mirror of
https://github.com/LCTT/TranslateProject.git
synced 2025-01-25 23:11:02 +08:00
[translated]Faster machine learning is coming...
This commit is contained in:
parent
396d1e131f
commit
c5918898a3
@ -1,54 +0,0 @@
|
||||
alim0x translating
|
||||
|
||||
Faster machine learning is coming to the Linux kernel
|
||||
============================================================
|
||||
|
||||
### The addition of heterogenous memory management to the Linux kernel will unlock new ways to speed up GPUs, and potentially other kinds of machine learning hardware
|
||||
|
||||
|
||||
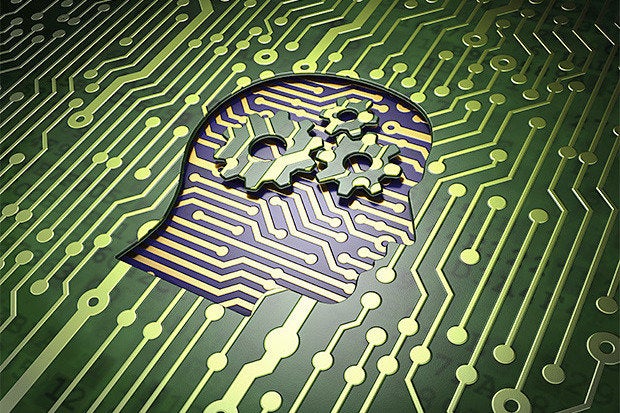
|
||||
>Credit: Thinkstock
|
||||
|
||||
It's been a long time in the works, but a memory management feature intended to give machine learning or other GPU-powered applications a major performance boost is close to making it into one of the next revisions of the kernel.
|
||||
|
||||
Heterogenous memory management (HMM) allows a device’s driver to mirror the address space for a process under its own memory management. As Red Hat developer Jérôme Glisse [explains][10], this makes it easier for hardware devices like GPUs to directly access the memory of a process without the extra overhead of copying anything. It also doesn't violate the memory protection features afforded by modern OSes.
|
||||
|
||||
|
||||
One class of application that stands to benefit most from HMM is GPU-based machine learning. Libraries like OpenCL and CUDA would be able to get a speed boost from HMM. HMM does this in much the same way as [speedups being done to GPU-based machine learning][11], namely by leaving data in place near the GPU, operating directly on it there, and moving it around as little as possible.
|
||||
|
||||
These kinds of speed-ups for CUDA, Nvidia’s library for GPU-based processing, would only benefit operations on Nvidia GPUs, but those GPUs currently constitute the vast majority of the hardware used to accelerate number crunching. However, OpenCL was devised to write code that could target multiple kinds of hardware—CPUs, GPUs, FPGAs, and so on—so HMM could provide much broader benefits as that hardware matures.
|
||||
|
||||
|
||||
There are a few obstacles to getting HMM into a usable state in Linux. First is kernel support, which has been under wraps for quite some time. HMM was first proposed as a Linux kernel patchset [back in 2014][12], with Red Hat and Nvidia both involved as key developers. The amount of work involved wasn’t trivial, but the developers believe code could be submitted for potential inclusion within the next couple of kernel releases.
|
||||
|
||||
The second obstacle is video driver support, which Nvidia has been working on separately. According to Glisse’s notes, AMD GPUs are likely to support HMM as well, so this particular optimization won’t be limited to Nvidia GPUs. AMD has been trying to ramp up its presence in the GPU market, potentially by [merging GPU and CPU processing][13] on the same die. However, the software ecosystem still plainly favors Nvidia; there would need to be a few more vendor-neutral projects like HMM, and OpenCL performance on a par with what CUDA can provide, to make real choice possible.
|
||||
|
||||
The third obstacle is hardware support, since HMM requires the presence of a replayable page faults hardware feature to work. Only Nvidia’s Pascal line of high-end GPUs supports this feature. In a way that’s good news, since it means Nvidia will only need to provide driver support for one piece of hardware—requiring less work on its part—to get HMM up and running.
|
||||
|
||||
Once HMM is in place, there will be pressure on public cloud providers with GPU instances to [support the latest-and-greatest generation of GPU][14]. Not just by swapping old-school Nvidia Kepler cards for bleeding-edge Pascal GPUs; as each succeeding generation of GPU pulls further away from the pack, support optimizations like HMM will provide strategic advantages.
|
||||
|
||||
--------------------------------------------------------------------------------
|
||||
|
||||
via: http://www.infoworld.com/article/3196884/linux/faster-machine-learning-is-coming-to-the-linux-kernel.html
|
||||
|
||||
作者:[Serdar Yegulalp][a]
|
||||
译者:[译者ID](https://github.com/译者ID)
|
||||
校对:[校对者ID](https://github.com/校对者ID)
|
||||
|
||||
本文由 [LCTT](https://github.com/LCTT/TranslateProject) 原创编译,[Linux中国](https://linux.cn/) 荣誉推出
|
||||
|
||||
[a]:http://www.infoworld.com/author/Serdar-Yegulalp/
|
||||
[1]:https://twitter.com/intent/tweet?url=http%3A%2F%2Fwww.infoworld.com%2Farticle%2F3196884%2Flinux%2Ffaster-machine-learning-is-coming-to-the-linux-kernel.html&via=infoworld&text=Faster+machine+learning+is+coming+to+the+Linux+kernel
|
||||
[2]:https://www.facebook.com/sharer/sharer.php?u=http%3A%2F%2Fwww.infoworld.com%2Farticle%2F3196884%2Flinux%2Ffaster-machine-learning-is-coming-to-the-linux-kernel.html
|
||||
[3]:http://www.linkedin.com/shareArticle?url=http%3A%2F%2Fwww.infoworld.com%2Farticle%2F3196884%2Flinux%2Ffaster-machine-learning-is-coming-to-the-linux-kernel.html&title=Faster+machine+learning+is+coming+to+the+Linux+kernel
|
||||
[4]:https://plus.google.com/share?url=http%3A%2F%2Fwww.infoworld.com%2Farticle%2F3196884%2Flinux%2Ffaster-machine-learning-is-coming-to-the-linux-kernel.html
|
||||
[5]:http://reddit.com/submit?url=http%3A%2F%2Fwww.infoworld.com%2Farticle%2F3196884%2Flinux%2Ffaster-machine-learning-is-coming-to-the-linux-kernel.html&title=Faster+machine+learning+is+coming+to+the+Linux+kernel
|
||||
[6]:http://www.stumbleupon.com/submit?url=http%3A%2F%2Fwww.infoworld.com%2Farticle%2F3196884%2Flinux%2Ffaster-machine-learning-is-coming-to-the-linux-kernel.html
|
||||
[7]:http://www.infoworld.com/article/3196884/linux/faster-machine-learning-is-coming-to-the-linux-kernel.html#email
|
||||
[8]:http://www.infoworld.com/article/3152565/linux/5-rock-solid-linux-distros-for-developers.html#tk.ifw-infsb
|
||||
[9]:http://www.infoworld.com/newsletters/signup.html#tk.ifw-infsb
|
||||
[10]:https://lkml.org/lkml/2017/4/21/872
|
||||
[11]:http://www.infoworld.com/article/3195437/machine-learning-analytics-get-a-boost-from-gpu-data-frame-project.html
|
||||
[12]:https://lwn.net/Articles/597289/
|
||||
[13]:http://www.infoworld.com/article/3099204/hardware/amd-mulls-a-cpugpu-super-chip-in-a-server-reboot.html
|
||||
[14]:http://www.infoworld.com/article/3126076/artificial-intelligence/aws-machine-learning-vms-go-faster-but-not-forward.html
|
@ -0,0 +1,52 @@
|
||||
更快的机器学习即将来到 Linux 内核
|
||||
============================================================
|
||||
|
||||
### Linux 内核新增的异构内存管理将解锁加速 GPU 的新途径,并挖掘其它机器学习硬件的潜能
|
||||
|
||||
|
||||
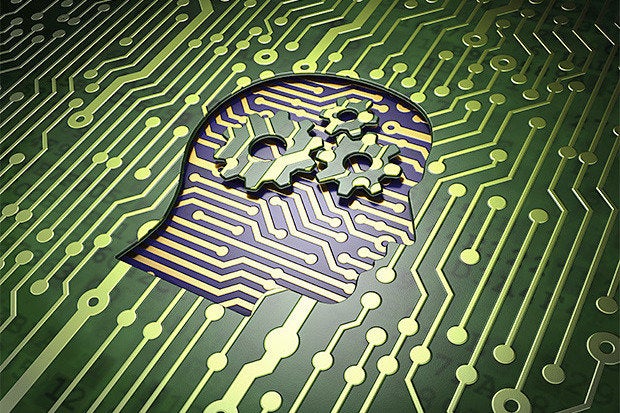
|
||||
>Credit: Thinkstock
|
||||
|
||||
一项开发了很久的内存管理技术将会给机器学习和其它 GPU 驱动的程序很大幅度的提升,而它也将在接下来的几个版本中进入 Linux 内核。
|
||||
|
||||
异构内存管理(HMM)可以允许设备驱动为在其自身内存管理下的进程镜像地址空间。正如红帽的开发者 Jérôme Glisse [所解释的][10],这让像 GPU 这样的硬件设备可以直接访问进程内存,而不用花费复制带来的额外开销。它还不违反现代才做系统提供的内存保护功能。
|
||||
|
||||
|
||||
一类会从 HMM 中获益最多的应用是基于 GPU 的机器学习。像 OpenCL 和 CUDA 这样的库能够从 HMM 中获得速度的提升。HMM 实现这个的方式和[加速基于 GPU 的机器学习][11]相似,就是让数据留在原地,靠近 GPU,在那里直接操作数据,尽可能少地移动数据。
|
||||
|
||||
像这样的加速对于 CUDA(英伟达基于 GPU 的处理库)来说,只会有益于在英伟达 GPU 上的操作,这些 GPU 也是目前加速数据处理的主要硬件。但是,OpenCL 设计用来编写可以针对多种硬件的代码——CPU,GPU,FPGA等等——随着这些硬件的成熟,HMM 能够提供更加广泛的益处。
|
||||
|
||||
|
||||
要让 Linux 中的 HMM 处于可用状态还有一些阻碍。第一个是内核支持,在很长一段时间里都很不明了。[早在 2014][12]年,HMM 最初作为 Linux 内核补丁集提出,红帽和英伟达都是关键开发者。需要做的工作不少,但是开发者相信可以提交代码,也许接下来的几个内核版本就能把它包含进去。
|
||||
|
||||
第二个阻碍是显卡驱动支持,英伟达一直在自己单独做一些工作。据 Glisse 的说法,AMD 的 GPU 可能也会支持 HMM,所以这种特殊优化不会仅限于英伟达的 GPU。AMD 一直都在尝试提升它的 GPU 市场占有率,有可能会[将 GPU 和 CPU 整合][13]到同一模具。但是,软件生态系统依然更偏向英伟达;要让可以选择成为现实,还需要更多的像 HMM 这样的中立项目,以及让 OpenCL 提供和 CUDA 相当的性能。
|
||||
|
||||
第三个阻碍是硬件支持,因为 HMM 的工作需要一项称作可重现页面故障(replayable page faults)的硬件特性。只有英伟达的帕斯卡系列高端 GPU 才支持这项特性。从某些意义上来说这是个好消息,因为这意味着英伟达只需要提供单一硬件的驱动支持就能让 HMM 正常使用,工作量就少了。
|
||||
|
||||
一旦 HMM 到位,对于提供 GPU 实例的公有云提供商就会面临压力,他们需要[支持最新最好一代的 GPU][14]。这并不是仅仅将老款的开普勒架构显卡换成最新的帕斯卡架构显卡就行了,因为后续的每一代显卡都会更加优秀,像 HMM 这样的支持优化将提供战略优势。
|
||||
|
||||
--------------------------------------------------------------------------------
|
||||
|
||||
via: http://www.infoworld.com/article/3196884/linux/faster-machine-learning-is-coming-to-the-linux-kernel.html
|
||||
|
||||
作者:[Serdar Yegulalp][a]
|
||||
译者:[alim0x](https://github.com/alim0x)
|
||||
校对:[校对者ID](https://github.com/校对者ID)
|
||||
|
||||
本文由 [LCTT](https://github.com/LCTT/TranslateProject) 原创编译,[Linux中国](https://linux.cn/) 荣誉推出
|
||||
|
||||
[a]:http://www.infoworld.com/author/Serdar-Yegulalp/
|
||||
[1]:https://twitter.com/intent/tweet?url=http%3A%2F%2Fwww.infoworld.com%2Farticle%2F3196884%2Flinux%2Ffaster-machine-learning-is-coming-to-the-linux-kernel.html&via=infoworld&text=Faster+machine+learning+is+coming+to+the+Linux+kernel
|
||||
[2]:https://www.facebook.com/sharer/sharer.php?u=http%3A%2F%2Fwww.infoworld.com%2Farticle%2F3196884%2Flinux%2Ffaster-machine-learning-is-coming-to-the-linux-kernel.html
|
||||
[3]:http://www.linkedin.com/shareArticle?url=http%3A%2F%2Fwww.infoworld.com%2Farticle%2F3196884%2Flinux%2Ffaster-machine-learning-is-coming-to-the-linux-kernel.html&title=Faster+machine+learning+is+coming+to+the+Linux+kernel
|
||||
[4]:https://plus.google.com/share?url=http%3A%2F%2Fwww.infoworld.com%2Farticle%2F3196884%2Flinux%2Ffaster-machine-learning-is-coming-to-the-linux-kernel.html
|
||||
[5]:http://reddit.com/submit?url=http%3A%2F%2Fwww.infoworld.com%2Farticle%2F3196884%2Flinux%2Ffaster-machine-learning-is-coming-to-the-linux-kernel.html&title=Faster+machine+learning+is+coming+to+the+Linux+kernel
|
||||
[6]:http://www.stumbleupon.com/submit?url=http%3A%2F%2Fwww.infoworld.com%2Farticle%2F3196884%2Flinux%2Ffaster-machine-learning-is-coming-to-the-linux-kernel.html
|
||||
[7]:http://www.infoworld.com/article/3196884/linux/faster-machine-learning-is-coming-to-the-linux-kernel.html#email
|
||||
[8]:http://www.infoworld.com/article/3152565/linux/5-rock-solid-linux-distros-for-developers.html#tk.ifw-infsb
|
||||
[9]:http://www.infoworld.com/newsletters/signup.html#tk.ifw-infsb
|
||||
[10]:https://lkml.org/lkml/2017/4/21/872
|
||||
[11]:http://www.infoworld.com/article/3195437/machine-learning-analytics-get-a-boost-from-gpu-data-frame-project.html
|
||||
[12]:https://lwn.net/Articles/597289/
|
||||
[13]:http://www.infoworld.com/article/3099204/hardware/amd-mulls-a-cpugpu-super-chip-in-a-server-reboot.html
|
||||
[14]:http://www.infoworld.com/article/3126076/artificial-intelligence/aws-machine-learning-vms-go-faster-but-not-forward.html
|
Loading…
Reference in New Issue
Block a user