mirror of
https://github.com/LCTT/TranslateProject.git
synced 2025-01-13 22:30:37 +08:00
Merge branch 'master' of https://github.com/LCTT/TranslateProject
This commit is contained in:
commit
d8c01a7595
@ -282,7 +282,7 @@ arch-chroot /mnt /bin/bash
|
||||
现在来更改语言配置:
|
||||
|
||||
```
|
||||
nano /etc/local.gen
|
||||
nano /etc/locale.gen
|
||||
```
|
||||
|
||||
[
|
||||
@ -328,7 +328,7 @@ LANG=en_US.UTF-8
|
||||
输入下面的命令来同步时区:
|
||||
|
||||
```
|
||||
ls user/share/zoneinfo
|
||||
ls /usr/share/zoneinfo
|
||||
```
|
||||
|
||||
下面你将看到整个世界的时区列表。
|
||||
@ -352,7 +352,7 @@ ln –s /usr/share/zoneinfo/Asia/Shanghai /etc/localtime
|
||||
使用下面的命令来设置标准时间:
|
||||
|
||||
```
|
||||
hwclock --systohc –utc
|
||||
hwclock --systohc --utc
|
||||
```
|
||||
|
||||
硬件时钟已同步。
|
||||
|
@ -1,28 +1,24 @@
|
||||
在 Kubernetes 上运行一个 Python 应用程序
|
||||
============================================================
|
||||
|
||||
### 这个分步指导教程教你通过在 Kubernetes 上部署一个简单的 Python 应用程序来学习部署的流程。
|
||||
> 这个分步指导教程教你通过在 Kubernetes 上部署一个简单的 Python 应用程序来学习部署的流程。
|
||||
|
||||

|
||||
图片来源:opensource.com
|
||||
|
||||
Kubernetes 是一个具备部署、维护、和可伸缩特性的开源平台。它在提供可移植性、可扩展性、以及自我修复能力的同时,简化了容器化 Python 应用程序的管理。
|
||||
Kubernetes 是一个具备部署、维护和可伸缩特性的开源平台。它在提供可移植性、可扩展性以及自我修复能力的同时,简化了容器化 Python 应用程序的管理。
|
||||
|
||||
不论你的 Python 应用程序是简单还是复杂,Kubernetes 都可以帮你高效地部署和伸缩它们,在有限的资源范围内滚动升级新特性。
|
||||
|
||||
在本文中,我将描述在 Kubernetes 上部署一个简单的 Python 应用程序的过程,它包括:
|
||||
|
||||
* 创建 Python 容器镜像
|
||||
|
||||
* 发布容器镜像到镜像注册中心
|
||||
|
||||
* 使用持久卷
|
||||
|
||||
* 在 Kubernetes 上部署 Python 应用程序
|
||||
|
||||
### 必需条件
|
||||
|
||||
你需要 Docker、kubectl、以及这个 [源代码][10]。
|
||||
你需要 Docker、`kubectl` 以及这个 [源代码][10]。
|
||||
|
||||
Docker 是一个构建和承载已发布的应用程序的开源平台。可以参照 [官方文档][11] 去安装 Docker。运行如下的命令去验证你的系统上运行的 Docker:
|
||||
|
||||
@ -40,7 +36,7 @@ WARNING: No memory limit support
|
||||
WARNING: No swap limit support
|
||||
```
|
||||
|
||||
kubectl 是在 Kubernetes 集群上运行命令的一个命令行界面。运行下面的 shell 脚本去安装 kubectl:
|
||||
`kubectl` 是在 Kubernetes 集群上运行命令的一个命令行界面。运行下面的 shell 脚本去安装 `kubectl`:
|
||||
|
||||
```
|
||||
curl -LO https://storage.googleapis.com/kubernetes-release/release/$(curl -s https://storage.googleapis.com/kubernetes-release/release/stable.txt)/bin/linux/amd64/kubectl
|
||||
@ -56,9 +52,9 @@ curl -LO https://storage.googleapis.com/kubernetes-release/release/$(curl -s
|
||||
|
||||
### 创建一个 Python 容器镜像
|
||||
|
||||
为创建这些镜像,我们将使用 Docker,它可以让我们在一个隔离的 Linux 软件容器中部署应用程序。Docker 可以使用来自一个 `Docker file` 中的指令来自动化构建镜像。
|
||||
为创建这些镜像,我们将使用 Docker,它可以让我们在一个隔离的 Linux 软件容器中部署应用程序。Docker 可以使用来自一个 Dockerfile 中的指令来自动化构建镜像。
|
||||
|
||||
这是我们的 Python 应用程序的 `Docker file`:
|
||||
这是我们的 Python 应用程序的 Dockerfile:
|
||||
|
||||
```
|
||||
FROM python:3.6
|
||||
@ -90,7 +86,7 @@ VOLUME ["/app-data"]
|
||||
CMD ["python", "app.py"]
|
||||
```
|
||||
|
||||
这个 `Docker file` 包含运行我们的示例 Python 代码的指令。它使用的开发环境是 Python 3.5。
|
||||
这个 Dockerfile 包含运行我们的示例 Python 代码的指令。它使用的开发环境是 Python 3.5。
|
||||
|
||||
### 构建一个 Python Docker 镜像
|
||||
|
||||
@ -128,45 +124,45 @@ Kubernetes 支持许多的持久存储提供商,包括 AWS EBS、CephFS、Glus
|
||||
|
||||
为使用 CephFS 存储 Kubernetes 的容器数据,我们将创建两个文件:
|
||||
|
||||
persistent-volume.yml
|
||||
`persistent-volume.yml` :
|
||||
|
||||
```
|
||||
apiVersion: v1
|
||||
kind: PersistentVolume
|
||||
metadata:
|
||||
name: app-disk1
|
||||
namespace: k8s_python_sample_code
|
||||
name: app-disk1
|
||||
namespace: k8s_python_sample_code
|
||||
spec:
|
||||
capacity:
|
||||
storage: 50Gi
|
||||
accessModes:
|
||||
- ReadWriteMany
|
||||
cephfs:
|
||||
monitors:
|
||||
- "172.17.0.1:6789"
|
||||
user: admin
|
||||
secretRef:
|
||||
name: ceph-secret
|
||||
readOnly: false
|
||||
capacity:
|
||||
storage: 50Gi
|
||||
accessModes:
|
||||
- ReadWriteMany
|
||||
cephfs:
|
||||
monitors:
|
||||
- "172.17.0.1:6789"
|
||||
user: admin
|
||||
secretRef:
|
||||
name: ceph-secret
|
||||
readOnly: false
|
||||
```
|
||||
|
||||
persistent_volume_claim.yaml
|
||||
`persistent_volume_claim.yaml`:
|
||||
|
||||
```
|
||||
apiVersion: v1
|
||||
kind: PersistentVolumeClaim
|
||||
metadata:
|
||||
name: appclaim1
|
||||
namespace: k8s_python_sample_code
|
||||
name: appclaim1
|
||||
namespace: k8s_python_sample_code
|
||||
spec:
|
||||
accessModes:
|
||||
- ReadWriteMany
|
||||
resources:
|
||||
requests:
|
||||
storage: 10Gi
|
||||
accessModes:
|
||||
- ReadWriteMany
|
||||
resources:
|
||||
requests:
|
||||
storage: 10Gi
|
||||
```
|
||||
|
||||
现在,我们将使用 kubectl 去添加持久卷并声明到 Kubernetes 集群中:
|
||||
现在,我们将使用 `kubectl` 去添加持久卷并声明到 Kubernetes 集群中:
|
||||
|
||||
```
|
||||
$ kubectl create -f persistent-volume.yml
|
||||
@ -185,16 +181,16 @@ $ kubectl create -f persistent-volume-claim.yml
|
||||
apiVersion: v1
|
||||
kind: Service
|
||||
metadata:
|
||||
labels:
|
||||
k8s-app: k8s_python_sample_code
|
||||
name: k8s_python_sample_code
|
||||
namespace: k8s_python_sample_code
|
||||
labels:
|
||||
k8s-app: k8s_python_sample_code
|
||||
name: k8s_python_sample_code
|
||||
namespace: k8s_python_sample_code
|
||||
spec:
|
||||
type: NodePort
|
||||
ports:
|
||||
- port: 5035
|
||||
selector:
|
||||
k8s-app: k8s_python_sample_code
|
||||
type: NodePort
|
||||
ports:
|
||||
- port: 5035
|
||||
selector:
|
||||
k8s-app: k8s_python_sample_code
|
||||
```
|
||||
|
||||
使用下列的内容创建部署文件并将它命名为 `k8s_python_sample_code.deployment.yml`:
|
||||
@ -227,7 +223,7 @@ spec:
|
||||
claimName: appclaim1
|
||||
```
|
||||
|
||||
最后,我们使用 kubectl 将应用程序部署到 Kubernetes:
|
||||
最后,我们使用 `kubectl` 将应用程序部署到 Kubernetes:
|
||||
|
||||
```
|
||||
$ kubectl create -f k8s_python_sample_code.deployment.yml $ kubectl create -f k8s_python_sample_code.service.yml
|
||||
@ -248,7 +244,7 @@ kubectl get services
|
||||
|
||||
### 关于作者
|
||||
|
||||
[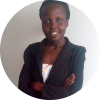][13] Joannah Nanjekye - Straight Outta 256 , 只要结果不问原因,充满激情的飞行员,喜欢用代码说话。[关于我的更多信息][8]
|
||||
[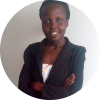][13] Joannah Nanjekye - Straight Outta 256,只要结果不问原因,充满激情的飞行员,喜欢用代码说话。[关于我的更多信息][8]
|
||||
|
||||
--------------------------------------------------------------------------------
|
||||
|
||||
@ -256,7 +252,7 @@ via: https://opensource.com/article/18/1/running-python-application-kubernetes
|
||||
|
||||
作者:[Joannah Nanjekye][a]
|
||||
译者:[qhwdw](https://github.com/qhwdw)
|
||||
校对:[校对者ID](https://github.com/校对者ID)
|
||||
校对:[wxy](https://github.com/wxy)
|
||||
|
||||
本文由 [LCTT](https://github.com/LCTT/TranslateProject) 原创编译,[Linux中国](https://linux.cn/) 荣誉推出
|
||||
|
@ -1,23 +1,25 @@
|
||||
供应链管理方面的 5 个开源软件工具
|
||||
======
|
||||
|
||||
> 跟踪您的库存和您需要的材料,用这些供应链管理工具制造产品。
|
||||
|
||||
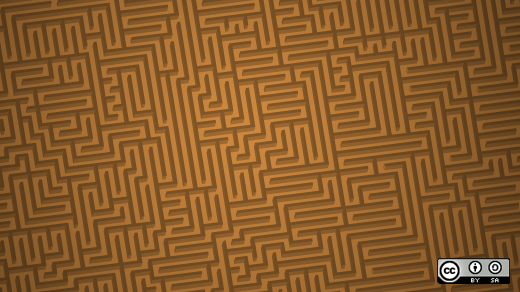
|
||||
|
||||
本文最初发表于 2016 年 1 月 14 日,最后的更新日期为 2018 年 3 月 2 日。
|
||||
|
||||
如果你正在管理着处理实体货物的业务,[供应链管理][1] 是你的业务流程中非常重要的一部分。不论你是经营着一个只有几个客户的小商店,还是在世界各地拥有数百万计客户和成千上万产品的世界财富 500 强的制造商或零售商,很清楚地知道你的库存和制造产品所需要的零部件,对你来说都是非常重要的事情。
|
||||
如果你正在管理着处理实体货物的业务,[供应链管理][1] 是你的业务流程中非常重要的一部分。不论你是经营着一个只有几个客户的小商店,还是在世界各地拥有数以百万计客户和成千上万产品的世界财富 500 强的制造商或零售商,很清楚地知道你的库存和制造产品所需要的零部件,对你来说都是非常重要的事情。
|
||||
|
||||
保持对货品、供应商、客户的持续跟踪,并且所有与它们相关的变动部分都会从中受益,并且,在某些情况下完全依赖专门的软件来帮助管理这些工作流。在本文中,我们将去了解一些免费的和开源的供应链管理方面的软件,以及它们的其中一些功能。
|
||||
保持对货品、供应商、客户的持续跟踪,而且所有与它们相关的变动部分都会受益于这些用来帮助管理工作流的专门软件,而在某些情况下需要完全依赖这些软件。在本文中,我们将去了解一些自由及开源的供应链管理方面的软件,以及它们的其中一些功能。
|
||||
|
||||
供应链管理比单纯的库存管理更为强大。它能帮你去跟踪货物流以降低成本,以及为可能发生的各种糟糕的变化来制定应对计划。它能够帮你对出口合规性进行跟踪,不论是合法性、最低品质要求、还是社会和环境的合规性。它能够帮你计划最低供应量,让你能够在订单数量和交付时间之间做出明智的决策。
|
||||
供应链管理比单纯的库存管理更为强大。它能帮你去跟踪货物流以降低成本,以及为可能发生的各种糟糕的变化来制定应对计划。它能够帮你对出口合规性进行跟踪,不论是否是出于法律要求、最低品质要求、还是社会和环境责任。它能够帮你计划最低供应量,让你能够在订单数量和交付时间之间做出明智的决策。
|
||||
|
||||
由于它的本质决定了许多供应链管理软件是与类似的软件捆绑在一起的,比如,[客户关系管理][2](CRM)和 [企业资源计划管理][3] (ERP)。因此,当你选择哪个工具更适合你的组织时,你可能会考虑与其它工具集成作为你的决策依据之一。
|
||||
由于其本质决定了许多供应链管理软件是与类似的软件捆绑在一起的,比如,[客户关系管理][2](CRM)和 [企业资源计划管理][3] (ERP)。因此,当你选择哪个工具更适合你的组织时,你可能会考虑与其它工具集成作为你的决策依据之一。
|
||||
|
||||
### Apache OFBiz
|
||||
|
||||
[Apache OFBiz][4] 是一套帮你管理多种业务流程的相关工具。虽然它能管理多种相关问题,比如,目录、电子商务网站、帐户、和销售点,它在供应链管理方面的主要功能关注于仓库管理、履行、订单、和生产管理。它的可定制性很强,但是,它需要大量的规划去设置和集成到你现有的流程中。这就是它适用于中大型业务的原因之一。项目的功能构建于三个层面:展示层、业务层、和数据层,它是一个弹性很好的解决方案,但是,再强调一遍,它也很复杂。
|
||||
[Apache OFBiz][4] 是一套帮你管理多种业务流程的相关工具。虽然它能管理多种相关问题,比如,分类、电子商务网站、会计和 POS,它在供应链管理方面的主要功能关注于仓库管理、履行、订单和生产管理。它的可定制性很强,但是,对应的它需要大量的规划去设置和集成到你现有的流程中。这就是它适用于中大型业务的原因之一。项目的功能构建于三个层面:展示层、业务层和数据层,它是一个弹性很好的解决方案,但是,再强调一遍,它也很复杂。
|
||||
|
||||
Apache OFBiz 的源代码在 [项目仓库][5] 中可以找到。Apache OFBiz 是用 Java 写的,并且它是按 [Apache 2.0 license][6] 授权的。
|
||||
Apache OFBiz 的源代码在其 [项目仓库][5] 中可以找到。Apache OFBiz 是用 Java 写的,并且它是按 [Apache 2.0 许可证][6] 授权的。
|
||||
|
||||
如果你对它感兴趣,你也可以去查看 [opentaps][7],它是在 OFBiz 之上构建的。Opentaps 强化了 OFBiz 的用户界面,并且添加了 ERP 和 CRM 的核心功能,包括仓库管理、采购和计划。它是按 [AGPL 3.0][8] 授权使用的,对于不接受开源授权的组织,它也提供了商业授权。
|
||||
|
||||
@ -25,25 +27,25 @@ Apache OFBiz 的源代码在 [项目仓库][5] 中可以找到。Apache OFBiz
|
||||
|
||||
[OpenBoxes][9] 是一个供应链管理和存货管理项目,最初的主要设计目标是为了医疗行业中的药品跟踪管理,但是,它可以通过修改去跟踪任何类型的货品和相关的业务流。它有一个需求预测工具,可以基于历史订单数量、存储跟踪、支持多种场所、过期日期跟踪、销售点支持等进行预测,并且它还有许多其它功能,这使它成为医疗行业的理想选择,但是,它也可以用于其它行业。
|
||||
|
||||
它在 [Eclipse Public License][10] 下可用,OpenBoxes 主要是由 Groovy 写的,它的源代码可以在 [GitHub][11] 上看到。
|
||||
它在 [Eclipse 公开许可证][10] 下可用,OpenBoxes 主要是由 Groovy 写的,它的源代码可以在 [GitHub][11] 上看到。
|
||||
|
||||
### OpenLMIS
|
||||
|
||||
与 OpenBoxes 类似,[OpenLMIS][12] 也是一个医疗行业的供应链管理工具,但是,它专用设计用于在非洲的资源缺乏地区使用,以确保有限的药物和医疗用品能够用到需要的病人上。它是 API 驱动的,这样用户可以去定制和扩展 OpenLMIS,同时还能维护一个与通用基准代码的连接。它是由络克菲勒基金会开发的,其它的贡献者包括联合国、美国国际开发署、和比尔 & 梅林达 盖茨基金会。
|
||||
与 OpenBoxes 类似,[OpenLMIS][12] 也是一个医疗行业的供应链管理工具,但是,它专用设计用于在非洲的资源缺乏地区使用,以确保有限的药物和医疗用品能够用到需要的病人上。它是 API 驱动的,这样用户可以去定制和扩展 OpenLMIS,同时还能维护一个与通用基准代码的连接。它是由洛克菲勒基金会开发的,其它的贡献者包括联合国、美国国际开发署、和比尔 & 梅林达·盖茨基金会。
|
||||
|
||||
OpenLMIS 是用 Java 和 JavaScript 的 AngularJS 写的。它在 [AGPL 3.0 license][13] 下使用,它的源代码在 [GitHub][13] 上可以找到。
|
||||
OpenLMIS 是用 Java 和 JavaScript 的 AngularJS 写的。它在 [AGPL 3.0 许可证][13] 下使用,它的源代码在 [GitHub][13] 上可以找到。
|
||||
|
||||
### Odoo
|
||||
|
||||
你可能在我们以前的 [ERP 项目][3] 榜的文章上见到过 [Odoo][14]。事实上,根据你的需要,一个全功能的 ERP 对你来说是最适合的。Odoo 的供应链管理工具主要围绕存货和采购管理,同时还与电子商务网站和销售点连接,但是,它也可以与其它的工具连接,比如,与 [frePPLe][15] 连接,它是一个开源的生产计划工具。
|
||||
你可能在我们以前的 [ERP 项目][3] 榜的文章上见到过 [Odoo][14]。事实上,根据你的需要,一个全功能的 ERP 对你来说是最适合的。Odoo 的供应链管理工具主要围绕存货和采购管理,同时还与电子商务网站和 POS 连接,但是,它也可以与其它的工具连接,比如,与 [frePPLe][15] 连接,它是一个开源的生产计划工具。
|
||||
|
||||
Odoo 既有软件即服务的解决方案,也有开源的社区版本。开源的版本是以 [LGPL][16] 版本 3 下发行的,源代码在 [GitHub][17] 上可以找到。Odoo 主要是用 Python 来写的。
|
||||
Odoo 既有软件即服务(SaaS)的解决方案,也有开源的社区版本。开源的版本是以 [LGPL][16] 版本 3 下发行的,源代码在 [GitHub][17] 上可以找到。Odoo 主要是用 Python 来写的。
|
||||
|
||||
### xTuple
|
||||
|
||||
[xTuple][18] 标称自己是“为成长中的企业提供供应链管理软件”,它专注于已经超越了传统的小型企业 ERP 和 CRM 解决方案的企业。它的开源版本称为 Postbooks,添加了一些存货、分销、采购、以及供应商报告的功能,它提供的核心功能是帐务、CRM、以及 ERP 功能,而它的商业版本扩展了制造和分销的 [功能][19]。
|
||||
[xTuple][18] 标称自己是“为成长中的企业提供供应链管理软件”,它专注于已经超越了其传统的小型企业 ERP 和 CRM 解决方案的企业。它的开源版本称为 Postbooks,添加了一些存货、分销、采购、以及供应商报告的功能,它提供的核心功能是会计、CRM、以及 ERP 功能,而它的商业版本扩展了制造和分销的 [功能][19]。
|
||||
|
||||
xTuple 在 [CPAL][20] 下使用,这个项目欢迎开发者去 fork 它,为基于存货的制造商去创建其它的业务软件。它的 Web 应用核心是用 JavaScript 写的,它的源代码在 [GitHub][21] 上可以找到。
|
||||
xTuple 在 [CPAL][20] 下使用,这个项目欢迎开发者去复刻它,为基于存货的制造商去创建其它的业务软件。它的 Web 应用核心是用 JavaScript 写的,它的源代码在 [GitHub][21] 上可以找到。
|
||||
|
||||
就这些,当然了,还有其它的可以帮你处理供应链管理的开源软件。如果你知道还有更好的软件,请在下面的评论区告诉我们。
|
||||
|
||||
@ -53,14 +55,14 @@ via: https://opensource.com/tools/supply-chain-management
|
||||
|
||||
作者:[Jason Baker][a]
|
||||
译者:[qhwdw](https://github.com/qhwdw)
|
||||
校对:[校对者ID](https://github.com/校对者ID)
|
||||
校对:[wxy](https://github.com/wxy)
|
||||
|
||||
本文由 [LCTT](https://github.com/LCTT/TranslateProject) 原创编译,[Linux中国](https://linux.cn/) 荣誉推出
|
||||
|
||||
[a]:https://opensource.com/users/jason-baker
|
||||
[1]:https://en.wikipedia.org/wiki/Supply_chain_management
|
||||
[2]:https://opensource.com/business/14/7/top-5-open-source-crm-tools
|
||||
[3]:https://opensource.com/resources/top-4-open-source-erp-systems
|
||||
[3]:https://linux.cn/article-9785-1.html
|
||||
[4]:http://ofbiz.apache.org/
|
||||
[5]:http://ofbiz.apache.org/source-repositories.html
|
||||
[6]:http://www.apache.org/licenses/LICENSE-2.0
|
43
published/20180702 My first sysadmin mistake.md
Normal file
43
published/20180702 My first sysadmin mistake.md
Normal file
@ -0,0 +1,43 @@
|
||||
我的第一个系统管理员错误
|
||||
======
|
||||
|
||||
> 如何在崩溃的局面中集中精力寻找解决方案。
|
||||
|
||||
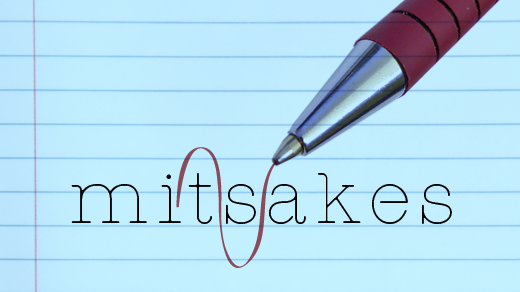
|
||||
|
||||
如果你在 IT 领域工作,你知道事情永远不会像你想象的那样完好。在某些时候,你会遇到错误或出现问题,你最终必须解决问题。这就是系统管理员的工作。
|
||||
|
||||
作为人类,我们都会犯错误。我们不是已经犯错,就是即将犯错。结果,我们最终还必须解决自己的错误。总是这样。我们都会失误、敲错字母或犯错。
|
||||
|
||||
作为一名年轻的系统管理员,我艰难地学到了这一课。我犯了一个大错。但是多亏了上级的指导,我学会了不去纠缠于我的错误,而是制定一个“错误策略”来做正确的事情。从错误中吸取教训。克服它,继续前进。
|
||||
|
||||
我的第一份工作是一家小公司的 Unix 系统管理员。真的,我是一名生嫩的系统管理员,但我大部分时间都独自工作。我们是一个小型 IT 团队,只有我们三个人。我是 20 或 30 台 Unix 工作站和服务器的唯一系统管理员。另外两个支持 Windows 服务器和桌面。
|
||||
|
||||
任何阅读这篇文章的系统管理员都不会对此感到意外,作为一个不成熟的初级系统管理员,我最终在错误的目录中运行了 `rm` 命令——作为 root 用户。我以为我正在为我们的某个程序删除一些陈旧的缓存文件。相反,我错误地清除了 `/etc` 目录中的所有文件。糟糕。
|
||||
|
||||
我意识到犯了错误是看到了一条错误消息,“`rm` 无法删除某些子目录”。但缓存目录应该只包含文件!我立即停止了 `rm` 命令,看看我做了什么。然后我惊慌失措。一下子,无数个想法涌入了我的脑中。我刚刚销毁了一台重要的服务器吗?系统会怎么样?我会被解雇吗?
|
||||
|
||||
幸运的是,我运行的是 `rm *` 而不是 `rm -rf *`,因此我只删除了文件。子目录仍在那里。但这并没有让我感觉更好。
|
||||
|
||||
我立刻去找我的主管告诉她我做了什么。她看到我对自己的错误感到愚蠢,但这是我犯的。尽管紧迫,她花了几分钟时间跟我做了一些指导。她说:“你不是第一个这样做的人,在你这种情况下,别人会怎么做?”这帮助我平静下来并专注。我开始更少考虑我刚刚做的愚蠢事情,而更多地考虑我接下来要做的事情。
|
||||
|
||||
我做了一个简单的策略:不要重启服务器。使用相同的系统作为模板,并重建 `/etc` 目录。
|
||||
|
||||
制定了行动计划后,剩下的就很容易了。只需运行正确的命令即可从另一台服务器复制 `/etc` 文件并编辑配置,使其与系统匹配。多亏了我对所有东西都做记录的习惯,我使用已有的文档进行最后的调整。我避免了完全恢复服务器,这意味着一个巨大的宕机事件。
|
||||
|
||||
可以肯定的是,我从这个错误中吸取了教训。在接下来作为系统管理员的日子中,我总是在运行任何命令之前确认我所在的目录。
|
||||
|
||||
我还学习了构建“错误策略”的价值。当事情出错时,恐慌并思考接下来可能发生的所有坏事是很自然的。这是人性。但是制定一个“错误策略”可以帮助我不再担心出了什么问题,而是专注于让事情变得更好。我仍然会想一下,但是知道我接下来的步骤可以让我“克服它”。
|
||||
|
||||
--------------------------------------------------------------------------------
|
||||
|
||||
via: https://opensource.com/article/18/7/my-first-sysadmin-mistake
|
||||
|
||||
作者:[Jim Hall][a]
|
||||
选题:[lujun9972](https://github.com/lujun9972)
|
||||
译者:[geekpi](https://github.com/geekpi)
|
||||
校对:[wxy](https://github.com/wxy)
|
||||
|
||||
本文由 [LCTT](https://github.com/LCTT/TranslateProject) 原创编译,[Linux中国](https://linux.cn/) 荣誉推出
|
||||
|
||||
[a]:https://opensource.com/users/jim-hall
|
@ -0,0 +1,147 @@
|
||||
如何在绝大部分类型的机器上安装 NVIDIA 显卡驱动
|
||||
======
|
||||
|
||||
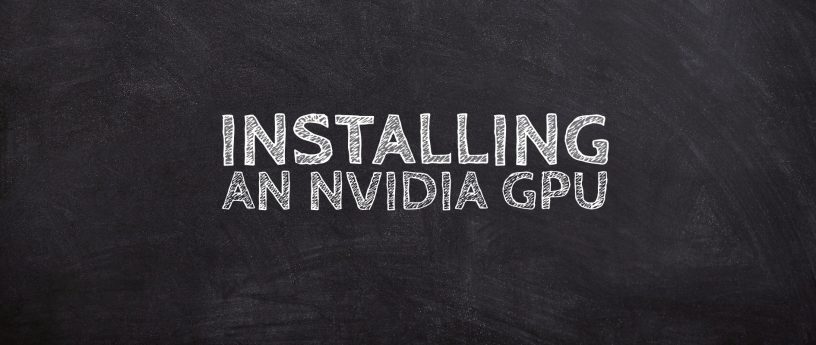
|
||||
|
||||
无论是研究还是娱乐,安装一个最新的显卡驱动都能提升你的计算机性能,并且使你能全方位地实现新功能。本安装指南使用 Fedora 28 的新的第三方仓库来安装 NVIDIA 驱动。它将引导您完成硬件和软件两方面的安装,并且涵盖需要让你的 NVIDIA 显卡启动和运行起来的一切知识。这个流程适用于任何支持 UEFI 的计算机和任意新的 NVIDIA 显卡。
|
||||
|
||||
### 准备
|
||||
|
||||
本指南依赖于下面这些材料:
|
||||
|
||||
* 一台使用 [UEFI][1] 的计算机,如果你不确定你的电脑是否有这种固件,请运行 `sudo dmidecode -t 0`。如果输出中出现了 “UEFI is supported”,你的安装过程就可以继续了。不然的话,虽然可以在技术上更新某些电脑来支持 UEFI,但是这个过程的要求很苛刻,我们通常不建议你这么使用。
|
||||
* 一个现代的、支持 UEFI 的 NVIDIA 的显卡
|
||||
* 一个满足你的 NVIDIA 显卡的功率和接线要求的电源(有关详细信息,请参考“硬件和修改”的章节)
|
||||
* 网络连接
|
||||
* Fedora 28 系统
|
||||
|
||||
### 安装实例
|
||||
|
||||
这个安装示例使用的是:
|
||||
|
||||
* 一台 Optiplex 9010 的主机(一台相当老的机器)
|
||||
* [NVIDIA GeForce GTX 1050 Ti XLR8 游戏超频版 4 GB GDDR5 PCI Express 3.0 显卡][2]
|
||||
* 为了满足新显卡的电源要求,电源升级为 [EVGA – 80 PLUS 600 W ATX 12V/EPS 12V][3],这个最新的电源(PSU)比推荐的最低要求高了 300 W,但在大部分情况下,满足推荐的最低要求就足够了。
|
||||
* 然后,当然的,Fedora 28 也别忘了.
|
||||
|
||||
### 硬件和修改
|
||||
|
||||
#### 电源(PSU)
|
||||
|
||||
打开你的台式机的机箱,检查印刷在电源上的最大输出功率。然后,查看你的 NVIDIA 显卡的文档,确定推荐的最小电源功率要求(以瓦特为单位)。除此之外,检查你的显卡,看它是否需要额外的接线,例如 6 针连接器,大多数的入门级显卡只从主板获取电力,但是有一些显卡需要额外的电力,如果出现以下情况,你需要升级你的电源:
|
||||
|
||||
1. 你的电源的最大输出功率低于显卡建议的最小电源功率。注意:根据一些显卡厂家的说法,比起推荐的功率,预先构建的系统可能会需要更多或更少的功率,而这取决于系统的配置。如果你使用的是一个特别耗电或者特别节能的配置,请灵活决定你的电源需求。
|
||||
2. 你的电源没有提供必须的接线口来为你的显卡供电。
|
||||
|
||||
电源的更换很容易,但是在你拆除你当前正在使用的电源之前,请务必注意你的接线布局。除此之外,请确保你选择的电源适合你的机箱。
|
||||
|
||||
#### CPU
|
||||
|
||||
虽然在大多数老机器上安装高性能的 NVIDIA 显卡是可能的,但是一个缓慢或受损的 CPU 会阻碍显卡性能的发挥,如果要计算在你的机器上瓶颈效果的影响,请点击[这里][4]。了解你的 CPU 性能来避免高性能的显卡和 CPU 无法保持匹配是很重要的。升级你的 CPU 是一个潜在的考虑因素。
|
||||
|
||||
#### 主板
|
||||
|
||||
在继续进行之前,请确认你的主板和你选择的显卡是兼容的。你的显卡应该插在最靠近散热器的 PCI-E x16 插槽中。确保你的设置为显卡预留了足够的空间。此外,请注意,现在大部分的显卡使用的都是 PCI-E 3.0 技术。虽然这些显卡如果插在 PCI-E 3.0 插槽上会运行地最好,但如果插在一个旧版的插槽上的话,性能也不会受到太大的影响。
|
||||
|
||||
### 安装
|
||||
|
||||
1、 首先,打开终端更新你的包管理器(如果没有更新的话):
|
||||
|
||||
```
|
||||
sudo dnf update
|
||||
```
|
||||
|
||||
2、 然后,使用这条简单的命令进行重启:
|
||||
|
||||
```
|
||||
reboot
|
||||
```
|
||||
|
||||
3、 在重启之后,安装 Fedora 28 的工作站的仓库:
|
||||
|
||||
```
|
||||
sudo dnf install fedora-workstation-repositories
|
||||
```
|
||||
|
||||
4、 接着,设置 NVIDIA 驱动的仓库:
|
||||
|
||||
```
|
||||
sudo dnf config-manager --set-enabled rpmfusion-nonfree-nvidia-driver
|
||||
```
|
||||
|
||||
5、 然后,再次重启。
|
||||
|
||||
6、 在这次重启之后,通过下面这条命令验证是否添加了仓库:
|
||||
|
||||
```
|
||||
sudo dnf repository-packages rpmfusion-nonfree-nvidia-driver info
|
||||
```
|
||||
|
||||
如果加载了多个 NVIDIA 工具和它们各自的 spec 文件,请继续进行下一步。如果没有,你可能在添加新仓库的时候遇到了一个错误。你应该再试一次。
|
||||
|
||||
7、 登录,连接到互联网,然后打开“软件”应用程序。点击“加载项>硬件驱动> NVIDIA Linux 图形驱动>安装”。
|
||||
|
||||
如果你使用更老的显卡或者想使用多个显卡,请进一步查看 [RPMFusion 指南][8]。最后,要确保启动成功,设置 `/etc/gdm/custom.conf` 中的 `WaylandEnable=false`,确认避免使用安全启动。
|
||||
接着,再一次重启。
|
||||
|
||||
8、这个过程完成后,关闭所有的应用并**关机**。拔下电源插头,然后按下电源按钮以释放余电,避免你被电击。如果你对电源有开关,关闭它。
|
||||
|
||||
9、 最后,安装显卡,拔掉老的显卡并将新的显卡插入到正确的 PCI-E x16 插槽中。成功安装新的显卡之后,关闭你的机箱,插入电源 ,然后打开计算机,它应该会成功启动。
|
||||
|
||||
**注意:** 要禁用此安装中使用的 NVIDIA 驱动仓库,或者要禁用所有的 Fedora 工作站仓库,请参考这个 [Fedora Wiki 页面][6]。
|
||||
|
||||
### 验证
|
||||
|
||||
1、 如果你新安装的 NVIDIA 显卡已连接到你的显示器并显示正确,则表明你的 NVIDIA 驱动程序已成功和显卡建立连接。
|
||||
|
||||
如果你想去查看你的设置,或者验证驱动是否在正常工作(这里,主板上安装了两块显卡),再次打开 “NVIDIA X 服务器设置应用程序”。这次,你应该不会得到错误信息提示,并且系统会给出有关 X 的设置文件和你的 NVIDIA 显卡的信息。(请参考下面的屏幕截图)
|
||||
|
||||
![NVIDIA X Server Settings][7]
|
||||
|
||||
通过这个应用程序,你可以根据你的需要需改 X 配置文件,并可以监控显卡的性能,时钟速度和温度信息。
|
||||
|
||||
2、 为确保新显卡以满功率运行,显卡性能测试是非常必要的。GL Mark 2,是一个提供后台处理、构建、照明、纹理等等有关信息的标准工具。它提供了一个优秀的解决方案。GL Mark 2 记录了各种各样的图形测试的帧速率,然后输出一个总体的性能评分(这被称为 glmark2 分数)。
|
||||
|
||||
**注意:** glxgears 只会测试你的屏幕或显示器的性能,不会测试显卡本身,请使用 GL Mark 2。
|
||||
|
||||
要运行 GLMark2:
|
||||
|
||||
1. 打开终端并关闭其他所有的应用程序
|
||||
2. 运行 `sudo dnf install glmark2` 命令
|
||||
3. 运行 `glmark2` 命令
|
||||
4. 允许运行完整的测试来得到最好的结果。检查帧速率是否符合你对这块显卡的预期。如果你想要额外的验证,你可以查阅网站来确认是否已有你这块显卡的 glmark2 测试评分被公布到网上,你可以比较这个分数来评估你这块显卡的性能。
|
||||
5. 如果你的帧速率或者 glmark2 评分低于预期,请思考潜在的因素。CPU 造成的瓶颈?其他问题导致?
|
||||
|
||||
|
||||
如果诊断的结果很好,就开始享受你的新显卡吧。
|
||||
|
||||
### 参考链接
|
||||
|
||||
- [How to benchmark your GPU on Linux][9]
|
||||
- [How to install a graphics card][10]
|
||||
- [The Fedora Wiki Page][6]
|
||||
- [The Bottlenecker][4]
|
||||
- [What Is Unified Extensible Firmware Interface (UEFI)][1]
|
||||
|
||||
--------------------------------------------------------------------------------
|
||||
|
||||
via: https://fedoramagazine.org/install-nvidia-gpu/
|
||||
|
||||
作者:[Justice del Castillo][a]
|
||||
选题:[lujun9972](https://github.com/lujun9972)
|
||||
译者:[hopefully2333](https://github.com/hopefully2333)
|
||||
校对:[wxy](https://github.com/wxy)
|
||||
|
||||
本文由 [LCTT](https://github.com/LCTT/TranslateProject) 原创编译,[Linux中国](https://linux.cn/) 荣誉推出
|
||||
|
||||
[a]:https://fedoramagazine.org/author/justice/
|
||||
[1]:https://whatis.techtarget.com/definition/Unified-Extensible-Firmware-Interface-UEFI
|
||||
[2]:https://www.cnet.com/products/pny-geforce-gtx-xlr8-gaming-1050-ti-overclocked-edition-graphics-card-gf-gtx-1050-ti-4-gb/specs/
|
||||
[3]:https://www.evga.com/products/product.aspx?pn=100-B1-0600-KR
|
||||
[4]:http://thebottlenecker.com (Home: The Bottle Necker)
|
||||
[5]:https://bytebucket.org/kenneym/fedora-28-nvidia-gpu-installation/raw/7bee7dc6effe191f1f54b0589fa818960a8fa18b/nvidia_xserver_error.jpg?token=c6a7effe35f1c592a155a4a46a068a19fd060a91 (NVIDIA X Sever Prompt)
|
||||
[6]:https://fedoraproject.org/wiki/Workstation/Third_Party_Software_Repositories
|
||||
[7]:https://bytebucket.org/kenneym/fedora-28-nvidia-gpu-installation/raw/7bee7dc6effe191f1f54b0589fa818960a8fa18b/NVIDIA_XCONFIG.png?token=64e1a7be21e5e9ba157f029b65e24e4eef54d88f (NVIDIA X Server Settings)
|
||||
[8]:https://rpmfusion.org/Howto/NVIDIA?highlight=%28CategoryHowto%29
|
||||
[9]: https://www.howtoforge.com/tutorial/linux-gpu-benchmark/
|
||||
[10]: https://www.pcworld.com/article/2913370/components-graphics/how-to-install-a-graphics-card.html
|
@ -1,41 +1,45 @@
|
||||
6 个用于了解互联网工作原理的 RFC
|
||||
6 个可以帮你理解互联网工作原理的 RFC
|
||||
======
|
||||
|
||||
> 以及 3 个有趣的 RFC。
|
||||
|
||||
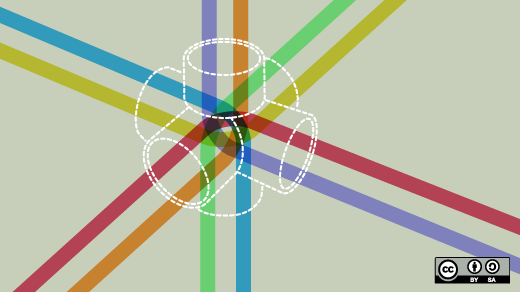
|
||||
|
||||
阅读源码是开源软件的重要组成部分。这意味着用户可以查看代码并了解做了什么。
|
||||
|
||||
但“阅读源码”并不仅适用于代码。理解代码实现的标准同样重要。这些标准由[互联网工程任务组][1](IETF)发布的称为“注释请求”(RFC)的文档中编写的。多年来已经发布了数以千计的 RFC,因此我们收集了一些我们的贡献者认为必读的内容。
|
||||
但“阅读源码”并不仅适用于代码。理解代码实现的标准同样重要。这些标准编写在由<ruby>[互联网工程任务组][1]<rt>Internet Engineering Task Force</rt></ruby>(IETF)发布的称为“<ruby>意见征集<rt>Requests for Comment</rt></ruby>”(RFC)的文档中。多年来已经发布了数以千计的 RFC,因此我们收集了一些我们的贡献者认为必读的内容。
|
||||
|
||||
### 6 个必读的 RFC
|
||||
|
||||
#### RFC 2119 - 在 RFC 中用于指示需求级别的关键字
|
||||
|
||||
这是一个快速阅读,但它对了解其他 RFC 非常重要。 [RFC 2119][2] 定义了后续 RFC 中使用的需求级别。 “MAY” 究竟意味着什么?如果标准说 “SHOULD”,你真的必须这样做吗?通过为需求提供明确定义的分类,RFC 2119 有助于避免歧义。
|
||||
这是一个快速阅读,但它对了解其它 RFC 非常重要。 [RFC 2119][2] 定义了后续 RFC 中使用的需求级别。 “MAY” 究竟意味着什么?如果标准说 “SHOULD”,你*真的*必须这样做吗?通过为需求提供明确定义的分类,RFC 2119 有助于避免歧义。
|
||||
|
||||
#### RFC 3339 - 互联网上的日期和时间:时间戳
|
||||
|
||||
时间是全世界程序员的祸根。 [RFC 3339][3] 定义了如何格式化时间戳。基于 [ISO 8601][4] 标准,3339 为我们提供了一种表达时间的常用方法。例如,像星期几这样的冗余信息不应该包含在存储的时间戳中,因为它很容易计算。
|
||||
|
||||
#### RFC 1918—私有互联网的地址分配
|
||||
#### RFC 1918 - 私有互联网的地址分配
|
||||
|
||||
有属于每个人的互联网,也有只属于你的互联网。专用网络一直在使用,[RFC 1918][5] 定义了这些网络。当然,你可以在路由器上设置路由公网地址,但这是一个坏主意。或者,你可以将未使用的公共 IP 地址视为内部网络。在任何一种情况下都表明你从未阅读过 RFC 1918。
|
||||
有属于每个人的互联网,也有只属于你的互联网。私有网络一直在使用,[RFC 1918][5] 定义了这些网络。当然,你可以在路由器上设置在内部使用公网地址,但这是一个坏主意。或者,你可以将未使用的公共 IP 地址视为内部网络。在任何一种情况下都表明你从未阅读过 RFC 1918。
|
||||
|
||||
#### RFC 1912—常见的 DNS 操作和配置错误
|
||||
#### RFC 1912 - 常见的 DNS 操作和配置错误
|
||||
|
||||
一切都是 #@%@ DNS 问题,对吧? [RFC 1912][6] 列出了管理员在试图保持互联网运行时所犯的错误。虽然它是在 1996 年发布的,但 DNS(以及人们犯的错误)并没有真正改变这么多。为了理解我们为什么首先需要 DNS,请考虑[ RFC 289-What we hope is an official list of host names]][7]如今看起来像什么。
|
||||
一切都是 #@%@ 的 DNS 问题,对吧? [RFC 1912][6] 列出了管理员在试图保持互联网运行时所犯的错误。虽然它是在 1996 年发布的,但 DNS(以及人们犯的错误)并没有真正改变这么多。为了理解我们为什么首先需要 DNS,如今我们再来看看 [RFC 289 - 我们希望正式的主机列表是什么样子的][7] 就知道了。
|
||||
|
||||
#### RFC 2822 — 互联网邮件格式
|
||||
|
||||
想想你知道什么是有效的电子邮件地址么?如果不接受我地址中 “+” 的站点的数量是任何迹象, 你就不会。 [RFC 2822][8] 定义了有效的电子邮件地址。它还详细介绍了电子邮件的其余部分。
|
||||
想想你知道什么是有效的电子邮件地址么?如果你知道有多少个站点不接受我邮件地址中 “+” 的话,你就知道你知道不知道了。 [RFC 2822][8] 定义了有效的电子邮件地址。它还详细介绍了电子邮件的其余部分。
|
||||
|
||||
#### RFC 7231—超文本传输协议(HTTP/1.1):语义和内容
|
||||
#### RFC 7231 - 超文本传输协议(HTTP/1.1):语义和内容
|
||||
|
||||
当你停下来思考它时,我们在网上做的几乎所有东西都依赖于 HTTP。 [RFC 7231][9] 是该协议的最新更新。它有超过 100 页,定义了方法、头和状态代码。
|
||||
想想看,几乎我们在网上做的一切都依赖于 HTTP。 [RFC 7231][9] 是该协议的最新更新。它有超过 100 页,定义了方法、请求头和状态代码。
|
||||
|
||||
### 3 个应该阅读的 RFC
|
||||
|
||||
好吧,并非每个RFC都是严肃的业务。
|
||||
好吧,并非每个 RFC 都是严肃的。
|
||||
|
||||
#### RFC 1149—在禽类载体上传输 IP 数据报的标准
|
||||
#### RFC 1149 - 在禽类载体上传输 IP 数据报的标准
|
||||
|
||||
网络以多种不同方式传递数据包。 [RFC 1149][10] 描述了鸽子载体的使用。当我距离州际高速公路一英里以外时,它们的可靠性不会低于我的移动提供商。
|
||||
|
||||
@ -56,7 +60,7 @@ via: https://opensource.com/article/18/7/requests-for-comments-to-know
|
||||
作者:[Ben Cotton][a]
|
||||
选题:[lujun9972](https://github.com/lujun9972)
|
||||
译者:[geekpi](https://github.com/geekpi)
|
||||
校对:[校对者ID](https://github.com/校对者ID)
|
||||
校对:[wxy](https://github.com/wxy)
|
||||
|
||||
本文由 [LCTT](https://github.com/LCTT/TranslateProject) 原创编译,[Linux中国](https://linux.cn/) 荣誉推出
|
||||
|
File diff suppressed because it is too large
Load Diff
@ -0,0 +1,614 @@
|
||||
MjSeven is translating
|
||||
|
||||
Understanding Python Dataclasses — Part 1
|
||||
============================================================
|
||||
|
||||
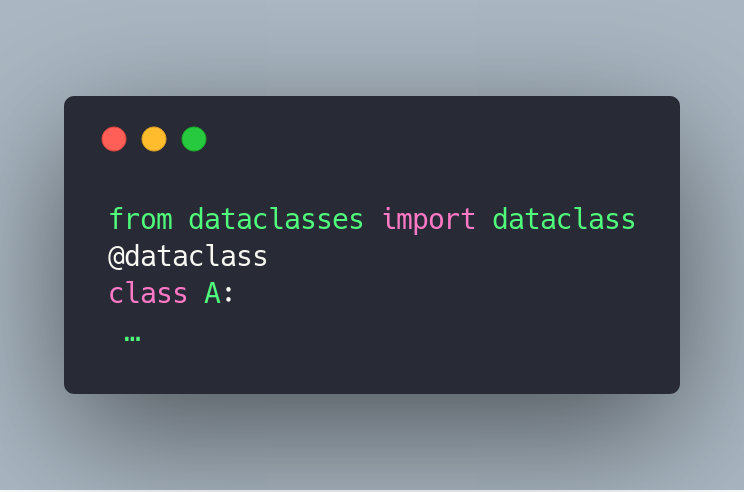
|
||||
|
||||
If you’re reading this, then you are already aware of Python 3.7 and the new features that come packed with it. Personally, I am most excited about `Dataclasses`. I have been waiting for them to arrive for a while.
|
||||
|
||||
This is a two part post:
|
||||
1\. Dataclass features overview in this post
|
||||
2\. Dataclass `fields` overview in the [next post][1]
|
||||
|
||||
### Introduction
|
||||
|
||||
`Dataclasses` are python classes but are suited for storing data objects. What are data objects, you ask? Here is a non-exhaustive list of features that define data objects:
|
||||
|
||||
* They store data and represent a certain data type. Ex: A number. For people familiar with ORMs, a model instance is a data object. It represents a specific kind of entity. It holds attributes that define or represent the entity.
|
||||
|
||||
* They can be compared to other objects of the same type. Ex: A number can be `greater than`, `less than`, or `equal to` another number
|
||||
|
||||
There are certainly more features, but this list is sufficient to help you understand the crux.
|
||||
|
||||
To understand `Dataclasses`, we shall be implementing a simple class that holds a number, and allows us to perform the above mentioned operations.
|
||||
First, we shall be using normal classes, and then we shall use `Dataclasses` to achieve the same result.
|
||||
|
||||
But before we begin, a word on the usage of `Dataclasses`
|
||||
|
||||
Python 3.7 provides a decorator [dataclass][2] that is used to convert a class into a dataclass.
|
||||
|
||||
All you have to do is wrap the class in the decorator:
|
||||
|
||||
```
|
||||
from dataclasses import dataclass
|
||||
```
|
||||
|
||||
```
|
||||
@dataclass
|
||||
class A:
|
||||
…
|
||||
```
|
||||
|
||||
Now, lets dive into the usage of how and what `dataclass` changes for us.
|
||||
|
||||
### Initialization
|
||||
|
||||
Usual
|
||||
|
||||
```
|
||||
class Number:
|
||||
```
|
||||
|
||||
```
|
||||
__init__(self, val):
|
||||
self.val = val
|
||||
|
||||
>>> one = Number(1)
|
||||
>>> one.val
|
||||
>>> 1
|
||||
```
|
||||
|
||||
With `dataclass`
|
||||
|
||||
```
|
||||
@dataclass
|
||||
class Number:
|
||||
val:int
|
||||
|
||||
>>> one = Number(1)
|
||||
>>> one.val
|
||||
>>> 1
|
||||
```
|
||||
|
||||
Here’s what’s changed with the dataclass decorator:
|
||||
|
||||
1\. No need of defining `__init__`and then assigning values to `self`, `d` takes care of it
|
||||
2\. We defined the member attributes in advance in a much more readable fashion, along with [type hinting][3]. We now know instantly that `val` is of type `int`. This is definitely more readable than the usual way of defining class members.
|
||||
|
||||
> Zen of Python: Readability counts
|
||||
|
||||
It is also possible to define default values:
|
||||
|
||||
```
|
||||
@dataclass
|
||||
class Number:
|
||||
val:int = 0
|
||||
```
|
||||
|
||||
### Representation
|
||||
|
||||
Object representation is a meaningful string representation of the object that is very useful in debugging.
|
||||
|
||||
Default python objects representation is not very meaningful:
|
||||
|
||||
```
|
||||
class Number:
|
||||
def __init__(self, val = 0):
|
||||
self.val = val
|
||||
|
||||
>>> a = Number(1)
|
||||
>>> a
|
||||
>>> <__main__.Number object at 0x7ff395b2ccc0>
|
||||
```
|
||||
|
||||
This gives us no insight as to the utility of the object, and will result in horrible a debugging experience.
|
||||
|
||||
A meaningful representation could be implemented by defining a `__repr__`method in the class definition.
|
||||
|
||||
```
|
||||
def __repr__(self):
|
||||
return self.val
|
||||
```
|
||||
|
||||
Now we get a meaningful representation of the object:
|
||||
|
||||
```
|
||||
>>> a = Number(1)
|
||||
>>> a
|
||||
>>> 1
|
||||
```
|
||||
|
||||
`dataclass` automatically add a `__repr__ `function, so that we don’t have to manually implement it.
|
||||
|
||||
```
|
||||
@dataclass
|
||||
class Number:
|
||||
val: int = 0
|
||||
```
|
||||
|
||||
```
|
||||
>>> a = Number(1)
|
||||
>>> a
|
||||
>>> Number(val = 1)
|
||||
```
|
||||
|
||||
### Data Comparison
|
||||
|
||||
Generally, data objects come with a need to be compared with each other.
|
||||
|
||||
Comparison between two objects `a` and `b` generally consists of the following operations:
|
||||
|
||||
* a < b
|
||||
|
||||
* a > b
|
||||
|
||||
* a == b
|
||||
|
||||
* a >= b
|
||||
|
||||
* a <= b
|
||||
|
||||
In python, it is possible to define [methods][4] in classes that can do the above operations. For the sake of simplicity and to not let this post run amuck, I shall be only demonstrating implementation of `==` and `<`.
|
||||
|
||||
Usual
|
||||
|
||||
```
|
||||
class Number:
|
||||
def __init__( self, val = 0):
|
||||
self.val = val
|
||||
|
||||
def __eq__(self, other):
|
||||
return self.val == other.val
|
||||
|
||||
def __lt__(self, other):
|
||||
return self.val < other.val
|
||||
```
|
||||
|
||||
With `dataclass`
|
||||
|
||||
```
|
||||
@dataclass(order = True)
|
||||
class Number:
|
||||
val: int = 0
|
||||
```
|
||||
|
||||
Yup, that’s it.
|
||||
|
||||
We dont need to define the `__eq__`and `__lt__` methods, because `dataclass`decorator automatically adds them to the class definition for us when called with `order = True`
|
||||
|
||||
Well, how does it do that?
|
||||
|
||||
When you use `dataclass,` it adds a functions `__eq__` and `__lt__` to the class definition. We already know that. So, how do these functions know how to check equality and do comparison?
|
||||
|
||||
A dataclass generated `__eq__` function will compare a tuple of its attributes with a tuple of attributes of the other instance of the same class. In our case here’s what the `automatically` generated `__eq__` function would be equivalent to:
|
||||
|
||||
```
|
||||
def __eq__(self, other):
|
||||
return (self.val,) == (other.val,)
|
||||
```
|
||||
|
||||
Let’s look at a more elaborate example:
|
||||
|
||||
We shall write a dataclass `Person `to hold their `name` and `age`.
|
||||
|
||||
```
|
||||
@dataclass(order = True)
|
||||
class Person:
|
||||
name: str
|
||||
age:int = 0
|
||||
```
|
||||
|
||||
The automatically generated `__eq__` method will be equivalent of:
|
||||
|
||||
```
|
||||
def __eq__(self, other):
|
||||
return (self.name, self.age) == ( other.name, other.age)
|
||||
```
|
||||
|
||||
Pay attention to the order of the attributes. They will always be generated in the order you defined them in the dataclass definition.
|
||||
|
||||
Similarly, the equivalent `__le__` function would be akin to:
|
||||
|
||||
```
|
||||
def __le__(self, other):
|
||||
return (self.name, self.age) <= (other.name, other.age)
|
||||
```
|
||||
|
||||
A need for defining a function like `__le__` generally arises, when you have to sort a list of your data objects. Python’s built-in [sorted][5] function relies on comparing two objects.
|
||||
|
||||
```
|
||||
|
||||
>>> import random
|
||||
```
|
||||
|
||||
```
|
||||
>>> a = [Number(random.randint(1,10)) for _ in range(10)] #generate list of random numbers
|
||||
```
|
||||
|
||||
```
|
||||
>>> a
|
||||
```
|
||||
|
||||
```
|
||||
>>> [Number(val=2), Number(val=7), Number(val=6), Number(val=5), Number(val=10), Number(val=9), Number(val=1), Number(val=10), Number(val=1), Number(val=7)]
|
||||
```
|
||||
|
||||
```
|
||||
>>> sorted_a = sorted(a) #Sort Numbers in ascending order
|
||||
```
|
||||
|
||||
```
|
||||
>>> [Number(val=1), Number(val=1), Number(val=2), Number(val=5), Number(val=6), Number(val=7), Number(val=7), Number(val=9), Number(val=10), Number(val=10)]
|
||||
```
|
||||
|
||||
```
|
||||
>>> reverse_sorted_a = sorted(a, reverse = True) #Sort Numbers in descending order
|
||||
```
|
||||
|
||||
```
|
||||
>>> reverse_sorted_a
|
||||
```
|
||||
|
||||
```
|
||||
>>> [Number(val=10), Number(val=10), Number(val=9), Number(val=7), Number(val=7), Number(val=6), Number(val=5), Number(val=2), Number(val=1), Number(val=1)]
|
||||
|
||||
```
|
||||
|
||||
### `dataclass` as a callable decorator
|
||||
|
||||
It is not always desirable to have all the `dunder` methods defined. Your use case might only consist of storing the values and checking equality. Thus, you only need the `__init__` and `__eq__` methods defined. If we could tell the decorator to not generate the other methods, it would reduce some overhead and we shall have correct operations available on the data object.
|
||||
|
||||
Fortunately, this can be achieved by using `dataclass` decorator as a callable.
|
||||
|
||||
From the official [docs][6], the decorator can be used as a callable with the following arguments:
|
||||
|
||||
```
|
||||
@dataclass(init=True, repr=True, eq=True, order=False, unsafe_hash=False, frozen=False)
|
||||
class C:
|
||||
…
|
||||
```
|
||||
|
||||
1. `init` : By default an `__init__` method will be generated. If passed as `False`, the class will not have an `__init__` method.
|
||||
|
||||
2. `repr` : `__repr__` method is generated by default. If passed as `False`, the class will not have an `__repr__` method.
|
||||
|
||||
3. `eq`: By default the `__eq__` method will be generated. If passed as `False`, the `__eq__` method will not be added by `dataclass`, but will default to the `object.__eq__`.
|
||||
|
||||
4. `order` : By default `__gt__` , `__ge__`, `__lt__`, `__le__` methods will be generated. If passed as `False`, they are omitted.
|
||||
|
||||
We shall discuss `frozen` in a while. The `unsafe_hash` argument deserves a separate post because of its complicated use cases.
|
||||
|
||||
Now, back to our use case, here’s what we need:
|
||||
|
||||
1. `__init__`
|
||||
2. `__eq__`
|
||||
|
||||
These functions are generated by default, so what we need is to not have the other functions generated. How do we do that? Simply pass the relevant arguments as false to the generator.
|
||||
|
||||
```
|
||||
@dataclass(repr = False) # order, unsafe_hash and frozen are False
|
||||
class Number:
|
||||
val: int = 0
|
||||
```
|
||||
|
||||
```
|
||||
>>> a = Number(1)
|
||||
```
|
||||
|
||||
```
|
||||
>>> a
|
||||
```
|
||||
|
||||
```
|
||||
>>> <__main__.Number object at 0x7ff395afe898>
|
||||
```
|
||||
|
||||
```
|
||||
>>> b = Number(2)
|
||||
```
|
||||
|
||||
```
|
||||
>>> c = Number(1)
|
||||
```
|
||||
|
||||
```
|
||||
>>> a == b
|
||||
```
|
||||
|
||||
```
|
||||
>>> False
|
||||
```
|
||||
|
||||
```
|
||||
>>> a < b
|
||||
```
|
||||
|
||||
```
|
||||
>>> Traceback (most recent call last):
|
||||
File “<stdin>”, line 1, in <module>
|
||||
TypeError: ‘<’ not supported between instances of ‘Number’ and ‘Number’
|
||||
```
|
||||
|
||||
### Frozen Instances
|
||||
|
||||
Frozen Instances are objects whose attributes cannot be modified after the object has been initialized.
|
||||
|
||||
> It is not possible to create truly immutable Python objects
|
||||
|
||||
To create immutable attributes on an object in Python is an arduous task, and something that I won’t dive into in this post.
|
||||
|
||||
Here’s what we expect from an immutable object:
|
||||
|
||||
```
|
||||
>>> a = Number(10) #Assuming Number class is immutable
|
||||
```
|
||||
|
||||
```
|
||||
>>> a.val = 10 # Raises Error
|
||||
```
|
||||
|
||||
With Dataclasses it is possible to define a frozen object by using `dataclass`decorator as a callable with argument `frozen=True` .
|
||||
|
||||
When a frozen dataclass object is instantiated, any attempt to modify the attributes of the object raises `FrozenInstanceError`.
|
||||
|
||||
```
|
||||
@dataclass(frozen = True)
|
||||
class Number:
|
||||
val: int = 0
|
||||
```
|
||||
|
||||
```
|
||||
>>> a = Number(1)
|
||||
```
|
||||
|
||||
```
|
||||
>>> a.val
|
||||
```
|
||||
|
||||
```
|
||||
>>> 1
|
||||
```
|
||||
|
||||
```
|
||||
>>> a.val = 2
|
||||
```
|
||||
|
||||
```
|
||||
>>> Traceback (most recent call last):
|
||||
File “<stdin>”, line 1, in <module>
|
||||
File “<string>”, line 3, in __setattr__
|
||||
dataclasses.FrozenInstanceError: cannot assign to field ‘val’
|
||||
```
|
||||
|
||||
So a frozen instance is a great way of storing
|
||||
|
||||
* constants
|
||||
|
||||
* settings
|
||||
|
||||
These generally do not change over the lifetime of the application and any attempt to modify them should generally be warded off.
|
||||
|
||||
### Post init processing
|
||||
|
||||
With Dataclasses the requirement of defining an `__init__` method to assign variables to `self` has been taken care of. But now we lose the flexibility of making function-calls/processing that might be required immediately after the variables have been assigned.
|
||||
|
||||
Let us discuss a use case where we define a class `Float` to contain float numbers, and we calculate the integer and decimal parts immediately after initialization.
|
||||
|
||||
Usual
|
||||
|
||||
```
|
||||
import math
|
||||
```
|
||||
|
||||
```
|
||||
class Float:
|
||||
def __init__(self, val = 0):
|
||||
self.val = val
|
||||
self.process()
|
||||
|
||||
def process(self):
|
||||
self.decimal, self.integer = math.modf(self.val)
|
||||
|
||||
>>> a = Float( 2.2)
|
||||
```
|
||||
|
||||
```
|
||||
>>> a.decimal
|
||||
```
|
||||
|
||||
```
|
||||
>>> 0.2000
|
||||
```
|
||||
|
||||
```
|
||||
>>> a.integer
|
||||
```
|
||||
|
||||
```
|
||||
>>> 2.0
|
||||
```
|
||||
|
||||
Fortunately, post initialization processing is already taken care of with [__post_init__][9] method.
|
||||
|
||||
The generated `__init__` method calls the `__post_init__` method before returning. So, any processing can be made in this functions.
|
||||
|
||||
```
|
||||
import math
|
||||
```
|
||||
|
||||
```
|
||||
@dataclass
|
||||
class FloatNumber:
|
||||
val: float = 0.0
|
||||
|
||||
def __post_init__(self):
|
||||
self.decimal, self.integer = math.modf(self.val)
|
||||
|
||||
>>> a = Number(2.2)
|
||||
```
|
||||
|
||||
```
|
||||
>>> a.val
|
||||
```
|
||||
|
||||
```
|
||||
>>> 2.2
|
||||
```
|
||||
|
||||
```
|
||||
>>> a.integer
|
||||
```
|
||||
|
||||
```
|
||||
>>> 2.0
|
||||
```
|
||||
|
||||
```
|
||||
>>> a.decimal
|
||||
```
|
||||
|
||||
```
|
||||
>>> 0.2
|
||||
```
|
||||
|
||||
Neat!
|
||||
|
||||
|
||||
### Inheritance
|
||||
|
||||
`Dataclasses` support inheritance like normal python classes.
|
||||
|
||||
So, the attributes defined in the parent class will be available in the child class.
|
||||
|
||||
```
|
||||
@dataclass
|
||||
class Person:
|
||||
age: int = 0
|
||||
name: str
|
||||
```
|
||||
|
||||
```
|
||||
@dataclass
|
||||
class Student(Person):
|
||||
grade: int
|
||||
```
|
||||
|
||||
```
|
||||
>>> s = Student(20, "John Doe", 12)
|
||||
```
|
||||
|
||||
```
|
||||
>>> s.age
|
||||
```
|
||||
|
||||
```
|
||||
>>> 20
|
||||
```
|
||||
|
||||
```
|
||||
>>> s.name
|
||||
```
|
||||
|
||||
```
|
||||
>>> "John Doe"
|
||||
```
|
||||
|
||||
```
|
||||
>>> s.grade
|
||||
```
|
||||
|
||||
```
|
||||
>>> 12
|
||||
```
|
||||
|
||||
Pay attention to the fact that the arguments to `Student` are in the order of fields defined in the class definition.
|
||||
|
||||
What about the behavior of `__post_init__` during inheritance?
|
||||
|
||||
Since `__post_init__` is just another function, it has to be invoked in the conventional form:
|
||||
|
||||
```
|
||||
@dataclass
|
||||
class A:
|
||||
a: int
|
||||
|
||||
def __post_init__(self):
|
||||
print("A")
|
||||
```
|
||||
|
||||
```
|
||||
@dataclass
|
||||
class B(A):
|
||||
b: int
|
||||
|
||||
def __post_init__(self):
|
||||
print("B")
|
||||
```
|
||||
|
||||
```
|
||||
>>> a = B(1,2)
|
||||
```
|
||||
|
||||
```
|
||||
>>> B
|
||||
```
|
||||
|
||||
In the above example, only `B's` `__post_init__` is called. How do we invoke `A's` `__post_init__` ?
|
||||
|
||||
Since it is a function of the parent class, it can be invoked using `super.`
|
||||
|
||||
```
|
||||
@dataclass
|
||||
class B(A):
|
||||
b: int
|
||||
|
||||
def __post_init__(self):
|
||||
super().__post_init__() #Call post init of A
|
||||
print("B")
|
||||
```
|
||||
|
||||
```
|
||||
>>> a = B(1,2)
|
||||
```
|
||||
|
||||
```
|
||||
>>> A
|
||||
B
|
||||
```
|
||||
|
||||
### Conclusion
|
||||
|
||||
So, above are a few ways in which Dataclasses make life easier for Python developers.
|
||||
I have tried to be thorough and cover most of the use cases, yet, no man is perfect. Reach out if you find mistakes, or want me to pay attention to relevant use cases.
|
||||
|
||||
I shall cover [dataclasses.field][10] and `unsafe_hash` in different posts.
|
||||
|
||||
Follow me on [Github][11], [Twitter][12].
|
||||
|
||||
Update: Post for `dataclasses.field` can be found [here][13].
|
||||
|
||||
--------------------------------------------------------------------------------
|
||||
|
||||
via: https://medium.com/mindorks/understanding-python-dataclasses-part-1-c3ccd4355c34
|
||||
|
||||
作者:[Shikhar Chauhan][a]
|
||||
译者:[译者ID](https://github.com/译者ID)
|
||||
校对:[校对者ID](https://github.com/校对者ID)
|
||||
|
||||
本文由 [LCTT](https://github.com/LCTT/TranslateProject) 原创编译,[Linux中国](https://linux.cn/) 荣誉推出
|
||||
|
||||
[a]:https://medium.com/@xsschauhan?source=post_header_lockup
|
||||
[1]:https://medium.com/@xsschauhan/understanding-python-dataclasses-part-2-660ecc11c9b8
|
||||
[2]:https://docs.python.org/3.7/library/dataclasses.html#dataclasses.dataclass
|
||||
[3]:https://stackoverflow.com/q/32557920/4333721
|
||||
[4]:https://docs.python.org/3/reference/datamodel.html#object.__lt__
|
||||
[5]:https://docs.python.org/3.7/library/functions.html#sorted
|
||||
[6]:https://docs.python.org/3/library/dataclasses.html#dataclasses.dataclass
|
||||
[7]:http://twitter.com/dataclass
|
||||
[8]:http://twitter.com/dataclass
|
||||
[9]:https://docs.python.org/3/library/dataclasses.html#post-init-processing
|
||||
[10]:https://docs.python.org/3/library/dataclasses.html#dataclasses.field
|
||||
[11]:http://github.com/xssChauhan/
|
||||
[12]:https://twitter.com/xssChauhan
|
||||
[13]:https://medium.com/@xsschauhan/understanding-python-dataclasses-part-2-660ecc11c9b8
|
394
sources/tech/20180710 Python Sets What Why and How.md
Normal file
394
sources/tech/20180710 Python Sets What Why and How.md
Normal file
@ -0,0 +1,394 @@
|
||||
MjSeven is translating
|
||||
|
||||
|
||||
Python Sets: What, Why and How
|
||||
============================================================
|
||||
|
||||
posted on 07/10/2018 by [wilfredinni][5]
|
||||
|
||||

|
||||
|
||||
Python comes equipped with several built-in data types to help us organize our data. These structures include lists, dictionaries, tuples and sets.
|
||||
|
||||
From the Python 3 documentation:
|
||||
|
||||
> A set is an _unordered collection_ with no _duplicate elements_ . Basic uses include _membership testing_ and _eliminating duplicate entries_ . Set objects also support mathematical operations like _union_ , _intersection_ , _difference_ , and _symmetric difference_ .
|
||||
|
||||
In this article, we are going to review and see examples of every one of the elements listed in the above definition. Let's start right away and see how we can create them.
|
||||
|
||||
### Initializing a Set
|
||||
|
||||
There are two ways to create a set: one is to provide the built-in function `set()` with a list of elements, and the other is to use the curly braces `{}`.
|
||||
|
||||
Initializing a set using the `set()` built-in function:
|
||||
|
||||
```
|
||||
>>> s1 = set([1, 2, 3])
|
||||
>>> s1
|
||||
{1, 2, 3}
|
||||
>>> type(s1)
|
||||
<class 'set'>
|
||||
|
||||
```
|
||||
|
||||
Initializing a set using curly braces `{}`
|
||||
|
||||
```
|
||||
>>> s2 = {3, 4, 5}
|
||||
>>> s2
|
||||
{3, 4, 5}
|
||||
>>> type(s2)
|
||||
<class 'set'>
|
||||
>>>
|
||||
|
||||
```
|
||||
|
||||
As you can see, both options are valid. The problem comes when what we want is an empty one:
|
||||
|
||||
```
|
||||
>>> s = {}
|
||||
>>> type(s)
|
||||
<class 'dict'>
|
||||
|
||||
```
|
||||
|
||||
That's right, we will get a dictionary instead of a set if we use empty curly braces =)
|
||||
|
||||
It's a good moment to mention that for the sake of simplicity, all the examples provided in this article will use single digit integers, but sets can have all the [hashable][6] data types that Python support. In other words, integers, strings and tuples, but not _mutable_ items like _lists_ or _dictionaries_ :
|
||||
|
||||
```
|
||||
>>> s = {1, 'coffee', [4, 'python']}
|
||||
Traceback (most recent call last):
|
||||
File "<stdin>", line 1, in <module>
|
||||
TypeError: unhashable type: 'list'
|
||||
|
||||
```
|
||||
|
||||
Now that you know how to create a set and what type of elements it can contain, let's continue and see _why_ we should always have them in our toolkit.
|
||||
|
||||
### Why You Should Use Them
|
||||
|
||||
When writing code, you can do it in more than a single way. Some are considered to be pretty bad, and others, _clear, concise and maintainable_ . Or " [_pythonic_][7] ".
|
||||
|
||||
From the [The Hitchhiker’s Guide to Python][8]:
|
||||
|
||||
> When a veteran Python developer (a Pythonista) calls portions of code not “Pythonic”, they usually mean that these lines of code do not follow the common guidelines and fail to express its intent in what is considered the best (hear: most readable) way.
|
||||
|
||||
Let's start exploring the way that Python sets can help us not just with readability, but also speeding up our programs execution time.
|
||||
|
||||
### Unordered Collection of Elements
|
||||
|
||||
First things first: you can't access a set element using indexes.
|
||||
|
||||
```
|
||||
>>> s = {1, 2, 3}
|
||||
>>> s[0]
|
||||
Traceback (most recent call last):
|
||||
File "<stdin>", line 1, in <module>
|
||||
TypeError: 'set' object does not support indexing
|
||||
|
||||
```
|
||||
|
||||
Or modify them with slices:
|
||||
|
||||
```
|
||||
>>> s[0:2]
|
||||
Traceback (most recent call last):
|
||||
File "<stdin>", line 1, in <module>
|
||||
TypeError: 'set' object is not subscriptable
|
||||
|
||||
```
|
||||
|
||||
BUT, if what we need is to remove duplicates, or do mathematical operations like combining lists (unions), we can, and _SHOULD_ always use Sets.
|
||||
|
||||
I have to mention that when iterating over, sets are outperformed by lists, so prefer them if that is what you need. Why? well, this article does not intend to explain the inner workings of sets, but if you are interested, here are a couple of links where you can read about it:
|
||||
|
||||
* [TimeComplexity][1]
|
||||
|
||||
* [How is set() implemented?][2]
|
||||
|
||||
* [Python Sets vs Lists][3]
|
||||
|
||||
* [Is there any advantage or disadvantage to using sets over list comps to ensure a list of unique entries?][4]
|
||||
|
||||
### No Duplicate Items
|
||||
|
||||
While writing this I cannot stop thinking in all the times I used the _for_ loop and the _if_ statement to check and remove duplicate elements in a list. My face turns red remembering that, more than once, I wrote something like this:
|
||||
|
||||
```
|
||||
>>> my_list = [1, 2, 3, 2, 3, 4]
|
||||
>>> no_duplicate_list = []
|
||||
>>> for item in my_list:
|
||||
... if item not in no_duplicate_list:
|
||||
... no_duplicate_list.append(item)
|
||||
...
|
||||
>>> no_duplicate_list
|
||||
[1, 2, 3, 4]
|
||||
|
||||
```
|
||||
|
||||
Or used a list comprehension:
|
||||
|
||||
```
|
||||
>>> my_list = [1, 2, 3, 2, 3, 4]
|
||||
>>> no_duplicate_list = []
|
||||
>>> [no_duplicate_list.append(item) for item in my_list if item not in no_duplicate_list]
|
||||
[None, None, None, None]
|
||||
>>> no_duplicate_list
|
||||
[1, 2, 3, 4]
|
||||
|
||||
```
|
||||
|
||||
But it's ok, nothing of that matters anymore because we now have the sets in our arsenal:
|
||||
|
||||
```
|
||||
>>> my_list = [1, 2, 3, 2, 3, 4]
|
||||
>>> no_duplicate_list = list(set(my_list))
|
||||
>>> no_duplicate_list
|
||||
[1, 2, 3, 4]
|
||||
>>>
|
||||
|
||||
```
|
||||
|
||||
Now let's use the _timeit_ module and see the excecution time of lists and sets when removing duplicates:
|
||||
|
||||
```
|
||||
>>> from timeit import timeit
|
||||
>>> def no_duplicates(list):
|
||||
... no_duplicate_list = []
|
||||
... [no_duplicate_list.append(item) for item in list if item not in no_duplicate_list]
|
||||
... return no_duplicate_list
|
||||
...
|
||||
>>> # first, let's see how the list perform:
|
||||
>>> print(timeit('no_duplicates([1, 2, 3, 1, 7])', globals=globals(), number=1000))
|
||||
0.0018683355819786227
|
||||
|
||||
```
|
||||
|
||||
```
|
||||
>>> from timeit import timeit
|
||||
>>> # and the set:
|
||||
>>> print(timeit('list(set([1, 2, 3, 1, 2, 3, 4]))', number=1000))
|
||||
0.0010220493243764395
|
||||
>>> # faster and cleaner =)
|
||||
|
||||
```
|
||||
|
||||
Not only we write _fewer lines_ with sets than with lists comprehensions, we also obtain more _readable_ and _performant_ code.
|
||||
|
||||
Note: remember that sets are unordered, so there is no guarantee that when converting them back to a list the order of the elements is going to be preserved.
|
||||
|
||||
From the [Zen of Python][9]:
|
||||
|
||||
> Beautiful is better than ugly.
|
||||
> Explicit is better than implicit.
|
||||
> Simple is better than complex.
|
||||
> Flat is better than nested.
|
||||
|
||||
Aren't sets just Beautiful, Explicit, Simple and Flat? =)
|
||||
|
||||
### Membership Tests
|
||||
|
||||
Every time we use an _if_ statement to check if an element is, for example, in a list, you are doing a membership test:
|
||||
|
||||
```
|
||||
my_list = [1, 2, 3]
|
||||
>>> if 2 in my_list:
|
||||
... print('Yes, this is a membership test!')
|
||||
...
|
||||
Yes, this is a membership test!
|
||||
|
||||
```
|
||||
|
||||
And sets are more performant than lists when doing them:
|
||||
|
||||
```
|
||||
>>> from timeit import timeit
|
||||
>>> def in_test(iterable):
|
||||
... for i in range(1000):
|
||||
... if i in iterable:
|
||||
... pass
|
||||
...
|
||||
>>> timeit('in_test(iterable)',
|
||||
... setup="from __main__ import in_test; iterable = list(range(1000))",
|
||||
... number=1000)
|
||||
12.459663048726043
|
||||
|
||||
```
|
||||
|
||||
```
|
||||
>>> from timeit import timeit
|
||||
>>> def in_test(iterable):
|
||||
... for i in range(1000):
|
||||
... if i in iterable:
|
||||
... pass
|
||||
...
|
||||
>>> timeit('in_test(iterable)',
|
||||
... setup="from __main__ import in_test; iterable = set(range(1000))",
|
||||
... number=1000)
|
||||
0.12354438152988223
|
||||
>>>
|
||||
|
||||
```
|
||||
|
||||
Note: the above tests come from [this][10] StackOverflow thread.
|
||||
|
||||
So if you are doing comparisons like this in huge lists, it should speed you a good bit if you convert that list into a set.
|
||||
|
||||
### How to Use Them
|
||||
|
||||
Now that you know what a set is and why you should use them, let's do a quick tour and see how can we modify and operate with them.
|
||||
|
||||
### Adding Elements
|
||||
|
||||
Depending on the number of elements to add, we will have to choose between the `add()` and `update()` methods.
|
||||
|
||||
`add()` will add a single element:
|
||||
|
||||
```
|
||||
>>> s = {1, 2, 3}
|
||||
>>> s.add(4)
|
||||
>>> s
|
||||
{1, 2, 3, 4}
|
||||
|
||||
```
|
||||
|
||||
And `update()` multiple ones:
|
||||
|
||||
```
|
||||
>>> s = {1, 2, 3}
|
||||
>>> s.update([2, 3, 4, 5, 6])
|
||||
>>> s
|
||||
{1, 2, 3, 4, 5, 6}
|
||||
|
||||
```
|
||||
|
||||
Remember, sets remove duplicates.
|
||||
|
||||
### Removing Elements
|
||||
|
||||
If you want to be alerted when your code tries to remove an element that is not in the set, use `remove()`. Otherwise, `discard()` provides a good alternative:
|
||||
|
||||
```
|
||||
>>> s = {1, 2, 3}
|
||||
>>> s.remove(3)
|
||||
>>> s
|
||||
{1, 2}
|
||||
>>> s.remove(3)
|
||||
Traceback (most recent call last):
|
||||
File "<stdin>", line 1, in <module>
|
||||
KeyError: 3
|
||||
|
||||
```
|
||||
|
||||
`discard()` won't raise any errors:
|
||||
|
||||
```
|
||||
>>> s = {1, 2, 3}
|
||||
>>> s.discard(3)
|
||||
>>> s
|
||||
{1, 2}
|
||||
>>> s.discard(3)
|
||||
>>> # nothing happens!
|
||||
|
||||
```
|
||||
|
||||
We can also use `pop()` to randomly discard an element:
|
||||
|
||||
```
|
||||
>>> s = {1, 2, 3, 4, 5}
|
||||
>>> s.pop() # removes an arbitrary element
|
||||
1
|
||||
>>> s
|
||||
{2, 3, 4, 5}
|
||||
|
||||
```
|
||||
|
||||
Or `clear()` to remove all the values from a set:
|
||||
|
||||
```
|
||||
>>> s = {1, 2, 3, 4, 5}
|
||||
>>> s.clear() # discard all the items
|
||||
>>> s
|
||||
set()
|
||||
|
||||
```
|
||||
|
||||
### union()
|
||||
|
||||
`union()` or `|` will create a new set that contains all the elements from the sets we provide:
|
||||
|
||||
```
|
||||
>>> s1 = {1, 2, 3}
|
||||
>>> s2 = {3, 4, 5}
|
||||
>>> s1.union(s2) # or 's1 | s2'
|
||||
{1, 2, 3, 4, 5}
|
||||
|
||||
```
|
||||
|
||||
### intersection()
|
||||
|
||||
`intersection` or `&` will return a set containing only the elements that are common in all of them:
|
||||
|
||||
```
|
||||
>>> s1 = {1, 2, 3}
|
||||
>>> s2 = {2, 3, 4}
|
||||
>>> s3 = {3, 4, 5}
|
||||
>>> s1.intersection(s2, s3) # or 's1 & s2 & s3'
|
||||
{3}
|
||||
|
||||
```
|
||||
|
||||
### difference()
|
||||
|
||||
Using `diference()` or `-`, creates a new set with the values that are in "s1" but not in "s2":
|
||||
|
||||
```
|
||||
>>> s1 = {1, 2, 3}
|
||||
>>> s2 = {2, 3, 4}
|
||||
>>> s1.difference(s2) # or 's1 - s2'
|
||||
{1}
|
||||
|
||||
```
|
||||
|
||||
### symmetric_diference()
|
||||
|
||||
`symetric_difference` or `^` will return all the values that are not common between the sets.
|
||||
|
||||
```
|
||||
>>> s1 = {1, 2, 3}
|
||||
>>> s2 = {2, 3, 4}
|
||||
>>> s1.symmetric_difference(s2) # or 's1 ^ s2'
|
||||
{1, 4}
|
||||
|
||||
```
|
||||
|
||||
### Conclusions
|
||||
|
||||
I hope that after reading this article you know what a set is, how to manipulate their elements and the operations they can perform. Knowing when to use a set will definitely help you write cleaner code and speed up your programs.
|
||||
|
||||
If you have any doubts, please leave a comment and I will gladly try to answer them. Also, don´t forget that if you already understand sets, they have their own [place][11] in the [Python Cheatsheet][12], where you can have a quick reference and refresh what you already know.
|
||||
|
||||
--------------------------------------------------------------------------------
|
||||
|
||||
via: https://www.pythoncheatsheet.org/blog/python-sets-what-why-how
|
||||
|
||||
作者:[wilfredinni][a]
|
||||
译者:[译者ID](https://github.com/译者ID)
|
||||
校对:[校对者ID](https://github.com/校对者ID)
|
||||
|
||||
本文由 [LCTT](https://github.com/LCTT/TranslateProject) 原创编译,[Linux中国](https://linux.cn/) 荣誉推出
|
||||
|
||||
[a]:https://www.pythoncheatsheet.org/author/wilfredinni
|
||||
[1]:https://wiki.python.org/moin/TimeComplexity
|
||||
[2]:https://stackoverflow.com/questions/3949310/how-is-set-implemented
|
||||
[3]:https://stackoverflow.com/questions/2831212/python-sets-vs-lists
|
||||
[4]:https://mail.python.org/pipermail/python-list/2011-June/606738.html
|
||||
[5]:https://www.pythoncheatsheet.org/author/wilfredinni
|
||||
[6]:https://docs.python.org/3/glossary.html#term-hashable
|
||||
[7]:http://docs.python-guide.org/en/latest/writing/style/
|
||||
[8]:http://docs.python-guide.org/en/latest/
|
||||
[9]:https://www.python.org/dev/peps/pep-0020/
|
||||
[10]:https://stackoverflow.com/questions/2831212/python-sets-vs-lists
|
||||
[11]:https://www.pythoncheatsheet.org/#sets
|
||||
[12]:https://www.pythoncheatsheet.org/
|
@ -1,41 +0,0 @@
|
||||
我的第一个系统管理员错误
|
||||
======
|
||||
|
||||
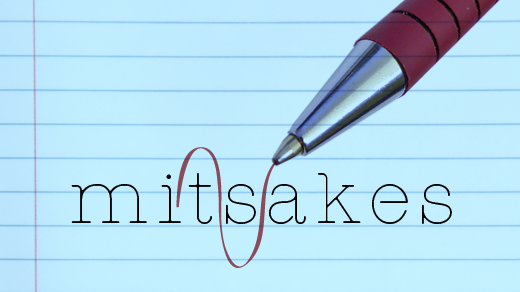
|
||||
|
||||
如果你在 IT 领域工作,你知道事情永远不会像你想象的那样完好。在某些时候,你会遇到错误或出现问题,你最终必须解决问题。这是系统管理员的工作。
|
||||
|
||||
作为人类,我们都会犯错误。有时,我们是过程中的错误,或者我们出了什么问题。结果,我们最终必须解决自己的错误。它们会发生。我们都犯错误,错别字或故障。
|
||||
|
||||
作为一名年轻的系统管理员,我艰难地学到了这一课。我犯了一个大错。但是多亏了上级的指导,我学会了不去纠缠于我的错误,而是制定一个“错误策略”来设置正确的事情。从错误中吸取教训。克服它,继续前进。
|
||||
|
||||
我的第一份工作是一家小公司的 Unix 系统管理员。真的,我是一名初级系统管理员,但我大部分时间都独自工作。我们是一个小型 IT 团队,只有我们三个人。我是 20 或 30 台 Unix 工作站和服务器的唯一系统管理员。另外两个支持 Windows 服务器和桌面。
|
||||
|
||||
任何阅读这篇文章的系统管理员都不会惊讶地发现,作为一个不成熟的初级系统管理员,我最终在错误的目录中运行了 `rm` 命令。作为 root,我以为我正在为我们的某个程序删除一些陈旧的缓存文件。相反,我错误地清除了 `/ etc` 目录中的所有文件。糟糕。
|
||||
|
||||
我意识到反了错误是看到了一条错误消息,`rm` 无法删除某些子目录。但缓存目录应该只包含文件!我立即停止了 `rm` 命令,看着我做了什么。然后我惊慌失措。一下子,无数个想法涌入了我的脑中。我刚刚销毁了一台重要的服务器吗?系统会发生什么?我会被解雇吗?
|
||||
|
||||
幸运的是,我运行的是 `rm *` 而不是 `rm -rf *`,因此我只删除了文件。子目录仍在那里。但这并没有让我感觉更好。
|
||||
|
||||
我立刻去找我的主管告诉她我做了什么。她看到我对自己的错误感到愚蠢,但是我犯的。尽管紧迫,她花了几分钟时间跟我做了一些指导。 她说:“你不是第一个这样做的人,在你这种情况下,别人会怎么做?”这帮助我平静下来并专注。我开始更少考虑我刚刚做的愚蠢事情,而更多地考虑我接下来要做的事情。
|
||||
|
||||
我做了一个简单的策略:不要重启服务器。使用相同的系统作为模板,并重新创建 `/ etc` 目录。
|
||||
|
||||
制定了行动计划后,剩下的就很容易了。只需运行正确的命令即可从另一台服务器复制 `/ etc` 文件并编辑配置,使其与系统匹配。多亏了我对所有东西都做记录,我使用现有的文档进行最后的调整。我避免了完全恢复服务器,这意味着一个巨大的中断。
|
||||
|
||||
可以肯定的是,我从这个错误中吸取了教训。在接下来作为系统管理员的日子中,我总是在运行任何命令之前确认我所在的目录。
|
||||
|
||||
我还学习了构建“错误策略”的价值。当事情出错时,恐慌并思考接下来可能发生的所有坏事是很自然的。这是人性。但是制定一个“错误策略”可以帮助我不再担心出了什么问题,而是专注于让事情变得更好。我仍然会想一下,但是知道我接下来的步骤可以让我“克服它”。
|
||||
|
||||
--------------------------------------------------------------------------------
|
||||
|
||||
via: https://opensource.com/article/18/7/my-first-sysadmin-mistake
|
||||
|
||||
作者:[Jim Hall][a]
|
||||
选题:[lujun9972](https://github.com/lujun9972)
|
||||
译者:[geekpi](https://github.com/geekpi)
|
||||
校对:[校对者ID](https://github.com/校对者ID)
|
||||
|
||||
本文由 [LCTT](https://github.com/LCTT/TranslateProject) 原创编译,[Linux中国](https://linux.cn/) 荣誉推出
|
||||
|
||||
[a]:https://opensource.com/users/jim-hall
|
@ -1,154 +0,0 @@
|
||||
在绝大部分类型的机器上安装 NVIDIA 显卡驱动
|
||||
======
|
||||
|
||||
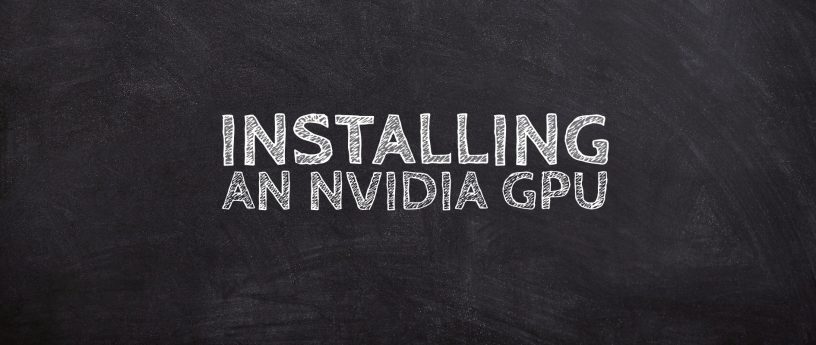
|
||||
|
||||
无论是研究还是娱乐,安装一个最新的显卡驱动都能提升你的计算机性能,并且使你能全方位地实现新功能。本安装指南使用 Fedora 28 的新的第三方仓库来安装 NVIDIA 驱动。它将引导您完成硬件和软件两方面的安装,并且涵盖你需要得到的 NVIDIA 显卡启动和运行的一切。这个流程适用于任何支持 UEFI 的计算机和任意新的 NVIDIA 显卡。
|
||||
|
||||
### 准备
|
||||
|
||||
本指南依赖于下面这些材料:
|
||||
|
||||
* 一台使用 UEFI 的计算机,如果你不确定你的电脑是否有这个这个固件,请运行 sudo dmidecode -t 0。如果输出中出现了“UEFI is supported”,你的安装过程就可以继续了。不然的话,虽然可以在技术上更新部分电脑来支持 UEFI,但是这个过程的要求很苛刻,我们通常不建议你这么使用。
|
||||
* 一个现代的,支持 UEFI 的 NVIDIA 的显卡
|
||||
* 一个满足你的 NVIDIA 显卡的功率和接线要求的电源(有关详细信息,请参考硬件和修改的章节)
|
||||
* 网络连接
|
||||
* Fedora 28 系统
|
||||
|
||||
|
||||
|
||||
### 安装实例
|
||||
|
||||
这个安装示例使用的是:
|
||||
|
||||
* 一台 Optiplex 9010 的主机(一台相当老的机器)
|
||||
* NVIDIA GeForce GTX 1050 Ti XLR8 游戏超频版 4 GB GDDR5 PCI Express 3.0 显卡
|
||||
* 为了满足新显卡的电源要求,电源升级为 EVGA – 80 PLUS 600 W ATX 12V/EPS 12V,这个最新的 PSU 比推荐的最低要求高了 300 W,但在大部分情况下,满足推荐的最低要求就足够了。
|
||||
* 然后,当然的,Fedora 28 也别忘了.
|
||||
|
||||
|
||||
|
||||
### 硬件和修改
|
||||
|
||||
#### PSU
|
||||
|
||||
打开你的台式机的机箱,检查印刷在电源上的最大输出功率。然后,查看你的 NVIDIA 显卡的文档,确定推荐的最小电源功率要求(以瓦特为单位)。除此之外,检查你的显卡,看它是否需要额外的接线,例如 6 针口连接器,大多数的入门级显卡只从主板获取电力,但是有一些显卡需要额外的电力,如果出现以下情况,你需要升级的 PSU:
|
||||
|
||||
1. 你的电源的最大输出功率低于显卡建议的最小电源功率。注意:根据一些显卡厂家的说法,比起推荐的功率,预先构建的系统可能会需要更多或更少的功率,而这取决于系统的配置。如果你使用的是一个特别耗电或者特别节能的配置,请灵活决定你的电源需求。
|
||||
2. 你的电源没有提供必须的接线口来为你的显卡供电。
|
||||
|
||||
|
||||
|
||||
PSU 的更换很容易,但是在你拆除你当前正在使用的电源之前,请务必注意你的接线布局。除此之外,请确保你选择的 PSU 适合你的机箱。
|
||||
|
||||
#### CPU
|
||||
|
||||
虽然在大多数老机器上安装高性能的 NVIDIA 显卡是可能的,但是一个缓慢或受损的 CPU 会阻碍显卡性能的发挥,如果要计算在你的机器上瓶颈效果的影响,请点击这里。知道你的 CPU 性能来避免高性能的显卡和 CPU 无法保持匹配是很重要的。升级你的 CPU 是一个潜在的考虑因素。
|
||||
|
||||
#### 主板
|
||||
|
||||
在继续进行之前,请确认你的主板和你选择的显卡是兼容的。你的显卡应该插在最靠近散热器的 PCI-E x16 插槽中。确保你的设置为显卡预留了足够的空间。此外,请注意,现在大部分的显卡使用的都是 PCI-E 3.0 技术。虽然这些显卡如果插在 PCI-E 3.0 插槽上会运行地最好,但如果插在一个旧版的插槽上的话,性能也不会受到太大的影响。
|
||||
|
||||
### 安装
|
||||
```
|
||||
sudo dnf update
|
||||
|
||||
```
|
||||
|
||||
2\. 然后,使用这条简单的命令进行重启:
|
||||
```
|
||||
reboot
|
||||
|
||||
```
|
||||
|
||||
3\. 在重启之后,安装 Fedora 28 的工作站仓库:
|
||||
```
|
||||
sudo dnf install fedora-workstation-repositories
|
||||
|
||||
```
|
||||
|
||||
4\. 接着,设置 NVIDIA 驱动仓库:
|
||||
```
|
||||
sudo dnf config-manager --set-enabled rpmfusion-nonfree-nvidia-driver
|
||||
|
||||
```
|
||||
|
||||
5\. 然后,再次重启。
|
||||
|
||||
6\. 在这次重启之后,通过下面这条命令验证是否添加了仓库:
|
||||
```
|
||||
sudo dnf repository-packages rpmfusion-nonfree-nvidia-driver info
|
||||
|
||||
```
|
||||
|
||||
如果加载了多个 NVIDIA 工具和它们各自的参数,请继续进行下一步。如果没有,你可能在添加新仓库的时候遇到了一个错误。你应该再试一次。
|
||||
|
||||
7\. 登陆,连接到互联网,然后打开软件应用程序。点击加载项>硬件驱动> NVIDIA Linux 图形驱动>安装。
|
||||
|
||||
接着,再一次重启。
|
||||
|
||||
8\. 在重新启动后,转到侧栏上的‘显示应用程序’,然后打开新添加的 NVIDIA X 服务器设置应用程序。一个图形界面会被打开,然后出现一个对话框并包含以下信息:
|
||||
|
||||
![NVIDIA X Server Prompt][5]
|
||||
|
||||
请参考应用程序的建议,但是在这样做之前,请确保你的 NVIDIA 显卡就在手里,并且已准备好去安装。请注意在以 root 身份运行 nvidia xconfig 的时候,如果在没有立刻安装显卡的情况下关闭电源,这可能会造成严重损坏。这样做可能会导致你的电脑无法启动,并强制你通过重启屏幕来修复系统。重新安装 Fedora 会修复这些问题,但是效果会更加糟糕。
|
||||
|
||||
如果你已准备好继续,请输入下面这条命令:
|
||||
```
|
||||
sudo nvidia-xconfig
|
||||
|
||||
```
|
||||
|
||||
如果系统提示你完成任何地下载,请选择接收然后继续。
|
||||
|
||||
9\. 一旦这个过程完成,关闭所有的应用程序并关闭电脑,拔掉机器的电源。然后,按一下电源按钮来释放掉多有的剩余电量,以此来保护你自己不会被点击。如果你的 PSU 有电源开关,请将其关闭。
|
||||
|
||||
10\. 最后,安装显卡,拔掉老的显卡并将新的显卡插入到正确的 PCI-E x16 插槽中,风扇朝着下方。如果这个位置已经没有空间让风扇通风。那作为代替,如果可以的话就把显卡面朝上放置。成功安装新的显卡之后,关闭你的机箱,插入 PSU ,然后打开计算机,它应该会成功启动。
|
||||
|
||||
**注意:** 要禁用此安装中使用的 NVIDIA 驱动仓库,或者要禁用所有的 Fedora 工作站仓库,请参考这个 Fedora Wiki 页面。
|
||||
|
||||
### 验证
|
||||
|
||||
1\. 如果你新安装的显卡已连接到你的显示器并显示正确,则表明你的 NVIDIA 驱动程序已成功和显卡建立连接。
|
||||
|
||||
如果你想去查看你的设置,或者验证驱动是否在正常工作(如果机箱的主板里安装了两块显卡),再次打开 NVIDIA X 服务器设置应用程序。这次,你应该不会被提示一个错误信息,并且系统会给出有关 X 的设置文件和你的 NVIDIA 显卡的信息。(请参考下面的屏幕截图)
|
||||
|
||||
![NVIDIA X Server Settings][7]
|
||||
|
||||
通过这个应用程序,你可以根据你的需要需改 X 配置文件,并可以监控显卡的性能,时钟速度和温度信息。
|
||||
|
||||
2\. 为确保新显卡以满功率运行,一次显卡性能测试是非常必要的。GL Mark 2,是一个提供后台处理、构建、照明、纹理等等有关信息的标准工具。它提供了一个优秀的解决方案。GL Mark 2 记录了各种各样的图形测试的帧速率,然后输出一个总体的性能评分(这被称为 glmark2 分数)。
|
||||
|
||||
**注意:** glxgears 只会测试你的屏幕或显示器的性能,不会测试显卡本身,请使用 GL Mark 2。
|
||||
|
||||
要运行 GLMark2:
|
||||
|
||||
1. 打开终端并关闭其他所有的应用程序
|
||||
2. 运行 sudo dnf install glmark2 命令
|
||||
3. 运行 glmark2 命令
|
||||
4. 允许运行完整的测试来得到最好的结果。检查帧速率是否符合你对这块显卡的预期。如果你想要额外的验证,你可以查阅网站来确认是否已有你这块显卡的 glmark2 测试评分被公布到网上,你可以比较这个分数来评估你这块显卡的性能。
|
||||
5. 如果你的帧速率或者 glmark2 评分低于预期,请思考潜在的因素。CPU 造成的瓶颈?其他问题导致?
|
||||
|
||||
|
||||
如果诊断的结果很好,就开始享受你的新显卡吧。
|
||||
|
||||
|
||||
--------------------------------------------------------------------------------
|
||||
|
||||
via: https://fedoramagazine.org/install-nvidia-gpu/
|
||||
|
||||
作者:[Justice del Castillo][a]
|
||||
选题:[lujun9972](https://github.com/lujun9972)
|
||||
译者:[hopefully2333](https://github.com/hopefully2333)
|
||||
校对:[校对者ID](https://github.com/校对者ID)
|
||||
|
||||
本文由 [LCTT](https://github.com/LCTT/TranslateProject) 原创编译,[Linux中国](https://linux.cn/) 荣誉推出
|
||||
|
||||
[a]:https://fedoramagazine.org/author/justice/
|
||||
[1]:https://whatis.techtarget.com/definition/Unified-Extensible-Firmware-Interface-UEFI
|
||||
[2]:https://www.cnet.com/products/pny-geforce-gtx-xlr8-gaming-1050-ti-overclocked-edition-graphics-card-gf-gtx-1050-ti-4-gb/specs/
|
||||
[3]:https://www.evga.com/products/product.aspx?pn=100-B1-0600-KR
|
||||
[4]:http://thebottlenecker.com (Home: The Bottle Necker)
|
||||
[5]:https://bytebucket.org/kenneym/fedora-28-nvidia-gpu-installation/raw/7bee7dc6effe191f1f54b0589fa818960a8fa18b/nvidia_xserver_error.jpg?token=c6a7effe35f1c592a155a4a46a068a19fd060a91 (NVIDIA X Sever Prompt)
|
||||
[6]:https://fedoraproject.org/wiki/Workstation/Third_Party_Software_Repositories
|
||||
[7]:https://bytebucket.org/kenneym/fedora-28-nvidia-gpu-installation/raw/7bee7dc6effe191f1f54b0589fa818960a8fa18b/NVIDIA_XCONFIG.png?token=64e1a7be21e5e9ba157f029b65e24e4eef54d88f (NVIDIA X Server Settings)
|
Loading…
Reference in New Issue
Block a user