mirror of
https://github.com/LCTT/TranslateProject.git
synced 2025-01-28 23:20:10 +08:00
Merge remote-tracking branch 'LCTT/master'
This commit is contained in:
commit
adf5741402
@ -1,513 +0,0 @@
|
||||
What every software engineer should know about search
|
||||
============================================================
|
||||
|
||||
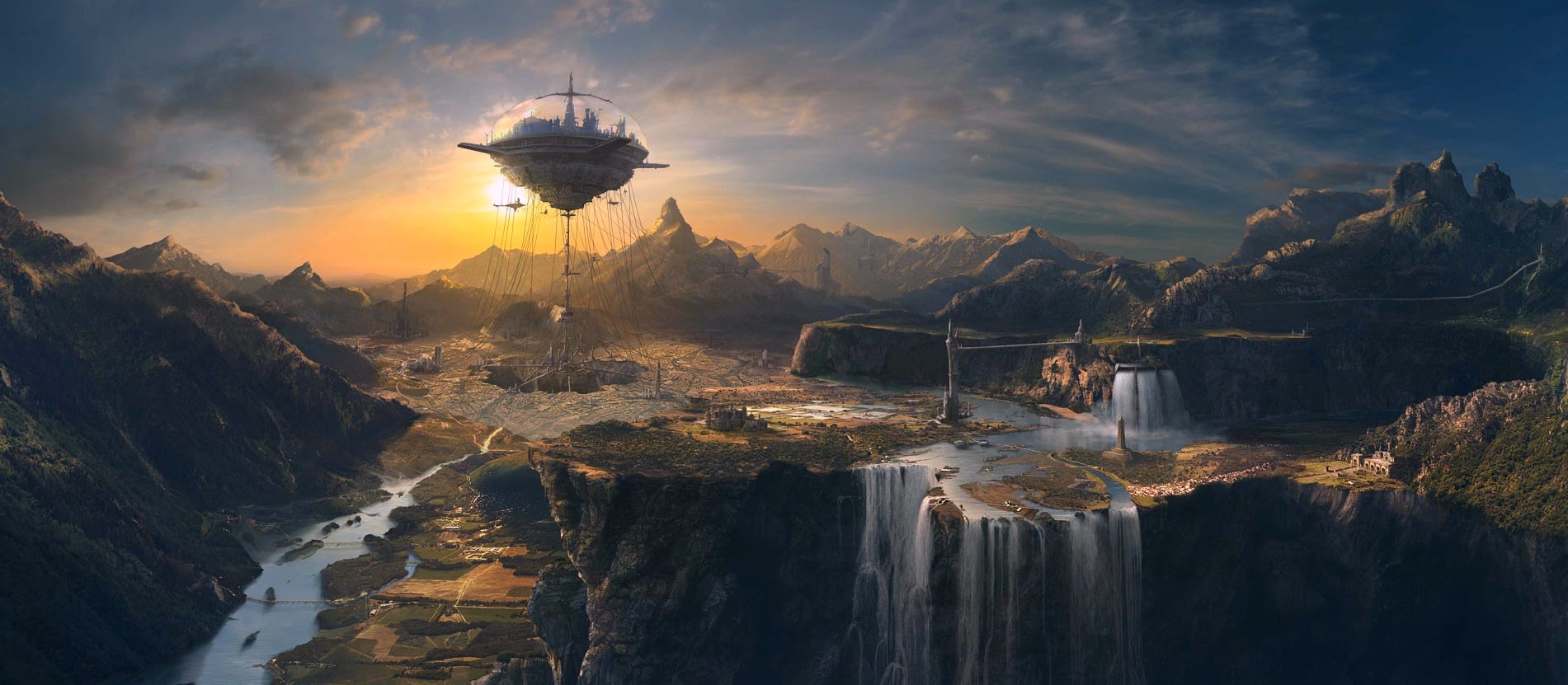
|
||||
|
||||
|
||||
### Want to build or improve a search experience? Start here.
|
||||
|
||||
Ask a software engineer: “[How would you add search functionality to your product?][78]” or “[How do I build a search engine?][79]” You’ll probably immediately hear back something like: “Oh, we’d just launch an ElasticSearch cluster. Search is easy these days.”
|
||||
|
||||
But is it? Numerous current products [still][80] [have][81] [suboptimal][82] [search][83] [experiences][84]. Any true search expert will tell you that few engineers have a very deep understanding of how search engines work, knowledge that’s often needed to improve search quality.
|
||||
|
||||
Even though many open source software packages exist, and the research is vast, the knowledge around building solid search experiences is limited to a select few. Ironically, [searching online][85] for search-related expertise doesn’t yield any recent, thoughtful overviews.
|
||||
|
||||
#### Emoji Legend
|
||||
|
||||
```
|
||||
❗ “Serious” gotcha: consequences of ignorance can be deadly
|
||||
🔷 Especially notable idea or piece of technology
|
||||
☁️ ️Cloud/SaaS
|
||||
🍺 Open source / free software
|
||||
🦏 JavaScript
|
||||
🐍 Python
|
||||
☕ Java
|
||||
🇨 C/C++
|
||||
```
|
||||
|
||||
### Why read this?
|
||||
|
||||
Think of this post as a collection of insights and resources that could help you to build search experiences. It can’t be a complete reference, of course, but hopefully we can improve it based on feedback (please comment or reach out!).
|
||||
|
||||
I’ll point at some of the most popular approaches, algorithms, techniques, and tools, based on my work on general purpose and niche search experiences of varying sizes at Google, Airbnb and several startups.
|
||||
|
||||
❗️Not appreciating or understanding the scope and complexity of search problems can lead to bad user experiences, wasted engineering effort, and product failure.
|
||||
|
||||
If you’re impatient or already know a lot of this, you might find it useful to jump ahead to the tools and services sections.
|
||||
|
||||
### Some philosophy
|
||||
|
||||
This is a long read. But most of what we cover has four underlying principles:
|
||||
|
||||
#### 🔷 Search is an inherently messy problem:
|
||||
|
||||
* Queries are highly variable. The search problems are highly variablebased on product needs.
|
||||
|
||||
* Think about how different Facebook search (searching a graph of people).
|
||||
|
||||
* YouTube search (searching individual videos).
|
||||
|
||||
* Or how different both of those are are from Kayak ([air travel planning is a really hairy problem][2]).
|
||||
|
||||
* Google Maps (making sense of geo-spacial data).
|
||||
|
||||
* Pinterest (pictures of a brunch you might cook one day).
|
||||
|
||||
#### Quality, metrics, and processes matter a lot:
|
||||
|
||||
* There is no magic bullet (like PageRank) nor a magic ranking formula that makes for a good approach. Processes are always evolving collection of techniques and processes that solve aspects of the problem and improve overall experience, usually gradually and continuously.
|
||||
|
||||
* ❗️In other words, search is not just just about building software that does ranking or retrieval (which we will discuss below) for a specific domain. Search systems are usually an evolving pipeline of components that are tuned and evolve over time and that build up to a cohesive experience.
|
||||
|
||||
* In particular, the key to success in search is building processes for evaluation and tuning into the product and development cycles. A search system architect should think about processes and metrics, not just technologies.
|
||||
|
||||
#### Use existing technologies first:
|
||||
|
||||
* As in most engineering problems, don’t reinvent the wheel yourself. When possible, use existing services or open source tools. If an existing SaaS (such as [Algolia][3] or managed Elasticsearch) fits your constraints and you can afford to pay for it, use it. This solution will likely will be the best choice for your product at first, even if down the road you need to customize, enhance, or replace it.
|
||||
|
||||
#### ❗️Even if you buy, know the details:
|
||||
|
||||
* Even if you are using an existing open source or commercial solution, you should have some sense of the complexity of the search problem and where there are likely to be pitfalls.
|
||||
|
||||
### Theory: the search problem
|
||||
|
||||
Search is different for every product, and choices depend on many technical details of the requirements. It helps to identify the key parameters of your search problem:
|
||||
|
||||
1. Size: How big is the corpus (a complete set of documents that need to be searched)? Is it thousands or billions of documents?
|
||||
|
||||
2. Media: Are you searching through text, images, graphical relationships, or geospatial data?
|
||||
|
||||
3. 🔷 Corpus control and quality: Are the sources for the documents under your control, or coming from a (potentially adversarial) third party? Are all the documents ready to be indexed or need to be cleaned up and selected?
|
||||
|
||||
4. Indexing speed: Do you need real-time indexing, or is building indices in batch is fine?
|
||||
|
||||
5. Query language: Are the queries structured, or you need to support unstructured ones?
|
||||
|
||||
6. Query structure: Are your queries textual, images, sounds? Street addresses, record ids, people’s faces?
|
||||
|
||||
7. Context-dependence: Do the results depend on who the user is, what is their history with the product, their geographical location, time of the day etc?
|
||||
|
||||
8. Suggest support: Do you need to support incomplete queries?
|
||||
|
||||
9. Latency: What are the serving latency requirements? 100 milliseconds or 100 seconds?
|
||||
|
||||
10. Access control: Is it entirely public or should users only see a restricted subset of the documents?
|
||||
|
||||
11. Compliance: Are there compliance or organizational limitations?
|
||||
|
||||
12. Internationalization: Do you need to support documents with multilingual character sets or Unicode? (Hint: Always use UTF-8 unless you really know what you’re doing.) Do you need to support a multilingual corpus? Multilingual queries?
|
||||
|
||||
Thinking through these points up front can help you make significant choices designing and building individual search system components.
|
||||
|
||||
** 此处有Canvas,请手动处理 **
|
||||
|
||||
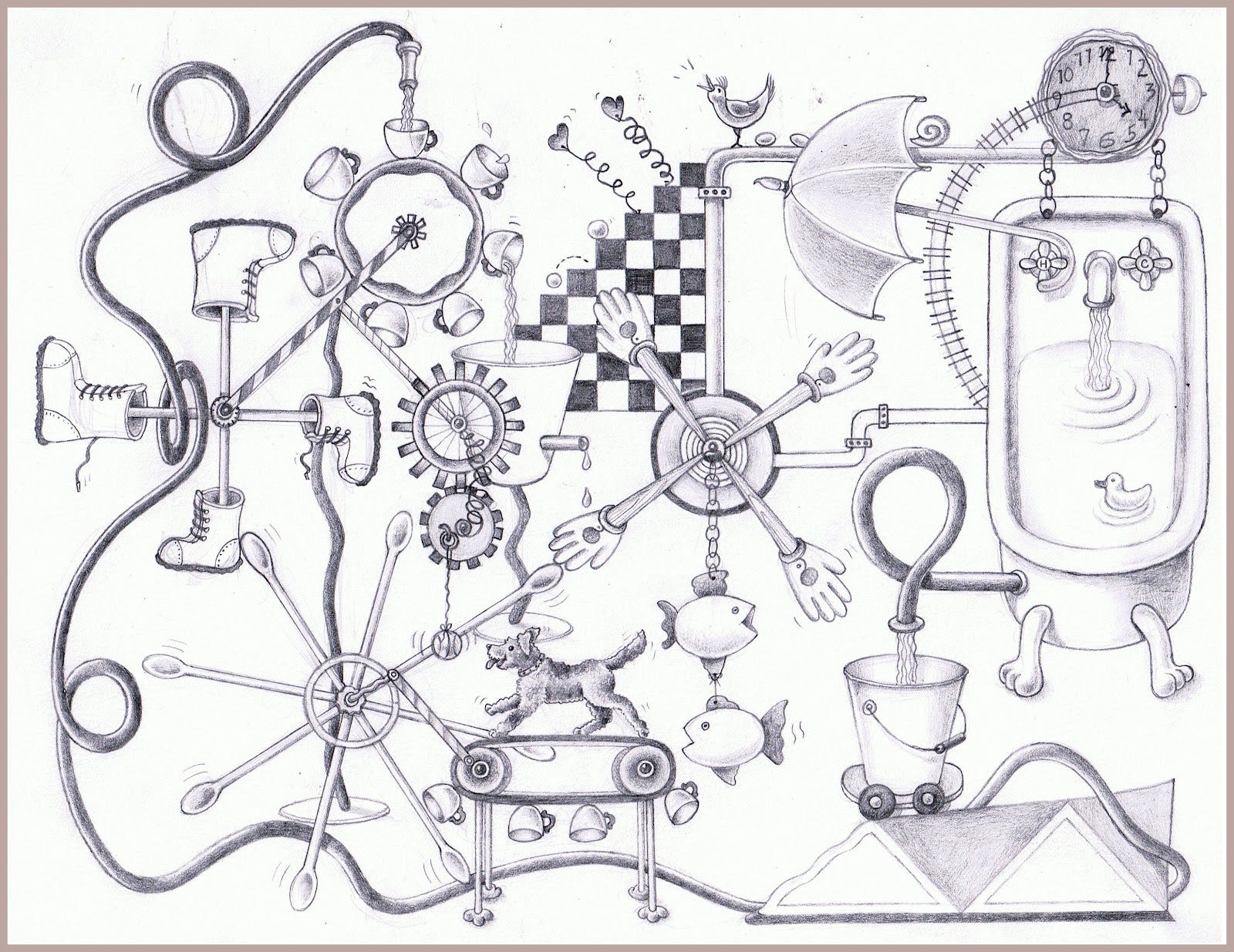
|
||||
A production indexing pipeline.
|
||||
|
||||
### Theory: the search pipeline
|
||||
|
||||
Now let’s go through a list of search sub-problems. These are usually solved by separate subsystems that form a pipeline. What that means is that a given subsystem consumes the output of previous subsystems, and produces input for the following subsystems.
|
||||
|
||||
This leads to an important property of the ecosystem: once you change how an upstream subsystem works, you need to evaluate the effect of the change and possibly change the behavior downstream.
|
||||
|
||||
Here are the most important problems you need to solve:
|
||||
|
||||
#### Index selection:
|
||||
|
||||
given a set of documents (e.g. the entirety of the Internet, all the Twitter posts, all the pictures on Instagram), select a potentially smaller subset of documents that may be worthy for consideration as search results and only include those in the index, discarding the rest. This is done to keep your indexes compact, and is almost orthogonal to selecting the documents to show to the user. Examples of particular classes of documents that don’t make the cut may include:
|
||||
|
||||
#### Spam:
|
||||
|
||||
oh, all the different shapes and sizes of search spam! A giant topic in itself, worthy of a separate guide. [A good web spam taxonomy overview][86].
|
||||
|
||||
#### Undesirable documents:
|
||||
|
||||
domain constraints might require filtering: [porn][87], illegal content, etc. The techniques are similar to spam filtering, probably with extra heuristics.
|
||||
|
||||
#### Duplicates:
|
||||
|
||||
Or near-duplicates and redundant documents. Can be done with [Locality-sensitive hashing][88], [similarity measures][89], clustering techniques or even [clickthrough data][90]. A [good overview][91] of techniques.
|
||||
|
||||
#### Low-utility documents:
|
||||
|
||||
The definition of utility depends highly on the problem domain, so it’s hard to recommend the approaches here. Some ideas are: it might be possible to build a utility function for your documents; heuristics might work, or example an image that contains only black pixels is not a useful document; utility might be learned from user behavior.
|
||||
|
||||
#### Index construction:
|
||||
|
||||
For most search systems, document retrieval is performed using an [inverted index][92] — often just called the index.
|
||||
|
||||
* The index is a mapping of search terms to documents. A search term could be a word, an image feature or any other document derivative useful for query-to-document matching. The list of the documents for a given term is called a [posting list][1]. It can be sorted by some metric, like document quality.
|
||||
|
||||
* Figure out whether you need to index the data in real time.❗️Many companies with large corpora of documents use a batch-oriented indexing approach, but then find this is unsuited to a product where users expect results to be current.
|
||||
|
||||
* With text documents, term extraction usually involves using NLP techniques, such as stop lists, [stemming][4] and [entity extraction][5]; for images or videos computer vision methods are used etc.
|
||||
|
||||
* In addition, documents are mined for statistical and meta information, such as references to other documents (used in the famous [PageRank][6]ranking signal), [topics][7], counts of term occurrences, document size, entities A mentioned etc. That information can be later used in ranking signal construction or document clustering. Some larger systems might contain several indexes, e.g. for documents of different types.
|
||||
|
||||
* Index formats. The actual structure and layout of the index is a complex topic, since it can be optimized in many ways. For instance there are [posting lists compression methods][8], one could target [mmap()able data representation][9] or use[ LSM-tree][10] for continuously updated index.
|
||||
|
||||
#### Query analysis and document retrieval:
|
||||
|
||||
Most popular search systems allow non-structured queries. That means the system has to extract structure out of the query itself. In the case of an inverted index, you need to extract search terms using [NLP][93] techniques.
|
||||
|
||||
The extracted terms can be used to retrieve relevant documents. Unfortunately, most queries are not very well formulated, so it pays to do additional query expansion and rewriting, like:
|
||||
|
||||
* [Term re-weighting][11].
|
||||
|
||||
* [Spell checking][12]. Historical query logs are very useful as a dictionary.
|
||||
|
||||
* [Synonym matching][13]. [Another survey][14].
|
||||
|
||||
* [Named entity recognition][15]. A good approach is to use [HMM-based language modeling][16].
|
||||
|
||||
* Query classification. Detect queries of particular type. For example, Google Search detects queries that contain a geographical entity, a porny query, or a query about something in the news. The retrieval algorithm can then make a decision about which corpora or indexes to look at.
|
||||
|
||||
* Expansion through [personalization][17] or [local context][18]. Useful for queries like “gas stations around me”.
|
||||
|
||||
#### Ranking:
|
||||
|
||||
Given a list of documents (retrieved in the previous step), their signals, and a processed query, create an optimal ordering (ranking) for those documents.
|
||||
|
||||
Originally, most ranking models in use were hand-tuned weighted combinations of all the document signals. Signal sets might include PageRank, clickthrough data, topicality information and [others][94].
|
||||
|
||||
To further complicate things, many of those signals, such as PageRank, or ones generated by [statistical language models][95] contain parameters that greatly affect the performance of a signal. Those have to be hand-tuned too.
|
||||
|
||||
Lately, 🔷 [learning to rank][96], signal-based discriminative supervised approaches are becoming more and more popular. Some popular examples of LtR are [McRank][97] and [LambdaRank][98] from Microsoft, and [MatrixNet][99] from Yandex.
|
||||
|
||||
A new, [vector space based approach][100] for semantic retrieval and ranking is gaining popularity lately. The idea is to learn individual low-dimensional vector document representations, then build a model which maps queries into the same vector space.
|
||||
|
||||
Then, retrieval is just finding several documents that are closest by some metric (e.g. Eucledian distance) to the query vector. Ranking is the distance itself. If the mapping of both the documents and queries is built well, the documents are chosen not by a fact of presence of some simple pattern (like a word), but how close the documents are to the query by _meaning_ .
|
||||
|
||||
### Indexing pipeline operation
|
||||
|
||||
Usually, each of the above pieces of the pipeline must be operated on a regular basis to keep the search index and search experience current.
|
||||
|
||||
❗️Operating a search pipeline can be complex and involve a lot of moving pieces. Not only is the data moving through the pipeline, but the code for each module and the formats and assumptions embedded in the data will change over time.
|
||||
|
||||
A pipeline can be run in “batch” or based on a regular or occasional basis (if indexing speed does not need to be real time) or in a streamed way (if real-time indexing is needed) or based on certain triggers.
|
||||
|
||||
Some complex search engines (like Google) have several layers of pipelines operating on different time scales — for example, a page that changes often (like [cnn.com][101]) is indexed with a higher frequency than a static page that hasn’t changed in years.
|
||||
|
||||
### Serving systems
|
||||
|
||||
Ultimately, the goal of a search system is to accept queries, and use the index to return appropriately ranked results. While this subject can be incredibly complex and technical, we mention a few of the key aspects to this part of the system.
|
||||
|
||||
* Performance: users notice when the system they interact with is laggy. ❗️Google has done [extensive research][19], and they have noticed that number of searches falls 0.6%, when serving is slowed by 300ms. They recommend to serve results under 200 ms for most of your queries. A good article [on the topic][20]. This is the hard part: the system needs to collect documents from, possibly, many computers, than merge them into possible a very long list and then sort that list in the ranking order. To complicate things further, ranking might be query-dependent, so, while sorting, the system is not just comparing 2 numbers, but performing computation.
|
||||
|
||||
* 🔷 Caching results: is often necessary to achieve decent performance. ❗️ But caches are just one large gotcha. The might show stale results when indices are updated or some results are blacklisted. Purging caches is a can of warm of itself: a search system might not have the capacity to serve the entire query stream with an empty (cold) cache, so the [cache needs to be pre-warmed][21] before the queries start arriving. Overall, caches complicate a system’s performance profile. Choosing a cache size and a replacement algorithm is also a [challenge][22].
|
||||
|
||||
* Availability: is often defined by an uptime/(uptime + downtime) metric. When index is distributed, in order to serve any search results, the system often needs to query all the shards for their share of results. ❗️That means, that if one shard is unavailable, the entire search system is compromised. The more machines are involved in serving the index — the higher the probability of one of them becoming defunct and bringing the whole system down.
|
||||
|
||||
* Managing multiple indices: Indices for large systems may separated into shards (pieces) or divided by media type or indexing cadence (fresh versus long-term indices). Results can then be merged.
|
||||
|
||||
* Merging results of different kinds: e.g. Google showing results from Maps, News etc.
|
||||
|
||||
** 此处有Canvas,请手动处理 **
|
||||
|
||||

|
||||
A human rater. Yeah, you should still have those.
|
||||
|
||||
### Quality, evaluation, and improvement
|
||||
|
||||
So you’ve launched your indexing pipeline and search servers, and it’s all running nicely. Unfortunately the road to a solid search experience only begins with running infrastructure.
|
||||
|
||||
Next, you’ll need to build a set of processes around continuous search quality evaluation and improvement. In fact, this is actually most of the work and the hardest problem you’ll have to solve.
|
||||
|
||||
🔷 What is quality? First, you’ll need to determine (and get your boss or the product lead to agree), what quality means in your case:
|
||||
|
||||
* Self-reported user satisfaction (includes UX)
|
||||
|
||||
* Perceived relevance of the returned results (not including UX)
|
||||
|
||||
* Satisfaction relative to competitors
|
||||
|
||||
* Satisfaction relative performance of the previous version of the search engine (e.g. last week)
|
||||
|
||||
* [User engagement][23]
|
||||
|
||||
Metrics: Some of these concepts can be quite hard to quantify. On the other hand, it’s incredibly useful to be able to express how well a search engine is performing in a single number, a quality metric.
|
||||
|
||||
Continuously computing such a metric for your (and your competitors’) system you can both track your progress and explain how well you are doing to your boss. Here are some classical ways to quantify quality, that can help you construct your magic quality metric formula:
|
||||
|
||||
* [Precision][24] and [recall][25] measure how well the retrieved set of documents corresponds to the set you expected to see.
|
||||
|
||||
* [F score][26] (specifically F1 score) is a single number, that represents both precision and recall well.
|
||||
|
||||
* [Mean Average Precision][27] (MAP) allows to quantify the relevance of the top returned results.
|
||||
|
||||
* 🔷 [Normalized Discounted Cumulative Gain][28] (nDCG) is like MAP, but weights the relevance of the result by its position.
|
||||
|
||||
* [Long and short clicks][29] — Allow to quantify how useful the results are to the real users.
|
||||
|
||||
* [A good detailed overview][30].
|
||||
|
||||
🔷 Human evaluations: Quality metrics might seem like statistical calculations, but they can’t all be done by automated calculations. Ultimately, metrics need to represent subjective human evaluation, and this is where a “human in the loop” comes into play.
|
||||
|
||||
❗️Skipping human evaluation is probably the most spread reason of sub-par search experiences.
|
||||
|
||||
Usually, at early stages the developers themselves evaluate the results manually. At later point [human raters][102] (or assessors) may get involved. Raters typically use custom tools to look at returned search results and provide feedback on the quality of the results.
|
||||
|
||||
Subsequently, you can use the feedback signals to guide development, help make launch decisions or even feed them back into the index selection, retrieval or ranking systems.
|
||||
|
||||
Here is the list of some other types of human-driven evaluation, that can be done on a search system:
|
||||
|
||||
* Basic user evaluation: The user ranks their satisfaction with the whole experience
|
||||
|
||||
* Comparative evaluation: Compare with other search results (compare with search results from earlier versions of the system or competitors)
|
||||
|
||||
* Retrieval evaluation: The query analysis and retrieval quality is often evaluated using manually constructed query-document sets. A user is shown a query and the list of the retrieved documents. She can then mark all the documents that are relevant to the query, and the ones that are not. The resulting pairs of (query, [relevant docs]) are called a “golden set”. Golden sets are remarkably useful. For one, an engineer can set up automatic retrieval regression tests using those sets. The selection signal from golden sets can also be fed back as ground truth to term re-weighting and other query re-writing models.
|
||||
|
||||
* Ranking evaluation: Raters are presented with a query and two documents side-by-side. The rater must choose the document that fits the query better. This creates a partial ordering on the documents for a given query. That ordering can be later be compared to the output of the ranking system. The usual ranking quality measures used are MAP and nDCG.
|
||||
|
||||
#### Evaluation datasets:
|
||||
|
||||
One should start thinking about the datasets used for evaluation (like “golden sets” mentioned above) early in the search experience design process. How you collect and update them? How you push them to the production eval pipeline? Is there a built-in bias?
|
||||
|
||||
Live experiments:
|
||||
|
||||
After your search engine catches on and gains enough users, you might want to start conducting [live search experiments][103] on a portion of your traffic. The basic idea is to turn some optimization on for a group of people, and then compare the outcome with that of a “control” group — a similar sample of your users that did not have the experiment feature on for them. How you would measure the outcome is, once again, very product specific: it could be clicks on results, clicks on ads etc.
|
||||
|
||||
Evaluation cycle time: How fast you improve your search quality is directly related to how fast you can complete the above cycle of measurement and improvement. It is essential from the beginning to ask yourself, “how fast can we measure and improve our performance?”
|
||||
|
||||
Will it take days, hours, minutes or seconds to make changes and see if they improve quality? ❗️Running evaluation should also be as easy as possible for the engineers and should not take too much hands-on time.
|
||||
|
||||
### 🔷 So… How do I PRACTICALLY build it?
|
||||
|
||||
This blogpost is not meant as a tutorial, but here is a brief outline of how I’d approach building a search experience right now:
|
||||
|
||||
1. As was said above, if you can afford it — just buy the existing SaaS (some good ones are listed below). An existing service fits if:
|
||||
|
||||
* Your experience is a “connected” one (your service or app has internet connection).
|
||||
|
||||
* Does it support all the functionality you need out of box? This post gives a pretty good idea of what functions would you want. To name a few, I’d at least consider: support for the media you are searching; real-time indexing support; query flexibility, including context-dependent queries.
|
||||
|
||||
* Given the size of the corpus and the expected [QpS][31], can you afford to pay for it for the next 12 months?
|
||||
|
||||
* Can the service support your expected traffic within the required latency limits? In case when you are querying the service from an app, make sure that the given service is accessible quickly enough from where your users are.
|
||||
|
||||
2\. If a hosted solution does not fit your needs or resources, you probably want to use one of the open source libraries or tools. In case of connected apps or websites, I’d choose ElasticSearch right now. For embedded experiences, there are multiple tools below.
|
||||
|
||||
3\. You most likely want to do index selection and clean up your documents (say extract relevant text from HTML pages) before uploading them to the search index. This will decrease the index size and make getting to good results easier. If your corpus fits on a single machine, just write a script (or several) to do that. If not, I’d use [Spark][104].
|
||||
|
||||
** 此处有Canvas,请手动处理 **
|
||||
|
||||
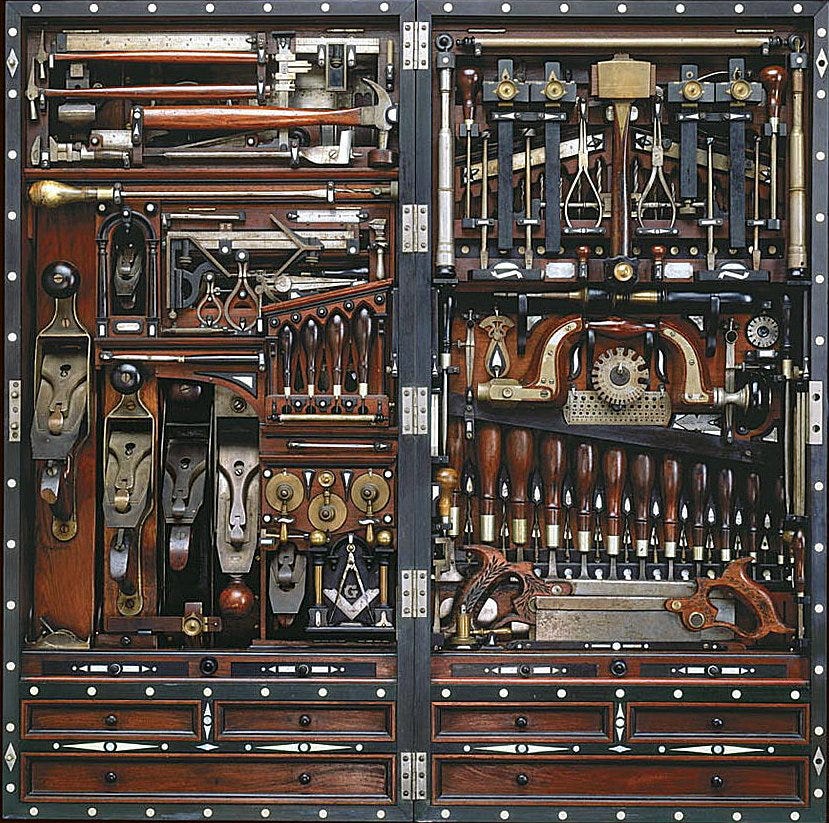
|
||||
You can never have too many tools.
|
||||
|
||||
### ☁️ SaaS
|
||||
|
||||
☁️ 🔷[Algolia][105] — a proprietary SaaS that indexes a client’s website and provides an API to search the website’s pages. They also have an API to submit your own documents, support context dependent searches and serve results really fast. If I were building a web search experience right now and could afford it, I’d probably use Algolia first — and buy myself time to build a comparable search experience.
|
||||
|
||||
* Various ElasticSearch providers: AWS (☁️ [ElasticSearch Cloud)][32], ☁️[elastic.co][33] and from ☁️ [Qbox][34].
|
||||
|
||||
* ☁️[ Azure Search][35] — a SaaS solution from Microsoft. Accessible through a REST API, it can scale to billions of documents. Has a Lucene query interface to simplify migrations from Lucene-based solutions.
|
||||
|
||||
* ☁️[ Swiftype][36] — an enterprise SaaS that indexes your company’s internal services, like Salesforce, G Suite, Dropbox and the intranet site.
|
||||
|
||||
### Tools and libraries
|
||||
|
||||
🍺☕🔷[ Lucene][106] is the most popular IR library. Implements query analysis, index retrieval and ranking. Either of the components can be replaced by an alternative implementation. There is also a C port — 🍺[Lucy][107].
|
||||
|
||||
* 🍺☕🔷[ Solr][37] is a complete search server, based on Lucene. It’s a part of the [Hadoop][38] ecosystem of tools.
|
||||
|
||||
* 🍺☕🔷[ Hadoop][39] is the most widely used open source MapReduce system, originally designed as a indexing pipeline framework for Solr. It has been gradually loosing ground to 🍺[Spark][40] as the batch data processing framework used for indexing. ☁️[EMR][41] is a proprietary implementation of MapReduce on AWS.
|
||||
|
||||
* 🍺☕🔷 [ElasticSearch][42] is also based on Lucene ([feature comparison with Solr][43]). It has been getting more attention lately, so much that a lot of people think of ES when they hear “search”, and for good reasons: it’s well supported, has [extensive API][44], [integrates with Hadoop][45] and [scales well][46]. There are open source and [Enterprise][47] versions. ES is also available as a SaaS on Can scale to billions of documents, but scaling to that point can be very challenging, so typical scenario would involve orders of magnitude smaller corpus.
|
||||
|
||||
* 🍺🇨 [Xapian][48] — a C++-based IR library. Relatively compact, so good for embedding into desktop or mobile applications.
|
||||
|
||||
* 🍺🇨 [Sphinx][49] — an full-text search server. Has a SQL-like query language. Can also act as a [storage engine for MySQL][50] or used as a library.
|
||||
|
||||
* 🍺☕ [Nutch][51] — a web crawler. Can be used in conjunction with Solr. It’s also the tool behind [🍺Common Crawl][52].
|
||||
|
||||
* 🍺🦏 [Lunr][53] — a compact embedded search library for web apps on the client-side.
|
||||
|
||||
* 🍺🦏 [searchkit][54] — a library of web UI components to use with ElasticSearch.
|
||||
|
||||
* 🍺🦏 [Norch][55] — a [LevelDB][56]-based search engine library for Node.js.
|
||||
|
||||
* 🍺🐍 [Whoosh][57] — a fast, full-featured search library implemented in pure Python.
|
||||
|
||||
* OpenStreetMaps has it’s own 🍺[deck of search software][58].
|
||||
|
||||
### Datasets
|
||||
|
||||
A few fun or useful data sets to try building a search engine or evaluating search engine quality:
|
||||
|
||||
* 🍺🔷 [Commoncrawl][59] — a regularly-updated open web crawl data. There is a [mirror on AWS][60], accessible for free within the service.
|
||||
|
||||
* 🍺🔷 [Openstreetmap data dump][61] is a very rich source of data for someone building a geospacial search engine.
|
||||
|
||||
* 🍺 [Google Books N-grams][62] can be very useful for building language models.
|
||||
|
||||
* 🍺 [Wikipedia dumps][63] are a classic source to build, among other things, an entity graph out of. There is a [wide range of helper tools][64] available.
|
||||
|
||||
* [IMDb dumps][65] are a fun dataset to build a small toy search engine for.
|
||||
|
||||
### References
|
||||
|
||||
* [Modern Information Retrieval][66] by R. Baeza-Yates and B. Ribeiro-Neto is a good, deep academic treatment of the subject. This is a good overview for someone completely new to the topic.
|
||||
|
||||
* [Information Retrieval][67] by S. Büttcher, C. Clarke and G. Cormack is another academic textbook with a wide coverage and is more up-to-date. Covers learn-to-rank and does a pretty good job at discussing theory of search systems evaluation. Also is a good overview.
|
||||
|
||||
* [Learning to Rank][68] by T-Y Liu is a best theoretical treatment of LtR. Pretty thin on practical aspects though. Someone considering building an LtR system should probably check this out.
|
||||
|
||||
* [Managing Gigabytes][69] — published in 1999, is still a definitive reference for anyone embarking on building an efficient index of a significant size.
|
||||
|
||||
* [Text Retrieval and Search Engines][70] — a MOOC from Coursera. A decent overview of basics.
|
||||
|
||||
* [Indexing the World Wide Web: The Journey So Far][71] ([PDF][72]), an overview of web search from 2012, by Ankit Jain and Abhishek Das of Google.
|
||||
|
||||
* [Why Writing Your Own Search Engine is Hard][73] a classic article from 2004 from Anna Patterson.
|
||||
|
||||
* [https://github.com/harpribot/awesome-information-retrieval][74] — a curated list of search-related resources.
|
||||
|
||||
* A [great blog][75] on everything search by [Daniel Tunkelang][76].
|
||||
|
||||
* Some good slides on [search engine evaluation][77].
|
||||
|
||||
This concludes my humble attempt to make a somewhat-useful “map” for an aspiring search engine engineer. Did I miss something important? I’m pretty sure I did — you know, [the margin is too narrow][108] to contain this enormous topic. Let me know if you think that something should be here and is not — you can reach [me][109] at[ forwidur@gmail.com][110] or at [@forwidur][111].
|
||||
|
||||
> P.S. — This post is part of a open, collaborative effort to build an online reference, the Open Guide to Practical AI, which we’ll release in draft form soon. See [this popular guide][112] for an example of what’s coming. If you’d like to get updates on or help with with this effort, sign up [here][113].
|
||||
|
||||
> Special thanks to [Joshua Levy][114], [Leo Polovets][115] and [Abhishek Das][116] for reading drafts of this and their invaluable feedback!
|
||||
|
||||
> Header image courtesy of [Mickaël Forrett][117]. The beautiful toolbox is called [The Studley Tool Chest][118].
|
||||
|
||||
--------------------------------------------------------------------------------
|
||||
|
||||
作者简介:
|
||||
|
||||
Max Grigorev
|
||||
distributed systems, data, AI
|
||||
|
||||
-------------
|
||||
|
||||
|
||||
via: https://medium.com/startup-grind/what-every-software-engineer-should-know-about-search-27d1df99f80d
|
||||
|
||||
作者:[Max Grigorev][a]
|
||||
译者:[译者ID](https://github.com/译者ID)
|
||||
校对:[校对者ID](https://github.com/校对者ID)
|
||||
|
||||
本文由 [LCTT](https://github.com/LCTT/TranslateProject) 原创编译,[Linux中国](https://linux.cn/) 荣誉推出
|
||||
|
||||
[a]:https://medium.com/@forwidur?source=post_header_lockup
|
||||
[1]:https://en.wikipedia.org/wiki/Inverted_index
|
||||
[2]:http://www.demarcken.org/carl/papers/ITA-software-travel-complexity/ITA-software-travel-complexity.pdf
|
||||
[3]:https://www.algolia.com/
|
||||
[4]:https://en.wikipedia.org/wiki/Stemming
|
||||
[5]:https://en.wikipedia.org/wiki/Named-entity_recognition
|
||||
[6]:http://ilpubs.stanford.edu:8090/422/1/1999-66.pdf
|
||||
[7]:https://gofishdigital.com/semantic-topic-modeling/
|
||||
[8]:https://nlp.stanford.edu/IR-book/html/htmledition/postings-file-compression-1.html
|
||||
[9]:https://deplinenoise.wordpress.com/2013/03/31/fast-mmapable-data-structures/
|
||||
[10]:https://en.wikipedia.org/wiki/Log-structured_merge-tree
|
||||
[11]:http://orion.lcg.ufrj.br/Dr.Dobbs/books/book5/chap11.htm
|
||||
[12]:http://norvig.com/spell-correct.html
|
||||
[13]:http://nlp.stanford.edu/IR-book/html/htmledition/query-expansion-1.html
|
||||
[14]:https://www.iro.umontreal.ca/~nie/IFT6255/carpineto-Survey-QE.pdf
|
||||
[15]:https://en.wikipedia.org/wiki/Named-entity_recognition
|
||||
[16]:http://www.aclweb.org/anthology/P02-1060
|
||||
[17]:https://en.wikipedia.org/wiki/Personalized_search
|
||||
[18]:http://searchengineland.com/future-search-engines-context-217550
|
||||
[19]:http://services.google.com/fh/files/blogs/google_delayexp.pdf
|
||||
[20]:http://highscalability.com/latency-everywhere-and-it-costs-you-sales-how-crush-it
|
||||
[21]:https://stackoverflow.com/questions/22756092/what-does-it-mean-by-cold-cache-and-warm-cache-concept
|
||||
[22]:https://en.wikipedia.org/wiki/Cache_performance_measurement_and_metric
|
||||
[23]:http://blog.popcornmetrics.com/5-user-engagement-metrics-for-growth/
|
||||
[24]:https://en.wikipedia.org/wiki/Information_retrieval#Precision
|
||||
[25]:https://en.wikipedia.org/wiki/Information_retrieval#Recall
|
||||
[26]:https://en.wikipedia.org/wiki/F1_score
|
||||
[27]:http://fastml.com/what-you-wanted-to-know-about-mean-average-precision/
|
||||
[28]:https://en.wikipedia.org/wiki/Discounted_cumulative_gain
|
||||
[29]:http://www.blindfiveyearold.com/short-clicks-versus-long-clicks
|
||||
[30]:https://arxiv.org/pdf/1302.2318.pdf
|
||||
[31]:https://en.wikipedia.org/wiki/Queries_per_second
|
||||
[32]:https://aws.amazon.com/elasticsearch-service/
|
||||
[33]:https://www.elastic.co/
|
||||
[34]:https://qbox.io/
|
||||
[35]:https://azure.microsoft.com/en-us/services/search/
|
||||
[36]:https://swiftype.com/
|
||||
[37]:http://lucene.apache.org/solr/
|
||||
[38]:http://hadoop.apache.org/
|
||||
[39]:http://hadoop.apache.org/
|
||||
[40]:http://spark.apache.org/
|
||||
[41]:https://aws.amazon.com/emr/
|
||||
[42]:https://www.elastic.co/products/elasticsearch
|
||||
[43]:http://solr-vs-elasticsearch.com/
|
||||
[44]:https://www.elastic.co/guide/en/elasticsearch/reference/current/docs.html
|
||||
[45]:https://github.com/elastic/elasticsearch-hadoop
|
||||
[46]:https://www.elastic.co/guide/en/elasticsearch/guide/current/distributed-cluster.html
|
||||
[47]:https://www.elastic.co/cloud/enterprise
|
||||
[48]:https://xapian.org/
|
||||
[49]:http://sphinxsearch.com/
|
||||
[50]:https://mariadb.com/kb/en/mariadb/sphinx-storage-engine/
|
||||
[51]:https://nutch.apache.org/
|
||||
[52]:http://commoncrawl.org/
|
||||
[53]:https://lunrjs.com/
|
||||
[54]:https://github.com/searchkit/searchkit
|
||||
[55]:https://github.com/fergiemcdowall/norch
|
||||
[56]:https://github.com/google/leveldb
|
||||
[57]:https://bitbucket.org/mchaput/whoosh/wiki/Home
|
||||
[58]:http://wiki.openstreetmap.org/wiki/Search_engines
|
||||
[59]:http://commoncrawl.org/
|
||||
[60]:https://aws.amazon.com/public-datasets/common-crawl/
|
||||
[61]:http://wiki.openstreetmap.org/wiki/Downloading_data
|
||||
[62]:http://commondatastorage.googleapis.com/books/syntactic-ngrams/index.html
|
||||
[63]:https://dumps.wikimedia.org/
|
||||
[64]:https://www.mediawiki.org/wiki/Alternative_parsers
|
||||
[65]:http://www.imdb.com/interfaces
|
||||
[66]:https://www.amazon.com/dp/0321416910
|
||||
[67]:https://www.amazon.com/dp/0262528878/
|
||||
[68]:https://www.amazon.com/dp/3642142664/
|
||||
[69]:https://www.amazon.com/dp/1558605703
|
||||
[70]:https://www.coursera.org/learn/text-retrieval
|
||||
[71]:https://research.google.com/pubs/pub37043.html
|
||||
[72]:https://pdfs.semanticscholar.org/28d8/288bff1b1fc693e6d80c238de9fe8b5e8160.pdf
|
||||
[73]:http://queue.acm.org/detail.cfm?id=988407
|
||||
[74]:https://github.com/harpribot/awesome-information-retrieval
|
||||
[75]:https://medium.com/@dtunkelang
|
||||
[76]:https://www.cs.cmu.edu/~quixote/
|
||||
[77]:https://web.stanford.edu/class/cs276/handouts/lecture8-evaluation_2014-one-per-page.pdf
|
||||
[78]:https://stackoverflow.com/questions/34314/how-do-i-implement-search-functionality-in-a-website
|
||||
[79]:https://www.quora.com/How-to-build-a-search-engine-from-scratch
|
||||
[80]:https://github.com/isaacs/github/issues/908
|
||||
[81]:https://www.reddit.com/r/Windows10/comments/4jbxgo/can_we_talk_about_how_bad_windows_10_search_sucks/d365mce/
|
||||
[82]:https://www.reddit.com/r/spotify/comments/2apwpd/the_search_function_sucks_let_me_explain/
|
||||
[83]:https://medium.com/@RohitPaulK/github-issues-suck-723a5b80a1a3#.yp8ui3g9i
|
||||
[84]:https://thenextweb.com/opinion/2016/01/11/netflix-search-sucks-flixed-fixes-it/
|
||||
[85]:https://www.google.com/search?q=building+a+search+engine
|
||||
[86]:http://airweb.cse.lehigh.edu/2005/gyongyi.pdf
|
||||
[87]:https://www.researchgate.net/profile/Gabriel_Sanchez-Perez/publication/262371199_Explicit_image_detection_using_YCbCr_space_color_model_as_skin_detection/links/549839cf0cf2519f5a1dd966.pdf
|
||||
[88]:https://en.wikipedia.org/wiki/Locality-sensitive_hashing
|
||||
[89]:https://en.wikipedia.org/wiki/Similarity_measure
|
||||
[90]:https://www.microsoft.com/en-us/research/wp-content/uploads/2011/02/RadlinskiBennettYilmaz_WSDM2011.pdf
|
||||
[91]:http://infolab.stanford.edu/~ullman/mmds/ch3.pdf
|
||||
[92]:https://en.wikipedia.org/wiki/Inverted_index
|
||||
[93]:https://en.wikipedia.org/wiki/Natural_language_processing
|
||||
[94]:http://backlinko.com/google-ranking-factors
|
||||
[95]:http://times.cs.uiuc.edu/czhai/pub/slmir-now.pdf
|
||||
[96]:https://en.wikipedia.org/wiki/Learning_to_rank
|
||||
[97]:https://papers.nips.cc/paper/3270-mcrank-learning-to-rank-using-multiple-classification-and-gradient-boosting.pdf
|
||||
[98]:https://www.microsoft.com/en-us/research/wp-content/uploads/2016/02/lambdarank.pdf
|
||||
[99]:https://yandex.com/company/technologies/matrixnet/
|
||||
[100]:https://arxiv.org/abs/1708.02702
|
||||
[101]:http://cnn.com/
|
||||
[102]:http://static.googleusercontent.com/media/www.google.com/en//insidesearch/howsearchworks/assets/searchqualityevaluatorguidelines.pdf
|
||||
[103]:https://googleblog.blogspot.co.uk/2008/08/search-experiments-large-and-small.html
|
||||
[104]:https://spark.apache.org/
|
||||
[105]:https://www.algolia.com/
|
||||
[106]:https://lucene.apache.org/
|
||||
[107]:https://lucy.apache.org/
|
||||
[108]:https://www.brainyquote.com/quotes/quotes/p/pierredefe204944.html
|
||||
[109]:https://www.linkedin.com/in/grigorev/
|
||||
[110]:mailto:forwidur@gmail.com
|
||||
[111]:https://twitter.com/forwidur
|
||||
[112]:https://github.com/open-guides/og-aws
|
||||
[113]:https://upscri.be/d29cfe/
|
||||
[114]:https://twitter.com/ojoshe
|
||||
[115]:https://twitter.com/lpolovets
|
||||
[116]:https://www.linkedin.com/in/abhishek-das-3280053/
|
||||
[117]:https://www.behance.net/gallery/3530289/-HORIZON-
|
||||
[118]:https://en.wikipedia.org/wiki/Henry_O._Studley
|
@ -1,153 +0,0 @@
|
||||
Anatomy of a perfect pull request
|
||||
======
|
||||
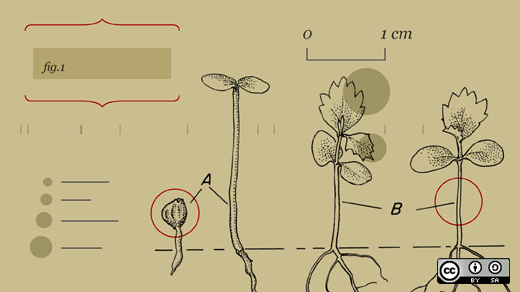
|
||||
|
||||
Writing clean code is just one of many factors you should care about when creating a pull request.
|
||||
|
||||
Large pull requests cause a big overhead during the code review and can facilitate bugs in the codebase.
|
||||
|
||||
That's why you need to care about the pull request itself. It should be short, have a clear title and description, and do only one thing.
|
||||
|
||||
### Why should you care?
|
||||
|
||||
* A good pull request will be reviewed quickly
|
||||
* It reduces bug introduction into codebase
|
||||
* It facilitates new developers onboarding
|
||||
* It does not block other developers
|
||||
* It speeds up the code review process and consequently, product development
|
||||
|
||||
|
||||
|
||||
### The size of the pull request
|
||||
|
||||
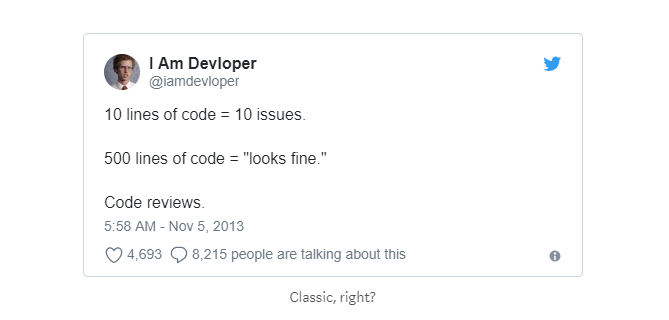
|
||||
|
||||
The first step to identifying problematic pull requests is to look for big diffs.
|
||||
|
||||
Several studies show that it is harder to find bugs when reviewing a lot of code.
|
||||
|
||||
In addition, large pull requests will block other developers who may be depending on the code.
|
||||
|
||||
#### How can we determine the perfect pull request size?
|
||||
|
||||
A [study of a Cisco Systems programming team][1] revealed that a review of 200-400 LOC over 60 to 90 minutes should yield 70-90% defect discovery.
|
||||
|
||||
With this number in mind, a good pull request should not have more than 250 lines of code changed.
|
||||
|
||||
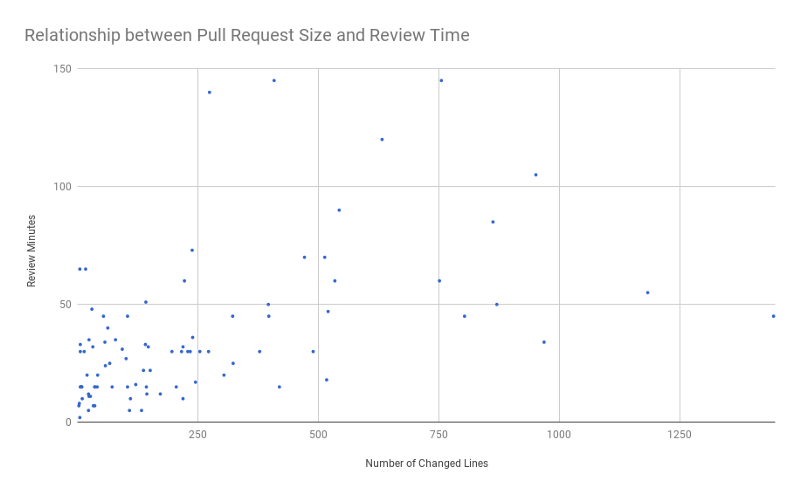
|
||||
|
||||
Image from [small business programming][2].
|
||||
|
||||
As shown in the chart above, pull requests with more than 250 lines of changes usually take more than one hour to review.
|
||||
|
||||
### Break down large pull requests into smaller ones
|
||||
|
||||
Feature breakdown is an art. The more you do it, the easier it gets.
|
||||
|
||||
What do I mean by feature breakdown?
|
||||
|
||||
Feature breakdown is understanding a big feature and breaking it into small pieces that make sense and that can be merged into the codebase piece by piece without breaking anything.
|
||||
|
||||
#### Learning by doing
|
||||
|
||||
Let’s say that you need to create a subscribe feature on your app. It's just a form that accepts an email address and saves it.
|
||||
|
||||
Without knowing how your app works, I can already break it into eight pull requests:
|
||||
|
||||
* Create a model to save emails
|
||||
* Create a route to receive requests
|
||||
* Create a controller
|
||||
* Create a service to save it in the database (business logic)
|
||||
* Create a policy to handle access control
|
||||
* Create a subscribe component (frontend)
|
||||
* Create a button to call the subscribe component
|
||||
* Add the subscribe button in the interface
|
||||
|
||||
|
||||
|
||||
As you can see, I broke this feature into many parts, most of which can be done simultaneously by different developers.
|
||||
|
||||
### Single responsibility principle
|
||||
|
||||
The single responsibility principle (SRP) is a computer programming principle that states that every [module][3] or [class][4] should have responsibility for a single part of the [functionality][5] provided by the [software][6], and that responsibility should be entirely [encapsulated][7] by the class.
|
||||
|
||||
Just like classes and modules, pull requests should do only one thing.
|
||||
|
||||
Following the SRP reduces the overhead caused by revising a code that attempts to solve several problems.
|
||||
|
||||
Before submitting a PR for review, try applying the single responsibility principle. If the code does more than one thing, break it into other pull requests.
|
||||
|
||||
### Title and description matter
|
||||
|
||||
When creating a pull request, you should care about the title and the description.
|
||||
|
||||
Imagine that the code reviewer is joining your team today without knowing what is going on. He should be able to understand the changes.
|
||||
|
||||
![good_title_and_description.png][9]
|
||||
|
||||
What a good title and description look like
|
||||
|
||||
The image above shows [what a good title and description look like][10].
|
||||
|
||||
### The title of the pull request should be self-explanatory
|
||||
|
||||
The title should make clear what is being changed.
|
||||
|
||||
Here are some examples:
|
||||
|
||||
#### Make a useful description
|
||||
|
||||
* Describe what was changed in the pull request
|
||||
* Explain why this PR exists
|
||||
* Make it clear how it does what it sets out to do— for example, does it change a column in the database? How is this done? What happens to the old data?
|
||||
* Use screenshots to demonstrate what has changed.
|
||||
|
||||
|
||||
|
||||
### Recap
|
||||
|
||||
#### Pull request size
|
||||
|
||||
The pull request must have a maximum of 250 lines of change.
|
||||
|
||||
#### Feature breakdown
|
||||
|
||||
Whenever possible, break pull requests into smaller ones.
|
||||
|
||||
#### Single Responsibility Principle
|
||||
|
||||
The pull request should do only one thing.
|
||||
|
||||
#### Title
|
||||
|
||||
Create a self-explanatory title that describes what the pull request does.
|
||||
|
||||
#### Description
|
||||
|
||||
Detail what was changed, why it was changed, and how it was changed.
|
||||
|
||||
_This article was originally posted at[Medium][11]. Reposted with permission._
|
||||
|
||||
--------------------------------------------------------------------------------
|
||||
|
||||
via: https://opensource.com/article/18/6/anatomy-perfect-pull-request
|
||||
|
||||
作者:[Hugo Dias][a]
|
||||
选题:[lujun9972](https://github.com/lujun9972)
|
||||
译者:[译者ID](https://github.com/译者ID)
|
||||
校对:[校对者ID](https://github.com/校对者ID)
|
||||
|
||||
本文由 [LCTT](https://github.com/LCTT/TranslateProject) 原创编译,[Linux中国](https://linux.cn/) 荣誉推出
|
||||
|
||||
[a]:https://opensource.com/users/hugodias
|
||||
[1]:https://smartbear.com/learn/code-review/best-practices-for-peer-code-review/
|
||||
[2]:https://smallbusinessprogramming.com/optimal-pull-request-size/
|
||||
[3]:https://en.wikipedia.org/wiki/Modular_programming
|
||||
[4]:https://en.wikipedia.org/wiki/Class_%28computer_programming%29
|
||||
[5]:https://en.wikipedia.org/wiki/Software_feature
|
||||
[6]:https://en.wikipedia.org/wiki/Software
|
||||
[7]:https://en.wikipedia.org/wiki/Encapsulation_(computer_programming)
|
||||
[8]:/file/400671
|
||||
[9]:https://opensource.com/sites/default/files/uploads/good_title_and_description.png (good_title_and_description.png)
|
||||
[10]:https://github.com/rails/rails/pull/32865
|
||||
[11]:https://medium.com/@hugooodias/the-anatomy-of-a-perfect-pull-request-567382bb6067
|
@ -1,189 +0,0 @@
|
||||
What Happens When You Want to Create a Special File with All Special Characters in Linux?
|
||||
============================================================
|
||||
|
||||
|
||||

|
||||
Learn how to handle creation of a special file filled with special characters.[Used with permission][1]
|
||||
|
||||
I recently joined Holberton School as a student, hoping to learn full-stack software development. What I did not expect was that in two weeks I would be pretty much proficient with creating shell scripts that would make my coding life easy and fast!
|
||||
|
||||
So what is the post about? It is about a novel problem that my peers and I faced when we were asked to create a file with no regular alphabets/ numbers but instead special characters!! Just to give you a look at what kind of file name we were dealing with —
|
||||
|
||||
### \*\\’”Holberton School”\’\\*$\?\*\*\*\*\*:)
|
||||
|
||||
What a novel file name! Of course, this question was met with the collective groaning and long drawn sighs of all 55 (batch #5) students!
|
||||
|
||||
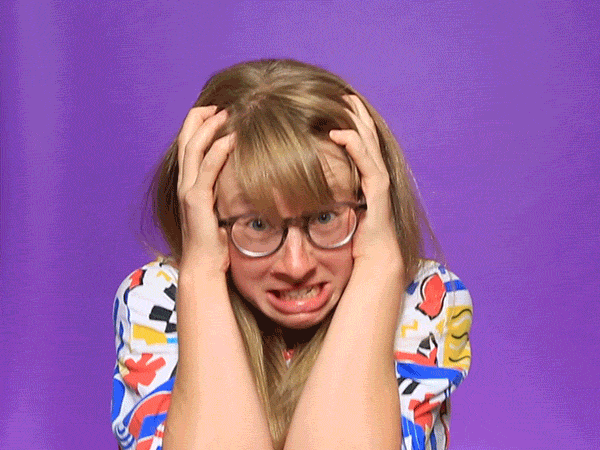
|
||||
|
||||
Some proceeded to make their lives easier by breaking the file name into pieces on a doc file and adding in the **“\\” or “\”** in front of certain special character which kind of resulted in this format -
|
||||
|
||||
#### \\*\\\\’\”Holberton School\”\\’\\\\*$\\?\\*\\*\\*\\*\\*:)
|
||||
|
||||
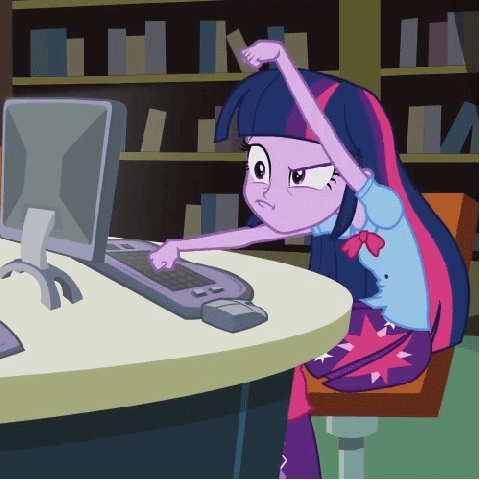
|
||||
|
||||
Everyone trying to get the \\ right
|
||||
|
||||
bamboozled? me, too! I did not want to believe that this was the only way to solve this, as I was getting frustrated with every “\\” or “\” that was required to escape and print those special characters as normal characters!
|
||||
|
||||
If you’re new to shell scripting, here is a quick walk through on why so many “\\” , “\” were required and where.
|
||||
|
||||
In shell scripting “ ” and ‘ ’ have special usage and once you understand and remember when and where to use them it can make your life easier!
|
||||
|
||||
#### Double Quoting
|
||||
|
||||
The first type of quoting we will look at is double quotes. **If you place text inside double quotes, all the special characters used by the shell lose their special meaning and are treated as ordinary characters. The exceptions are “$”, “\” (backslash), and “`” (back- quote).**This means that word-splitting, pathname expansion, tilde expansion, and brace expansion are suppressed, but parameter expansion, arithmetic expansion, and command substitution are still carried out. Using double quotes, we can cope with filenames containing embedded spaces.
|
||||
|
||||
So this means that you can create file with names that have spaces between words — if that is your thing, but I would suggest you to not do that as it is inconvenient and rather an unpleasant experience for you to try to find that file when you need !
|
||||
|
||||
**Quoting “THE” guide for linux I follow and read like it is the Harry Potter of the linux coding world —**
|
||||
|
||||
Say you were the unfortunate victim of a file called two words.txt. If you tried to use this on the command line, word-splitting would cause this to be treated as two separate arguments rather than the desired single argument:
|
||||
|
||||
**[[me@linuxbox][3] me]$ ls -l two words.txt**
|
||||
|
||||
```
|
||||
ls: cannot access two: No such file or directory
|
||||
ls: cannot access words.txt: No such file or directory
|
||||
```
|
||||
|
||||
By using double quotes, you can stop the word-splitting and get the desired result; further, you can even repair the damage:
|
||||
|
||||
```
|
||||
[me@linuxbox me]$ ls -l “two words.txt”
|
||||
-rw-rw-r — 1 me me 18 2008–02–20 13:03 two words.txt
|
||||
[me@linuxbox me]$ mv “two words.txt” two_words.t
|
||||
```
|
||||
|
||||
There! Now we don’t have to keep typing those pesky double quotes.
|
||||
|
||||
Now, let us talk about single quotes and what is their significance in shell —
|
||||
|
||||
#### Single Quotes
|
||||
|
||||
Enclosing characters in single quotes (‘’’) preserves the literal value of each character within the quotes. A single quote may not occur between single quotes, even when preceded by a backslash.
|
||||
|
||||
Yes! that got me and I was wondering how will I use it, apparently when I was googling to find and easier way to do it I stumbled across this piece of information on the internet —
|
||||
|
||||
### Strong quoting
|
||||
|
||||
Strong quoting is very easy to explain:
|
||||
|
||||
Inside a single-quoted string **nothing** is interpreted, except the single-quote that closes the string.
|
||||
|
||||
```
|
||||
echo 'Your PATH is: $PATH'
|
||||
```
|
||||
|
||||
`$PATH` won't be expanded, it's interpreted as ordinary text because it's surrounded by strong quotes.
|
||||
|
||||
In practice that means to produce a text like `Here's my test…` as a single-quoted string, **you have to leave and re-enter the single quoting to get the character "`'`" as literal text:**
|
||||
|
||||
```
|
||||
# WRONG
|
||||
echo 'Here's my test...'
|
||||
```
|
||||
|
||||
```
|
||||
# RIGHT
|
||||
echo 'Here'\''s my test...'
|
||||
```
|
||||
|
||||
```
|
||||
# ALTERNATIVE: It's also possible to mix-and-match quotes for readability:
|
||||
echo "Here's my test"
|
||||
```
|
||||
|
||||
Well now you’re wondering — “well that explains the quotes but what about the “\”??”
|
||||
|
||||
So for certain characters we need a special way to escape those pesky “\” we saw in that file name.
|
||||
|
||||
#### Escaping Characters
|
||||
|
||||
Sometimes you only want to quote a single character. To do this, you can precede a character with a backslash, which in this context is called the _escape character_ . Often this is done inside double quotes to selectively prevent an expansion:
|
||||
|
||||
```
|
||||
[me@linuxbox me]$ echo “The balance for user $USER is: \$5.00”
|
||||
The balance for user me is: $5.00
|
||||
```
|
||||
|
||||
It is also common to use escaping to eliminate the special meaning of a character in a filename. For example, it is possible to use characters in filenames that normally have special meaning to the shell. These would include “$”, “!”, “&”, “ “, and others. To include a special character in a filename you can to this:
|
||||
|
||||
```
|
||||
[me@linuxbox me]$ mv bad\&filename good_filename
|
||||
```
|
||||
|
||||
> _**To allow a backslash character to appear, escape it by typing “\\”. Note that within single quotes, the backslash loses its special meaning and is treated as an ordinary character.**_
|
||||
|
||||
Looking at the filename now we can understand better as to why the “\\” were used in front of all those “\”s.
|
||||
|
||||
So, to print the file name without losing “\” and other special characters what others did was to suppress the “\” with “\\” and to print the single quotes there are a few ways you can do that.
|
||||
|
||||
```
|
||||
1. echo $'It\'s Shell Programming' # ksh, bash, and zsh only, does not expand variables
|
||||
2. echo "It's Shell Programming" # all shells, expands variables
|
||||
3. echo 'It'\''s Shell Programming' # all shells, single quote is outside the quotes
|
||||
4\. echo 'It'"'"'s Shell Programming' # all shells, single quote is inside double quotes
|
||||
```
|
||||
|
||||
```
|
||||
for further reading please follow this link
|
||||
```
|
||||
|
||||
Looking at option 3, I realized this would mean that I would only need to use “\” and single quotes at certain places to be able to write the whole file without getting frustrated with “\\” placements.
|
||||
|
||||
So with the hope in mind and lesser trial and errors I was actually able to print out the file name like this:
|
||||
|
||||
#### ‘\*\\’\’’”Holberton School”\’\’’\\*$\?\*\*\*\*\*:)’
|
||||
|
||||
to understand better I have added an **“a”** instead of my single quotes so that the file name and process becomes more clearer. For a better understanding, I’ll break them down into modules:
|
||||
|
||||
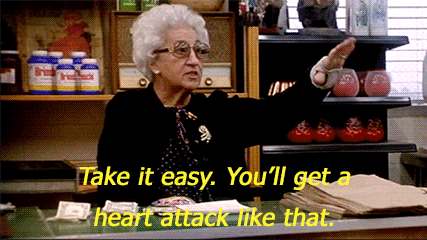
|
||||
|
||||
#### a\*\\a \’ a”Holberton School”\a \’ a\\*$\?\*\*\*\*\*:)a
|
||||
|
||||
#### Module 1 — a\*\\a
|
||||
|
||||
Here the use of single quote (a) creates a safe suppression for \*\\ and as mentioned before in strong quoting, the only way we can print the ‘ is to leave and re-enter the single quoting to get the character.
|
||||
|
||||
#### Module 2 , 4— \’
|
||||
|
||||
The \ suppresses the single quote as a standalone module.
|
||||
|
||||
#### Module 3 — a”Holberton School”\a
|
||||
|
||||
Here the use of single quote (a) creates a safe suppression for double quotes and \ along with regular text.
|
||||
|
||||
#### Module 5 — a\\*$\?\*\*\*\*\*:)a
|
||||
|
||||
Here the use of single quote (a) creates a safe suppression for all special characters being used such as *, \, $, ?, : and ).
|
||||
|
||||
so in the end I was able to be lazy and maintain my sanity, and got away with only using single quotes to create small modules and “\” in certain places.
|
||||
|
||||
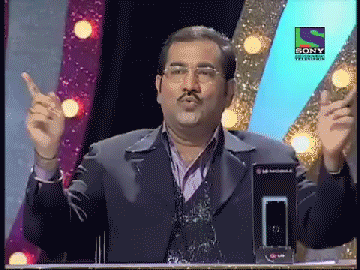
|
||||
|
||||
And, that is how I was able to get the file to work right! After a few misses, it felt amazing and it was great to learn a new way to do things!
|
||||
|
||||
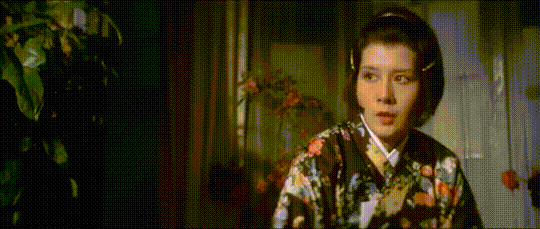
|
||||
|
||||
Handled that curve-ball pretty well! Hope this helps you in the future when, someday you might need to create a special file for a special reason in shell!
|
||||
|
||||
_**Mitali Sengupta **is a former digital marketing professional, currently enrolled as a full-stack engineering student at Holberton School. She is passionate about innovation in AI and Blockchain technologies.. You can contact Mitali on [Twitter][4], [LinkedIn][5] or [GitHub][6]._
|
||||
|
||||
--------------------------------------------------------------------------------
|
||||
|
||||
via: https://www.linux.com/blog/what-happens-when-you-want-create-special-file-all-special-characters-linux
|
||||
|
||||
作者:[MITALI SENGUPTA ][a]
|
||||
译者:[译者ID](https://github.com/译者ID)
|
||||
校对:[校对者ID](https://github.com/校对者ID)
|
||||
|
||||
本文由 [LCTT](https://github.com/LCTT/TranslateProject) 原创编译,[Linux中国](https://linux.cn/) 荣誉推出
|
||||
|
||||
[a]:https://www.linux.com/users/mitalisengupta
|
||||
[1]:https://www.linux.com/licenses/category/used-permission
|
||||
[2]:https://www.linux.com/files/images/special-charspng
|
||||
[3]:mailto:me@linuxbox
|
||||
[4]:https://twitter.com/aadhiBangalan
|
||||
[5]:https://www.linkedin.com/in/mitali-sengupta-auger
|
||||
[6]:https://github.com/MitaliSengupta
|
||||
[7]:http://mywiki.wooledge.org/Quotes#Examples
|
@ -1,104 +0,0 @@
|
||||
Refreshing old computers with Linux
|
||||
============================================================
|
||||
|
||||
### A middle school's Tech Stewardship program is now an elective class for science and technology students.
|
||||
|
||||
|
||||

|
||||
Image by : opensource.com
|
||||
|
||||
It's nearly impossible to enter a school these days without seeing an abundance of technology. Despite this influx of computers into education, funding inequity forces school systems to make difficult choices. Some educators see things as they are and wonder, "Why?" while others see problems as opportunities and think, "Why not?"
|
||||
|
||||
[Andrew Dobbie ][31]is one of those visionaries who saw his love of Linux and computer reimaging as a unique learning opportunity for his students.
|
||||
|
||||
Andrew teaches sixth grade at Centennial Senior Public School in Brampton, Ontario, Canada, and is a[Google Certified Innovator][16]. Andrew said, "Centennial Senior Public School hosts a special regional science & technology program that invites students from throughout the region to spend three years learning Ontario curriculum through the lens of science and technology." However, the school's students were in danger of falling prey to the digital divide that's exacerbated by hardware and software product lifecycles and inadequate funding.
|
||||
|
||||

|
||||
|
||||
Image courtesy of [Affordable Tech for All][6]
|
||||
|
||||
Although there was a school-wide need for access to computers in the classrooms, Andrew and his students discovered that dozens of old computers were being shipped out of the school because they were too old and slow to keep up with the latest proprietary operating systems or function on the school's network.
|
||||
|
||||
Andrew saw this problem as a unique learning opportunity for his students and created the [Tech Stewardship][17] program. He works in partnership with two other teachers, Mike Doiu and Neil Lyons, and some students, who "began experimenting with open source operating systems like [Lubuntu][18] and [CubLinux][19] to help develop a solution to our in-class computer problem," he says.
|
||||
|
||||
The sixth-grade students deployed the reimaged computers into classrooms throughout the school. When they exhausted the school's supply of surplus computers, they sourced more free computers from a local nonprofit organization called [Renewed Computer Technology Ontario][20]. In all, the Tech Stewardship program has provided more than 200 reimaged computers for students to use in classrooms throughout the school.
|
||||
|
||||
|
||||

|
||||
|
||||
Image courtesy of [Affordable Tech for All][7]
|
||||
|
||||
The Tech Stewardship program is now an elective class for the school's science and technology students in grades six, seven, and eight. Not only are the students learning about computer reimaging, they're also giving back to their local communities through this open source outreach program.
|
||||
|
||||
### A broad impact
|
||||
|
||||
The Tech Stewardship program is linked directly to the school's curriculum, especially in social studies by teaching the [United Nations' Sustainable Development Goals][21] (SDGs). The program is a member of [Teach SDGs][22], and Andrew serves as a Teach SDGs ambassador. Also, as a Google Certified Innovator, Andrew partners with Google and the [EdTechTeam][23], and Tech Stewardship has participated in Ontario's [Bring it Together][24] conference for educational technology.
|
||||
|
||||
Andrew's students also serve as mentors to their fellow students. In one instance, a group of girls taught a grade 3 class about effective use of Google Drive and helped these younger students to make the best use of their Linux computers. Andrew said, "outreach and extension of learning beyond the classroom at Centennial is a major goal of the Tech Stewardship program."
|
||||
|
||||
### What the students say
|
||||
|
||||
Linux and open source are an integral part of the program. A girl named Ashna says, "In grade 6, Mr. Dobbie had shown us how to reimage a computer into Linux to use it for educational purposes. Since then, we have been learning more and growing." Student Shradhaa says, "At the very beginning, we didn't even know how to reimage with Linux. Mr. Dobbie told us to write steps for how to reimage Linux devices, and using those steps we are trying to reimage the computers."
|
||||
|
||||
|
||||

|
||||
|
||||
Image courtesy of [Affordable Tech for All][8]
|
||||
|
||||
The students were quick to add that Tech Stewardship has become a portal for discussion about being advocates for the change they want to see in the world. Through their hands-on activity, students learn to support the United Nations Sustainable Development goals. They also learn lessons far beyond the curriculum itself. For example, a student named Areez says he has learned how to find other resources, including donations, that allow the project to expand, since the class work upfitting older computers doesn't produce an income stream.
|
||||
|
||||
Another student, Harini, thinks the Tech Stewardship program has demonstrated to other students what is possible and how one small initiative can change the world. After learning about the program, 40 other schools and individuals are reimaging computers with Linux. Harini says, "The more people who use them for educational purposes, the more outstanding the future will become since those educated people will lead out new, amazing lives with jobs."
|
||||
|
||||
Joshua, another student in the program, sees it this way: "I thought of it as just a fun experience, but as it went on, we continued learning and understanding how what we were doing was making such a big impact on the world!" Later, he says, "a school reached out to us and asked us if we could reimage some computers for them. We went and completed the task. Then it continued to grow, as people from Europe came to see how we were fixing broken computers and started doing it when they went back."
|
||||
|
||||
Andrew Dobbie is keen to share his experience with schools and interested individuals. You can contact him on [Twitter][25] or through his [website][26].
|
||||
|
||||
|
||||
### About the author
|
||||
|
||||
[][27] Don Watkins - Educator, education technology specialist, entrepreneur, open source advocate. M.A. in Educational Psychology, MSED in Educational Leadership, Linux system administrator, CCNA, virtualization using Virtual Box. Follow me at [@Don_Watkins .][13][More about me][14]
|
||||
|
||||
--------------------------------------------------------------------------------
|
||||
|
||||
via: https://opensource.com/article/18/1/new-linux-computers-classroom
|
||||
|
||||
作者:[Don Watkins ][a]
|
||||
译者:[译者ID](https://github.com/译者ID)
|
||||
校对:[校对者ID](https://github.com/校对者ID)
|
||||
|
||||
本文由 [LCTT](https://github.com/LCTT/TranslateProject) 原创编译,[Linux中国](https://linux.cn/) 荣誉推出
|
||||
|
||||
[a]:https://opensource.com/users/don-watkins
|
||||
[1]:https://opensource.com/resources/what-is-linux?intcmp=70160000000h1jYAAQ&utm_source=intcallout&utm_campaign=linuxcontent
|
||||
[2]:https://opensource.com/resources/what-are-linux-containers?intcmp=70160000000h1jYAAQ&utm_source=intcallout&utm_campaign=linuxcontent
|
||||
[3]:https://developers.redhat.com/promotions/linux-cheatsheet/?intcmp=70160000000h1jYAAQ&utm_source=intcallout&utm_campaign=linuxcontent
|
||||
[4]:https://developers.redhat.com/cheat-sheet/advanced-linux-commands-cheatsheet?intcmp=70160000000h1jYAAQ&utm_source=intcallout&utm_campaign=linuxcontent
|
||||
[5]:https://opensource.com/tags/linux?intcmp=70160000000h1jYAAQ&utm_source=intcallout&utm_campaign=linuxcontent
|
||||
[6]:https://photos.google.com/share/AF1QipPnm-q9OIQnrzDD4n7oWIBBIE7RQ6BI9lv486RaU5lKBrs88pq3gPKM8VAgY0prkw?key=cS1RdEZ3ZHdXLWp0bUwzMEk3UnFQRkUwbWl1dWhn
|
||||
[7]:https://photos.google.com/share/AF1QipPnm-q9OIQnrzDD4n7oWIBBIE7RQ6BI9lv486RaU5lKBrs88pq3gPKM8VAgY0prkw?key=cS1RdEZ3ZHdXLWp0bUwzMEk3UnFQRkUwbWl1dWhn
|
||||
[8]:https://photos.google.com/share/AF1QipPnm-q9OIQnrzDD4n7oWIBBIE7RQ6BI9lv486RaU5lKBrs88pq3gPKM8VAgY0prkw?key=cS1RdEZ3ZHdXLWp0bUwzMEk3UnFQRkUwbWl1dWhn
|
||||
[9]:https://opensource.com/file/384581
|
||||
[10]:https://opensource.com/file/384591
|
||||
[11]:https://opensource.com/file/384586
|
||||
[12]:https://opensource.com/article/18/1/new-linux-computers-classroom?rate=bK5X7pRc5y9TyY6jzOZeLDW6ehlWmNPXuP38DYsQ-6I
|
||||
[13]:https://twitter.com/Don_Watkins
|
||||
[14]:https://opensource.com/users/don-watkins
|
||||
[15]:https://opensource.com/user/15542/feed
|
||||
[16]:https://edutrainingcenter.withgoogle.com/certification_innovator
|
||||
[17]:https://sites.google.com/view/mrdobbie/tech-stewardship
|
||||
[18]:https://lubuntu.net/
|
||||
[19]:https://en.wikipedia.org/wiki/Cub_Linux
|
||||
[20]:http://www.rcto.ca/
|
||||
[21]:http://www.un.org/sustainabledevelopment/sustainable-development-goals/
|
||||
[22]:http://www.teachsdgs.org/
|
||||
[23]:https://www.edtechteam.com/team/
|
||||
[24]:http://bringittogether.ca/
|
||||
[25]:https://twitter.com/A_Dobbie11
|
||||
[26]:http://bit.ly/linuxresources
|
||||
[27]:https://opensource.com/users/don-watkins
|
||||
[28]:https://opensource.com/users/don-watkins
|
||||
[29]:https://opensource.com/users/don-watkins
|
||||
[30]:https://opensource.com/article/18/1/new-linux-computers-classroom#comments
|
||||
[31]:https://twitter.com/A_Dobbie11
|
||||
[32]:https://opensource.com/tags/education
|
||||
[33]:https://opensource.com/tags/linux
|
@ -1,123 +0,0 @@
|
||||
[#]: collector: (lujun9972)
|
||||
[#]: translator: ( )
|
||||
[#]: reviewer: ( )
|
||||
[#]: publisher: ( )
|
||||
[#]: url: ( )
|
||||
[#]: subject: (What it means to be Cloud-Native approach — the CNCF way)
|
||||
[#]: via: (https://medium.com/@sonujose993/what-it-means-to-be-cloud-native-approach-the-cncf-way-9e8ab99d4923)
|
||||
[#]: author: (Sonu Jose https://medium.com/@sonujose993)
|
||||
|
||||
What it means to be Cloud-Native approach — the CNCF way
|
||||
======
|
||||
|
||||

|
||||
|
||||
While discussing on Digital Transformation and modern application development Cloud-Native is a term which frequently comes in. But what does it actually means to be cloud-native? This blog is all about giving a good understanding of the cloud-native approach and the ways to achieve it in the CNCF way.
|
||||
|
||||
Michael Dell once said that “the cloud isn’t a place, it’s a way of doing IT”. He was right, and the same can be said of cloud-native.
|
||||
|
||||
Cloud-native is an approach to building and running applications that exploit the advantages of the cloud computing delivery model. Cloud-native is about how applications are created and deployed, not where. … It’s appropriate for both public and private clouds.
|
||||
|
||||
Cloud native architectures take full advantage of on-demand delivery, global deployment, elasticity, and higher-level services. They enable huge improvements in developer productivity, business agility, scalability, availability, utilization, and cost savings.
|
||||
|
||||
### CNCF (Cloud native computing foundation)
|
||||
|
||||
Google has been using containers for many years and they led the Kubernetes project which is a leading container orchestration platform. But alone they can’t really change the broad perspective in the industry around modern applications. So there was a huge need for industry leaders to come together and solve the major problems facing the modern approach. In order to achieve this broader vision, Google donated kubernetes to the Cloud Native foundation and this lead to the birth of CNCF in 2015.
|
||||
|
||||

|
||||
|
||||
Cloud Native computing foundation is created in the Linux foundation for building and managing platforms and solutions for modern application development. It really is a home for amazing projects that enable modern application development. CNCF defines cloud-native as “scalable applications” running in “modern dynamic environments” that use technologies such as containers, microservices, and declarative APIs. Kubernetes is the world’s most popular container-orchestration platform and the first CNCF project.
|
||||
|
||||
### The approach…
|
||||
|
||||
CNCF created a trail map to better understand the concept of Cloud native approach. In this article, we will be discussed based on this landscape. The newer version is available at https://landscape.cncf.io/
|
||||
|
||||
The Cloud Native Trail Map is CNCF’s recommended path through the cloud-native landscape. This doesn’t define a specific path with which we can approach digital transformation rather there are many possible paths you can follow to align with this concept based on your business scenario. This is just a trail to simplify the journey to cloud-native.
|
||||
|
||||
|
||||
Let's start discussing the steps defined in this trail map.
|
||||
|
||||
### 1. CONTAINERIZATION
|
||||
|
||||
![][1]
|
||||
|
||||
You can’t do cloud-native without containerizing your application. It doesn’t matter what size the application is any type of application will do. **A container is a standard unit of software that packages up the code and all its dependencies** so the application runs quickly and reliably from one computing environment to another. Docker is the most preferred platform for containerization. A **Docker container** image is a lightweight, standalone, executable package of software that includes everything needed to run an application.
|
||||
|
||||
### 2. CI/CD
|
||||
|
||||
![][2]
|
||||
|
||||
Setup Continuous Integration/Continuous Delivery (CI/CD) so that changes to your source code automatically result in a new container being built, tested, and deployed to staging and eventually, perhaps, to production. Next thing we need to setup is automated rollouts, rollbacks as well as testing. There are a lot of platforms for CI/CD: **Jenkins, VSTS, Azure DevOps** , TeamCity, JFRog, Spinnaker, etc..
|
||||
|
||||
### 3. ORCHESTRATION
|
||||
|
||||
![][3]
|
||||
|
||||
Container orchestration is all about managing the lifecycles of containers, especially in large, dynamic environments. Software teams use container orchestration to control and automate many tasks. **Kubernetes** is the market-leading orchestration solution. There are other orchestrators like Docker swarm, Mesos, etc.. **Helm Charts** help you define, install, and upgrade even the most complex Kubernetes application.
|
||||
|
||||
### 4. OBSERVABILITY & ANALYSIS
|
||||
|
||||
Kubernetes provides no native storage solution for log data, but you can integrate many existing logging solutions into your Kubernetes cluster. Kubernetes provides detailed information about an application’s resource usage at each of these levels. This information allows you to evaluate your application’s performance and where bottlenecks can be removed to improve overall performance.
|
||||
|
||||
![][4]
|
||||
|
||||
Pick solutions for monitoring, logging, and tracing. Consider CNCF projects Prometheus for monitoring, Fluentd for logging and Jaeger for TracingFor tracing, look for an OpenTracing-compatible implementation like Jaeger.
|
||||
|
||||
### 5. SERVICE MESH
|
||||
|
||||
As its name says it’s all about connecting services, the **discovery of services** , **health checking, routing** and it is used to **monitoring ingress** from the internet. A service mesh also often has more complex operational requirements, like A/B testing, canary rollouts, rate limiting, access control, and end-to-end authentication.
|
||||
|
||||
![][5]
|
||||
|
||||
**Istio** provides behavioral insights and operational control over the service mesh as a whole, offering a complete solution to satisfy the diverse requirements of microservice applications. **CoreDNS** is a fast and flexible tool that is useful for service discovery. **Envoy** and **Linkerd** each enable service mesh architectures.
|
||||
|
||||
### 6. NETWORKING AND POLICY
|
||||
|
||||
It is really important to enable more flexible networking layers. To enable more flexible networking, use a CNI compliant network project like Calico, Flannel, or Weave Net. Open Policy Agent (OPA) is a general purpose policy engine with uses ranging from authorization and admission control to data filtering
|
||||
|
||||
### 7. DISTRIBUTED DATABASE
|
||||
|
||||
A distributed database is a database in which not all storage devices are attached to a common processor. It may be stored in multiple computers, located in the same physical location; or may be dispersed over a network of interconnected computers.
|
||||
|
||||
![][6]
|
||||
|
||||
When you need more resiliency and scalability than you can get from a single database, **Vitess** is a good option for running MySQL at scale through sharding. Rook is a storage orchestrator that integrates a diverse set of storage solutions into Kubernetes. Serving as the “brain” of Kubernetes, etcd provides a reliable way to store data across a cluster of machine
|
||||
|
||||
### 8. MESSAGING
|
||||
|
||||
When you need higher performance than JSON-REST, consider using gRPC or NATS. gRPC is a universal RPC framework. NATS is a multi-modal messaging system that includes request/reply, pub/sub and load balanced queues. It is also applicable and take care of much newer and use cases like IoT.
|
||||
|
||||
### 9. CONTAINER REGISTRY & RUNTIMES
|
||||
|
||||
Container Registry is a single place for your team to manage Docker images, perform vulnerability analysis, and decide who can access what with fine-grained access control. There are many container registries available in market docker hub, Azure Container registry, Harbor, Nexus registry, Amazon Elastic Container Registry and way more…
|
||||
|
||||
![][7]
|
||||
|
||||
Container runtime **containerd** is available as a daemon for Linux and Windows. It manages the complete container lifecycle of its host system, from image transfer and storage to container execution and supervision to low-level storage to network attachments and beyond.
|
||||
|
||||
### 10. SOFTWARE DISTRIBUTION
|
||||
|
||||
If you need to do secure software distribution, evaluate Notary, implementation of The Update Framework (TUF).
|
||||
|
||||
TUF provide a framework (a set of libraries, file formats, and utilities) that can be used to secure new and existing software update systems. The framework should enable applications to be secure from all known attacks on the software update process. It is not concerned with exposing information about what software is being updated (and thus what software the client may be running) or the contents of updates.
|
||||
|
||||
--------------------------------------------------------------------------------
|
||||
|
||||
via: https://medium.com/@sonujose993/what-it-means-to-be-cloud-native-approach-the-cncf-way-9e8ab99d4923
|
||||
|
||||
作者:[Sonu Jose][a]
|
||||
选题:[lujun9972][b]
|
||||
译者:[译者ID](https://github.com/译者ID)
|
||||
校对:[校对者ID](https://github.com/校对者ID)
|
||||
|
||||
本文由 [LCTT](https://github.com/LCTT/TranslateProject) 原创编译,[Linux中国](https://linux.cn/) 荣誉推出
|
||||
|
||||
[a]: https://medium.com/@sonujose993
|
||||
[b]: https://github.com/lujun9972
|
||||
[1]: https://cdn-images-1.medium.com/max/1200/1*glD7bNJG3SlO0_xNmSGPcQ.png
|
||||
[2]: https://cdn-images-1.medium.com/max/1600/1*qOno8YNzmwimlaL9j2fSbA.png
|
||||
[3]: https://cdn-images-1.medium.com/max/1200/1*fw8YJnfF32dWsX_beQpWOw.png
|
||||
[4]: https://cdn-images-1.medium.com/max/1600/1*sbjPYNq76s9lR7D_FK4ltg.png
|
||||
[5]: https://cdn-images-1.medium.com/max/1600/1*kUFBuGfjZSS-n-32CCjtwQ.png
|
||||
[6]: https://cdn-images-1.medium.com/max/1600/1*4OGiB3HHQZBFsALjaRb9pA.jpeg
|
||||
[7]: https://cdn-images-1.medium.com/max/1600/1*VMCJN41mGZs4p2lQHD0nDw.png
|
@ -1,205 +0,0 @@
|
||||
[#]: collector: (lujun9972)
|
||||
[#]: translator: ( )
|
||||
[#]: reviewer: ( )
|
||||
[#]: publisher: ( )
|
||||
[#]: url: ( )
|
||||
[#]: subject: (Why ['1', '7', '11'].map(parseInt) returns [1, NaN, 3] in Javascript)
|
||||
[#]: via: (https://medium.com/dailyjs/parseint-mystery-7c4368ef7b21)
|
||||
[#]: author: (Eric Tong https://medium.com/@erictongs)
|
||||
|
||||
Why ['1', '7', '11'].map(parseInt) returns [1, NaN, 3] in Javascript
|
||||
======
|
||||
![][1]![][2]???
|
||||
|
||||
**Javascript is weird.** Don’t believe me? Try converting an array of strings into integers using map and parseInt. Fire up your console (F12 on Chrome), paste in the following, and press enter (or run the pen below).
|
||||
|
||||
```
|
||||
['1', '7', '11'].map(parseInt);
|
||||
```
|
||||
|
||||
Instead of giving us an array of integers `[1, 7, 11]`, we end up with `[1, NaN, 3]`. What? To find out what on earth is going on, we’ll first have to talk about some Javascript concepts. If you’d like a TLDR, I’ve included a quick summary at the end of this story.
|
||||
|
||||
### Truthiness & Falsiness
|
||||
|
||||
Here’s a simple if-else statement in Javascript:
|
||||
|
||||
```
|
||||
if (true) {
|
||||
// this always runs
|
||||
} else {
|
||||
// this never runs
|
||||
}
|
||||
```
|
||||
|
||||
In this case, the condition of the if-else statement is true, so the if-block is always executed and the else-block is ignored. This is a trivial example because true is a boolean. What if we put a non-boolean as the condition?
|
||||
|
||||
```
|
||||
if ("hello world") {
|
||||
// will this run?
|
||||
console.log("Condition is truthy");
|
||||
} else {
|
||||
// or this?
|
||||
console.log("Condition is falsy");
|
||||
}
|
||||
```
|
||||
|
||||
Try running this code in your developer’s console (F12 on Chrome). You should find that the if-block runs. This is because the string object `"hello world"` is truthy.
|
||||
|
||||
Every Javascript object is either **truthy** or **falsy**. When placed in a boolean context, such as an if-else statement, objects are treated as true or false based on their truthiness. So which objects are truthy and which are falsy? Here’s a simple rule to follow:
|
||||
|
||||
**All values are truthy except for:** `false` **,** `0` **,** `""` **(empty string),** `null` **,** `undefined` **, and** `NaN` **.**
|
||||
|
||||
Confusingly, this means that the string `"false"`, the string `"0"`, an empty object `{}`, and an empty array `[]` are all truthy. You can double check this by passing an object into the Boolean function (e.g. `Boolean("0");`).
|
||||
|
||||
For our purposes, it is enough to remember that `0` is falsy.
|
||||
|
||||
### Radix
|
||||
|
||||
```
|
||||
0 1 2 3 4 5 6 7 8 9 10
|
||||
```
|
||||
|
||||
When we count from zero to nine, we have different symbols for each of the numbers (0–9). However, once we reach ten, we need two different symbols (1 and 0) to represent the number. This is because our decimal counting system has a radix (or base) of ten.
|
||||
|
||||
Radix is the smallest number which can only be represented by more than one symbol. **Different counting systems have different radixes, and so, the same digits can refer to different numbers in counting systems.**
|
||||
|
||||
```
|
||||
DECIMAL BINARY HEXADECIMAL
|
||||
RADIX=10 RADIX=2 RADIX=16
|
||||
0 0 0
|
||||
1 1 1
|
||||
2 10
|
||||
2
|
||||
3
|
||||
11 3
|
||||
4 100 4
|
||||
5 101 5
|
||||
6 110 6
|
||||
7 111 7
|
||||
8 1000 8
|
||||
9 1001 9
|
||||
10 1010 A
|
||||
11 1011 B
|
||||
12 1100 C
|
||||
13 1101 D
|
||||
14 1110 E
|
||||
15 1111 F
|
||||
16 10000 10
|
||||
17 10001
|
||||
11
|
||||
```
|
||||
|
||||
For example, looking at the table above, we see that the same digits 11 can mean different numbers in different counting systems. If the radix is 2, then it refers to the number 3. If the radix is 16, then it refers to the number 17.
|
||||
|
||||
You may have noticed that in our example, parseInt returned 3 when the input is 11, which corresponds to the Binary column in the table above.
|
||||
|
||||
### Function arguments
|
||||
|
||||
Functions in Javascript can be called with any number of arguments, even if they do not equal the number of declared function parameters. Missing arguments are treated as undefined and extra arguments are ignored (but stored in the array-like [arguments object][3]).
|
||||
|
||||
```
|
||||
function foo(x, y) {
|
||||
console.log(x);
|
||||
console.log(y);
|
||||
}
|
||||
foo(1, 2); // logs 1, 2
|
||||
foo(1); // logs 1, undefined
|
||||
foo(1, 2, 3); // logs 1, 2
|
||||
```
|
||||
|
||||
### map()
|
||||
|
||||
We’re almost there!
|
||||
|
||||
Map is a method in the Array prototype that returns a new array of the results of passing each element of the original array into a function. For example, the following code multiplies each element in the array by 3:
|
||||
|
||||
```
|
||||
function multiplyBy3(x) {
|
||||
return x * 3;
|
||||
}
|
||||
const result = [1, 2, 3, 4, 5].map(multiplyBy3);console.log(result); // logs [3, 6, 9, 12, 15];
|
||||
```
|
||||
|
||||
Now, let’s say I want to log each element using `map()` (with no return statements). I should be able to pass `console.log` as an argument into `map()`… right?
|
||||
|
||||
```
|
||||
[1, 2, 3, 4, 5].map(console.log);
|
||||
```
|
||||
|
||||
![][4]![][5]
|
||||
|
||||
Something very strange is going on. Instead of logging just the value, each `console.log` call also logged the index and full array.
|
||||
|
||||
```
|
||||
[1, 2, 3, 4, 5].map(console.log);// The above is equivalent to[1, 2, 3, 4, 5].map(
|
||||
(val, index, array) => console.log(val, index, array)
|
||||
);
|
||||
// and not equivalent to[1, 2, 3, 4, 5].map(
|
||||
val => console.log(val)
|
||||
);
|
||||
```
|
||||
|
||||
When a function is passed into `map()`, for each iteration, **three arguments are passed into the function** : `currentValue`, `currentIndex`, and the full `array`. That is why three entries are logged for each iteration.
|
||||
|
||||
We now have all the pieces we need to solve this mystery.
|
||||
|
||||
### Bringing it together
|
||||
|
||||
ParseInt takes two arguments: `string` and `radix`. If the radix provided is falsy, then by default, radix is set to 10.
|
||||
|
||||
```
|
||||
parseInt('11'); => 11
|
||||
parseInt('11', 2); => 3
|
||||
parseInt('11', 16); => 17
|
||||
parseInt('11', undefined); => 11 (radix is falsy)
|
||||
parseInt('11', 0); => 11 (radix is falsy)
|
||||
```
|
||||
|
||||
Now let’s run our example step-by-step.
|
||||
|
||||
```
|
||||
['1', '7', '11'].map(parseInt); => [1, NaN, 3]// First iteration: val = '1', index = 0, array = ['1', '7', '11']parseInt('1', 0, ['1', '7', '11']); => 1
|
||||
```
|
||||
|
||||
Since 0 is falsy, the radix is set to the default value 10. `parseInt()` only takes two arguments, so the third argument `['1', '7', '11']` is ignored. The string `'1'` in radix 10 refers to the number 1.
|
||||
|
||||
```
|
||||
// Second iteration: val = '7', index = 1, array = ['1', '7', '11']parseInt('7', 1, ['1', '7', '11']); => NaN
|
||||
```
|
||||
|
||||
In a radix 1 system, the symbol `'7'` does not exist. As with the first iteration, the last argument is ignored. So, `parseInt()` returns `NaN`.
|
||||
|
||||
```
|
||||
// Third iteration: val = '11', index = 2, array = ['1', '7', '11']parseInt('11', 2, ['1', '7', '11']); => 3
|
||||
```
|
||||
|
||||
In a radix 2 (binary) system, the symbol `'11'` refers to the number 3. The last argument is ignored.
|
||||
|
||||
### Summary (TLDR)
|
||||
|
||||
`['1', '7', '11'].map(parseInt)` doesn’t work as intended because `map` passes three arguments into `parseInt()` on each iteration. The second argument `index` is passed into parseInt as a `radix` parameter. So, each string in the array is parsed using a different radix. `'7'` is parsed as radix 1, which is `NaN`, `'11'` is parsed as radix 2, which is 3. `'1'` is parsed as the default radix 10, because its index 0 is falsy.
|
||||
|
||||
And so, the following code will work as intended:
|
||||
|
||||
```
|
||||
['1', '7', '11'].map(numStr => parseInt(numStr));
|
||||
```
|
||||
|
||||
--------------------------------------------------------------------------------
|
||||
|
||||
via: https://medium.com/dailyjs/parseint-mystery-7c4368ef7b21
|
||||
|
||||
作者:[Eric Tong][a]
|
||||
选题:[lujun9972][b]
|
||||
译者:[译者ID](https://github.com/译者ID)
|
||||
校对:[校对者ID](https://github.com/校对者ID)
|
||||
|
||||
本文由 [LCTT](https://github.com/LCTT/TranslateProject) 原创编译,[Linux中国](https://linux.cn/) 荣誉推出
|
||||
|
||||
[a]: https://medium.com/@erictongs
|
||||
[b]: https://github.com/lujun9972
|
||||
[1]: https://miro.medium.com/max/60/1*uq5ZTlw6HLRRqbHaPYYL0g.png?q=20
|
||||
[2]: https://miro.medium.com/max/1400/1*uq5ZTlw6HLRRqbHaPYYL0g.png
|
||||
[3]: https://javascriptweblog.wordpress.com/2011/01/18/javascripts-arguments-object-and-beyond/
|
||||
[4]: https://miro.medium.com/max/60/1*rbrCQ_aNOft_OjayDtL6xg.png?q=20
|
||||
[5]: https://miro.medium.com/max/1400/1*rbrCQ_aNOft_OjayDtL6xg.png
|
Loading…
Reference in New Issue
Block a user