mirror of
https://github.com/LCTT/TranslateProject.git
synced 2025-02-28 01:01:09 +08:00
Finish Translating 《tech/20160620 Detecting cats in images with OpenCV.md》 (#4162)
* sources/tech/20160609 How to record your terminal session on Linux.md * translated/tech/20160609 How to record your terminal session on Linux.md * sources/tech/20160620 Detecting cats in images with OpenCV.md * sources/tech/20160620 Detecting cats in images with OpenCV.md * sources/tech/20160620 Detecting cats in images with OpenCV.md * sources/tech/20160620 Detecting cats in images with OpenCV.md * sources/tech/20160620 Detecting cats in images with OpenCV.md * translated/tech/20160620 Detecting cats in images with OpenCV.md * remove source
This commit is contained in:
parent
23b2147154
commit
d62ef3e803
@ -1,231 +0,0 @@
|
||||
Detecting cats in images with OpenCV
|
||||
=======================================
|
||||
|
||||
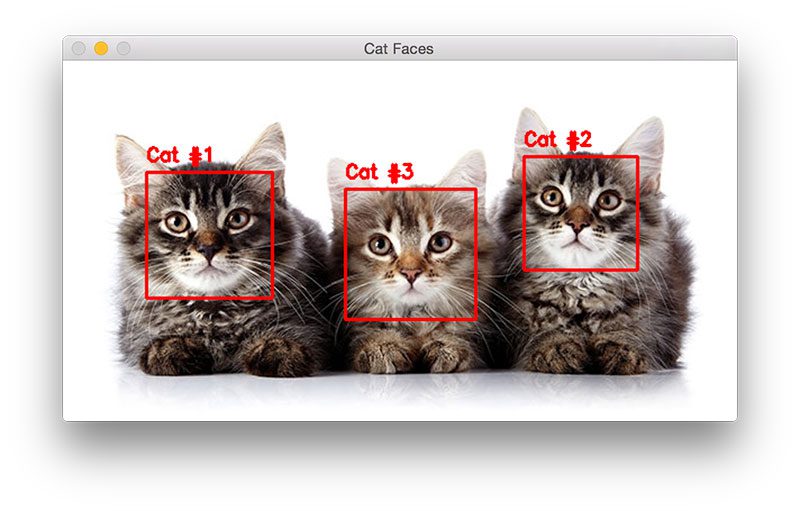
|
||||
|
||||
Did you know that OpenCV can detect cat faces in images…right out-of-the-box with no extras?
|
||||
|
||||
I didn’t either.
|
||||
|
||||
But after [Kendrick Tan broke the story][1], I had to check it out for myself…and do a little investigative work to see how this cat detector seemed to sneak its way into the OpenCV repository without me noticing (much like a cat sliding into an empty cereal box, just waiting to be discovered).
|
||||
|
||||
In the remainder of this blog post, I’ll demonstrate how to use OpenCV’s cat detector to detect cat faces in images. This same technique can be applied to video streams as well.
|
||||
|
||||
>Looking for the source code to this post? [Jump right to the downloads section][2].
|
||||
|
||||
|
||||
### Detecting cats in images with OpenCV
|
||||
|
||||
If you take a look at the [OpenCV repository][3], specifically within the [haarcascades directory][4] (where OpenCV stores all its pre-trained Haar classifiers to detect various objects, body parts, etc.), you’ll notice two files:
|
||||
|
||||
- haarcascade_frontalcatface.xml
|
||||
- haarcascade_frontalcatface_extended.xml
|
||||
|
||||
Both of these Haar cascades can be used detecting “cat faces” in images. In fact, I used these very same cascades to generate the example image at the top of this blog post.
|
||||
|
||||
Doing a little investigative work, I found that the cascades were trained and contributed to the OpenCV repository by the legendary [Joseph Howse][5] who’s authored a good many tutorials, books, and talks on computer vision.
|
||||
|
||||
In the remainder of this blog post, I’ll show you how to utilize Howse’s Haar cascades to detect cats in images.
|
||||
|
||||
Cat detection code
|
||||
|
||||
Let’s get started detecting cats in images with OpenCV. Open up a new file, name it cat_detector.py , and insert the following code:
|
||||
|
||||
### Detecting cats in images with OpenCVPython
|
||||
|
||||
```
|
||||
# import the necessary packages
|
||||
import argparse
|
||||
import cv2
|
||||
|
||||
# construct the argument parse and parse the arguments
|
||||
ap = argparse.ArgumentParser()
|
||||
ap.add_argument("-i", "--image", required=True,
|
||||
help="path to the input image")
|
||||
ap.add_argument("-c", "--cascade",
|
||||
default="haarcascade_frontalcatface.xml",
|
||||
help="path to cat detector haar cascade")
|
||||
args = vars(ap.parse_args())
|
||||
```
|
||||
|
||||
Lines 2 and 3 import our necessary Python packages while Lines 6-12 parse our command line arguments. We only require a single argument here, the input `--image` that we want to detect cat faces in using OpenCV.
|
||||
|
||||
We can also (optionally) supply a path our Haar cascade via the `--cascade` switch. We’ll default this path to `haarcascade_frontalcatface.xml` and assume you have the `haarcascade_frontalcatface.xml` file in the same directory as your cat_detector.py script.
|
||||
|
||||
Note: I’ve conveniently included the code, cat detector Haar cascade, and example images used in this tutorial in the “Downloads” section of this blog post. If you’re new to working with Python + OpenCV (or Haar cascades), I would suggest downloading the provided .zip file to make it easier to follow along.
|
||||
|
||||
Next, let’s detect the cats in our input image:
|
||||
|
||||
```
|
||||
# load the input image and convert it to grayscale
|
||||
image = cv2.imread(args["image"])
|
||||
gray = cv2.cvtColor(image, cv2.COLOR_BGR2GRAY)
|
||||
|
||||
# load the cat detector Haar cascade, then detect cat faces
|
||||
# in the input image
|
||||
detector = cv2.CascadeClassifier(args["cascade"])
|
||||
rects = detector.detectMultiScale(gray, scaleFactor=1.3,
|
||||
minNeighbors=10, minSize=(75, 75))
|
||||
```
|
||||
|
||||
On Lines 15 and 16 we load our input image from disk and convert it to grayscale (a normal pre-processing step before passing the image to a Haar cascade classifier, although not strictly required).
|
||||
|
||||
Line 20 loads our Haar cascade from disk (in this case, the cat detector) and instantiates the cv2.CascadeClassifier object.
|
||||
|
||||
Detecting cat faces in images with OpenCV is accomplished on Lines 21 and 22 by calling the detectMultiScale method of the detector object. We pass four parameters to the detectMultiScale method, including:
|
||||
|
||||
1. Our image, gray , that we want to detect cat faces in.
|
||||
2.A scaleFactor of our [image pyramid][6] used when detecting cat faces. A larger scale factor will increase the speed of the detector, but could harm our true-positive detection accuracy. Conversely, a smaller scale will slow down the detection process, but increase true-positive detections. However, this smaller scale can also increase the false-positive detection rate as well. See the “A note on Haar cascades” section of this blog post for more information.
|
||||
3. The minNeighbors parameter controls the minimum number of detected bounding boxes in a given area for the region to be considered a “cat face”. This parameter is very helpful in pruning false-positive detections.
|
||||
4. Finally, the minSize parameter is pretty self-explanatory. This value ensures that each detected bounding box is at least width x height pixels (in this case, 75 x 75).
|
||||
|
||||
The detectMultiScale function returns rects , a list of 4-tuples. These tuples contain the (x, y)-coordinates and width and height of each detected cat face.
|
||||
|
||||
Finally, let’s draw a rectangle surround each cat face in the image:
|
||||
|
||||
```
|
||||
# loop over the cat faces and draw a rectangle surrounding each
|
||||
for (i, (x, y, w, h)) in enumerate(rects):
|
||||
cv2.rectangle(image, (x, y), (x + w, y + h), (0, 0, 255), 2)
|
||||
cv2.putText(image, "Cat #{}".format(i + 1), (x, y - 10),
|
||||
cv2.FONT_HERSHEY_SIMPLEX, 0.55, (0, 0, 255), 2)
|
||||
|
||||
# show the detected cat faces
|
||||
cv2.imshow("Cat Faces", image)
|
||||
cv2.waitKey(0)
|
||||
```
|
||||
|
||||
Given our bounding boxes (i.e., rects ), we loop over each of them individually on Line 25.
|
||||
|
||||
We then draw a rectangle surrounding each cat face on Line 26, while Lines 27 and 28 displays an integer, counting the number of cats in the image.
|
||||
|
||||
Finally, Lines 31 and 32 display the output image to our screen.
|
||||
|
||||
### Cat detection results
|
||||
|
||||
To test our OpenCV cat detector, be sure to download the source code to this tutorial using the “Downloads” section at the bottom of this post.
|
||||
|
||||
Then, after you have unzipped the archive, you should have the following three files/directories:
|
||||
|
||||
1. cat_detector.py : Our Python + OpenCV script used to detect cats in images.
|
||||
2. haarcascade_frontalcatface.xml : The cat detector Haar cascade.
|
||||
3. images : A directory of testing images that we’re going to apply the cat detector cascade to.
|
||||
|
||||
From there, execute the following command:
|
||||
|
||||
Detecting cats in images with OpenCVShell
|
||||
|
||||
```
|
||||
$ python cat_detector.py --image images/cat_01.jpg
|
||||
```
|
||||
|
||||
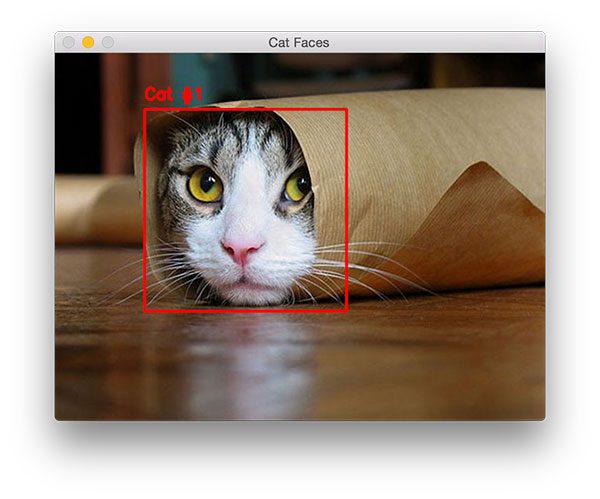
|
||||
>Figure 1: Detecting a cat face in an image, even with parts of the cat occluded
|
||||
|
||||
Notice that we have been able to detect the cat face in the image, even though the rest of its body is obscured.
|
||||
|
||||
Let’s try another image:
|
||||
|
||||
```
|
||||
python cat_detector.py --image images/cat_02.jpg
|
||||
```
|
||||
|
||||
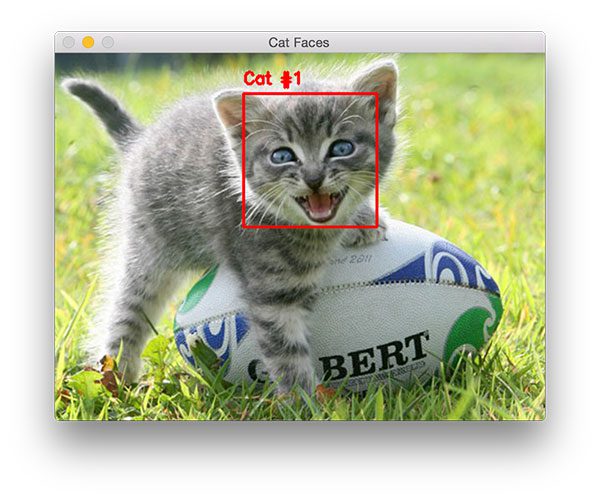
|
||||
>Figure 2: A second example of detecting a cat in an image with OpenCV, this time the cat face is slightly different
|
||||
|
||||
This cat’s face is clearly different from the other one, as it’s in the middle of a “meow”. In either case, the cat detector cascade is able to correctly find the cat face in the image.
|
||||
|
||||
The same is true for this image as well:
|
||||
|
||||
```
|
||||
$ python cat_detector.py --image images/cat_03.jpg
|
||||
```
|
||||
|
||||
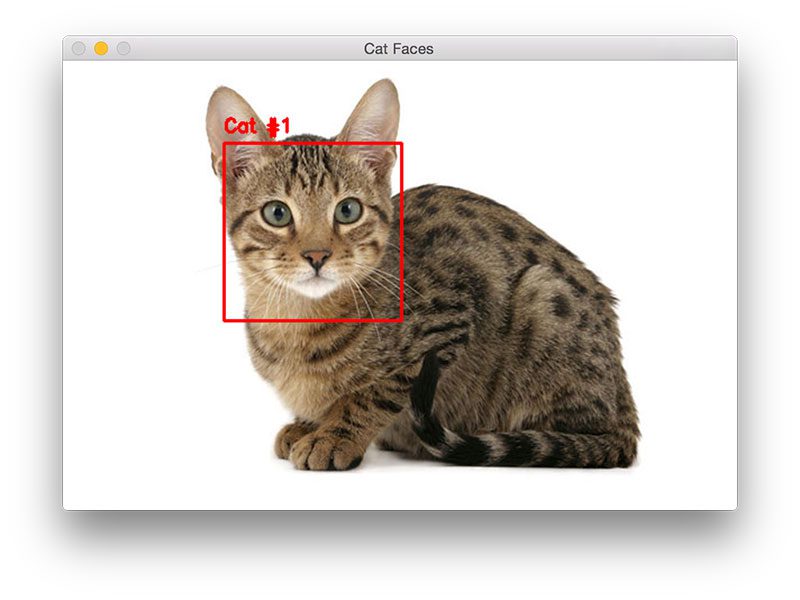
|
||||
>Figure 3: Cat detection with OpenCV and Python
|
||||
|
||||
Our final example demonstrates detecting multiple cats in an image using OpenCV and Python:
|
||||
|
||||
```
|
||||
$ python cat_detector.py --image images/cat_04.jpg
|
||||
```
|
||||
|
||||
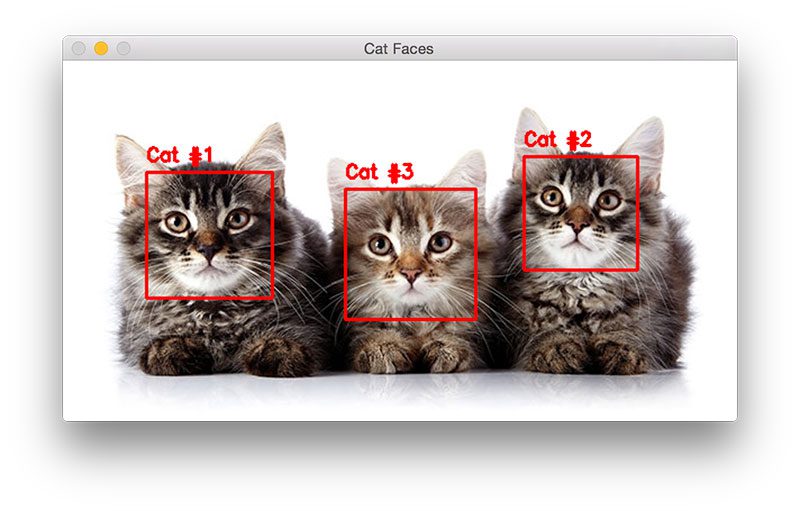
|
||||
>Figure 4: Detecting multiple cats in the same image with OpenCV
|
||||
|
||||
Note that the Haar cascade can return bounding boxes in an order that you may not like. In this case, the middle cat is actually labeled as the third cat. You can resolve this “issue” by sorting the bounding boxes according to their (x, y)-coordinates for a consistent ordering.
|
||||
|
||||
#### A quick note on accuracy
|
||||
|
||||
It’s important to note that in the comments section of the .xml files, Joseph Howe details that the cat detector Haar cascades can report cat faces where there are actually human faces.
|
||||
|
||||
In this case, he recommends performing both face detection and cat detection, then discarding any cat bounding boxes that overlap with the face bounding boxes.
|
||||
|
||||
#### A note on Haar cascades
|
||||
|
||||
First published in 2001 by Paul Viola and Michael Jones, [Rapid Object Detection using a Boosted Cascade of Simple Features][7], this original work has become one of the most cited papers in computer vision.
|
||||
|
||||
This algorithm is capable of detecting objects in images, regardless of their location and scale. And perhaps most intriguing, the detector can run in real-time on modern hardware.
|
||||
|
||||
In their paper, Viola and Jones focused on training a face detector; however, the framework can also be used to train detectors for arbitrary “objects”, such as cars, bananas, road signs, etc.
|
||||
|
||||
#### The problem?
|
||||
|
||||
The biggest problem with Haar cascades is getting the detectMultiScale parameters right, specifically scaleFactor and minNeighbors . You can easily run into situations where you need to tune both of these parameters on an image-by-image basis, which is far from ideal when utilizing an object detector.
|
||||
|
||||
The scaleFactor variable controls your [image pyramid][8] used to detect objects at various scales of an image. If your scaleFactor is too large, then you’ll only evaluate a few layers of the image pyramid, potentially leading to you missing objects at scales that fall in between the pyramid layers.
|
||||
|
||||
On the other hand, if you set scaleFactor too low, then you evaluate many pyramid layers. This will help you detect more objects in your image, but it (1) makes the detection process slower and (2) substantially increases the false-positive detection rate, something that Haar cascades are known for.
|
||||
|
||||
To remember this, we often apply [Histogram of Oriented Gradients + Linear SVM detection][9] instead.
|
||||
|
||||
The HOG + Linear SVM framework parameters are normally much easier to tune — and best of all, HOG + Linear SVM enjoys a much smaller false-positive detection rate. The only downside is that it’s harder to get HOG + Linear SVM to run in real-time.
|
||||
|
||||
### Interested in learning more about object detection?
|
||||
|
||||
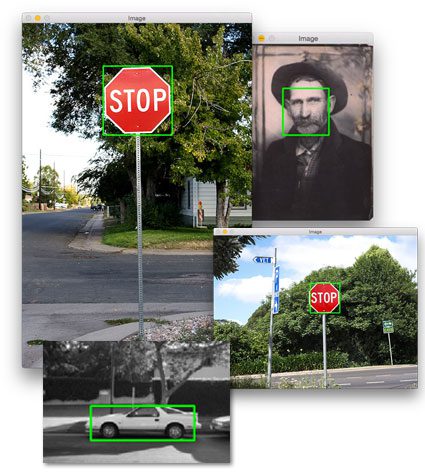
|
||||
>Figure 5: Learn how to build custom object detectors inside the PyImageSearch Gurus course.
|
||||
|
||||
If you’re interested in learning how to train your own custom object detectors, be sure to take a look at the PyImageSearch Gurus course.
|
||||
|
||||
Inside the course, I have 15 lessons covering 168 pages of tutorials dedicated to teaching you how to build custom object detectors from scratch. You’ll discover how to detect road signs, faces, cars (and nearly any other object) in images by applying the HOG + Linear SVM framework for object detection.
|
||||
|
||||
To learn more about the PyImageSearch Gurus course (and grab 10 FREE sample lessons), just click the button below:
|
||||
|
||||
### Summary
|
||||
|
||||
In this blog post, we learned how to detect cats in images using the default Haar cascades shipped with OpenCV. These Haar cascades were trained and contributed to the OpenCV project by [Joseph Howse][9], and were originally brought to my attention [in this post][10] by Kendrick Tan.
|
||||
|
||||
While Haar cascades are quite useful, we often use HOG + Linear SVM instead, as it’s a bit easier to tune the detector parameters, and more importantly, we can enjoy a much lower false-positive detection rate.
|
||||
|
||||
I detail how to build custom HOG + Linear SVM object detectors to recognize various objects in images, including cars, road signs, and much more [inside the PyImageSearch Gurus course][11].
|
||||
|
||||
Anyway, I hope you enjoyed this blog post!
|
||||
|
||||
Before you go, be sure to signup for the PyImageSearch Newsletter using the form below to be notified when new blog posts are published.
|
||||
|
||||
--------------------------------------------------------------------------------
|
||||
|
||||
via: http://www.pyimagesearch.com/2016/06/20/detecting-cats-in-images-with-opencv/
|
||||
|
||||
作者:[Adrian Rosebrock][a]
|
||||
译者:[译者ID](https://github.com/译者ID)
|
||||
校对:[校对者ID](https://github.com/校对者ID)
|
||||
|
||||
本文由 [LCTT](https://github.com/LCTT/TranslateProject) 原创编译,[Linux中国](https://linux.cn/) 荣誉推出
|
||||
|
||||
[a]: http://www.pyimagesearch.com/author/adrian/
|
||||
[1]: http://kendricktan.github.io/find-cats-in-photos-using-computer-vision.html
|
||||
[2]: http://www.pyimagesearch.com/2016/06/20/detecting-cats-in-images-with-opencv/#
|
||||
[3]: https://github.com/Itseez/opencv
|
||||
[4]: https://github.com/Itseez/opencv/tree/master/data/haarcascades
|
||||
[5]: http://nummist.com/
|
||||
[6]: http://www.pyimagesearch.com/2015/03/16/image-pyramids-with-python-and-opencv/
|
||||
[7]: https://www.cs.cmu.edu/~efros/courses/LBMV07/Papers/viola-cvpr-01.pdf
|
||||
[8]: http://www.pyimagesearch.com/2015/03/16/image-pyramids-with-python-and-opencv/
|
||||
[9]: http://www.pyimagesearch.com/2014/11/10/histogram-oriented-gradients-object-detection/
|
||||
[10]: http://kendricktan.github.io/find-cats-in-photos-using-computer-vision.html
|
||||
[11]: https://www.pyimagesearch.com/pyimagesearch-gurus/
|
||||
|
||||
|
||||
|
228
translated/tech/20160620 Detecting cats in images with OpenCV.md
Normal file
228
translated/tech/20160620 Detecting cats in images with OpenCV.md
Normal file
@ -0,0 +1,228 @@
|
||||
使用 OpenCV 识别图片中的猫
|
||||
=======================================
|
||||
|
||||
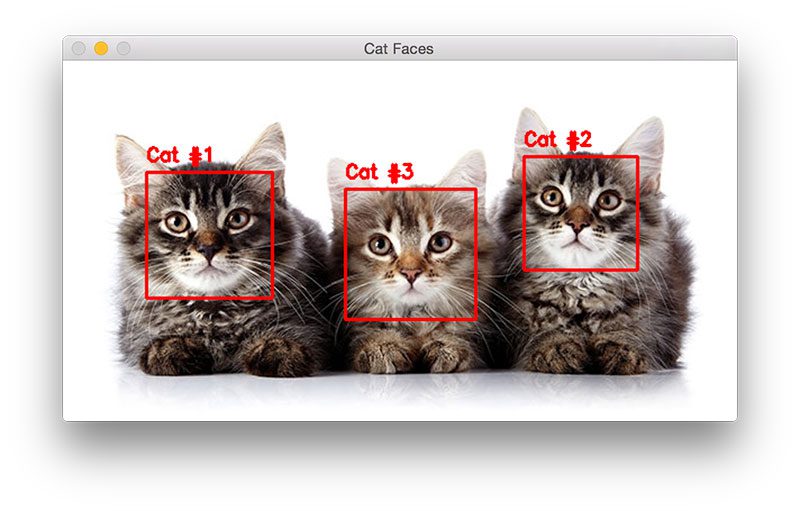
|
||||
|
||||
你知道 OpenCV 可以识别在图片中识别猫脸吗?还是在开箱即用的情况下,无需多余的附件。
|
||||
|
||||
我也不知道。
|
||||
|
||||
但是在看完'[Kendrick Tan broke the story][1]'这个故事之后, 我需要亲自体验一下...去看看到OpenCV 是如何在我没有察觉到的情况下,将这一个功能添加进了他的软件库。
|
||||
|
||||
作为这个博客的大纲,我将会展示如何使用 OpenCV 的猫检测器在图片中识别猫脸。同样的,你也可以在视频流中使用该技术。
|
||||
|
||||
> 想找这篇博客的源码?[请点这][2]。
|
||||
|
||||
|
||||
### 使用 OpenCV 在图片中检测猫
|
||||
|
||||
如果你看一眼[OpenCV 的代码库][3],尤其是在[haarcascades 目录][4](OpenCV 用来保存处理他对多种目标检测的Cascade预先训练的级联图像分类), 你将会注意到这两个文件:
|
||||
|
||||
- haarcascade_frontalcatface.xml
|
||||
- haarcascade_frontalcatface_extended.xml
|
||||
|
||||
这两个 Haar Cascade 文件都将被用来在图片中检测猫脸。实际上,我使用了相同的方式来生成这篇博客顶端的图片。
|
||||
|
||||
在做了一些调查工作之后,我发现训练这些记过并且将其提供给 OpenCV 仓库的是鼎鼎大名的 [Joseph Howse][5],他在计算机视觉领域有着很高的声望。
|
||||
|
||||
在博客的剩余部分,我将会展示给你如何使用 Howse 的 Haar 级联模型来检测猫。
|
||||
|
||||
让我们开工。新建一个叫 cat_detector.py 的文件,并且输入如下的代码:
|
||||
|
||||
### 使用 OpenCVPython 来检测猫
|
||||
|
||||
```
|
||||
# import the necessary packages
|
||||
import argparse
|
||||
import cv2
|
||||
|
||||
# construct the argument parse and parse the arguments
|
||||
ap = argparse.ArgumentParser()
|
||||
ap.add_argument("-i", "--image", required=True,
|
||||
help="path to the input image")
|
||||
ap.add_argument("-c", "--cascade",
|
||||
default="haarcascade_frontalcatface.xml",
|
||||
help="path to cat detector haar cascade")
|
||||
args = vars(ap.parse_args())
|
||||
```
|
||||
|
||||
第2和第3行主要是导入了必要的 python 包。6-12行主要是我们的命令行参数。我们在这只需要使用单独的参数'--image'。
|
||||
|
||||
我们可以指定一个 Haar cascade 的路径通过 `--cascade` 参数。默认使用 `haarcascades_frontalcatface.xml`,同时需要保证这个文件和你的 `cat_detector.py` 在同一目录下。
|
||||
|
||||
注意:我已经打包了猫的检测代码,还有在这个教程里的样本图片。你可以在博客的'Downloads' 部分下载到。如果你是刚刚接触 Python+OpenCV(或者 Haar 级联模型), 我会建议你下载 zip 压缩包,这个会方便你进行操作。
|
||||
|
||||
接下来,就是检测猫的时刻了:
|
||||
|
||||
```
|
||||
# load the input image and convert it to grayscale
|
||||
image = cv2.imread(args["image"])
|
||||
gray = cv2.cvtColor(image, cv2.COLOR_BGR2GRAY)
|
||||
|
||||
# load the cat detector Haar cascade, then detect cat faces
|
||||
# in the input image
|
||||
detector = cv2.CascadeClassifier(args["cascade"])
|
||||
rects = detector.detectMultiScale(gray, scaleFactor=1.3,
|
||||
minNeighbors=10, minSize=(75, 75))
|
||||
```
|
||||
|
||||
在15,16行,我们从硬盘上读取了图片,并且进行灰度化(一个常用的图片预处理,方便 Haar cascade 进行分类,尽管不是必须)
|
||||
|
||||
20行,我们加载了Haar casacade,即猫检测器,并且初始化了 cv2.CascadeClassifier 对象。
|
||||
|
||||
使用 OpenCV 检测猫脸的步骤是21,22行,通过调用 detectMultiScale 方法。我们使用四个参数来调用。包括:
|
||||
|
||||
1. 灰度化的图片,即样本图片。
|
||||
2. scaleFactor 参数,[图片金字塔][6]使用的检测猫脸时的检测粒度。一个更大的粒度将会加快检测的速度,但是会对准确性产生影响。相反的,一个更小的粒度将会影响检测的时间,但是会增加正确性。但是,细粒度也会增加错误的检测数量。你可以看博客的 'Haar 级联模型笔记' 部分来获得更多的信息。
|
||||
3. minNeighbors 参数控制了检测的最小数量,即在给定区域最小的检测猫脸的次数。这个参数很好的可以排除错误的检测结果。
|
||||
4. 最后,minSize 参数很好的自我说明了用途。即最后图片的最小大小,这个例子中就是 75\*75
|
||||
|
||||
detectMultiScale 函数 return rects,这是一个4维数组链表。这些item 中包含了猫脸的(x,y)坐标值,还有宽度,高度。
|
||||
|
||||
最后,让我们在图片上画下这些矩形来标识猫脸:
|
||||
|
||||
```
|
||||
# loop over the cat faces and draw a rectangle surrounding each
|
||||
for (i, (x, y, w, h)) in enumerate(rects):
|
||||
cv2.rectangle(image, (x, y), (x + w, y + h), (0, 0, 255), 2)
|
||||
cv2.putText(image, "Cat #{}".format(i + 1), (x, y - 10),
|
||||
cv2.FONT_HERSHEY_SIMPLEX, 0.55, (0, 0, 255), 2)
|
||||
|
||||
# show the detected cat faces
|
||||
cv2.imshow("Cat Faces", image)
|
||||
cv2.waitKey(0)
|
||||
```
|
||||
|
||||
给相关的区域(举个例子,rects),我们在25行依次历遍它。
|
||||
|
||||
在26行,我们在每张猫脸的周围画上一个矩形。27,28行展示了一个整数,即图片中猫的数量。
|
||||
|
||||
最后,31,32行在屏幕上展示了输出的图片。
|
||||
|
||||
### 猫检测结果
|
||||
|
||||
为了测试我们的 OpenCV 毛检测器,可以在文章的最后,下载教程的源码。
|
||||
|
||||
然后,在你解压缩之后,你将会得到如下的三个文件/目录:
|
||||
|
||||
1. cat_detector.py:我们的主程序
|
||||
2. haarcascade_frontalcatface.xml: Haar cascade 猫检测资源
|
||||
3. images:我们将会使用的检测图片目录。
|
||||
|
||||
到这一步,执行以下的命令:
|
||||
|
||||
使用 OpenCVShell 检测猫。
|
||||
|
||||
```
|
||||
$ python cat_detector.py --image images/cat_01.jpg
|
||||
```
|
||||
|
||||
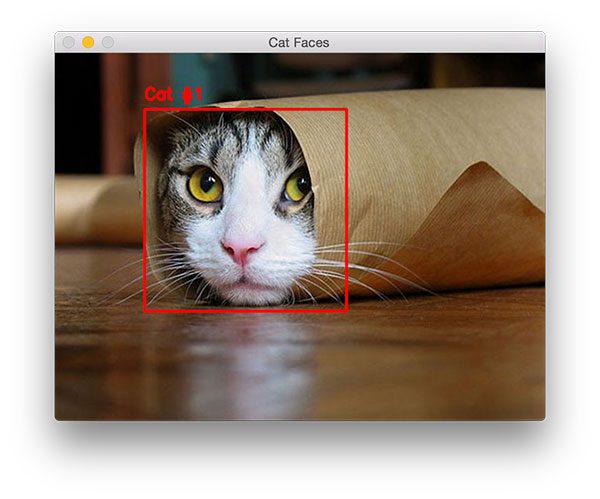
|
||||
>1. 在图片中检测猫脸,甚至是猫的一部分。
|
||||
|
||||
注意,我们已经可以检测猫脸了,即使他的其余部分是被隐藏的。
|
||||
|
||||
试下另外的一张图片:
|
||||
|
||||
```
|
||||
python cat_detector.py --image images/cat_02.jpg
|
||||
```
|
||||
|
||||
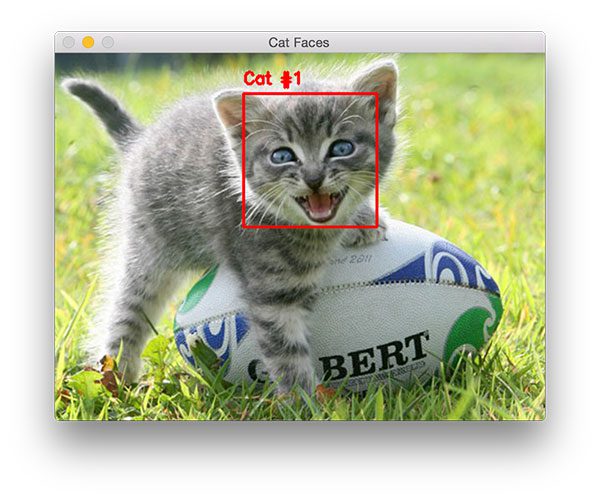
|
||||
>2. 第二个例子就是在略微不同的猫脸中检测。
|
||||
|
||||
这次的猫脸和第一次的明显不同,因为它在'Meow'的中央。这种情况下,我们依旧能检测到正确的猫脸。
|
||||
|
||||
这张图片的结果也是正确的:
|
||||
|
||||
```
|
||||
$ python cat_detector.py --image images/cat_03.jpg
|
||||
```
|
||||
|
||||
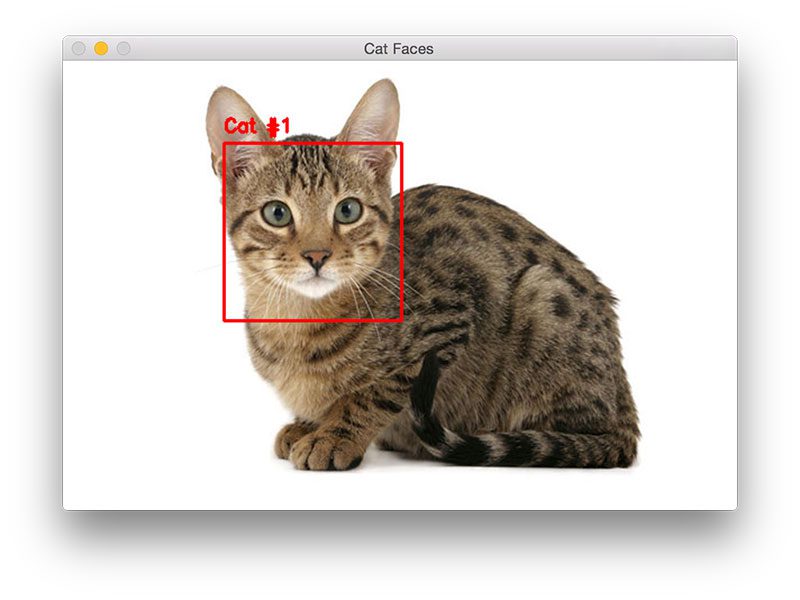
|
||||
>3. 使用 OpenCV 和 python 检测猫脸
|
||||
|
||||
我们最后的一个样例就是在一张图中检测多张猫脸:
|
||||
|
||||
```
|
||||
$ python cat_detector.py --image images/cat_04.jpg
|
||||
```
|
||||
|
||||
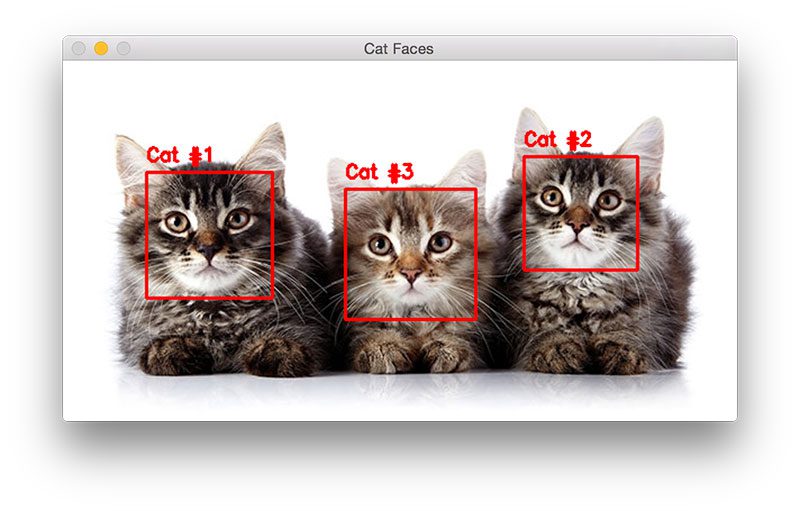
|
||||
>Figure 4: Detecting multiple cats in the same image with OpenCV
|
||||
>4. 在同一张图片中使用 OpenCV 检测多只猫
|
||||
|
||||
注意,Haar cascade 的返回值并不是有序的。这种情况下,中间的那只猫会被标记成第三只。你可以通过判断他们的(x, y)坐标来自己排序。
|
||||
|
||||
#### 精度的 Tips
|
||||
|
||||
xml 文件中的注释,非常重要,Joseph Hower 提到了猫 脸检测器有可能会将人脸识别成猫脸。
|
||||
|
||||
这种情况下,他推荐使用两种检测器(人脸&猫脸),然后将出现在人脸识别结果中的结果剔除掉。
|
||||
|
||||
#### Haar 级联模型注意事项
|
||||
|
||||
这个方法首先出现在 Paul Viola 和 Michael Jones 2001 年发布的 [Rapid Object Detection using a Boosted Cascade of Simple Features] 论文中。现在它已经成为了计算机识别领域引用最多的成果之一。
|
||||
|
||||
这个算法能够识别图片中的对象,无论地点,规模。并且,他也能在现有的硬件条件下实现实时计算。
|
||||
|
||||
在他们的论文中,Viola 和 Jones 关注在训练人脸检测器;但是,这个框架也能用来检测各类事物,如汽车,香蕉,路标等等。
|
||||
|
||||
#### 有问题?
|
||||
|
||||
Haar 级联模型最大的问题就是如何确定 detectMultiScale 方法的参数正确。特别是 scaleFactor 和 minNeighbors 参数。你很容易陷入,一张一张图片调参数的坑,这个就是该模型很难被实用化的原因。
|
||||
|
||||
这个 scaleFactor 变量控制了用来检测图片各种对象的[图像棱锥图][8]。如何参数过大,你就会得到更少的特征值,这会导致你无法在图层中识别一些目标。
|
||||
|
||||
换句话说,如果参数过低,你会检测出过多的图层。这虽然可以能帮助你检测更多的对象。但是他会造成计算速度的降低还会提高错误率。
|
||||
|
||||
为了避免这个,我们通常使用[Histogram of Oriented Gradients + Linear SVM detection][9]。
|
||||
|
||||
HOG + 线性 SVM 框架,它的参数更加容易的进行调优。而且也有更低的错误识别率,但是最大的缺点及时无法实时运算。
|
||||
|
||||
### 对对象识别感兴趣?并且希望了解更多?
|
||||
|
||||
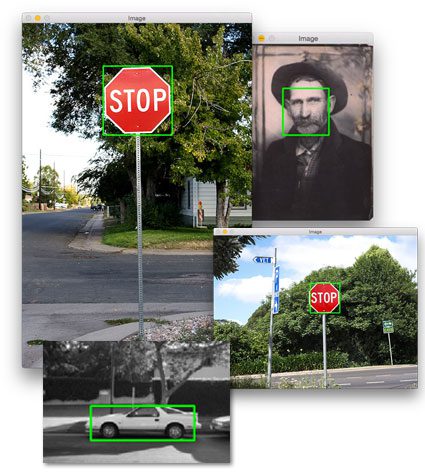
|
||||
>5. 在 PyImageSearch Gurus 课程中学习如何构建自定义的对象识别器。
|
||||
|
||||
如果你对学习如何训练自己的自定义对象识别器,请务必要去学习 PyImageSearch Gurus 的课程。
|
||||
|
||||
在这个课程中,我提供了15节课还有超过168页的教程,来教你如何从0开始构建自定义的对象识别器。你会掌握如何应用 HOG+线性 SVM 计算框架来构建自己的对象识别器。
|
||||
|
||||
### 总结
|
||||
|
||||
在这篇博客里,我们学习了如何使用默认的 Haar 级联模型来识别图片中的猫脸。这些 Haar casacades 是通过[Joseph Howse][9] 贡献给 OpenCV 项目的。我是在[这篇文章][10]中开始注意到这个。
|
||||
|
||||
尽管 Haar 级联模型相当有用,但是我们也经常用 HOG 和 线性 SVM 替代。因为后者相对而言更容易使用,并且可以有效地降低错误的识别概率。
|
||||
|
||||
我也会在[在 PyImageSearch Gurus 的课程中][11]详细的讲述如何使用 HOG 和线性 SVM 对象识别器,来识别包括汽车,路标在内的各种事物。
|
||||
|
||||
不管怎样,我希望你享受这篇博客。
|
||||
|
||||
在你离开之前,确保你会使用这下面的表单注册 PyImageSearch Newsletter。这样你能收到最新的消息。
|
||||
|
||||
--------------------------------------------------------------------------------
|
||||
|
||||
via: http://www.pyimagesearch.com/2016/06/20/detecting-cats-in-images-with-opencv/
|
||||
|
||||
作者:[Adrian Rosebrock][a]
|
||||
译者:[译者ID](https://github.com/MikeCoder)
|
||||
校对:[校对者ID](https://github.com/校对者ID)
|
||||
|
||||
本文由 [LCTT](https://github.com/LCTT/TranslateProject) 原创编译,[Linux中国](https://linux.cn/) 荣誉推出
|
||||
|
||||
[a]: http://www.pyimagesearch.com/author/adrian/
|
||||
[1]: http://kendricktan.github.io/find-cats-in-photos-using-computer-vision.html
|
||||
[2]: http://www.pyimagesearch.com/2016/06/20/detecting-cats-in-images-with-opencv/#
|
||||
[3]: https://github.com/Itseez/opencv
|
||||
[4]: https://github.com/Itseez/opencv/tree/master/data/haarcascades
|
||||
[5]: http://nummist.com/
|
||||
[6]: http://www.pyimagesearch.com/2015/03/16/image-pyramids-with-python-and-opencv/
|
||||
[7]: https://www.cs.cmu.edu/~efros/courses/LBMV07/Papers/viola-cvpr-01.pdf
|
||||
[8]: http://www.pyimagesearch.com/2015/03/16/image-pyramids-with-python-and-opencv/
|
||||
[9]: http://www.pyimagesearch.com/2014/11/10/histogram-oriented-gradients-object-detection/
|
||||
[10]: http://kendricktan.github.io/find-cats-in-photos-using-computer-vision.html
|
||||
[11]: https://www.pyimagesearch.com/pyimagesearch-gurus/
|
||||
|
||||
|
||||
|
Loading…
Reference in New Issue
Block a user