
via: https://www.linux.com/learn/intro-to-linux/2017/10/3-tools-help-you-remember-linux-commands
|
||||||
|
|
||||||
|
作者:[JACK WALLEN ][a]
|
||||||
|
译者:[译者ID](https://github.com/译者ID)
|
||||||
|
校对:[校对者ID](https://github.com/校对者ID)
|
||||||
|
|
||||||
|
本文由 [LCTT](https://github.com/LCTT/TranslateProject) 原创编译,[Linux中国](https://linux.cn/) 荣誉推出
|
||||||
|
|
||||||
|
[a]:https://www.linux.com/users/jlwallen
|
||||||
|
[1]:https://www.linux.com/licenses/category/used-permission
|
||||||
|
[2]:https://www.linux.com/licenses/category/used-permission
|
||||||
|
[3]:https://www.linux.com/licenses/category/used-permission
|
||||||
|
[4]:https://www.linux.com/licenses/category/used-permission
|
||||||
|
[5]:https://www.linux.com/licenses/category/used-permission
|
||||||
|
[6]:https://www.linux.com/files/images/commands1jpg
|
||||||
|
[7]:https://www.linux.com/files/images/commands2jpg
|
||||||
|
[8]:https://www.linux.com/files/images/commands3jpg
|
||||||
|
[9]:https://www.linux.com/files/images/commands4jpg
|
||||||
|
[10]:https://www.linux.com/files/images/commands-mainjpg
|
||||||
|
[11]:http://download.opensuse.org/repositories/shells:fish:release:2/CentOS_7/shells:fish:release:2.repo
|
@ -0,0 +1,81 @@
|
|||||||
|
translating---geekpi
|
||||||
|
|
||||||
|
Running Android on Top of a Linux Graphics Stack
|
||||||
|
============================================================
|
||||||
|
|
||||||
|
|
||||||
|

|
||||||
|
|
||||||
|
>You can now run Android on top of a regular Linux graphics stack, which is hugely empowering, according to Robert Foss, a Linux graphic stack contributor and Software Engineer at Collabora. Learn more in this preview of his talk at Embedded Linux Conference Europe.[Creative Commons Zero][2]Pixabay
|
||||||
|
|
||||||
|
You can now run Android on top of a regular Linux graphics stack. This was not the case before, and according to Robert Foss, a Linux graphic stack contributor and Software Engineer at Collabora, this is hugely empowering. In his upcoming talk at [Embedded Linux Conference Europe,][5] Foss will cover recent developments in this area and discuss how these changes allow you to take advantage of new features and improvements in kernels.
|
||||||
|
|
||||||
|
|
||||||
|

|
||||||
|
|
||||||
|
Robert Foss, Linux graphic stack contributor and Software Engineer at Collabora[Used with permission][1]
|
||||||
|
|
||||||
|
In this article, Foss explains more and offers a preview of his talk.
|
||||||
|
|
||||||
|
**Linux.com: Can you please tell us a bit about the graphics stack you’re talking about?**
|
||||||
|
|
||||||
|
**Foss: **Traditional Linux graphics systems (like X11) mostly did not use planes. But modern graphics systems like Android and Wayland can take full advantage of it.
|
||||||
|
|
||||||
|
Android has the most mature implementation of plane support in HWComposer, and its graphics stack is a bit different from the usual Linux desktop graphics stack. On desktops, the typical compositor just uses the GPU for all composition, because this is the only thing that exists on the desktop.
|
||||||
|
|
||||||
|
Most embedded and mobile chips have specialized 2D composition hardware that Android is designed around. The way this is done is by dividing the things that are displayed into layers, and then intelligently feeding the layers to hardware that is optimized to handle layers. This frees up the GPU to work on the things you actually care about, while at the same time, it lets hardware that is more efficient do what it does best.
|
||||||
|
|
||||||
|
**Linux.com: When you say Android, do you mean the Android Open Source Project (the AOSP)?**
|
||||||
|
|
||||||
|
**Foss: **The Android Open Source Project (the AOSP), is the base upon which many Android products is built, and there's not much of a distinction between AOSP and Android.
|
||||||
|
|
||||||
|
Specifically, my work has been done in the AOSP realm, but nothing is preventing this work from being pulled into a shipped Android product.
|
||||||
|
|
||||||
|
The distinction is more about licensing and fulfilling the requirements of Google for calling a product Android, than it is about code.
|
||||||
|
|
||||||
|
**Linux.com: Who would want to run that and why? What are some advantages?**
|
||||||
|
|
||||||
|
**Foss: **AOSP gives you a lot of things for free, such as a software stack optimized for usability, low power, and diverse hardware. It's a lot more polished and versatile than what any single company feasibly could develop on their own, without putting a lot of resources behind it.
|
||||||
|
|
||||||
|
As a manufacturer it also provides you with access to a large pool of developers that are immediately able to develop for your platform.
|
||||||
|
|
||||||
|
**Linux.com: What are some practical use cases?**
|
||||||
|
|
||||||
|
**Foss: **The new part here is the ability to run Android on top of the regular Linux graphics stack. Being able to do this with mainline/upstream kernels and drivers allows you to take advantage of new features and improvements in kernels as well, not just depend on whatever massively forked BSP you get from your vendor.
|
||||||
|
|
||||||
|
For any GPU that has reasonable standard Linux support, you are now able to run Android on top of it. This was not the case before. And in that way it is hugely enabling and empowering.
|
||||||
|
|
||||||
|
It also matter in the sense, that it incentivizes GPU designers to work with upstream for their drivers. Now there's a straightforward path for them to provide one driver that works for Android and Linux with no added effort. Their costs will be lower, and maintaining their GPU driver upstream is a lot more appealing.
|
||||||
|
|
||||||
|
For example, we would like to see mainline support Qualcomm SOCs, and we would like to be a part of making that a reality.
|
||||||
|
|
||||||
|
To summarize, this will help the hardware ecosystem get better software support and the software ecosystem have more hardware to work with.
|
||||||
|
|
||||||
|
* It improves the economy of SBC/devboard manufacturers: they can provide a single well-tested stack which works on both, rather than having to provide a "Linux stack" and an Android stack.
|
||||||
|
|
||||||
|
* It simplifies work for driver developers, since there's just a single target for optimisation and enablement.
|
||||||
|
|
||||||
|
* It enables the Android community, since Android running on mainline allows them to share the upstream improvements being made.
|
||||||
|
|
||||||
|
* It helps upstream, because we get a product-quality stack, that has been tested and developed with help from the designer of the hardware.
|
||||||
|
|
||||||
|
Previously, Mesa was looked upon as a second-class stack, but it's now shown that it is up-to-date (fully compliant with Vulkan 1.0, OpenGL 4.6, OpenGL ES 3.2 on release day), as well as performant, and product quality.
|
||||||
|
|
||||||
|
That means that driver developers can be involved in Mesa, and be confident that they're sharing in the hard work of others, and also getting a great base to build on.
|
||||||
|
|
||||||
|
--------------------------------------------------------------------------------
|
||||||
|
|
||||||
|
via: https://www.linux.com/blog/event/elce/2017/10/running-android-top-linux-graphics-stack
|
||||||
|
|
||||||
|
作者:[ SWAPNIL BHARTIYA][a]
|
||||||
|
译者:[译者ID](https://github.com/译者ID)
|
||||||
|
校对:[校对者ID](https://github.com/校对者ID)
|
||||||
|
|
||||||
|
本文由 [LCTT](https://github.com/LCTT/TranslateProject) 原创编译,[Linux中国](https://linux.cn/) 荣誉推出
|
||||||
|
|
||||||
|
[a]:https://www.linux.com/users/arnieswap
|
||||||
|
[1]:https://www.linux.com/licenses/category/used-permission
|
||||||
|
[2]:https://www.linux.com/licenses/category/creative-commons-zero
|
||||||
|
[3]:https://www.linux.com/files/images/robert-fosspng
|
||||||
|
[4]:https://www.linux.com/files/images/linux-graphics-stackjpg
|
||||||
|
[5]:http://events.linuxfoundation.org/events/embedded-linux-conference-europe
|
@ -0,0 +1,287 @@
|
|||||||
|
服务端 I/O 性能: Node , PHP , Java , Go 的对比
|
||||||
|
============
|
||||||
|
|
||||||
|
了解应用程序的输入/输出(I / O)模型意味着处理其所受负载的应用程序之间的差异,以及遇到真实环境的例子。或许你的应用程序很小,承受不了很大的负载;但随着应用程序的流量负载增加,可能因为使用低效的 I / O 模型导致承受不了而崩溃。
|
||||||
|
|
||||||
|
和大多数情况一样,处理这种问题的方法是多种的,这不仅仅是一个择优的问题,而是理解权衡的问题。 接下来我们来看看 I / O 到底是什么。
|
||||||
|
|
||||||
|
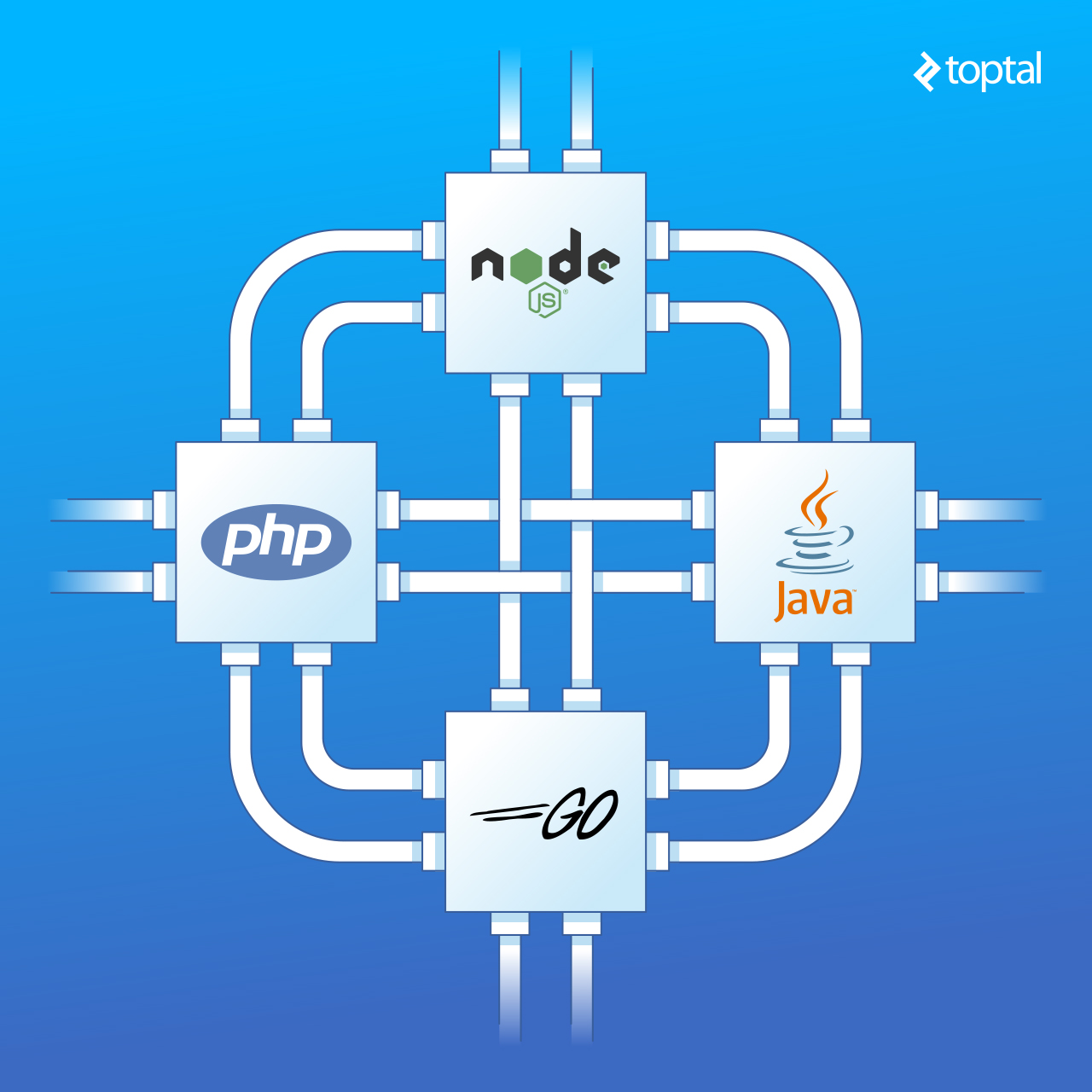
|
||||||
|
|
||||||
|
在本文中,我们将对 Node,Java,Go 和 PHP 与 Apache 进行对比,讨论不同语言如何模拟其 I / O ,每个模型的优缺点,并总结一些基本的规律。如果您担心下一个 Web 应用程序的 I / O 性能,本文将给您最优的解答。
|
||||||
|
|
||||||
|
### I/O 基础知识: 快速复习
|
||||||
|
|
||||||
|
要了解 I / O 涉及的因素,我们首先在操作系统层面检查这些概念。虽然不可能直接处理这些概念,但您可以通过应用程序的运行时环境间接处理它们。细节很重要。
|
||||||
|
|
||||||
|
### 系统调用
|
||||||
|
|
||||||
|
首先是系统调用,我们的描述如下:
|
||||||
|
|
||||||
|
* 您的程序(在“用户本地”中)说,它们必须要求操作系统内核代表它执行 I / O 操作。
|
||||||
|
|
||||||
|
* “系统调用”是您的程序要求内核执行某些操作的方法。这些实现的细节在操作系统之间有所不同,但基本概念是相同的。将有一些具体的指令将控制从您的程序转移到内核(如函数调用,但是使用专门用于处理这种情况的专用调剂)。一般来说,系统调用会阻塞,这意味着你的程序会等待内核返回你的代码。
|
||||||
|
|
||||||
|
* 内核在有问题的物理设备( 磁盘,网卡等 )上执行底层的I / O 操作,并回复系统调用。在现实世界中,内核可能需要做许多事情来满足您的要求,包括等待设备准备就绪,更新其内部状态等,但作为应用程序开发人员,您不在乎这些。这是内核该做的工作。
|
||||||
|
|
||||||
|
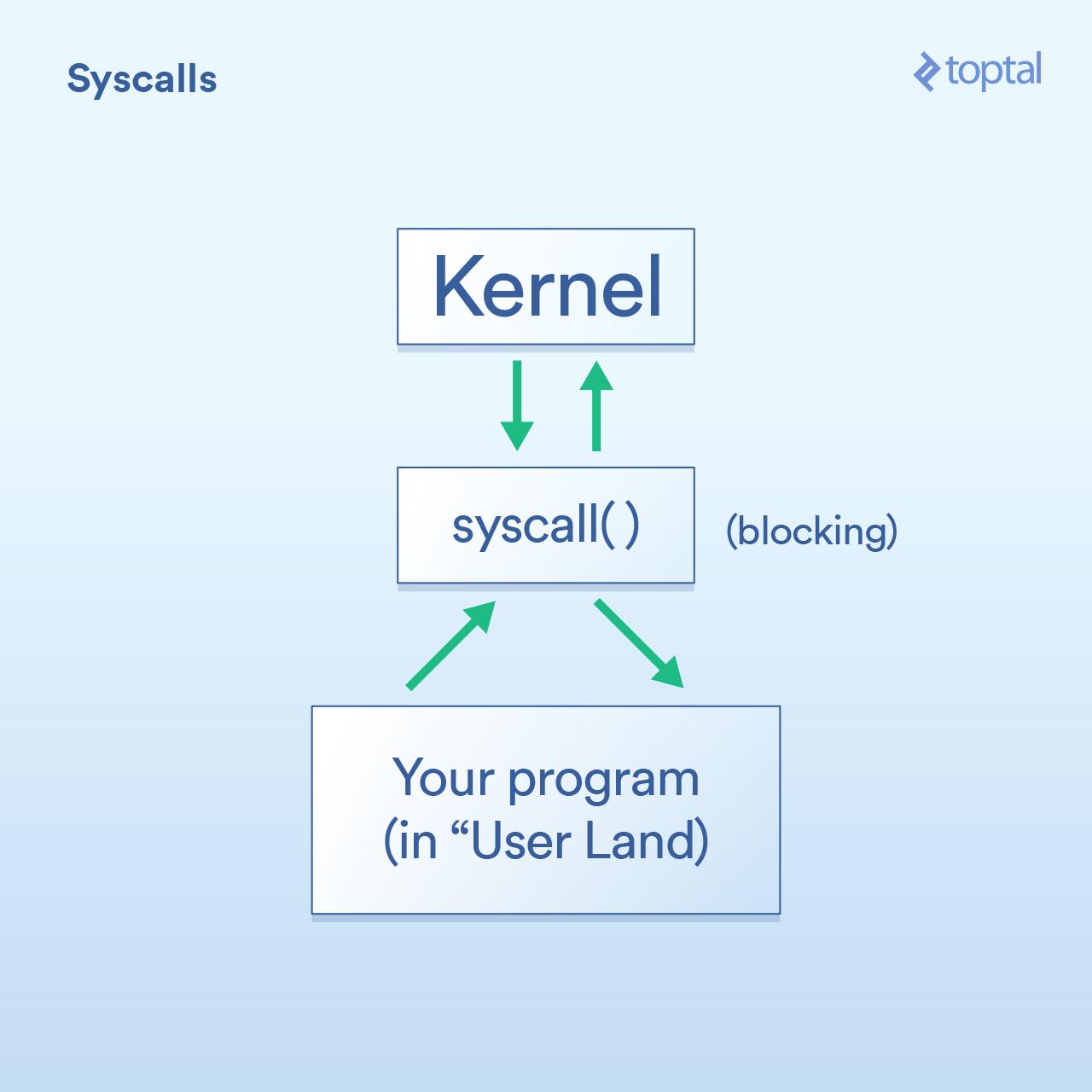
|
||||||
|
|
||||||
|
### 阻塞与非阻塞
|
||||||
|
|
||||||
|
现在,我刚刚在上面说过,系统调用是阻塞的,一般来说是这样。然而,一些调用被分为“非阻塞”,这意味着内核会接收您的请求,将其放在某个地方的队列或缓冲区中,然后立即返回而不等待实际的 I / O 发生。所以它只是在很短的时间内“阻挡”,只需要排队你的请求。
|
||||||
|
|
||||||
|
一些例子(Linux系统调用)可能有助于理解: - `read()` 是一个阻塞调用 - 你传递一个句柄,指出哪个文件和缓冲区在哪里传送它读取的数据,当数据在那里时,该调用返回。这具有简单的优点。- `epoll_create()`, `epoll_ctl()` 和 `epoll_wait()` 是分别调用的,您可以创建一组句柄来侦听,添加/删除该组中的处理程序,然后阻止直到有任何活动。这允许您通过单个线程有效地控制大量的 I / O 操作。
|
||||||
|
|
||||||
|
了解这里的时间差异的数量级是很重要的。如果 CPU 内核运行在 3GHz,而不用进行 CPU 优化,那么它每秒执行 30 亿次周期(或每纳秒 3 个周期)。非阻塞系统调用可能需要 10 秒的周期来完成或者 “相对较少的纳秒” 的时间完成。阻止通过网络接收信息的调用可能需要更长的时间 - 例如 200 毫秒(1/5秒)。比方说,非阻塞电话需要 20 纳秒,阻塞电话就需要 2 亿个纳秒。您的进程只是等待了 1000 万次的阻塞调用。
|
||||||
|
|
||||||
|
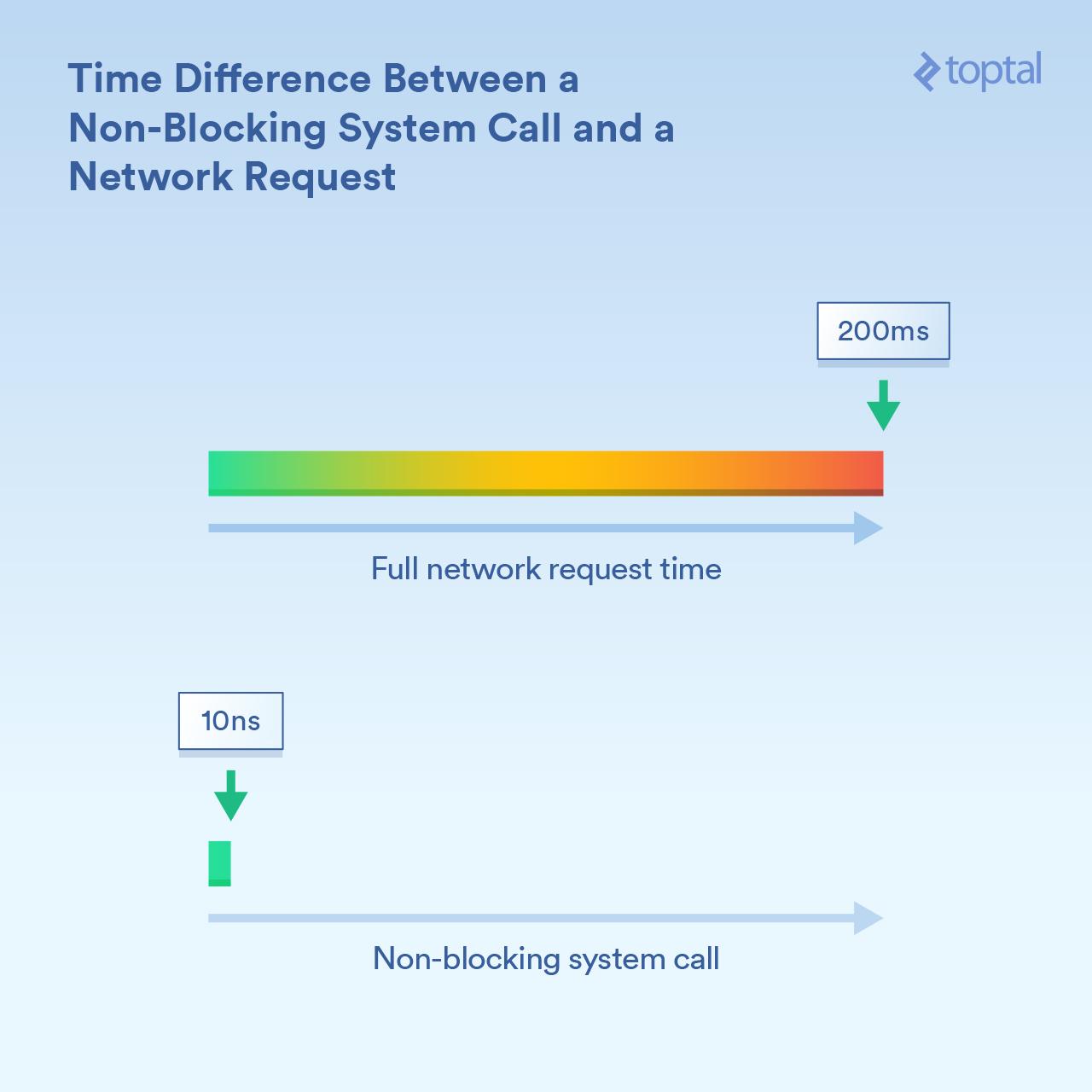
|
||||||
|
内核提供了阻塞 I / O (“从此网络连接读取并给出数据”)和非阻塞 I / O (“告知我何时这些网络连接具有新数据”)的方法。使用哪种机制将阻止调用过程显示不同的时间长度。
|
||||||
|
|
||||||
|
### 调度
|
||||||
|
|
||||||
|
关键的第三件事是当你有很多线程或进程开始阻止时会发生什么。
|
||||||
|
|
||||||
|
根据我们的理解,线程和进程之间没有很大的区别。在现实生活中,最显著的性能相关差异在于,由于线程共享相同的内存,而进程每个都有自己的内存空间,使得单独的进程往往占用更多的内存。但是当我们谈论调度时,它真正归结为一系列事情(线程和进程),每个都需要在可用的 CPU 内核上获得一段执行时间。如果您有 300 个线程运行在 8 个内核上,则必须将时间分成几个,以便每个内核获取其共享,每个内核运行一段时间,然后移动到下一个线程。这是通过 “上下文切换” 完成的,使 CPU 从一个线程/进程运行到下一个。
|
||||||
|
|
||||||
|
这些上下文切换具有与它们相关联的成本 - 它们需要一些时间。在一些快速的情况下,它可能小于 100 纳秒,但根据实际情况,处理器速度/体系结构,CPU缓存等,采取 1000 纳秒或更长时间并不常见。
|
||||||
|
|
||||||
|
而更多的线程(或进程),更多的上下文切换。当我们谈论数以千计的线程时,每个线程数百纳秒,事情就会变得很慢。
|
||||||
|
|
||||||
|
然而,非阻塞调用告诉内核“只有在这些连接中有一些新的数据或事件时才会给我”。这些非阻塞调用旨在有效地处理大量 I / O 负载并减少上下文交换。
|
||||||
|
|
||||||
|
到目前为止,我们现在看有趣的部分:我们来看看一些流行的语言使用,并得出关于易用性和性能与其他有趣的事情之间的权衡的结论。
|
||||||
|
|
||||||
|
声明,本文中显示的示例是微不足道的(部分的,只显示相关的信息); 数据库访问,外部缓存系统( memcache 等等)和任何需要 I / O 的东西都将执行某种类型的 I / O 调用,这将与所示的简单示例具有相同的效果。此外,对于将 I / O 描述为“阻塞”( PHP,Java )的情况,HTTP 请求和响应读取和写入本身就是阻止调用:系统中隐藏更多 I / O 及其伴随考虑到的性能问题。
|
||||||
|
|
||||||
|
选择一个项目的编程语言有很多因素。当你只考虑效率时,还有很多其它的因素。但是,如果您担心您的程序将主要受到 I/O 的限制,如果 I/O 性能是对项目的成败,那么这些是您需要了解的。## “保持简单”方法:PHP
|
||||||
|
|
||||||
|
早在90年代,很多人都穿着 [Converse][1] 鞋,并在 Perl 中编写了 CGI 脚本。然后 PHP 来了,就像一些人喜欢涂抹一样,它使得动态网页更容易。
|
||||||
|
|
||||||
|
PHP使用的模型相当简单。有一些变化,但您的平均 PHP 服务器来看:
|
||||||
|
|
||||||
|
HTTP请求来自用户的浏览器,并且访问您的 Apache Web 服务器。 Apache 为每个请求创建一个单独的进程,通过一些优化来重新使用它们,以最大限度地减少它需要执行的次数( 相对而言,创建进程较慢 )。Apache 调用 PHP 并告诉它 `.php` 在磁盘上运行相应的文件。PHP 代码执行并阻止 I / O 调用。你调用 `file_get_contents()` , PHP 并在引擎盖下使 read() 系统调用并等待结果。
|
||||||
|
|
||||||
|
当然,实际的代码只是直接嵌入你的页面,并且操作被阻止:
|
||||||
|
|
||||||
|
```
|
||||||
|
<?php
|
||||||
|
|
||||||
|
// blocking file I/O
|
||||||
|
$file_data = file_get_contents(‘/path/to/file.dat’);
|
||||||
|
|
||||||
|
// blocking network I/O
|
||||||
|
$curl = curl_init('http://example.com/example-microservice');
|
||||||
|
$result = curl_exec($curl);
|
||||||
|
|
||||||
|
// some more blocking network I/O
|
||||||
|
$result = $db->query('SELECT id, data FROM examples ORDER BY id DESC limit 100');
|
||||||
|
|
||||||
|
?>
|
||||||
|
|
||||||
|
```
|
||||||
|
|
||||||
|
关于如何与系统集成,就像这样:
|
||||||
|
|
||||||
|
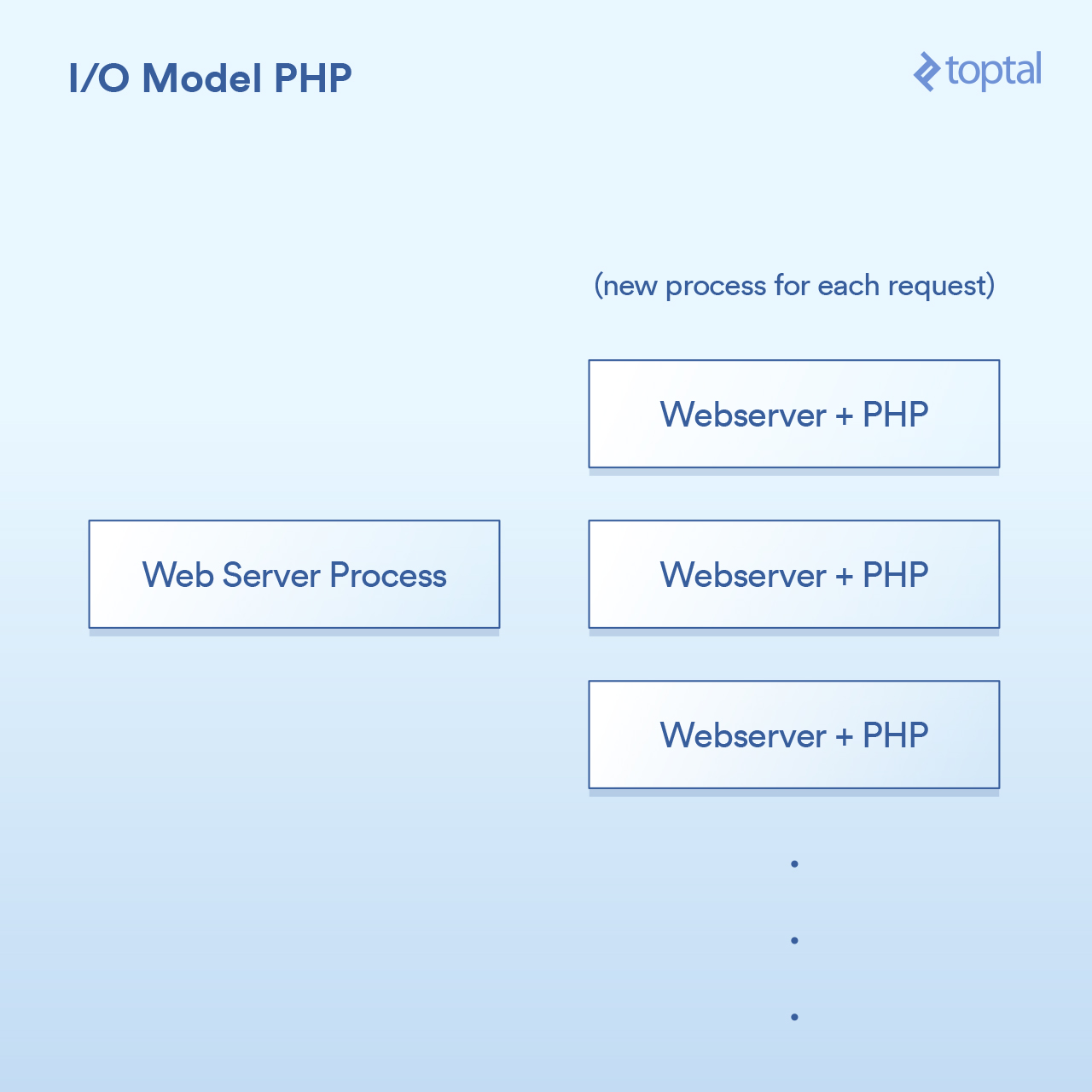
|
||||||
|
|
||||||
|
很简单:每个请求一个进程。 I / O 只是阻塞。优点是简单,缺点是,同时与20,000个客户端连接,您的服务器将会崩溃。这种方法不能很好地扩展,因为内核提供的用于处理大容量 I / O (epoll等) 的工具没有被使用。 为了增加人数,为每个请求运行一个单独的过程往往会使用大量的系统资源,特别是内存,这通常是您在这样一个场景中遇到的第一件事情。
|
||||||
|
|
||||||
|
_注意: Ruby使用的方法与PHP非常相似,在广泛而普遍的手工波浪方式下,它们可以被认为是相同的。_
|
||||||
|
|
||||||
|
### 多线程方法: Java
|
||||||
|
|
||||||
|
所以 Java 来了,就是你购买你的第一个域名的时候,在一个句子后随机说出 “dot com” 很酷。而 Java 具有内置于该语言中的多线程(特别是在创建时)非常棒。
|
||||||
|
|
||||||
|
大多数 Java Web 服务器通过为每个请求启动一个新的执行线程,然后在该线程中最终调用您作为应用程序开发人员编写的函数。
|
||||||
|
|
||||||
|
在 Java Servlet 中执行 I / O 往往看起来像:
|
||||||
|
|
||||||
|
```
|
||||||
|
public void doGet(HttpServletRequest request,
|
||||||
|
HttpServletResponse response) throws ServletException, IOException
|
||||||
|
{
|
||||||
|
|
||||||
|
// blocking file I/O
|
||||||
|
InputStream fileIs = new FileInputStream("/path/to/file");
|
||||||
|
|
||||||
|
// blocking network I/O
|
||||||
|
URLConnection urlConnection = (new URL("http://example.com/example-microservice")).openConnection();
|
||||||
|
InputStream netIs = urlConnection.getInputStream();
|
||||||
|
|
||||||
|
// some more blocking network I/O
|
||||||
|
out.println("...");
|
||||||
|
}
|
||||||
|
|
||||||
|
```
|
||||||
|
|
||||||
|
由于我们 `doGet` 上面的方法对应于一个请求并且在其自己的线程中运行,而不是需要自己内存每个请求单独进程,我们有一个单独的线程。这样有一些好的优点,就像能够在线程之间共享状态,缓存的数据等,因为它们可以访问对方的内存,但是它与调度的交互影响与 PHP 中的内容几乎相同以前的例子。每个请求获得一个新线程和该线程内的各种 I / O 操作块,直到请求被完全处理为止。线程被汇集以最小化创建和销毁它们的成本,但是仍然有数千个连接意味着数千个线程,这对调度程序是不利的。
|
||||||
|
|
||||||
|
重要的里程碑中,在1.4版本的Java(和 1.7 中的重要升级)中,获得了执行非阻塞 I / O 调用的能力。大多数应用程序,网络和其他,不使用它,但至少它是可用的。一些 Java Web 服务器尝试以各种方式利用这一点; 然而,绝大多数部署的 Java 应用程序仍然如上所述工作。
|
||||||
|
|
||||||
|
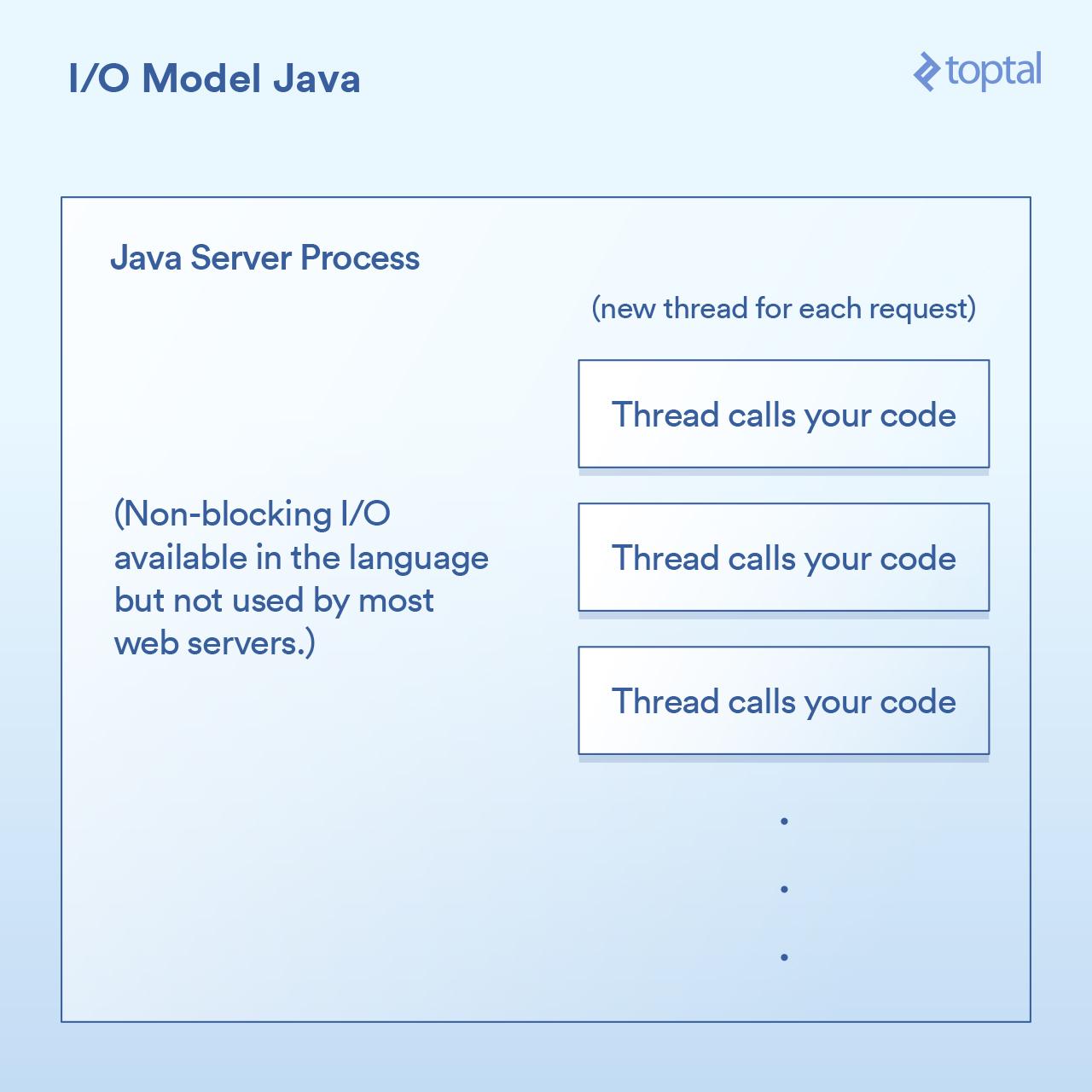
|
||||||
|
|
||||||
|
Java 让我们更接近,肯定有一些很好的开箱即用的 I / O 功能,但它仍然没有真正解决当你有一个大量的 I / O 绑定的应用程序被捣毁时会发生什么的问题,有数千个阻塞线程?。
|
||||||
|
|
||||||
|
<form action="https://www.toptal.com/blog/subscription" class="embeddable_form" data-entity="blog_subscription" data-remote="" data-view="form#form" method="post" style="border: 0px; vertical-align: baseline; min-height: 0px; min-width: 0px;">喜欢你正在阅读什么?首先获取最新的更新。Like what you're reading?Get the latest updates first.<input autocomplete="off" class="input is-medium" data-role="email" name="blog_subscription[email]" placeholder="Enter your email address..." type="text" style="-webkit-appearance: none; background: rgb(250, 250, 250); border-radius: 4px; border-width: 1px; border-style: solid; border-color: rgb(238, 238, 238); color: rgb(60, 60, 60); font-family: proxima-nova, Arial, sans-serif; font-size: 14px; padding: 15px 12px; transition: all 0.2s; width: 799.36px;"><input class="button is-green_candy is-default is-full_width" data-loader-text="Subscribing..." data-role="submit" type="submit" value="Get Exclusive Updates" style="-webkit-appearance: none; font-weight: 600; border-radius: 4px; transition: background 150ms; background: linear-gradient(rgb(67, 198, 146), rgb(57, 184, 133)); border-width: 1px; border-style: solid; border-color: rgb(31, 124, 87); box-shadow: rgb(79, 211, 170) 0px 1px inset; color: rgb(255, 255, 255); position: relative; text-shadow: rgb(28, 143, 61) 0px 1px 0px; font-size: 14px; padding: 15px 20px; width: 549.32px;">没有垃圾邮件。只是伟大的工程职位。</form>
|
||||||
|
|
||||||
|
### 无阻塞 I / O 作为一流公民: Node
|
||||||
|
|
||||||
|
操作块更好的 I / O 是 Node.js. 曾经对 Node 的最简单的介绍的人都被告知这是“非阻塞”,它有效地处理 I / O。这在一般意义上是正确的。但魔鬼的细节和这个巫术的实现手段在涉及演出时是重要的。
|
||||||
|
|
||||||
|
Node实现的范例基本上不是说 “在这里写代码来处理请求”,而是说 “在这里编写代码来开始处理请求”。每次你需要做一些涉及到 I / O ,您提出请求并给出一个回调函数,Node 将在完成之后调用该函数。
|
||||||
|
|
||||||
|
在请求中执行 I / O 操作的典型节点代码如下所示:
|
||||||
|
|
||||||
|
```
|
||||||
|
http.createServer(function(request, response) {
|
||||||
|
fs.readFile('/path/to/file', 'utf8', function(err, data) {
|
||||||
|
response.end(data);
|
||||||
|
});
|
||||||
|
});
|
||||||
|
|
||||||
|
```
|
||||||
|
|
||||||
|
你可以看到,这里有两个回调函数。当请求开始时,第一个被调用,当文件数据可用时,第二个被调用。
|
||||||
|
|
||||||
|
这样做的基本原理是让 Node 有机会有效地处理这些回调之间的 I / O 。在 Node 中进行数据库调用的方式更为相关,但是我不会在这个例子中啰嗦,因为它是完全相同的原则:启动数据库调用,并给 Node 一个回调函数使用非阻塞调用单独执行 I / O 操作,然后在您要求的数据可用时调用回调函数。排队 I / O 调用和让 Node 处理它然后获取回调的机制称为“事件循环”。它的工作原理很好。
|
||||||
|
|
||||||
|
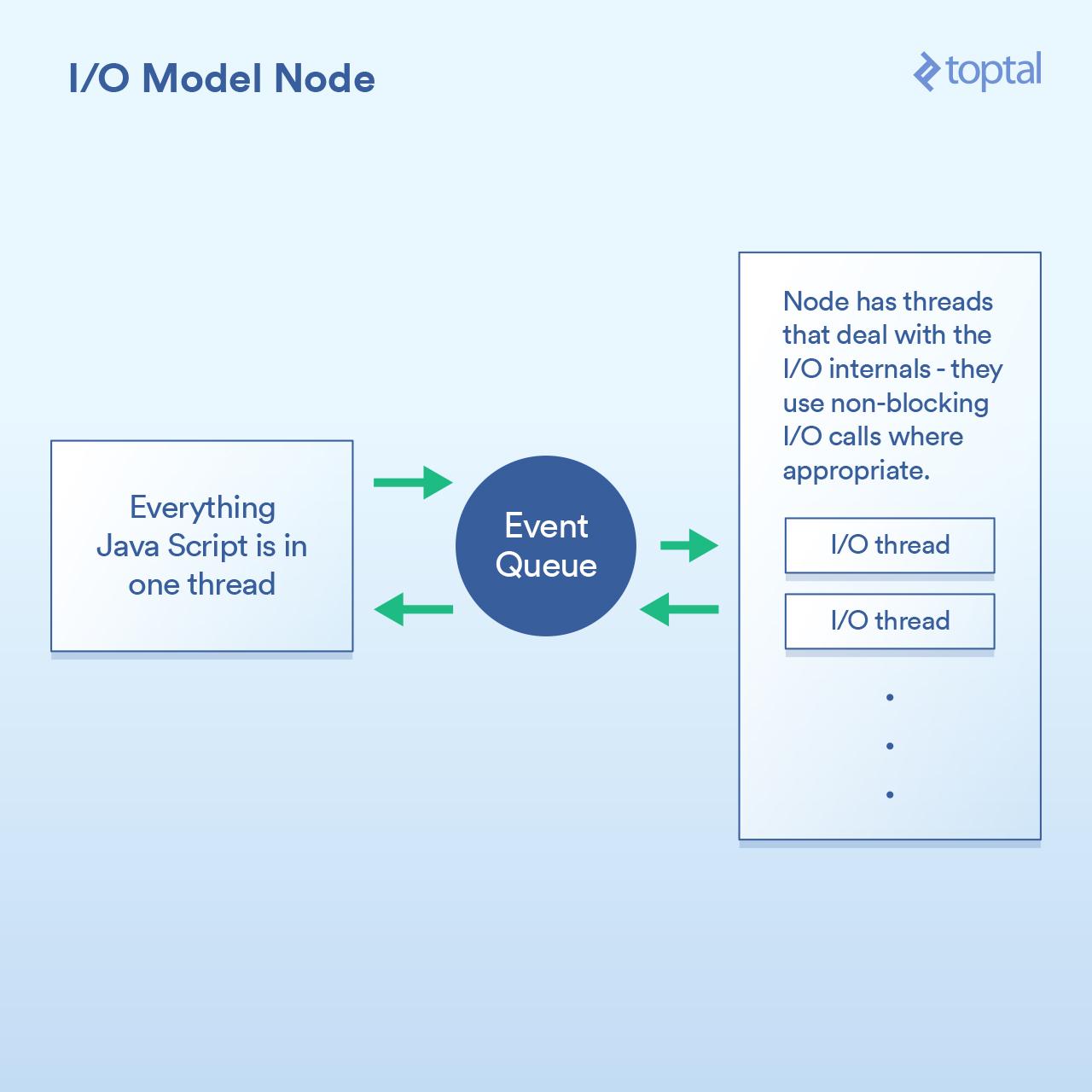
|
||||||
|
|
||||||
|
然而,这个模型的要点是在引擎盖下,其原因有很多更多的是如何在 V8 JavaScript 引擎(即使用节点 Chrome 浏览器的 JS 引擎)实现 [<sup style="border: 0px; vertical-align: super; min-height: 0px; min-width: 0px;">1</sup>][2] 比什么都重要。您编写的所有 JS 代码都运行在单个线程中。想一会儿 这意味着当使用高效的非阻塞技术执行 I / O 时,您的 JS 可以在单个线程中运行 CPU 绑定操作,每个代码块阻止下一个。可能出现这种情况的一个常见例子是在数据库记录之前循环,以某种方式处理它们,然后再将其输出到客户端。这是一个示例,显示如何工作:
|
||||||
|
|
||||||
|
```
|
||||||
|
var handler = function(request, response) {
|
||||||
|
|
||||||
|
connection.query('SELECT ...', function (err, rows) {
|
||||||
|
|
||||||
|
if (err) { throw err };
|
||||||
|
|
||||||
|
for (var i = 0; i < rows.length; i++) {
|
||||||
|
// do processing on each row
|
||||||
|
}
|
||||||
|
|
||||||
|
response.end(...); // write out the results
|
||||||
|
|
||||||
|
})
|
||||||
|
|
||||||
|
};
|
||||||
|
|
||||||
|
```
|
||||||
|
|
||||||
|
虽然 Node 确实有效地处理了 I / O ,但是 `for` 上面的例子中的循环是在你的一个主线程中使用 CPU 周期。这意味着如果您有 10,000 个连接,则该循环可能会使您的整个应用程序进行爬网,具体取决于需要多长时间。每个请求必须在主线程中共享一段时间,一次一个。
|
||||||
|
|
||||||
|
这个整体概念的前提是 I / O 操作是最慢的部分,因此最重要的是要有效地处理这些操作,即使这意味着连续进行其他处理。这在某些情况下是正确的,但不是全部。
|
||||||
|
|
||||||
|
另一点是,虽然这只是一个意见,但是写一堆嵌套回调可能是相当令人讨厌的,有些则认为它使代码更难以遵循。看到回调在 Node 代码中嵌套甚至更多级别并不罕见。
|
||||||
|
|
||||||
|
我们再回到权衡。如果您的主要性能问题是 I / O,则 Node 模型工作正常。然而,它的跟腱是,您可以进入处理 HTTP 请求的功能,并放置 CPU 密集型代码,并将每个连接都抓取。
|
||||||
|
|
||||||
|
### 最自然的非阻塞: Go
|
||||||
|
|
||||||
|
在我进入Go部分之前,我应该披露我是一个Go的粉丝。我已经使用它为许多项目,我公开表示其生产力优势的支持者,我看到他们在我的工作中。
|
||||||
|
|
||||||
|
也就是说,我们来看看它如何处理 I / O 。Go 语言的一个关键特征是它包含自己的调度程序。而不是每个线程的执行对应于一个单一的 OS 线程,它的作用与 “goroutines” 的概念。而 Go 运行时可以将一个 goroutine 分配给一个 OS 线程,并使其执行或暂停它,并且它不与一个 OS 线程,基于 goroutine 正在做什么。来自 Go 的 HTTP 服务器的每个请求都在单独的 Goroutine 中处理。
|
||||||
|
|
||||||
|
调度程序的工作原理如图所示:
|
||||||
|
|
||||||
|
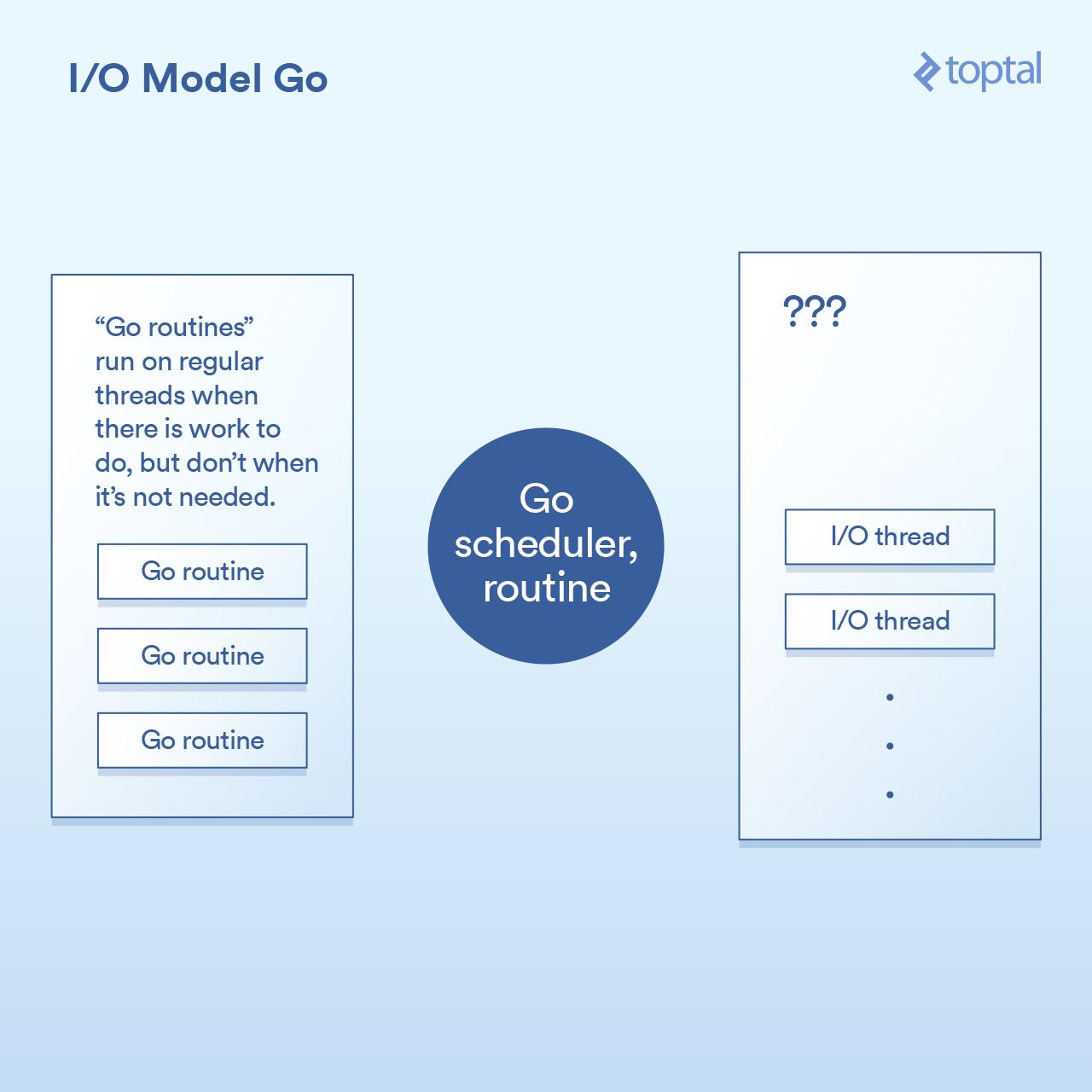
|
||||||
|
|
||||||
|
在引擎下,通过 Go 执行程序中的各个点实现的,通过使当前的 goroutine 进入睡眠状态,通过将请求写入/读取/连接等来实现 I / O 调用,通过将信息唤醒回来可采取进一步行动。
|
||||||
|
|
||||||
|
实际上,Go 运行时正在做一些与 Node 正在做的不太相似的事情,除了回调机制内置到 I / O 调用的实现中,并自动与调度程序交互。它也不会受到必须让所有处理程序代码在同一个线程中运行的限制,Go 将根据其调度程序中的逻辑自动将 Goroutines 映射到其认为适当的 OS 线程。结果是这样的代码:
|
||||||
|
|
||||||
|
```
|
||||||
|
func ServeHTTP(w http.ResponseWriter, r *http.Request) {
|
||||||
|
|
||||||
|
// the underlying network call here is non-blocking
|
||||||
|
rows, err := db.Query("SELECT ...")
|
||||||
|
|
||||||
|
for _, row := range rows {
|
||||||
|
// do something with the rows,
|
||||||
|
// each request in its own goroutine
|
||||||
|
}
|
||||||
|
|
||||||
|
w.Write(...) // write the response, also non-blocking
|
||||||
|
|
||||||
|
}
|
||||||
|
|
||||||
|
```
|
||||||
|
|
||||||
|
如上所述,我们正在做的类似于更简单的方法的基本代码结构,并且在引擎下实现了非阻塞 I / O。
|
||||||
|
|
||||||
|
在大多数情况下,最终都是“两个世界最好的”。非阻塞 I / O 用于所有重要的事情,但是您的代码看起来像是阻塞,因此更容易理解和维护。Go 调度程序和OS调度程序之间的交互处理其余部分。这不是完整的魔法,如果你建立一个大型系统,那么值得我们来看看有关它的工作原理的更多细节; 但与此同时,您获得的“开箱即用”的环境可以很好地工作和扩展。
|
||||||
|
|
||||||
|
Go 可能有其缺点,但一般来说,它处理 I / O 的方式不在其中。
|
||||||
|
|
||||||
|
### 谎言,可恶的谎言和基准
|
||||||
|
|
||||||
|
对这些各种模式的上下文切换进行准确的定时是很困难的。我也可以认为这对你来说不太有用。相反,我会给出一些比较这些服务器环境的 HTTP 服务器性能的基本基准。请记住,整个端到端 HTTP 请求/响应路径的性能有很多因素,这里提供的数字只是我将一些样本放在一起进行基本比较。
|
||||||
|
|
||||||
|
对于这些环境中的每一个,我写了适当的代码以随机字节读取 64k 文件,在其上运行了一个 SHA-256 哈希 N 次( N 在 URL 的查询字符串中指定,例如 .../test.php?n=100),并打印出结果十六进制散列 我选择了这一点,因为使用一些一致的 I / O 和受控的方式来运行相同的基准测试是一个非常简单的方法来增加 CPU 使用率。
|
||||||
|
|
||||||
|
有关使用的环境的更多细节,请参阅 [基准笔记][3] 。
|
||||||
|
|
||||||
|
首先,我们来看一些低并发的例子。运行 2000 次迭代,具有 300 个并发请求,每个请求只有一个散列(N = 1)给我们这样:
|
||||||
|
|
||||||
|
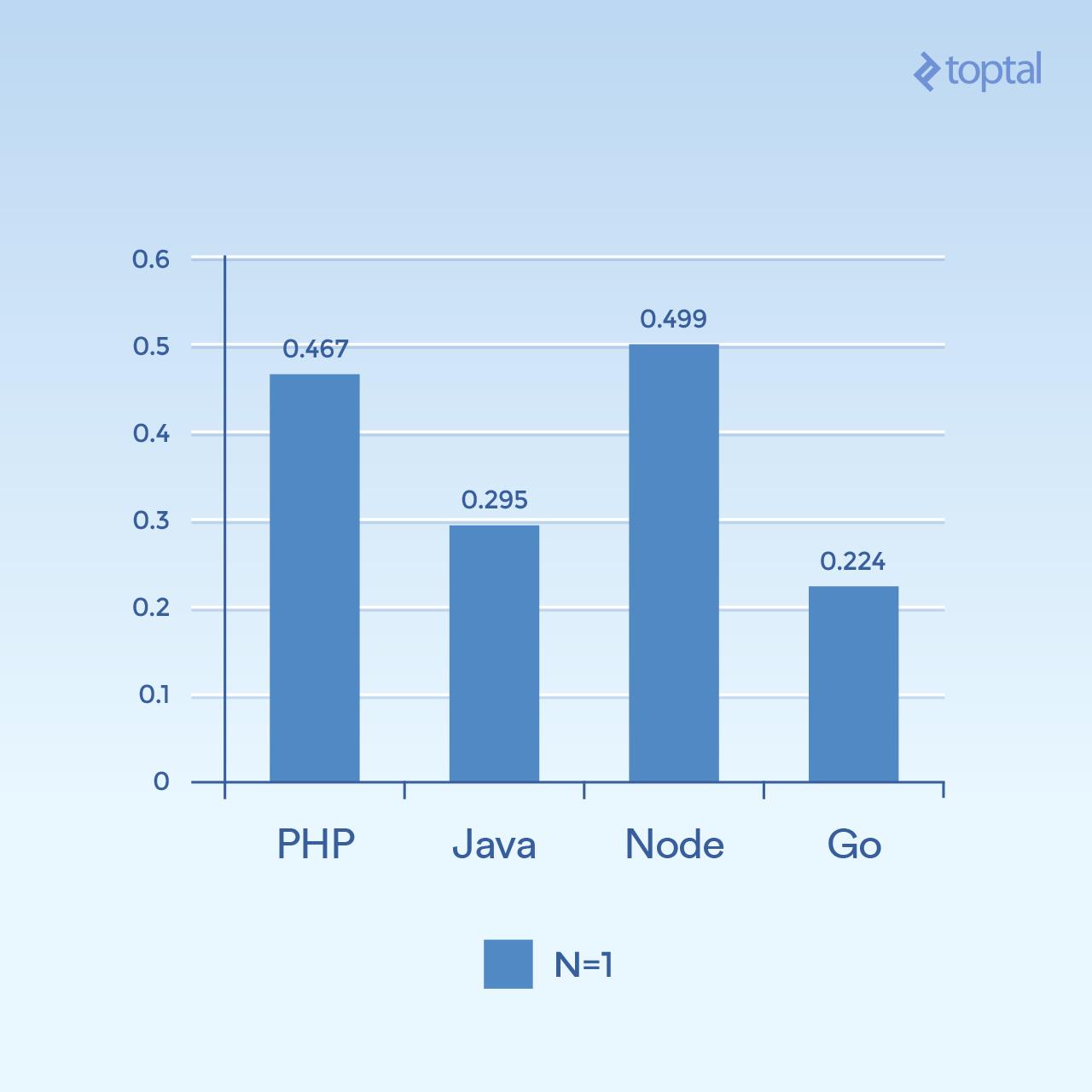
|
||||||
|
|
||||||
|
时间是在所有并发请求中完成请求的平均毫秒数。低于更好。
|
||||||
|
|
||||||
|
很难从这个图中得出结论,但是对我来说,似乎在这个连接和计算量上,我们看到时间更多地与语言本身的一般执行有关,这样更多的是 I / O。请注意,被认为是“脚本语言”(松散类型,动态解释)的语言执行速度最慢。
|
||||||
|
|
||||||
|
但是,如果我们将 N 增加到 1000,仍然有 300 个并发请求,则会发生相同的负载,但是更多的哈希迭代是 100 倍(显着增加了 CPU 负载):
|
||||||
|
|
||||||
|
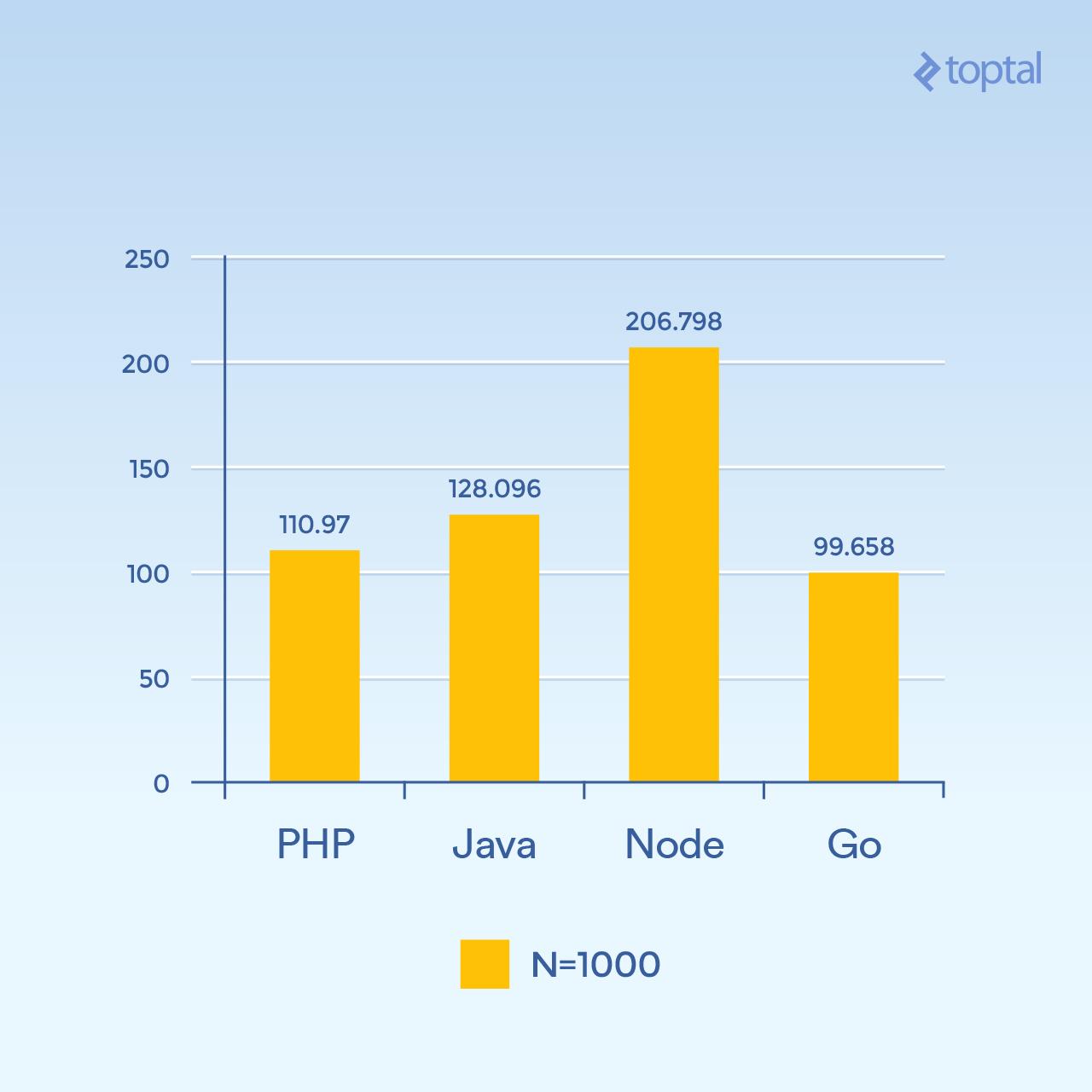
|
||||||
|
|
||||||
|
时间是在所有并发请求中完成请求的平均毫秒数。低于更好。
|
||||||
|
|
||||||
|
突然间,节点性能显着下降,因为每个请求中的 CPU 密集型操作都相互阻塞。有趣的是,在这个测试中,PHP 的性能要好得多(相对于其他的),并且打败了 Java。(值得注意的是,在 PHP 中,SHA-256 实现是用 C 编写的,执行路径在这个循环中花费更多的时间,因为现在我们正在进行 1000 个哈希迭代)。
|
||||||
|
|
||||||
|
现在让我们尝试 5000 个并发连接(N = 1) - 或者接近我可以来的连接。不幸的是,对于大多数这些环境,故障率并不显着。对于这个图表,我们来看每秒的请求总数。 _越高越好_ :
|
||||||
|
|
||||||
|
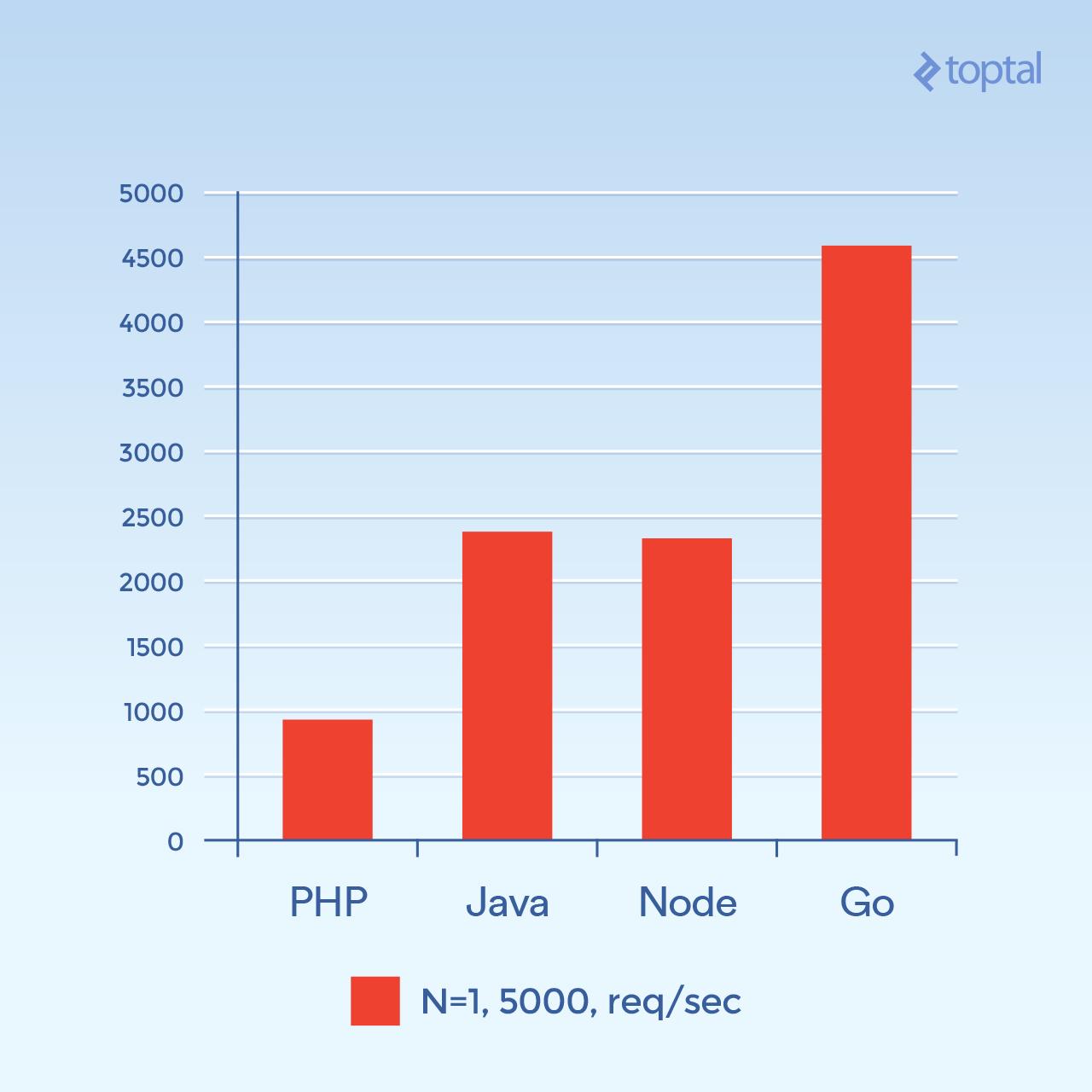
|
||||||
|
|
||||||
|
每秒请求总数。越高越好。
|
||||||
|
|
||||||
|
而且这张照片看起来有很大的不同 这是一个猜测,但是看起来像在高连接量时,产生新进程所涉及的每连接开销以及与 PHP + Apache 相关联的附加内存似乎成为主要因素,并阻止了 PHP 的性能。显然,Go 是这里的赢家,其次是 Java,Node 和 PHP。
|
||||||
|
|
||||||
|
虽然与您的整体吞吐量相关的因素很多,并且在应用程序之间也有很大的差异,但是您了解更多关于发生什么的事情以及所涉及的权衡,您将会越有效。
|
||||||
|
|
||||||
|
### 总结
|
||||||
|
|
||||||
|
以上所有这一切,很显然,随着语言的发展,处理大量 I / O 的大型应用程序的解决方案也随之发展。
|
||||||
|
|
||||||
|
为了公平起见,PHP 和 Java,尽管这篇文章中的描述,确实有 [实现][4] [ 非阻塞I / O][5] 和 [可使用][6] [ web 应用程序][7] 。但是这些方法并不像上述方法那么常见,并且需要考虑使用这种方法来维护服务器的随之而来的操作开销。更不用说您的代码必须以与这些环境相适应的方式进行结构化; 您的 “正常” PHP 或 Java Web 应用程序通常不会在这样的环境中进行重大修改。
|
||||||
|
|
||||||
|
作为比较,如果我们考虑影响性能和易用性的几个重要因素,我们得出以下结论:
|
||||||
|
|
||||||
|
| 语言 | 线程与进程 | 非阻塞 I/O | 使用便捷性 |
|
||||||
|
| --- | --- | --- | --- |
|
||||||
|
| PHP | 进程 | No | |
|
||||||
|
| Java | 线程 | Available | 需要回调 |
|
||||||
|
| Node.js | 线程 | Yes | 需要回调 |
|
||||||
|
| Go | 线程 (Goroutines) | Yes | 不需要回调 |
|
||||||
|
|
||||||
|
线程通常要比进程更高的内存效率,因为它们共享相同的内存空间,而进程没有进程。结合与非阻塞 I / O 相关的因素,我们可以看到,至少考虑到上述因素,当我们向下移动列表时,与 I / O 相关的一般设置得到改善。所以如果我不得不在上面的比赛中选择一个赢家,那肯定会是 Go。
|
||||||
|
|
||||||
|
即使如此,在实践中,选择构建应用程序的环境与您的团队对所述环境的熟悉程度以及您可以实现的总体生产力密切相关。因此,每个团队只需潜入并开始在 Node 或 Go 中开发 Web 应用程序和服务可能就没有意义。事实上,寻找开发人员或您内部团队的熟悉度通常被认为是不使用不同语言和/或环境的主要原因。也就是说,过去十五年来,时代已经发生了变化。
|
||||||
|
|
||||||
|
希望以上内容可以帮助您更清楚地了解引擎下发生的情况,并为您提供如何处理应用程序的现实可扩展性的一些想法。
|
||||||
|
|
||||||
|
--------------------------------------------------------------------------------
|
||||||
|
|
||||||
|
via: https://www.toptal.com/back-end/server-side-io-performance-node-php-java-go
|
||||||
|
|
||||||
|
作者:[ BRAD PEABODY][a]
|
||||||
|
译者:[MonkeyDEcho](https://github.com/MonkeyDEcho)
|
||||||
|
校对:[校对者ID](https://github.com/校对者ID)
|
||||||
|
|
||||||
|
本文由 [LCTT](https://github.com/LCTT/TranslateProject) 原创编译,[Linux中国](https://linux.cn/) 荣誉推出
|
||||||
|
|
||||||
|
[a]:https://www.toptal.com/resume/brad-peabody
|
||||||
|
[1]:https://www.pinterest.com/pin/414401603185852181/
|
||||||
|
[2]:http://www.journaldev.com/7462/node-js-architecture-single-threaded-event-loop
|
||||||
|
[3]:https://peabody.io/post/server-env-benchmarks/
|
||||||
|
[4]:http://reactphp.org/
|
||||||
|
[5]:http://amphp.org/
|
||||||
|
[6]:http://undertow.io/
|
||||||
|
[7]:https://netty.io/
|
@ -0,0 +1,421 @@
|
|||||||
|
使用 Docker 构建你的 Serverless 树莓派集群
|
||||||
|
============================================================
|
||||||
|
|
||||||
|
|
||||||
|
这篇博文将向你展示如何使用 Docker 和 [OpenFaaS][33] 框架构建你自己的 Serverless 树莓派集群。大家常常问我他们能用他们的集群来做些什么?这个应用完美匹配卡片尺寸的设备——只需添加更多的树莓派就能获取更强的计算能力。
|
||||||
|
|
||||||
|
> “Serverless” 是事件驱动架构的一种设计模式,与“桥接模式”、“外观模式”、“工厂模式”和“云”这些名词一样,都是一种抽象概念。
|
||||||
|
|
||||||
|
这是我在本文中描述的集群,用黄铜支架分隔每个设备。
|
||||||
|
|
||||||
|
|
||||||
|
|
||||||
|
### Serverless 是什么?它为何重要?
|
||||||
|
|
||||||
|
行业对于“serverless”这个术语的含义有几种解释。在这篇博文中,我们就把它理解为一种事件驱动的架构模式,它能让你用自己喜欢的任何语言编写轻量可复用的功能。[更多关于 Serverless 的资料][22]。
|
||||||
|
|
||||||
|
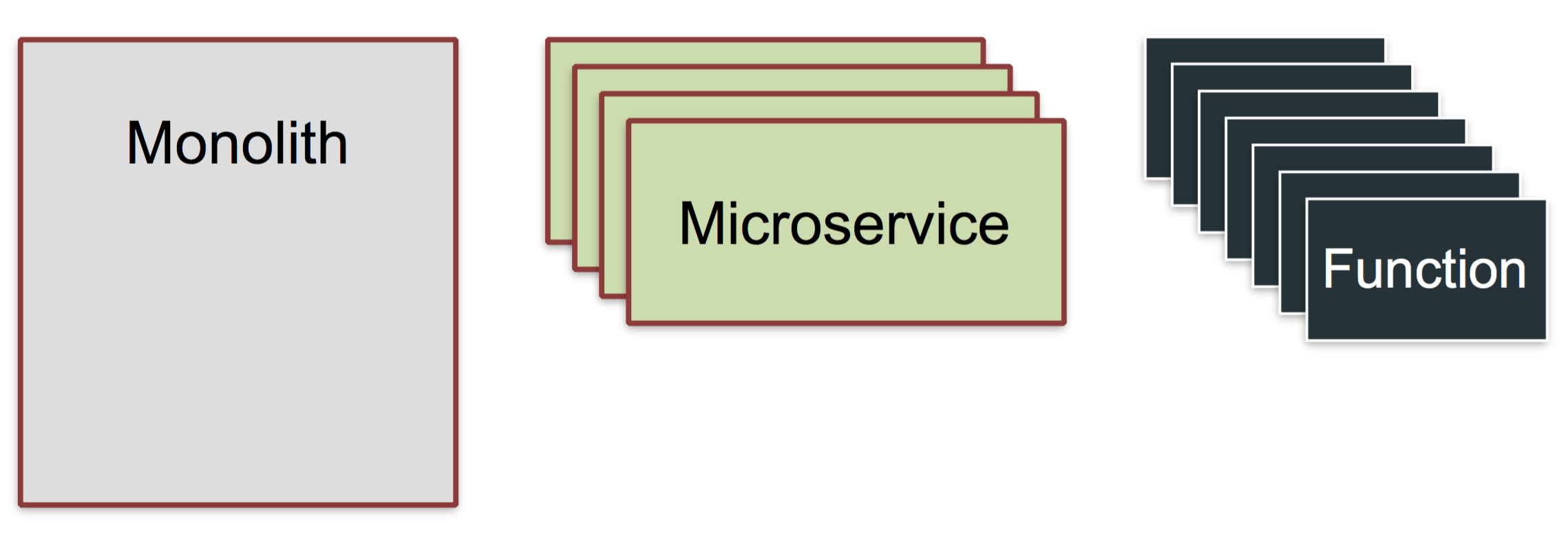
|
||||||
|
_Serverless 架构也引出了“功能即服务服务”模式,简称 FaaS_
|
||||||
|
|
||||||
|
Serverless 的“功能”可以做任何事,但通常用于处理给定的输入——例如来自 GitHub、Twitter、PayPal、Slack、Jenkins CI pipeline 的事件;或者以树莓派为例,处理像红外运动传感器、激光绊网、温度计等真实世界的传感器的输入。
|
||||||
|
|
||||||
|

|
||||||
|
|
||||||
|
Serverless 功能能够更好地结合第三方的后端服务,使系统整体的能力大于各部分之和。
|
||||||
|
|
||||||
|
|
||||||
|
了解更多背景信息,可以阅读我最近一偏博文:[Introducing Functions as a Service (FaaS)][34]。
|
||||||
|
|
||||||
|
### 概述
|
||||||
|
|
||||||
|
我们将使用 [OpenFaaS][35], 它能够让主机或者集群作为支撑 Serverless 功能运行的后端。任何能够使用 Docker 部署的可执行二进制文件、脚本或者编程语言都能在 [OpenFaaS][36] 上运作,你可以根据速度和伸缩性选择部署的规模。另一个优点是,它还内建了用户界面和监控系统。
|
||||||
|
|
||||||
|
这是我们要执行的步骤:
|
||||||
|
|
||||||
|
* 在一个或多个主机上配置 Docker (树莓派 2 或者 3);
|
||||||
|
|
||||||
|
* 利用 Docker Swarm 将它们连接;
|
||||||
|
|
||||||
|
* 部署 [OpenFaaS][23];
|
||||||
|
|
||||||
|
* 使用 Python 编写我们的第一个功能。
|
||||||
|
|
||||||
|
### Docker Swarm
|
||||||
|
|
||||||
|
Docker 是一项打包和部署应用的技术,支持集群上运行,有着安全的默认设置,而且在搭建集群时只需要一条命令。OpenFaaS 使用 Docker 和 Swarm 在你的可用树莓派上传递你的 Serverless 功能。
|
||||||
|
|
||||||
|
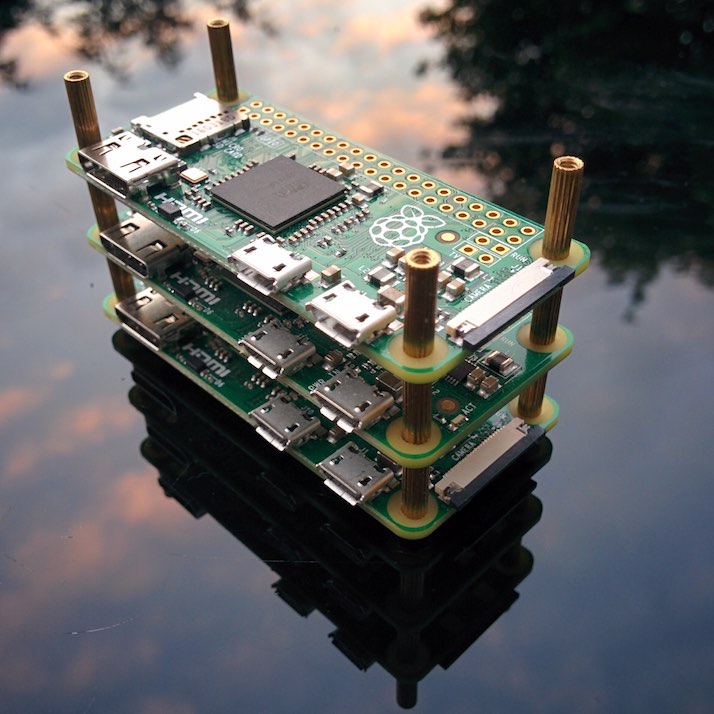
|
||||||
|
_图片:3 个 Raspberry Pi Zero_
|
||||||
|
|
||||||
|
我推荐你在这个项目中使用带树莓派 2 或者 3,以太网交换机和[强大的 USB 多端口电源适配器][37]。
|
||||||
|
|
||||||
|
### 准备 Raspbian
|
||||||
|
|
||||||
|
把 [Raspbian Jessie Lite][38] 写入 SD 卡(8GB 容量就正常工作了,但还是推荐使用 16GB 的 SD 卡)。
|
||||||
|
|
||||||
|
_注意:不要下载成 Raspbian Stretch 了_
|
||||||
|
|
||||||
|
> 社区在努力让 Docker 支持 Raspbian Stretch,但是还未能做到完美运行。请从[树莓派基金会网站][24]下载 Jessie Lite 镜像。
|
||||||
|
|
||||||
|
我推荐使用 [Etcher.io][39] 烧写镜像。
|
||||||
|
|
||||||
|
> 在引导树莓派之前,你需要在引导分区创建名为“ssh”的空白文件。这样才能允许远程登录。
|
||||||
|
|
||||||
|
* 接通电源,然后修改主机名
|
||||||
|
|
||||||
|
现在启动树莓派的电源并且使用`ssh`连接:
|
||||||
|
|
||||||
|
```
|
||||||
|
$ ssh pi@raspberrypi.local
|
||||||
|
|
||||||
|
```
|
||||||
|
|
||||||
|
> 默认密码是`raspberry`
|
||||||
|
|
||||||
|
使用 `raspi-config` 工具把主机名改为 `swarm-1` 或者类似的名字,然后重启。
|
||||||
|
|
||||||
|
当你到了这一步,你还可以把划分给 GPU (显卡)的内存设置为 16MB。
|
||||||
|
|
||||||
|
* 现在安装 Docker
|
||||||
|
|
||||||
|
我们可以使用通用脚本来安装:
|
||||||
|
|
||||||
|
```
|
||||||
|
$ curl -sSL https://get.docker.com | sh
|
||||||
|
|
||||||
|
```
|
||||||
|
|
||||||
|
> 这个安装方式在将来可能会发生变化。如上文所说,你的系统需要是 Jessie,这样才能得到一个确定的配置。
|
||||||
|
|
||||||
|
你可能会看到类似下面的警告,不过你可以安全地忽略它并且成功安装上 Docker CE 17.05:
|
||||||
|
|
||||||
|
```
|
||||||
|
WARNING: raspbian is no longer updated @ https://get.docker.com/
|
||||||
|
Installing the legacy docker-engine package...
|
||||||
|
|
||||||
|
```
|
||||||
|
|
||||||
|
之后,用下面这个命令确保你的用户帐号可以访问 Docker 客户端:
|
||||||
|
|
||||||
|
```
|
||||||
|
$ usermod pi -aG docker
|
||||||
|
|
||||||
|
```
|
||||||
|
|
||||||
|
> 如果你的用户名不是 `pi`,那就把它替换成你的用户名。
|
||||||
|
|
||||||
|
* 修改默认密码
|
||||||
|
|
||||||
|
输入 `$sudo passwd pi`,然后设置一个新密码,请不要跳过这一步!
|
||||||
|
|
||||||
|
* 重复以上步骤
|
||||||
|
|
||||||
|
现在为其它的树莓派重复上述步骤。
|
||||||
|
|
||||||
|
### 创建你的 Swarm 集群
|
||||||
|
|
||||||
|
登录你的第一个树莓派,然后输入下面的命令:
|
||||||
|
|
||||||
|
```
|
||||||
|
$ docker swarm init
|
||||||
|
Swarm initialized: current node (3ra7i5ldijsffjnmubmsfh767) is now a manager.
|
||||||
|
|
||||||
|
To add a worker to this swarm, run the following command:
|
||||||
|
|
||||||
|
docker swarm join \
|
||||||
|
--token SWMTKN-1-496mv9itb7584pzcddzj4zvzzfltgud8k75rvujopw15n3ehzu-af445b08359golnzhncbdj9o3 \
|
||||||
|
192.168.0.79:2377
|
||||||
|
|
||||||
|
```
|
||||||
|
|
||||||
|
你会看到它显示了一个口令,以及其它结点加入集群的命令。接下来使用 `ssh` 登录每个树莓派,运行这个加入集群的命令。
|
||||||
|
|
||||||
|
等待连接完成后,在第一个树莓派上查看集群的结点:
|
||||||
|
|
||||||
|
```
|
||||||
|
$ docker node ls
|
||||||
|
ID HOSTNAME STATUS AVAILABILITY MANAGER STATUS
|
||||||
|
3ra7i5ldijsffjnmubmsfh767 * swarm1 Ready Active Leader
|
||||||
|
k9mom28s2kqxocfq1fo6ywu63 swarm3 Ready Active
|
||||||
|
y2p089bs174vmrlx30gc77h4o swarm4 Ready Active
|
||||||
|
|
||||||
|
```
|
||||||
|
|
||||||
|
恭喜你!你现在拥有一个树莓派集群了!
|
||||||
|
|
||||||
|
* _更多关于集群的内容_
|
||||||
|
|
||||||
|
你可以看到三个结点启动运行。这时只有一个结点是集群管理者。如果我们的管理结点_死机_了,集群就进入了不可修复的状态。我们可以通过添加冗余的管理结点解决这个问题。而且它们依然会运行工作负载,除非你明确设置了让你的服务只运作在工作结点上。
|
||||||
|
|
||||||
|
要把一个工作结点升级为管理结点,只需要在其中一个管理结点上运行 `docker node promote <node_name>` 命令。
|
||||||
|
|
||||||
|
> 注意: Swarm 命令,例如 `docker service ls` 或者 `docker node ls` 只能在管理结点上运行。
|
||||||
|
|
||||||
|
想深入了解管理结点与工作结点如何保持一致性,可以查阅 [Docker Swarm 管理指南][40]。
|
||||||
|
|
||||||
|
### OpenFaaS
|
||||||
|
|
||||||
|
现在我们继续部署程序,让我们的集群能够运行 Serverless 功能。[OpenFaaS][41] 是一个利用 Docker 在任何硬件或者云上让任何进程或者容器成为一个 Serverless 功能的框架。因为 Docker 和 Golang 的可移植性,它也能很好地运行在树莓派上。
|
||||||
|
|
||||||
|
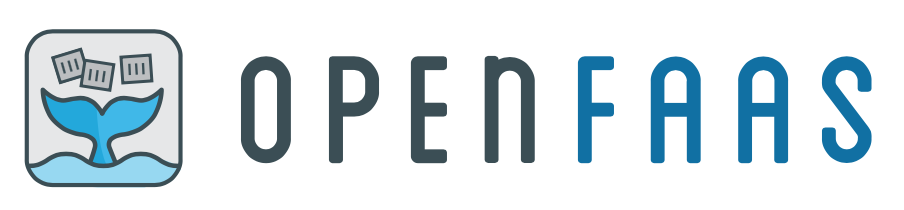
|
||||||
|
|
||||||
|
> 如果你支持 [OpenFaaS][41],希望你能 **start** [OpenFaaS][25] 的 GitHub 仓库。
|
||||||
|
|
||||||
|
登录你的第一个树莓派(你运行 `docker swarm init` 的结点),然后部署这个项目:
|
||||||
|
|
||||||
|
```
|
||||||
|
$ git clone https://github.com/alexellis/faas/
|
||||||
|
$ cd faas
|
||||||
|
$ ./deploy_stack.armhf.sh
|
||||||
|
Creating network func_functions
|
||||||
|
Creating service func_gateway
|
||||||
|
Creating service func_prometheus
|
||||||
|
Creating service func_alertmanager
|
||||||
|
Creating service func_nodeinfo
|
||||||
|
Creating service func_markdown
|
||||||
|
Creating service func_wordcount
|
||||||
|
Creating service func_echoit
|
||||||
|
|
||||||
|
```
|
||||||
|
|
||||||
|
你的其它树莓派会收到 Docer Swarm 的指令,开始从网上拉取这个 Docker 镜像,并且解压到 SD 卡上。这些工作会分布到各个结点上,所以没有哪个结点产生过高的负载。
|
||||||
|
|
||||||
|
这个过程会持续几分钟,你可以用下面指令查看它的完成状况:
|
||||||
|
|
||||||
|
```
|
||||||
|
$ watch 'docker service ls'
|
||||||
|
ID NAME MODE REPLICAS IMAGE PORTS
|
||||||
|
57ine9c10xhp func_wordcount replicated 1/1 functions/alpine:latest-armhf
|
||||||
|
d979zipx1gld func_prometheus replicated 1/1 alexellis2/prometheus-armhf:1.5.2 *:9090->9090/tcp
|
||||||
|
f9yvm0dddn47 func_echoit replicated 1/1 functions/alpine:latest-armhf
|
||||||
|
lhbk1fc2lobq func_markdown replicated 1/1 functions/markdownrender:latest-armhf
|
||||||
|
pj814yluzyyo func_alertmanager replicated 1/1 alexellis2/alertmanager-armhf:0.5.1 *:9093->9093/tcp
|
||||||
|
q4bet4xs10pk func_gateway replicated 1/1 functions/gateway-armhf:0.6.0 *:8080->8080/tcp
|
||||||
|
v9vsvx73pszz func_nodeinfo replicated 1/1 functions/nodeinfo:latest-armhf
|
||||||
|
|
||||||
|
```
|
||||||
|
|
||||||
|
我们希望看到每个服务都显示“1/1”。
|
||||||
|
|
||||||
|
你可以根据服务名查看该服务被调度到哪个树莓派上:
|
||||||
|
|
||||||
|
```
|
||||||
|
$ docker service ps func_markdown
|
||||||
|
ID IMAGE NODE STATE
|
||||||
|
func_markdown.1 functions/markdownrender:latest-armhf swarm4 Running
|
||||||
|
|
||||||
|
```
|
||||||
|
|
||||||
|
状态一项应该显示 `Running`,如果它是 `Pending`,那么镜像可能还在下载中。
|
||||||
|
|
||||||
|
在这时,查看树莓派的 IP 地址,然后在浏览器中访问它的 8080 端口:
|
||||||
|
|
||||||
|
```
|
||||||
|
$ ifconfig
|
||||||
|
|
||||||
|
```
|
||||||
|
|
||||||
|
例如,如果你的 IP 地址是 192.168.0.100,那就访问 [http://192.168.0.100:8080][42]。
|
||||||
|
|
||||||
|
这是你会看到 FaaS UI(也叫 API 网关)。这是你定义、测试、调用功能的地方。
|
||||||
|
|
||||||
|
点击名称为 func_markdown 的 Markdown 转换功能,输入一些 Markdown(这是 Wikipedia 用来组织内容的语言)文本。
|
||||||
|
|
||||||
|
然后点击 `invoke`。你会看到调用计数增加,屏幕下方显示功能调用的结果。
|
||||||
|
|
||||||
|
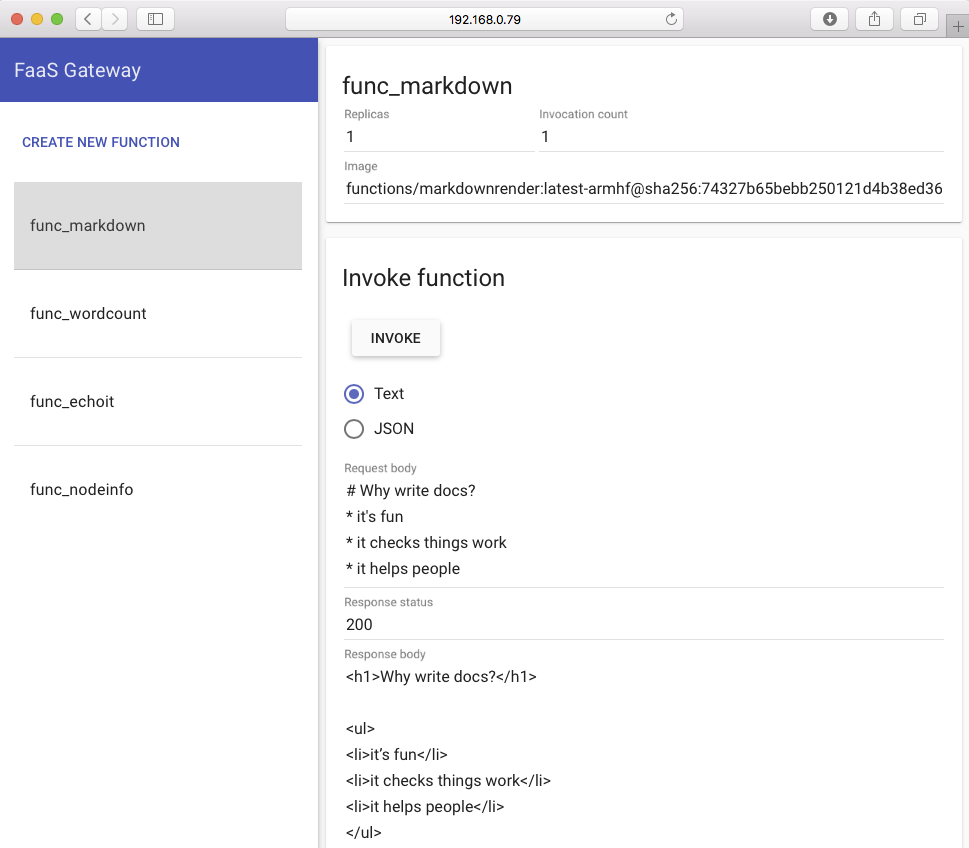
|
||||||
|
|
||||||
|
### 部署你的第一个 Serverless 功能:
|
||||||
|
|
||||||
|
这一节的内容已经有相关的教程,但是我们需要几个步骤来配置树莓派。
|
||||||
|
|
||||||
|
* 获取 FaaS-CLI:
|
||||||
|
|
||||||
|
```
|
||||||
|
$ curl -sSL cli.openfaas.com | sudo sh
|
||||||
|
armv7l
|
||||||
|
Getting package https://github.com/alexellis/faas-cli/releases/download/0.4.5-b/faas-cli-armhf
|
||||||
|
|
||||||
|
```
|
||||||
|
|
||||||
|
* 下载样例:
|
||||||
|
|
||||||
|
```
|
||||||
|
$ git clone https://github.com/alexellis/faas-cli
|
||||||
|
$ cd faas-cli
|
||||||
|
|
||||||
|
```
|
||||||
|
|
||||||
|
* 为树莓派修补样例:
|
||||||
|
|
||||||
|
我们临时修改我们的模版,让它们能在树莓派上工作:
|
||||||
|
|
||||||
|
```
|
||||||
|
$ cp template/node-armhf/Dockerfile template/node/
|
||||||
|
$ cp template/python-armhf/Dockerfile template/python/
|
||||||
|
|
||||||
|
```
|
||||||
|
|
||||||
|
这么做是因为树莓派和我们平时关注的大多数计算机使用不一样的处理器架构。
|
||||||
|
|
||||||
|
> 了解 Docker 在树莓派上的最新状况,请查阅: [5 Things you need to know][26]
|
||||||
|
|
||||||
|
现在你可以跟着下面为 PC,笔记本和云端所写的教程操作,但我们在树莓派上要先运行一些命令。
|
||||||
|
|
||||||
|
* [使用 OpenFaaS 运行你的第一个 Serverless Python 功能][27]
|
||||||
|
|
||||||
|
注意第 3 步:
|
||||||
|
|
||||||
|
* 把你的功能放到先前从 GitHub 下载的 `faas-cli` 文件夹中,而不是 `~/functinos/hello-python` 里。
|
||||||
|
|
||||||
|
* 同时,在 `stack.yml` 文件中把 `localhost` 替换成第一个树莓派的 IP 地址。
|
||||||
|
|
||||||
|
集群可能会花费几分钟把 Serverless 功能下载到相关的树莓派上。你可以用下面的命令查看你的服务,确保副本一项显示“1/1”:
|
||||||
|
|
||||||
|
```
|
||||||
|
$ watch 'docker service ls'
|
||||||
|
pv27thj5lftz hello-python replicated 1/1 alexellis2/faas-hello-python-armhf:latest
|
||||||
|
|
||||||
|
```
|
||||||
|
|
||||||
|
**继续阅读教程:** [使用 OpenFaaS 运行你的第一个 Serverless Python 功能][43]
|
||||||
|
|
||||||
|
关于 Node.js 或者其它语言的更多信息,可以进一步访问 [FaaS 仓库][44]。
|
||||||
|
|
||||||
|
### 检查功能的指标
|
||||||
|
|
||||||
|
既然使用 Serverless,你也不想花时间监控你的功能。幸运的是,OpenFaaS 内建了 [Prometheus][45] 指标检测,这意味着你可以追踪每个功能的运行时长和调用频率。
|
||||||
|
|
||||||
|
_指标驱动自动伸缩_
|
||||||
|
|
||||||
|
如果你给一个功能生成足够的负载,OpenFaaS 将自动扩展你的功能;当需求消失时,你又会回到单一副本的状态。
|
||||||
|
|
||||||
|
这个请求样例你可以复制到浏览器中:
|
||||||
|
|
||||||
|
只要把 IP 地址改成你的即可。
|
||||||
|
|
||||||
|
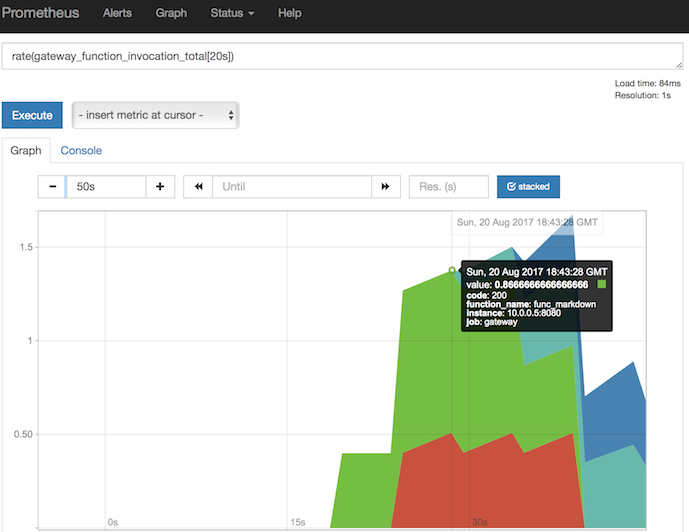
|
||||||
|
|
||||||
|
```
|
||||||
|
http://192.168.0.25:9090/graph?g0.range_input=15m&g0.stacked=1&g0.expr=rate(gateway_function_invocation_total%5B20s%5D)&g0.tab=0&g1.range_input=1h&g1.expr=gateway_service_count&g1.tab=0
|
||||||
|
|
||||||
|
```
|
||||||
|
|
||||||
|
这些请求使用 PromQL(Prometheus 请求语言)编写。第一个请求返回功能调用的频率:
|
||||||
|
|
||||||
|
```
|
||||||
|
rate(gateway_function_invocation_total[20s])
|
||||||
|
|
||||||
|
```
|
||||||
|
|
||||||
|
第二个请求显示每个功能的副本数量,最开始应该是每个功能只有一个副本。
|
||||||
|
|
||||||
|
```
|
||||||
|
gateway_service_count
|
||||||
|
|
||||||
|
```
|
||||||
|
|
||||||
|
如果你想触发自动扩展,你可以在树莓派上尝试下面指令:
|
||||||
|
|
||||||
|
```
|
||||||
|
$ while [ true ]; do curl -4 localhost:8080/function/func_echoit --data "hello world" ; done
|
||||||
|
|
||||||
|
```
|
||||||
|
|
||||||
|
查看 Prometheus 的“alerts”页面,可以知道你是否产生足够的负载来触发自动扩展。如果没有,你可以尝试在多个终端同时运行上面的指令。
|
||||||
|
|
||||||
|
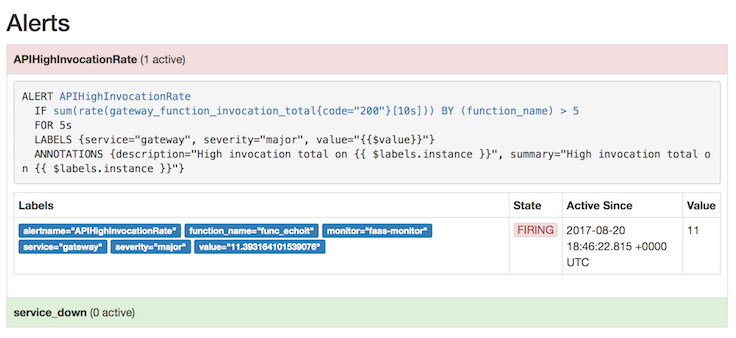
|
||||||
|
|
||||||
|
当你降低负载,副本数量显示在你的第二个图表中,并且 `gateway_service_count` 指标再次降回 1。
|
||||||
|
|
||||||
|
### 结束演讲
|
||||||
|
|
||||||
|
我们现在配置好了 Docker、Swarm, 并且让 OpenFaaS 运行代码,把树莓派像大型计算机一样使用。
|
||||||
|
|
||||||
|
> 希望大家支持这个项目,**Star** [FaaS 的 GitHub 仓库][28]。
|
||||||
|
|
||||||
|
你是如何搭建好了自己的 Docker Swarm 集群并且运行 OpenFaaS 的呢?在 Twitter [@alexellisuk][46] 上分享你的照片或推文吧。
|
||||||
|
|
||||||
|
**观看我在 Dockercon 上关于 OpenFaaS 的视频**
|
||||||
|
|
||||||
|
我在 [Austin 的 Dockercon][47] 上展示了 OpenFaaS。——观看介绍和互动例子的视频:
|
||||||
|
|
||||||
|
** 此处有iframe,请手动处理 **
|
||||||
|
|
||||||
|
有问题?在下面的评论中提出,或者给我发邮件,邀请我进入你和志同道合者讨论树莓派、Docker、Serverless 的 Slack channel。
|
||||||
|
|
||||||
|
**想要学习更多关于树莓派上运行 Docker 的内容?**
|
||||||
|
|
||||||
|
我建议从 [5 Things you need to know][48] 开始,它包含了安全性、树莓派和普通 PC 间微妙差别等话题。
|
||||||
|
|
||||||
|
* [Dockercon tips: Docker & Raspberry Pi][18]
|
||||||
|
|
||||||
|
* [Control GPIO with Docker Swarm][19]
|
||||||
|
|
||||||
|
* [Is that a Docker Engine in your pocket??][20]
|
||||||
|
|
||||||
|
_在 Twitter 上分享_
|
||||||
|
|
||||||
|
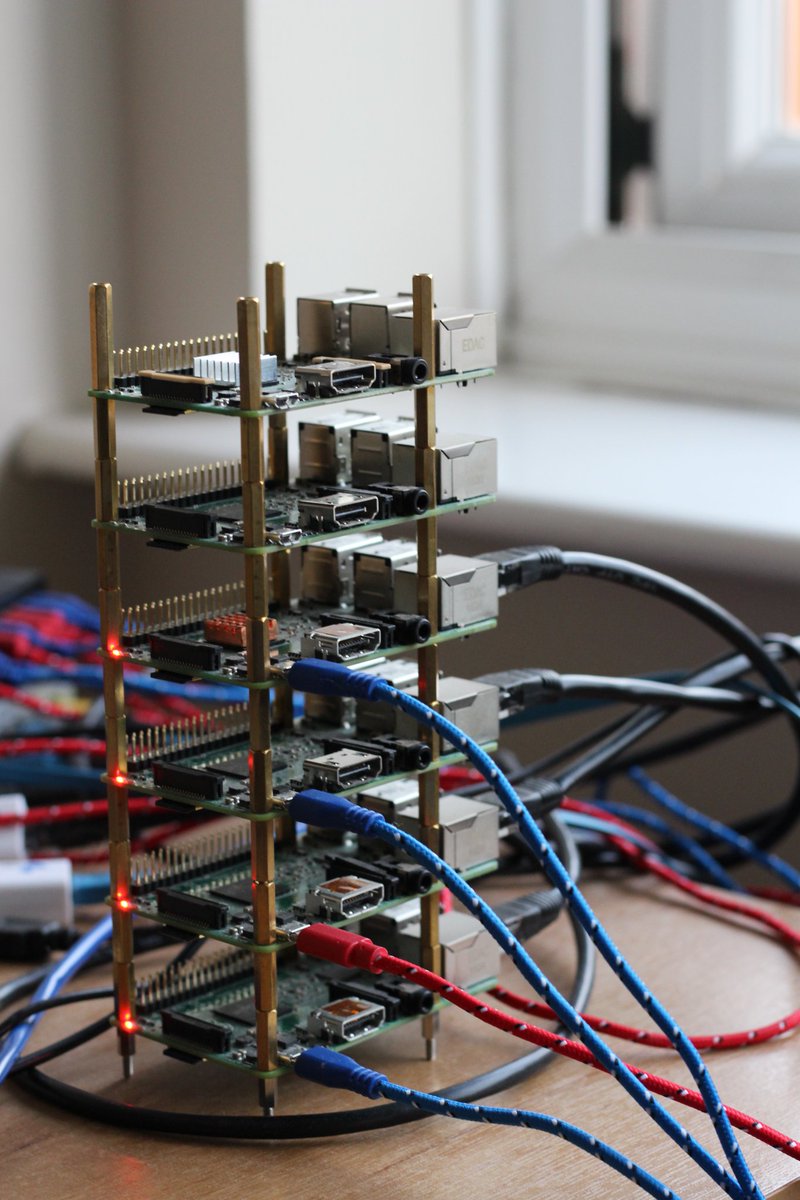
|
||||||
|
|
||||||
|
|
||||||
|
|
||||||
|
--------------------------------------------------------------------------------
|
||||||
|
|
||||||
|
via: https://blog.alexellis.io/your-serverless-raspberry-pi-cluster/
|
||||||
|
|
||||||
|
作者:[Alex Ellis ][a]
|
||||||
|
译者:[haoqixu](https://github.com/haoqixu)
|
||||||
|
校对:[校对者ID](https://github.com/校对者ID)
|
||||||
|
|
||||||
|
本文由 [LCTT](https://github.com/LCTT/TranslateProject) 原创编译,[Linux中国](https://linux.cn/) 荣誉推出
|
||||||
|
|
||||||
|
[a]:https://twitter.com/alexellisuk
|
||||||
|
[1]:https://twitter.com/alexellisuk
|
||||||
|
[2]:https://twitter.com/intent/tweet?in_reply_to=898978596773138436
|
||||||
|
[3]:https://twitter.com/intent/retweet?tweet_id=898978596773138436
|
||||||
|
[4]:https://twitter.com/intent/like?tweet_id=898978596773138436
|
||||||
|
[5]:https://twitter.com/alexellisuk
|
||||||
|
[6]:https://twitter.com/alexellisuk
|
||||||
|
[7]:https://twitter.com/Docker
|
||||||
|
[8]:https://twitter.com/Raspberry_Pi
|
||||||
|
[9]:https://twitter.com/alexellisuk/status/898978596773138436
|
||||||
|
[10]:https://twitter.com/alexellisuk/status/899545370916728832/photo/1
|
||||||
|
[11]:https://twitter.com/alexellisuk
|
||||||
|
[12]:https://twitter.com/alexellisuk/status/898978596773138436/photo/1
|
||||||
|
[13]:https://twitter.com/alexellisuk/status/898978596773138436/photo/1
|
||||||
|
[14]:https://twitter.com/alexellisuk/status/898978596773138436/photo/1
|
||||||
|
[15]:https://twitter.com/alexellisuk/status/898978596773138436/photo/1
|
||||||
|
[16]:https://twitter.com/alexellisuk/status/899545370916728832/photo/1
|
||||||
|
[17]:https://support.twitter.com/articles/20175256
|
||||||
|
[18]:https://blog.alexellis.io/dockercon-tips-docker-raspberry-pi/
|
||||||
|
[19]:https://blog.alexellis.io/gpio-on-swarm/
|
||||||
|
[20]:https://blog.alexellis.io/docker-engine-in-your-pocket/
|
||||||
|
[21]:https://news.ycombinator.com/item?id=15052192
|
||||||
|
[22]:https://blog.alexellis.io/introducing-functions-as-a-service/
|
||||||
|
[23]:https://github.com/alexellis/faas
|
||||||
|
[24]:http://downloads.raspberrypi.org/raspbian_lite/images/raspbian_lite-2017-07-05/
|
||||||
|
[25]:https://github.com/alexellis/faas
|
||||||
|
[26]:https://blog.alexellis.io/5-things-docker-rpi/
|
||||||
|
[27]:https://blog.alexellis.io/first-faas-python-function
|
||||||
|
[28]:https://github.com/alexellis/faas
|
||||||
|
[29]:https://blog.alexellis.io/tag/docker/
|
||||||
|
[30]:https://blog.alexellis.io/tag/raspberry-pi/
|
||||||
|
[31]:https://blog.alexellis.io/tag/openfaas/
|
||||||
|
[32]:https://blog.alexellis.io/tag/faas/
|
||||||
|
[33]:https://github.com/alexellis/faas
|
||||||
|
[34]:https://blog.alexellis.io/introducing-functions-as-a-service/
|
||||||
|
[35]:https://github.com/alexellis/faas
|
||||||
|
[36]:https://github.com/alexellis/faas
|
||||||
|
[37]:https://www.amazon.co.uk/Anker-PowerPort-Family-Sized-Technology-Smartphones/dp/B00PK1IIJY
|
||||||
|
[38]:http://downloads.raspberrypi.org/raspbian/images/raspbian-2017-07-05/
|
||||||
|
[39]:https://etcher.io/
|
||||||
|
[40]:https://docs.docker.com/engine/swarm/admin_guide/
|
||||||
|
[41]:https://github.com/alexellis/faas
|
||||||
|
[42]:http://192.168.0.100:8080/
|
||||||
|
[43]:https://blog.alexellis.io/first-faas-python-function
|
||||||
|
[44]:https://github.com/alexellis/faas
|
||||||
|
[45]:https://prometheus.io/
|
||||||
|
[46]:https://twitter.com/alexellisuk
|
||||||
|
[47]:https://blog.alexellis.io/dockercon-2017-captains-log/
|
||||||
|
[48]:https://blog.alexellis.io/5-things-docker-rpi/
|
@ -0,0 +1,55 @@
|
|||||||
|
PostgreSQL 的哈希索引现在很酷
|
||||||
|
======
|
||||||
|
由于我刚刚提交了最后一个改进 PostgreSQL 11 哈希索引的补丁,并且大部分哈希索引的改进都致力于预计下周发布的 PostgreSQL 10,因此现在似乎是对过去 18 个月左右所做的工作进行简要回顾的好时机。在版本 10 之前,哈希索引在并发性能方面表现不佳,缺少预写日志记录,因此在宕机或复制时都是不安全的,并且还有其他二等公民。在 PostgreSQL 10 中,这在很大程度上被修复了。
|
||||||
|
|
||||||
|
虽然我参与了一些设计,但改进哈希索引的首要功劳来自我的同事 Amit Kapila,[他在这个话题下的博客值得一读][1]。哈希索引的问题不仅在于没有人打算写预写日志记录的代码,还在于代码没有以某种方式进行结构化,使其可以添加实际上正常工作的预写日志记录。要拆分一个桶,系统将锁定已有的桶(使用一种十分低效的锁定机制),将半个元组移动到新的桶中,压缩已有的桶,然后松开锁。即使记录了个别更改,在错误的时刻发生崩溃也会使索引处于损坏状态。因此,Aimt 首先做的是重新设计锁定机制。[新的机制][2]在某种程度上允许扫描和拆分并行进行,并且允许稍后完成那些因报错或崩溃而被中断的拆分。一完成一系列漏洞的修复和一些重构工作,Aimt 就打了另一个补丁,[添加了支持哈希索引的预写日志记录][3]。
|
||||||
|
|
||||||
|
与此同时,我们发现哈希索引已经错过了许多已应用于 B 树多年的相当明显的性能改进。因为哈希索引不支持预写日志记录,以及旧的锁定机制十分笨重,所以没有太多的动机去提升其他的性能。而这意味着如果哈希索引会成为一个非常有用的技术,那么需要做的事只是添加预写日志记录而已。PostgreSQL 索引存取方法的抽象层允许索引保留有关其信息的后端专用缓存,避免了重复查询索引本身来获取相关的元数据。B 树和 SQLite 的索引正在使用这种机制,但哈希索引没有,所以我的同事 Mithun Cy 写了一个补丁来[使用此机制缓存哈希索引的元页][4]。同样,B 树索引有一个称为“单页回收”的优化,它巧妙地从索引页移除没用的索引指针,从而防止了大量索引膨胀,否则这将发生。我的同事 Ashutosh Sharma 打了一个补丁将[这个逻辑移植到哈希索引上][5],也大大减少了索引的膨胀。最后,B 树索引[自 2006 年以来][6]就有了一个功能,可以避免重复锁定和解锁同一个索引页——所有元组都在页中一次性删除,然后一次返回一个。Ashutosh Sharma 也[将此逻辑移植到了哈希索引中][7],但是由于缺少时间,这个优化没有在版本 10 中完成。在这个博客提到的所有内容中,这是唯一一个直到版本 11 才会出现的改进。
|
||||||
|
|
||||||
|
关于哈希索引的工作有一个更有趣的地方是,很难确定行为是否真的正确。锁定行为的更改只可能在繁重的并发状态下失败,而预写日志记录中的错误可能仅在崩溃恢复的情况下显示出来。除此之外,在每种情况下,问题可能是微妙的。没有东西崩溃还不够;它们还必须在所有情况下产生正确的答案,并且这似乎很难去验证。为了协助这项工作,我的同事 Kuntal Ghosh 先后跟进了最初由 Heikki Linnakangas 和 Michael Paquier 开始的工作,并且制作了一个 WAL 一致性检查器,它不仅可以作为开发人员测试的专用补丁,还能真正[致力于 PostgreSQL][8]。在提交之前,我们对哈希索引的预写日志代码使用此工具进行了广泛的测试,并十分成功地查找到了一些漏洞。这个工具并不仅限于哈希索引,相反:它也可用于其他模块的预写日志记录代码,包括堆,当今的 AMS 索引,以及一些以后开发的其他东西。事实上,它已经成功地[在 BRIN 中找到了一个漏洞][9]。
|
||||||
|
|
||||||
|
虽然 WAL 一致性检查是主要的开发者工具——尽管它也适合用户使用,如果怀疑有错误——也可以升级到专为数据库管理人员提供的几种工具。Jesper Pedersen 写了一个补丁来[升级 pageinspect contrib 模块来支持哈希索引][10],Ashutosh Sharma 做了进一步的工作,Peter Eisentraut 提供了测试用例(这是一个很好的办法,因为这些测试用例迅速失败,引发了几轮漏洞修复)。多亏了 Ashutosh Sharma 的工作,pgstattuple contrib 模块[也支持哈希索引了][11]。
|
||||||
|
|
||||||
|
一路走来,也有一些其他性能的改进。我一开始没有意识到的是,当一个哈希索引开始新一轮的桶拆分时,磁盘上的大小会突然加倍,这对于 1MB 的索引来说并不是一个问题,但是如果你碰巧有一个 64GB 的索引,那就有些不幸了。Mithun Cy 通过编写一个补丁,把加倍[分为四个阶段][12]在某个程度上解决了这一问题,这意味着我们将从 64GB 到 80GB 到 96GB 到 112GB 到 128GB,而不是一次性从 64GB 到 128GB。这个问题可以进一步改进,但需要对磁盘格式进行更深入的重构,并且需要仔细考虑对查找性能的影响。
|
||||||
|
|
||||||
|
七月时,一份[来自于“AP”测试人员][13]的报告使我们感到需要做进一步的调整。AP 发现,若试图将 20 亿行数据插入到新创建的哈希索引中会导致错误。为了解决这个问题,Amit 修改了拆分桶的代码,[使得在每次拆分之后清理旧的桶][14],大大减少了溢出页的累积。为了得以确保,Aimt 和我也[增加了四倍的位图页的最大数量][15],用于跟踪溢出页分配。
|
||||||
|
|
||||||
|
虽然还是有更多的事情要做,但我觉得,我和我的同事们——以及在 PostgreSQL 团队中的其他人的帮助下——已经完成了我们的目标,使哈希索引成为一个一流的功能,而不是被严重忽视的半成品。不过,你或许会问,这个功能可能有哪些应用场景。我在文章开头提到的(以及链接中的)Amit 的博客内容表明,即使是 pgbench 的工作负载,哈希索引页也可能在低级和高级并发发面优于 B 树。然而,从某种意义上说,这确实是最坏的情况。哈希索引的卖点之一是,索引存储的是字段的哈希值,而不是原始值——所以,我希望像 UUID 或者长字符串的宽键将有更大的改进。它们可能会在读取繁重的工作负载时做得更好。我们没有像优化读取那种程度来优化写入,但我鼓励任何对此技术感兴趣的人去尝试并将结果发到邮件列表(或发私人电子邮件),因为对于开发一个功能而言,真正关键的并不是一些开发人员去思考在实验室中会发生什么,而是在实际中发生了什么。
|
||||||
|
|
||||||
|
最后,我要感谢 Jeff Janes 和 Jesper Pedersen 为这个项目及其相关所做的宝贵的测试工作。这样一个规模适当的项目并不易得,以及有一群坚持不懈的测试人员,他们勇于打破任何废旧的东西的决心起了莫大的帮助。除了以上提到的人之外,其他人同样在测试,审查以及各种各样的日常帮助方面值得赞扬,其中包括 Andreas Seltenreich,Dilip Kumar,Tushar Ahuja,Alvaro Herrera,Micheal Paquier,Mark Kirkwood,Tom Lane,Kyotaro Horiguchi。谢谢你们,也同样感谢那些本该被提及却被我无意中忽略的所有朋友。
|
||||||
|
|
||||||
|
---
|
||||||
|
via:https://rhaas.blogspot.jp/2017/09/postgresqls-hash-indexes-are-now-cool.html?showComment=1507079869582#c6521238465677174123
|
||||||
|
|
||||||
|
作者:[Robert Haas][a]
|
||||||
|
译者:[polebug](https://github.com/polebug)
|
||||||
|
校对:[校对者ID](https://github.com/id)
|
||||||
|
本文由[LCTT](https://github.com/LCTT/TranslateProject)原创编译,[Linux中国](https://linux.cn/)荣誉推出
|
||||||
|
|
||||||
|
[a]:http://rhaas.blogspot.jp
|
||||||
|
[1]:http://amitkapila16.blogspot.jp/2017/03/hash-indexes-are-faster-than-btree.html
|
||||||
|
[2]:https://git.postgresql.org/gitweb/?p=postgresql.git;a=commitdiff;h=6d46f4783efe457f74816a75173eb23ed8930020
|
||||||
|
[3]:https://git.postgresql.org/gitweb/?p=postgresql.git;a=commitdiff;h=c11453ce0aeaa377cbbcc9a3fc418acb94629330
|
||||||
|
[4]:https://git.postgresql.org/gitweb/?p=postgresql.git;a=commitdiff;h=293e24e507838733aba4748b514536af2d39d7f2
|
||||||
|
[5]:https://git.postgresql.org/gitweb/?p=postgresql.git;a=commitdiff;h=6977b8b7f4dfb40896ff5e2175cad7fdbda862eb
|
||||||
|
[6]:https://git.postgresql.org/gitweb/?p=postgresql.git;a=commitdiff;h=7c75ef571579a3ad7a1d3ee909f11dba5e0b9440
|
||||||
|
[7]:https://git.postgresql.org/gitweb/?p=postgresql.git;a=commitdiff;h=a507b86900f695aacc8d52b7d2cfcb65f58862a2
|
||||||
|
[8]:https://git.postgresql.org/gitweb/?p=postgresql.git;a=commitdiff;h=7403561c0f6a8c62b79b6ddf0364ae6c01719068
|
||||||
|
[9]:https://git.postgresql.org/gitweb/?p=postgresql.git;a=commitdiff;h=08bf6e529587e1e9075d013d859af2649c32a511
|
||||||
|
[10]:https://git.postgresql.org/gitweb/?p=postgresql.git;a=commitdiff;h=e759854a09d49725a9519c48a0d71a32bab05a01
|
||||||
|
[11]:https://git.postgresql.org/gitweb/?p=postgresql.git;a=commitdiff;h=ea69a0dead5128c421140dc53fac165ba4af8520
|
||||||
|
[12]:https://www.postgresql.org/message-id/20170704105728.mwb72jebfmok2nm2@zip.com.au
|
||||||
|
[13]:https://git.postgresql.org/gitweb/?p=postgresql.git;a=commitdiff;h=ff98a5e1e49de061600feb6b4de5ce0a22d386af
|
||||||
|
[14]:https://git.postgresql.org/gitweb/?p=postgresql.git;a=commitdiff;h=ff98a5e1e49de061600feb6b4de5ce0a22d386af
|
||||||
|
[15]:https://www.postgresql.org/message-id/CA%2BTgmoax6DhnKsuE_gzY5qkvmPEok77JAP1h8wOTbf%2Bdg2Ycrw%40mail.gmail.com
|
||||||
|
|
||||||
|
|
||||||
|
|
||||||
|
|
||||||
|
|
||||||
|
|
||||||
|
|
||||||
|
|
||||||
|
|
||||||
|
|
||||||
|
|
93
translated/tech/20171011 Why Linux Works.md
Normal file
93
translated/tech/20171011 Why Linux Works.md
Normal file
@ -0,0 +1,93 @@
|
|||||||
|
Linux 是如何运作的
|
||||||
|
============================================================
|
||||||
|
|
||||||
|
_在大量金钱与围绕 Linux 激烈争夺的公司之间的,正是那些真正给操作系统带来活力的开发者。_
|
||||||
|
|
||||||
|
实际上,[Linux 社区][7]本身无需太过担心社区的正常运作。Linux 已经尽可能的在多个领域占据着主导的地位 —— 从超级计算机到移动设备再到云计算 —— Linux 内核开发人员更多的是关注于代码本身,而不是其所在公司的利益。
|
||||||
|
|
||||||
|
这是一个出现在[Dawn Foster 博士的成果][8]中关于 Linux 内核的合作审查的著名的结论。Foster 博士是在英特尔公司和木偶实验室的前社区领导人,他写到,“很多人优先把自己看作是 Linux 内核开发者,其次才是作为一名雇员。”
|
||||||
|
|
||||||
|
大量的“基金洗劫型”公司已经强加于各种开源项目上,意图在虚构的社区面具之下隐藏企业特权,但 Linux 一直设法保持自身的纯粹。问题是怎么做到。
|
||||||
|
|
||||||
|
**跟随金钱的脚步**
|
||||||
|
|
||||||
|
毕竟,如果有开源项目需参与到企业的利欲中,那它一定是 Linux。回到 2008 年,[ Linux 生态系统的估值已经达到了最高 250 亿美元][9]。最近10年,伴随着数量众多的云服务,移动端,以及大数据基础设施对于 Linux 的依赖,这一数值一定还在急剧增长。甚至在像 Oracle 这样的单独一个公司,就实现了十亿美元的价值。
|
||||||
|
|
||||||
|
那么有点惊奇,这里有这样一个 landgrab 通过代码来影响 Linux 的方向。
|
||||||
|
|
||||||
|
看看在过去一年中那些对 Linux 最活跃的贡献者以及这些企业像“海龟”背地球一样撑起的版图, 就像[Linux 基金会的最新报道][10]中的截图:
|
||||||
|
|
||||||
|

|
||||||
|
|
||||||
|
这些企业花费大量的资金来雇佣开发者去构建自由软件,并且每个企业都有赖于这些投资所带来的回报。因为借由 Linux 潜在的企业灵活性,导致一些企业对 Linux 基金会的领导人[表示不满][11]。在像 Microsoft 这样曾为开源界宿敌的企业加入 Linux 基金会之后,这些批评言论正变得越来越响亮。
|
||||||
|
|
||||||
|
但老实说,这样一位假想的宿敌已经有点过时了。
|
||||||
|
|
||||||
|
虽然企业排队资金赞助 Linux 基金会已经成为了事实,不过这些赞助并没有收买基金会而影响到代码。在最伟大的开源社区中,金钱可以帮助招募开发者,但这些开发者相比企业更优先专注于代码。就像 Linux 基金会执行董事[ Jim Zemlin 强调的那样][12]:
|
||||||
|
|
||||||
|
“我们的项目中技术角色都是独立于企业的。没有人会在其提交的内容上标记他们的企业身份:在 Linux 基金会的项目中进行密切的代码交流。在我们的项目中,开发者可以从一个公司切换到另一个公司并且不会改变他们在项目中所扮演的角色。之后企业或政府采纳了这些代码所创造的价值,反过来可以使该项目获得投资。这样的良性循环对大家都有好处,并且也是我们项目的目标之一。”
|
||||||
|
|
||||||
|
读过 [Linus Torvalds 的][13] 的邮寄列表批注的人很难相信他就曾是这样的企业的上当者。对其他杰出贡献者保持同样的信任。他们总是普遍被大公司所雇佣,通常这些企业实际上会为开发者已经有意识的去完成并已经在进行的工作支付了一定的费用。
|
||||||
|
|
||||||
|
归根结底,很少有公司会有耐心或者必备的风险预测来为一群 Linux 内核骇客提供资金,并在内核团队有影响力的位置为一些他们 _可能_ 贡献质量足够的代码等上数年时间。所以他们选择雇佣已有的值得信赖的开发者。正如 [2016 Linux 基金会报告][14]所写的,“无薪开发者的数量继续[d]缓慢下降,同时 Linux 内核的开发证明是雇主们对有价值的技能需求日益增长,确保了有经验的 kernel 开发者不会在无薪阶段停留太长时间。”
|
||||||
|
|
||||||
|
这是代码所带来的信任,并不是通过企业的金钱。因此没有一个 Linux 内核开发者会为眼前的金钱而丢掉他们已经积攒的信任,那样会在出现新的利益冲突时妥协代码质量并很快失去信任。
|
||||||
|
|
||||||
|
**不是康巴亚,就是权利的游戏,非此即彼**
|
||||||
|
|
||||||
|
最终,Linux 内核开发是关于认同, Foster 的部分研究是这样认为的。
|
||||||
|
|
||||||
|
在 Google 工作会很棒,而且也许带有一个体面的头衔以及免费的干洗。然而,作为一个重要的 Linux 内核子系统的维护人员,很难承诺并保证,不会被其他提供更高薪水的公司所雇佣。
|
||||||
|
|
||||||
|
Foster 这样写到, “他们甚至享受当前的工作并且觉得他们的雇主不错,许多 [Linux 内核开发者] 倾向于审视一些临时的工作关系,而且他们作为内核开发者的身份被看作更有经验且更加重要。”
|
||||||
|
|
||||||
|
由于作为一名 Linux 开发者的身份优先,企业职员的身份第二,Linux 内核开发者甚至可以轻松地与其雇主的竞争对手合作。因为雇主们无力去引导他们开发者的工作,这也呼应了上边的原因。Foster 深入研究了这一问题:
|
||||||
|
|
||||||
|
“尽管企业对其雇员所贡献的领域产生了一些影响,在他们如何去完成工作这点上,雇员还是很自由的。许多人在日常工作中几乎没有接受任何指导,来自雇主的信任对工作是非常有帮助的。然而,他们偶尔会被要求做一些特定的零碎工作或者是在一个对公司重要的特定领域投入兴趣。
|
||||||
|
|
||||||
|
许多内核开发者同样与他们的竞争者进行日常的基础协作,在这里他们仅作为个人相互交流而不需要关心雇主之间的竞争。这是我在 Intel 工作时经常见到的一幕,因为我们内核开发者几乎都是与我们主要的竞争对手一同工作的。”
|
||||||
|
|
||||||
|
那些企业会在芯片上通过运行 Linux,或 Linux 发行版,亦或者是被其他健壮的操作系统支持的软件来进行竞争,但开发者们主要专注于一件事情:尽可能的使用 Linux 。同样,这是因为他们的身份被捆在 Linux 上,而不是坐在防火墙后面写代码。
|
||||||
|
|
||||||
|
Foster 通过 USB 子系统邮寄列表(在 2013 年到 2015 年之间)说明了这种相互作用,用深色线条着重描绘的公司之间电子邮件交互:
|
||||||
|
|
||||||
|

|
||||||
|
|
||||||
|
在价格讨论中很明显一些公司可能会在反垄断的权利中增加疑虑,但这种简单的商业行为在 Linux 大陆中一如既往。结果导致为各方产生一个操作系统并迫使他们在自由市场相互竞争。
|
||||||
|
|
||||||
|
**寻找合适的平衡**
|
||||||
|
|
||||||
|
Novell 公司的创始人 Ray Noorda 或许就是这样在最佳的开源社区之间的“合作竞争”,但只工作在真正的社区存在的地方。这很难做到,举个例子,为一个由单一供应商所主导的项目实现正确的紧张合作。由 Google 发起的[Kubernetes][15]就表明这是可能的,但其他的像是 Docker 这样的项目却在为同样的目标而挣扎,很大一部分原因是他们一直不愿放弃对自己项目的技术领导。
|
||||||
|
|
||||||
|
也许 Kubernetes 能够很好的工作是因为 Google 并不觉得必须占据重要地位,而且事实上,是 _希望_ 其他公司担负起开发领导的职责。通过一个梦幻般的代码库,如果 Google 帮助培养,就有利于像 Kubernetes 这样的项目获得成功,然后开辟一条道路,这就鼓励了 Red Hat 及其他公司做出杰出的贡献。
|
||||||
|
|
||||||
|
不过,Kubernetes 是个例外,就像 Linux 曾经那样。这里有许多 _因为_ 企业的利欲而获得成功的例子,并且在利益竞争中获取平衡。如果一个项目仅仅被公司自己的利益所控制,常常会在公司的技术管理上体现出来,而且再怎么开源许可也无法对企业产生影响。
|
||||||
|
|
||||||
|
简而言之,Linux 的运作是因为众多企业都想要控制它但却难以做到,由于其在工业中的重要性,使得开发者和构建人员更加灵活的作为一名 _Linux 开发者_ 而不是 Red Hat (或 Intel 亦或 Oracle … )工程师。
|
||||||
|
|
||||||
|
--------------------------------------------------------------------------------
|
||||||
|
|
||||||
|
via: https://www.datamation.com/open-source/why-linux-works.html
|
||||||
|
|
||||||
|
作者:[Matt Asay][a]
|
||||||
|
译者:[softpaopao](https://github.com/softpaopao)
|
||||||
|
校对:[校对者ID](https://github.com/校对者ID)
|
||||||
|
|
||||||
|
本文由 [LCTT](https://github.com/LCTT/TranslateProject) 原创编译,[Linux中国](https://linux.cn/) 荣誉推出
|
||||||
|
|
||||||
|
[a]:https://www.datamation.com/author/Matt-Asay-1133910.html
|
||||||
|
[1]:https://www.datamation.com/feedback/https://www.datamation.com/open-source/why-linux-works.html
|
||||||
|
[2]:https://www.datamation.com/author/Matt-Asay-1133910.html
|
||||||
|
[3]:https://www.datamation.com/e-mail/https://www.datamation.com/open-source/why-linux-works.html
|
||||||
|
[4]:https://www.datamation.com/print/https://www.datamation.com/open-source/why-linux-works.html
|
||||||
|
[5]:https://www.datamation.com/open-source/why-linux-works.html#comment_form
|
||||||
|
[6]:https://www.datamation.com/author/Matt-Asay-1133910.html
|
||||||
|
[7]:https://www.datamation.com/open-source/
|
||||||
|
[8]:https://opensource.com/article/17/10/collaboration-linux-kernel
|
||||||
|
[9]:http://www.osnews.com/story/20416/Linux_Ecosystem_Worth_25_Billion
|
||||||
|
[10]:https://www.linux.com/publications/linux-kernel-development-how-fast-it-going-who-doing-it-what-they-are-doing-and-who-5
|
||||||
|
[11]:https://www.datamation.com/open-source/the-linux-foundation-and-the-uneasy-alliance.html
|
||||||
|
[12]:https://thenewstack.io/linux-foundation-critics/
|
||||||
|
[13]:https://github.com/torvalds
|
||||||
|
[14]:https://www.linux.com/publications/linux-kernel-development-how-fast-it-going-who-doing-it-what-they-are-doing-and-who-5
|
||||||
|
[15]:https://kubernetes.io/
|
@ -0,0 +1,113 @@
|
|||||||
|
为什么要在 Docker 中使用 R? 一位 DevOps 的视角
|
||||||
|
============================================================
|
||||||
|
|
||||||
|
[][11]
|
||||||
|
|
||||||
|
有几篇关于为什么要在 Docker 中使用 R 的文章。在这篇文章中,我将尝试加入一个 DevOps 的观点,并解释在 OpenCPU 系统的上下文中如何使用容器化 R 来构建和部署 R 服务器。
|
||||||
|
|
||||||
|
> 有在 [#rstats][2] 世界的人真正地写*为什么*他们使用 Docker,而不是*如何*么?
|
||||||
|
>
|
||||||
|
> — Jenny Bryan (@JennyBryan) [September 29, 2017][3]
|
||||||
|
|
||||||
|
### 1:轻松开发
|
||||||
|
|
||||||
|
OpenCPU 系统的旗舰是[ OpenCPU 服务器][12]:它是一个成熟且强大的 Linux 栈,用于在系统和应用程序中嵌入 R。因为 OpenCPU 是完全开源的,我们可以在 DockerHub 上构建和发布。可以使用以下命令启动(使用端口8004或80)一个可以立即使用的 OpenCPU 和 RStudio 的 Linux 服务器:
|
||||||
|
|
||||||
|
```
|
||||||
|
docker run -t -p 8004:8004 opencpu/rstudio
|
||||||
|
|
||||||
|
```
|
||||||
|
|
||||||
|
现在只需在你的浏览器打开 [http://localhost:8004/ocpu/][13] 和 [http://localhost:8004/rstudio/][14]!在 rstudio 中用用户 `opencpu`(密码:`opencpu`)登录来构建或安装应用程序。有关详细信息,请参阅[自述文件][15]。
|
||||||
|
|
||||||
|
Docker 让开始使用 OpenCPU 变得简单。容器给你一个充分灵活的 Linux,而无需在系统上安装任何东西。你可以通过 rstudio 服务器安装软件包或应用程序,也可以使用 `docker exec` 到正在运行的服务器中的 root shell 中:
|
||||||
|
|
||||||
|
```
|
||||||
|
# Lookup the container ID
|
||||||
|
docker ps
|
||||||
|
|
||||||
|
# Drop a shell
|
||||||
|
docker exec -i -t eec1cdae3228 /bin/bash
|
||||||
|
|
||||||
|
```
|
||||||
|
|
||||||
|
你可以在服务器的 shell 中安装其他软件,自定义 apache2 httpd 配置(auth,代理等),调整 R 选项,通过预加载数据或包等来优化性能。
|
||||||
|
|
||||||
|
### 2: 通过 DockerHub 发布和部署
|
||||||
|
|
||||||
|
最强大的是,Docker 可以通过 Dockerhub 发布和部署。要创建一个完全独立的应用程序容器,只需使用标准[ opencpu 镜像][16]并添加你的程序。
|
||||||
|
|
||||||
|
为了本文的目的,我通过在每个仓库中添加一个非常简单的 “Dockerfile” 将一些[示例程序][17]打包为 docker 容器。例如:[nabel][18] 的 [Dockerfile][19] 包含以下内容:
|
||||||
|
|
||||||
|
```
|
||||||
|
FROM opencpu/base
|
||||||
|
|
||||||
|
RUN R -e 'devtools::install_github("rwebapps/nabel")'
|
||||||
|
|
||||||
|
```
|
||||||
|
|
||||||
|
它采用标准的 [opencpu/base][20] 镜像,并从 Github [仓库][21]安装 nabel。结果是一个完全隔离独立的程序。任何人可以使用下面这样的命令启动程序:
|
||||||
|
|
||||||
|
```
|
||||||
|
docker run -d 8004:8004 rwebapps/nabel
|
||||||
|
|
||||||
|
```
|
||||||
|
|
||||||
|
`-d` 代表守护进程监听 8004 端口。很显然,你可以调整 `Dockerfile` 来安装任何其他的软件或设置你需要的程序。
|
||||||
|
|
||||||
|
容器化部署展示了 Docker 的真正能力:它可以发布可以开箱即用的独立软件,而无需安装任何软件或依赖付费托管服务。如果你更喜欢专业的托管,那会有许多公司乐意在可扩展的基础设施上为你托管 docker 程序。
|
||||||
|
|
||||||
|
### 3: 跨平台构建
|
||||||
|
|
||||||
|
Docker用于OpenCPU的第三种方式。每次发布,我们都构建 6 个操作系统的 `opencpu-server` 安装包,它们在 [https://archive.opencpu.org][22] 上公布。这个过程已经使用 DockerHub 完全自动化了。以下镜像从源代码自动构建所有栈:
|
||||||
|
|
||||||
|
* [opencpu/ubuntu-16.04][4]
|
||||||
|
|
||||||
|
* [opencpu/debian-9][5]
|
||||||
|
|
||||||
|
* [opencpu/fedora-25][6]
|
||||||
|
|
||||||
|
* [opencpu/fedora-26][7]
|
||||||
|
|
||||||
|
* [opencpu/centos-6][8]
|
||||||
|
|
||||||
|
* [opencpu/centos-7][9]
|
||||||
|
|
||||||
|
当 Github 上发布新版本时,DockerHub 会自动重建此镜像。要做的就是运行一个[脚本][23],它会取回镜像并将 `opencpu-server` 二进制复制到[归档服务器上][24]。
|
||||||
|
|
||||||
|
--------------------------------------------------------------------------------
|
||||||
|
|
||||||
|
via: https://www.r-bloggers.com/why-use-docker-with-r-a-devops-perspective/
|
||||||
|
|
||||||
|
作者:[Jeroen Ooms][a]
|
||||||
|
译者:[geekpi](https://github.com/geekpi)
|
||||||
|
校对:[校对者ID](https://github.com/校对者ID)
|
||||||
|
|
||||||
|
本文由 [LCTT](https://github.com/LCTT/TranslateProject) 原创编译,[Linux中国](https://linux.cn/) 荣誉推出
|
||||||
|
|
||||||
|
[a]:https://www.r-bloggers.com/author/jeroen-ooms/
|
||||||
|
[1]:https://www.opencpu.org/posts/opencpu-with-docker/
|
||||||
|
[2]:https://twitter.com/hashtag/rstats?src=hash&ref_src=twsrc%5Etfw
|
||||||
|
[3]:https://twitter.com/JennyBryan/status/913785731998289920?ref_src=twsrc%5Etfw
|
||||||
|
[4]:https://hub.docker.com/r/opencpu/ubuntu-16.04/
|
||||||
|
[5]:https://hub.docker.com/r/opencpu/debian-9/
|
||||||
|
[6]:https://hub.docker.com/r/opencpu/fedora-25/
|
||||||
|
[7]:https://hub.docker.com/r/opencpu/fedora-26/
|
||||||
|
[8]:https://hub.docker.com/r/opencpu/centos-6/
|
||||||
|
[9]:https://hub.docker.com/r/opencpu/centos-7/
|
||||||
|
[10]:https://www.r-bloggers.com/
|
||||||
|
[11]:https://www.opencpu.org/posts/opencpu-with-docker
|
||||||
|
[12]:https://www.opencpu.org/download.html
|
||||||
|
[13]:http://localhost:8004/ocpu/
|
||||||
|
[14]:http://localhost:8004/rstudio/
|
||||||
|
[15]:https://hub.docker.com/r/opencpu/rstudio/
|
||||||
|
[16]:https://hub.docker.com/u/opencpu/
|
||||||
|
[17]:https://www.opencpu.org/apps.html
|
||||||
|
[18]:https://rwebapps.ocpu.io/nabel/www/
|
||||||
|
[19]:https://github.com/rwebapps/nabel/blob/master/Dockerfile
|
||||||
|
[20]:https://hub.docker.com/r/opencpu/base/
|
||||||
|
[21]:https://github.com/rwebapps
|
||||||
|
[22]:https://archive.opencpu.org/
|
||||||
|
[23]:https://github.com/opencpu/archive/blob/gh-pages/update.sh
|
||||||
|
[24]:https://archive.opencpu.org/
|
||||||
|
[25]:https://www.r-bloggers.com/author/jeroen-ooms/
|
42
translated/tech/20171018 IoT Cybersecurity what is plan b.md
Normal file
42
translated/tech/20171018 IoT Cybersecurity what is plan b.md
Normal file
@ -0,0 +1,42 @@
|
|||||||
|
# IoT 网络安全:后备计划是什么?
|
||||||
|
|
||||||
|
八月份,四名美国参议员提出了一项旨在改善物联网 (IoT) 安全性的法案。2017 年的“ 物联网网络安全改进法” 是一项小幅的立法。它没有规范物联网市场。它没有任何特别关注的行业,或强制任何公司做任何事情。甚至不修改嵌入式软件的法律责任。无论安全多么糟糕,公司可以继续销售物联网设备。
|
||||||
|
|
||||||
|
法案的做法是利用政府的购买力推动市场:政府购买的任何物联网产品都必须符合最低安全标准。它要求供应商确保设备不仅可以打补丁,而且可以通过认证和及时的方式进行修补,没有不可更改的默认密码,并且没有已知的漏洞。这是一个你可以设置的低安全值,并且将大大提高安全性可以说明关于物联网安全性的当前状态。(全面披露:我帮助起草了一些法案的安全性要求。)
|
||||||
|
|
||||||
|
该法案还将修改“计算机欺诈和滥用”和“数字千年版权”法案,以便安全研究人员研究政府购买的物联网设备的安全性。这比我们的行业需求要窄得多。但这是一个很好的第一步,这可能是对这个立法最好的事。
|
||||||
|
|
||||||
|
不过,这一步甚至不可能采取。我在八月份写这个专栏,毫无疑问,这个法案你在十月份或以后读的时候会没有了。如果听证会举行,它们无关紧要。该法案不会被任何委员会投票,不会在任何立法日程上。这个法案成为法律的可能性是零。这不仅仅是因为目前的政治 - 我在奥巴马政府下同样悲观。
|
||||||
|
|
||||||
|
但情况很严重。互联网是危险的 - 物联网不仅给了眼睛和耳朵,而且还给手脚。一旦有影响到位和字节的安全漏洞、利用和攻击现在会影响血肉和血肉。
|
||||||
|
|
||||||
|
正如我们在过去一个世纪一再学到的那样,市场是改善产品和服务安全的可怕机制。汽车、食品、餐厅、飞机、火灾和金融仪器安全都是如此。原因很复杂,但基本上卖家不会在安全方面进行竞争,因为买方无法根据安全考虑有效区分产品。市场使用的竞相降低门槛的机制价格降到最低的同时也将质量降至最低。没有政府干预,物联网仍然会很不安全。
|
||||||
|
|
||||||
|
美国政府对干预没有兴趣,所以我们不会看到严肃的安全和保障法规、新的联邦机构或更好的责任法。我们可能在欧盟有更好的机会。根据“通用数据保护条例”在数据隐私的规定,欧盟可能会在 5 年内通过类似的安全法。没有其他国家有足够的市场份额来做改变。
|
||||||
|
|
||||||
|
有时我们可以选择不使用物联网,但是这个选择变得越来越少见了。去年,我试着不连接网络购买新车但是失败了。再过几年, 就几乎不可能不连接到物联网。我们最大的安全风险将不会来自我们与之有市场关系的设备,而是来自其他人的汽车、照相机、路由器、无人机等等。
|
||||||
|
|
||||||
|
我们可以尝试为理想买单,并要求更多的安全性,但企业不会在物联网安全方面进行竞争 - 而且我们的安全专家不是一个足够大的市场力量来产生影响。
|
||||||
|
|
||||||
|
我们需要一个后备计划,虽然我不知道是什么。如果你有任何想法请评论。
|
||||||
|
|
||||||
|
这篇文章以前出现在_ 9/10 月的 IEEE安全与隐私_上。
|
||||||
|
|
||||||
|
--------------------------------------------------------------------------------
|
||||||
|
|
||||||
|
作者简介:
|
||||||
|
|
||||||
|
自从 2004 年以来,我一直在博客上写关于安全的文章,以及从 1998 年以来我的每月订阅中也有。我写书、文章和学术论文。目前我是 IBM Resilient 的首席技术官,哈佛伯克曼中心的研究员,EFF 的董事会成员。
|
||||||
|
|
||||||
|
------------------
|
||||||
|
|
||||||
|
|
||||||
|
via: https://www.schneier.com/blog/archives/2017/10/iot_cybersecuri.html
|
||||||
|
|
||||||
|
作者:[Bruce Schneier][a]
|
||||||
|
译者:[geekpi](https://github.com/geekpi)
|
||||||
|
校对:[校对者ID](https://github.com/校对者ID)
|
||||||
|
|
||||||
|
本文由 [LCTT](https://github.com/LCTT/TranslateProject) 原创编译,[Linux中国](https://linux.cn/) 荣誉推出
|
||||||
|
|
||||||
|
[a]:https://www.schneier.com/blog/about/
|
Loading…
Reference in New Issue
Block a user