mirror of
https://github.com/LCTT/TranslateProject.git
synced 2025-01-25 23:11:02 +08:00
翻译完成
对 [General purpose] 一词没有把握。
This commit is contained in:
parent
6eaa9fd46d
commit
c0c483068e
@ -1,51 +0,0 @@
|
||||
martin
|
||||
|
||||
What we learned in Seoul with AlphaGo
|
||||
================================================================================
|
||||
Go isn't just a game—it's a living, breathing culture of players, analysts, fans, and legends. Over the last 10 days in Seoul, South Korea, we've been lucky enough to witness some of that incredible excitement firsthand. We've also had the chance to see something that's never happened before: [DeepMind's][1] AlphaGo took on and defeated legendary Go player, Lee Sedol (9-dan professional with 18 world titles), marking a major milestone for artificial intelligence.
|
||||
|
||||
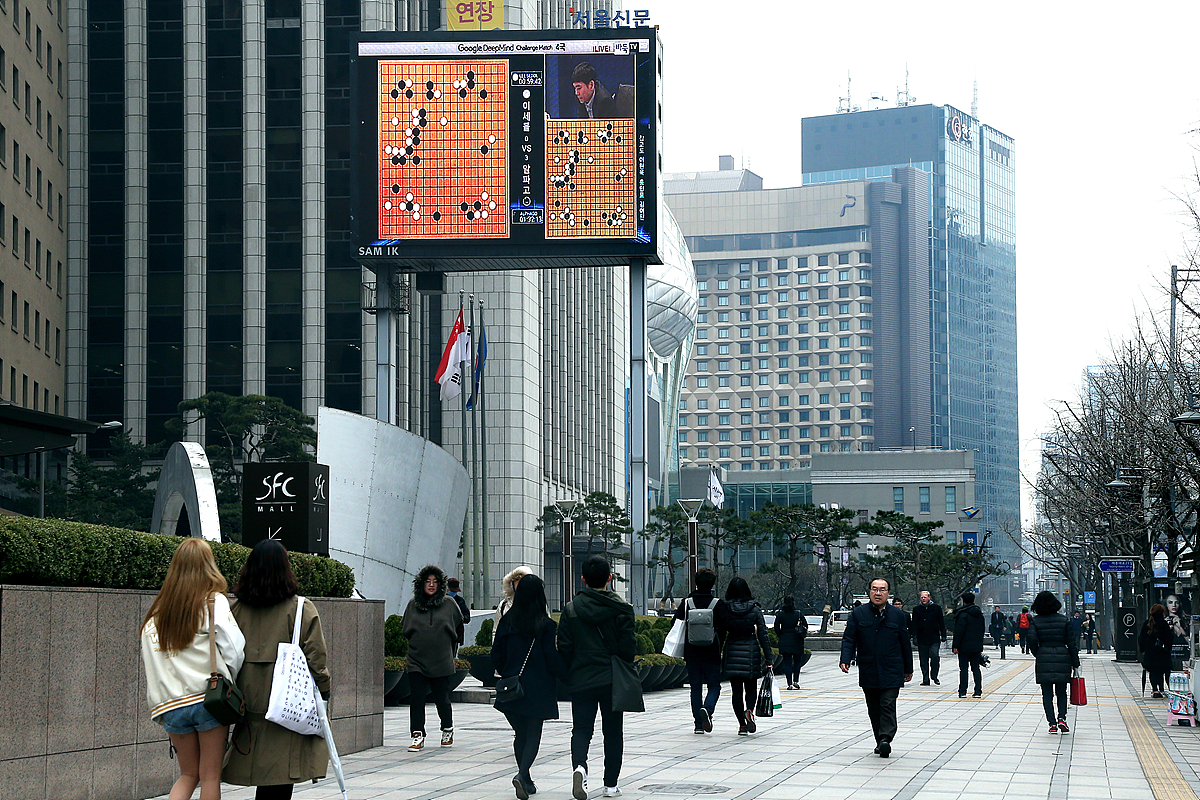
|
||||
|
||||
Go may be one of the oldest games in existence, but the attention to our five-game tournament exceeded even our wildest imaginations. Searches for Go rules and Go boards spiked in the U.S. In China, tens of millions watched live streams of the matches, and the “Man vs. Machine Go Showdown” hashtag saw 200 million pageviews on Sina Weibo. Sales of Go boards even [surged][2] in Korea.
|
||||
|
||||
Our public test of AlphaGo, however, was about more than winning at Go. We founded DeepMind in 2010 to create general-purpose artificial intelligence (AI) that can learn on its own—and, eventually, be used as a tool to help society solve some of its biggest and most pressing problems, from climate change to disease diagnosis.
|
||||
|
||||
Like many researchers before us, we've been developing and testing our algorithms through games. We first revealed [AlphaGo][3] in January—the first AI program that could beat a professional player at the most complex board game mankind has devised, using [deep learning][4] and [reinforcement learning][5]. The [ultimate challenge][6] was for AlphaGo to take on the best Go player of the past decade—Lee Sedol.
|
||||
|
||||
To everyone's surprise, including ours, AlphaGo won four of the five games. Commentators noted that AlphaGo played many unprecedented, creative, and even [“beautiful”][7] moves. Based on our data, AlphaGo's bold [move 37][8] in Game 2 had a 1 in 10,000 chance of being played by a human. Lee countered with innovative moves of his own, such as his [move 78][9] against AlphaGo in Game 4—again, a 1 in 10,000 chance of being played—which ultimately resulted in a win.
|
||||
|
||||
The final score was 4-1. We're contributing the $1 million in prize money to organizations that support science, technology, engineering and math (STEM) education and Go, as well as UNICEF.
|
||||
|
||||
We've learned two important things from this experience. First, this test bodes well for AI's potential in solving other problems. AlphaGo has the ability to look “globally” across a board—and find solutions that humans either have been trained not to play or would not consider. This has huge potential for using AlphaGo-like technology to find solutions that humans don't necessarily see in other areas. Second, while the match has been widely billed as "man vs. machine," AlphaGo is really a human achievement. Lee Sedol and the AlphaGo team both pushed each other toward new ideas, opportunities and solutions—and in the long run that's something we all stand to benefit from.
|
||||
|
||||
But as they say about Go in Korean: “Don't be arrogant when you win or you'll lose your luck.” This is just one small, albeit significant, step along the way to making machines smart. We've demonstrated that our cutting edge deep reinforcement learning techniques can be used to make strong Go and [Atari][10] players. Deep neural networks are already used at Google for specific tasks—like [image recognition][11], [speech recognition][12], and [Search ranking][13]. However, we're still a long way from a machine that can learn to flexibly perform the full range of intellectual tasks a human can—the hallmark of true [artificial general intelligence][14].
|
||||
|
||||
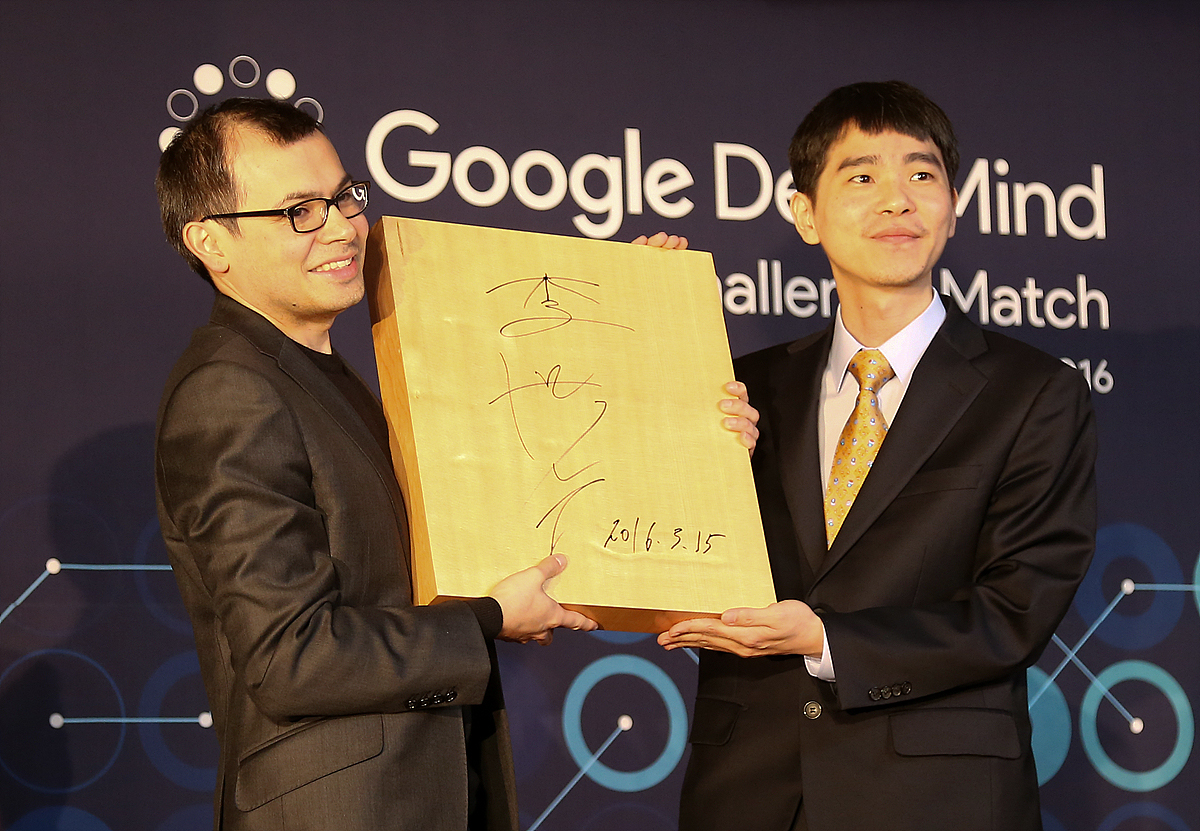
|
||||
|
||||
With this tournament, we wanted to test the limits of AlphaGo. The genius of Lee Sedol did that brilliantly—and we'll spend the next few weeks studying the games he and AlphaGo played in detail. And because the machine learning methods we've used in AlphaGo are general purpose, we hope to apply some of these techniques to other challenges in the future. Game on!
|
||||
|
||||
--------------------------------------------------------------------------------
|
||||
|
||||
via: https://googleblog.blogspot.com/2016/03/what-we-learned-in-seoul-with-alphago.html
|
||||
|
||||
作者:[Demis Hassabis][a]
|
||||
译者:[译者ID](https://github.com/译者ID)
|
||||
校对:[校对者ID](https://github.com/校对者ID)
|
||||
|
||||
本文由 [LCTT](https://github.com/LCTT/TranslateProject) 原创编译,[Linux中国](https://linux.cn/) 荣誉推出
|
||||
|
||||
[a]:http://demishassabis.com/
|
||||
[1]:https://deepmind.com/
|
||||
[2]:http://www.hankookilbo.com/m/v/3e7deaa26a834f76929a1689ecd388ea
|
||||
[3]:https://googleblog.blogspot.com/2016/01/alphago-machine-learning-game-go.html
|
||||
[4]:https://en.wikipedia.org/wiki/Deep_learning
|
||||
[5]:https://en.wikipedia.org/wiki/Reinforcement_learning
|
||||
[6]:https://deepmind.com/alpha-go.html
|
||||
[7]:http://www.wired.com/2016/03/sadness-beauty-watching-googles-ai-play-go/
|
||||
[8]:https://youtu.be/l-GsfyVCBu0?t=1h17m50s
|
||||
[9]:https://youtu.be/yCALyQRN3hw?t=3h10m25s
|
||||
[10]:http://googleresearch.blogspot.sg/2015/02/from-pixels-to-actions-human-level.html
|
||||
[11]:http://googleresearch.blogspot.sg/2013/06/improving-photo-search-step-across.html
|
||||
[12]:http://googleresearch.blogspot.sg/2015/08/the-neural-networks-behind-google-voice.html
|
||||
[13]:http://www.bloomberg.com/news/articles/2015-10-26/google-turning-its-lucrative-web-search-over-to-ai-machines
|
||||
[14]:https://en.wikipedia.org/wiki/Artificial_general_intelligence
|
@ -0,0 +1,49 @@
|
||||
我们和 AlphaGo 在首尔学到了什么
|
||||
================================================================================
|
||||
围棋并不仅仅是一个游戏——她是一伙活生生的玩家们,分析家们,爱好者们以及传奇大师们。在过去的十天里,在韩国首尔,我们有幸亲眼目睹那份难以置信的激动。我们也有幸也目睹了那前所未有的场景:[DeepMind][1] 的 AlphaGo 迎战并战胜了传奇围棋大师,李世石(职业9段,身负 18 个世界头衔),这是人工智能的里程碑。
|
||||
|
||||
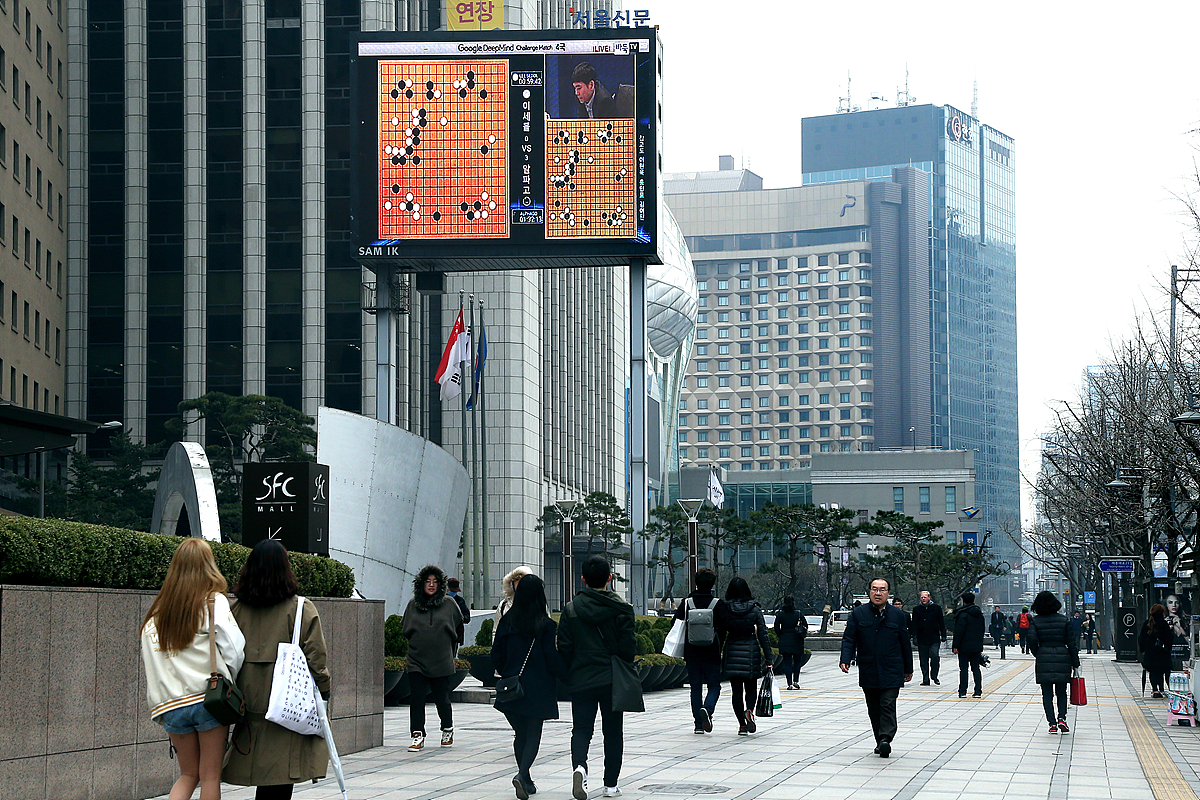
|
||||
|
||||
虽说围棋可能是存世的最为悠久的游戏之一了,但对于这五盘比赛的关注度还是大大的超出了我们的想象。搜索围棋规则和围棋盘的用户在美国迅速飙升。在中国,数以千万计的用户通过直播观看了这场比赛,并且新浪微博“人机围棋大战”话题的浏览量破 2 亿。韩国的围棋盘也销量[激增][2]。
|
||||
|
||||
然而我们如此公然的测试 AlphaGo,并不仅仅是为了赢棋而已。我们自 2010 年成立 DeepMind,为的是创造出具有独立学习能力的通用型人工智能(AI),并致力于将其作为工具协助解决,从气候变化到诊断疾病,这类最为棘手且急迫的问题为最终目标。
|
||||
|
||||
亦如许多前辈学者们一样,我们也是通过游戏来开发并测试我们的算法的。在一月份,我们第一次披露了 [AlphaGo][3]——作为第一个通过使用 [深度学习][4] 和 [强化学习][5],可以在人类发明的最为复杂的棋盘类游戏中击败职业选手的 AI 程序。而 AlphaGo 迎战过去十年间最厉害的围棋选手——李世石,绝对称得上是 [终极挑战][6]。
|
||||
|
||||
结果震惊了包括我们在内的每个人,AlphaGo 五战四胜。评论家指出了 AlphaGo 下出的许多前所未见、极富创意或者要用 [“漂亮”][7] 来形容的妙手。基于我们的数据分析,AlphaGo 在第 2 局中的 [37 手][8],在人类选手中出现的几率仅有万分之一。而李一反常态的创新下法,如第 4 局中的 [78 手][9]——也是既存下法中的万中之一的——这一手也最终造就了一场胜利。
|
||||
|
||||
最后比分定格在 4-1。我们为支持科学、技术、工程、数学(STEM)教育和围棋的组织,以及 UNICEF (联合国儿童基金会)赢得了 $1 百万的捐助。
|
||||
|
||||
经此一役,我们将收获总结成以下两点:第一,此次测试很好的预示了 AI 有解决其他问题的潜力。AlphaGo 在棋盘上能够做到兼顾“全局”——并找出人类已经被训化而不会走或想到的妙手。运用 AlphaGo 这类的技术,在人类目所不能及的领域中探索,会有很大的潜力。第二,虽说这场比赛已经被广泛的标榜成“人机大战”,但 AlphaGo 却是人类实实在在的成果。无论是李世石还是 AlphaGo 团队相互之间互相促进,产生了新的想法、观点和答案——并且长远来看,我们都将从中受益。
|
||||
|
||||
但正如韩国对于围棋的观点:“胜而不骄,是以常胜。”这只是使机器聪明的漫长道路中的一个小小而显著的一步而已。我们已经证明了尖端深度强化学习技术可以被用作制作强大的围棋选手和 [Atari][10] 玩家。深度神经网络在 Google 已经与 [图像识别][11],[语音识别][12] 以及 [搜索排名][13] 一样被应用到具体的任务中了。然而,从会学习的机器到可以像人一样全方位灵活实施智能任务——真正达到 [强人工智能][14] 的特性,此中的道路还很漫长。
|
||||
|
||||
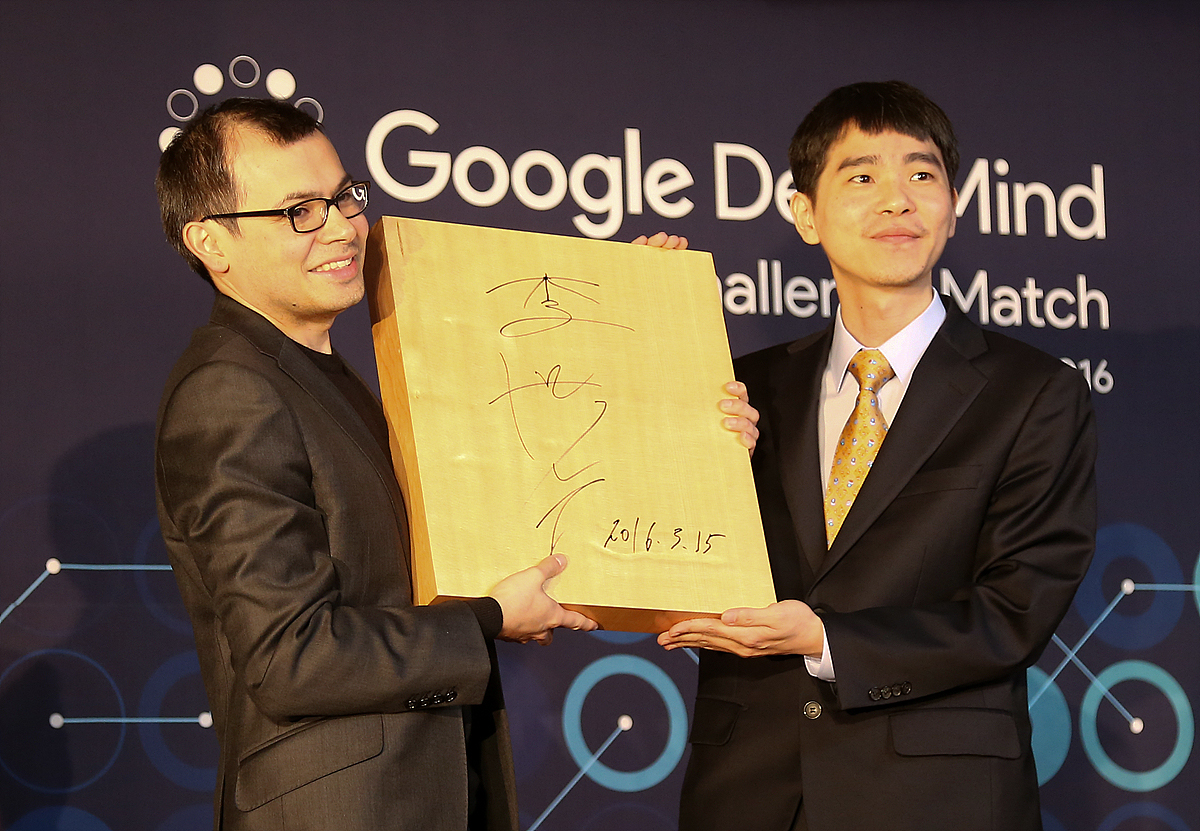
|
||||
|
||||
我们想通过这场比赛来测试 AlphaGo 的极限。李世石大师做的十分出色—我们在接下来的数周内会研究他与 AlphaGo 的对战细节。同时因为我们在 AlphaGo 中使用的机器学习方法是通用型的,我们十分希望在不久的将来,将这种技术应用于其他的挑战中。游戏开始!
|
||||
|
||||
--------------------------------------------------------------------------------
|
||||
|
||||
via: https://googleblog.blogspot.com/2016/03/what-we-learned-in-seoul-with-alphago.html
|
||||
|
||||
作者:[Demis Hassabis][a]
|
||||
译者:[martin2011qi](https://github.com/martin2011qi)
|
||||
校对:[校对者ID](https://github.com/校对者ID)
|
||||
|
||||
本文由 [LCTT](https://github.com/LCTT/TranslateProject) 原创编译,[Linux中国](https://linux.cn/) 荣誉推出
|
||||
|
||||
[a]:http://demishassabis.com/
|
||||
[1]:https://deepmind.com/
|
||||
[2]:http://www.hankookilbo.com/m/v/3e7deaa26a834f76929a1689ecd388ea
|
||||
[3]:https://googleblog.blogspot.com/2016/01/alphago-machine-learning-game-go.html
|
||||
[4]:https://en.wikipedia.org/wiki/Deep_learning
|
||||
[5]:https://en.wikipedia.org/wiki/Reinforcement_learning
|
||||
[6]:https://deepmind.com/alpha-go.html
|
||||
[7]:http://www.wired.com/2016/03/sadness-beauty-watching-googles-ai-play-go/
|
||||
[8]:https://youtu.be/l-GsfyVCBu0?t=1h17m50s
|
||||
[9]:https://youtu.be/yCALyQRN3hw?t=3h10m25s
|
||||
[10]:http://googleresearch.blogspot.sg/2015/02/from-pixels-to-actions-human-level.html
|
||||
[11]:http://googleresearch.blogspot.sg/2013/06/improving-photo-search-step-across.html
|
||||
[12]:http://googleresearch.blogspot.sg/2015/08/the-neural-networks-behind-google-voice.html
|
||||
[13]:http://www.bloomberg.com/news/articles/2015-10-26/google-turning-its-lucrative-web-search-over-to-ai-machines
|
||||
[14]:https://en.wikipedia.org/wiki/Artificial_general_intelligence
|
Loading…
Reference in New Issue
Block a user