mirror of
https://github.com/LCTT/TranslateProject.git
synced 2025-01-10 22:21:11 +08:00
翻译完成: 3 open source distributed tracing tools
This commit is contained in:
parent
5d56926e1c
commit
a0678c4404
@ -1,90 +0,0 @@
|
||||
translating by belitex
|
||||
|
||||
3 open source distributed tracing tools
|
||||
======
|
||||
|
||||
Find performance issues quickly with these tools, which provide a graphical view of what's happening across complex software systems.
|
||||
|
||||

|
||||
|
||||
Distributed tracing systems enable users to track a request through a software system that is distributed across multiple applications, services, and databases as well as intermediaries like proxies. This allows for a deeper understanding of what is happening within the software system. These systems produce graphical representations that show how much time the request took on each step and list each known step.
|
||||
|
||||
A user reviewing this content can determine where the system is experiencing latencies or blockages. Instead of testing the system like a binary search tree when requests start failing, operators and developers can see exactly where the issues begin. This can also reveal where performance changes might be occurring from deployment to deployment. It’s always better to catch regressions automatically by alerting to the anomalous behavior than to have your customers tell you.
|
||||
|
||||
How does this tracing thing work? Well, each request gets a special ID that’s usually injected into the headers. This ID uniquely identifies that transaction. This transaction is normally called a trace. The trace is the overall abstract idea of the entire transaction. Each trace is made up of spans. These spans are the actual work being performed, like a service call or a database request. Each span also has a unique ID. Spans can create subsequent spans called child spans, and child spans can have multiple parents.
|
||||
|
||||
Once a transaction (or trace) has run its course, it can be searched in a presentation layer. There are several tools in this space that we’ll discuss later, but the picture below shows [Jaeger][1] from my [Istio walkthrough][2]. It shows multiple spans of a single trace. The power of this is immediately clear as you can better understand the transaction’s story at a glance.
|
||||
|
||||
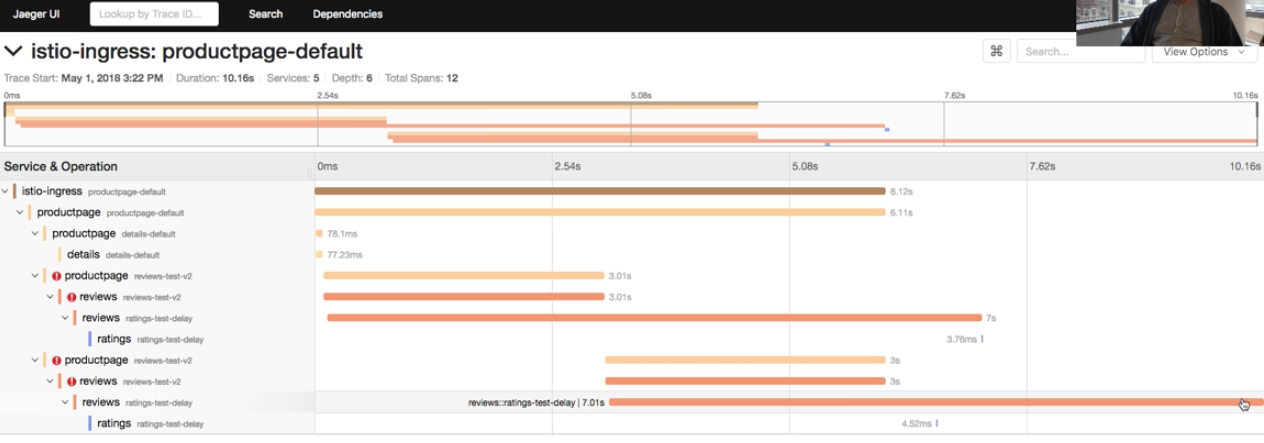
|
||||
|
||||
This demo uses Istio’s built-in OpenTracing implementation, so I can get tracing without even modifying my application. It also uses Jaeger, which is OpenTracing-compatible.
|
||||
|
||||
So what is OpenTracing? Let’s find out.
|
||||
|
||||
### OpenTracing API
|
||||
|
||||
[OpenTracing][3] is a spec that grew out of [Zipkin][4] to provide cross-platform compatibility. It offers a vendor-neutral API for adding tracing to applications and delivering that data into distributed tracing systems. A library written for the OpenTracing spec can be used with any system that is OpenTracing-compliant. Zipkin, Jaeger, and Appdash are examples of open source tools that have adopted the open standard, but even proprietary tools like [Datadog][5] and [Instana][6] are adopting it. This is expected to continue as OpenTracing reaches ubiquitous status.
|
||||
|
||||
### OpenCensus
|
||||
|
||||
Okay, we have OpenTracing, but what is this [OpenCensus][7] thing that keeps popping up in my searches? Is it a competing standard, something completely different, or something complementary?
|
||||
|
||||
The answer depends on who you ask. I will do my best to explain the difference (as I understand it): OpenCensus takes a more holistic or all-inclusive approach. OpenTracing is focused on establishing an open API and spec and not on open implementations for each language and tracing system. OpenCensus provides not only the specification but also the language implementations and wire protocol. It also goes beyond tracing by including additional metrics that are normally outside the scope of distributed tracing systems.
|
||||
|
||||
OpenCensus allows viewing data on the host where the application is running, but it also has a pluggable exporter system for exporting data to central aggregators. The current exporters produced by the OpenCensus team include Zipkin, Prometheus, Jaeger, Stackdriver, Datadog, and SignalFx, but anyone can create an exporter.
|
||||
|
||||
From my perspective, there’s a lot of overlap. One isn’t necessarily better than the other, but it’s important to know what each does and doesn’t do. OpenTracing is primarily a spec, with others doing the implementation and opinionation. OpenCensus provides a holistic approach for the local component with more opinionation but still requires other systems for remote aggregation.
|
||||
|
||||
### Tool options
|
||||
|
||||
#### Zipkin
|
||||
|
||||
Zipkin was one of the first systems of this kind. It was developed by Twitter based on the [Google Dapper paper][8] about the internal system Google uses. Zipkin was written using Java, and it can use Cassandra or ElasticSearch as a scalable backend. Most companies should be satisfied with one of those options. The lowest supported Java version is Java 6. It also uses the [Thrift][9] binary communication protocol, which is popular in the Twitter stack and is hosted as an Apache project.
|
||||
|
||||
The system consists of reporters (clients), collectors, a query service, and a web UI. Zipkin is meant to be safe in production by transmitting only a trace ID within the context of a transaction to inform receivers that a trace is in process. The data collected in each reporter is then transmitted asynchronously to the collectors. The collectors store these spans in the database, and the web UI presents this data to the end user in a consumable format. The delivery of data to the collectors can occur in three different methods: HTTP, Kafka, and Scribe.
|
||||
|
||||
The [Zipkin community][10] has also created [Brave][11], a Java client implementation compatible with Zipkin. It has no dependencies, so it won’t drag your projects down or clutter them with libraries that are incompatible with your corporate standards. There are many other implementations, and Zipkin is compatible with the OpenTracing standard, so these implementations should also work with other distributed tracing systems. The popular Spring framework has a component called [Spring Cloud Sleuth][12] that is compatible with Zipkin.
|
||||
|
||||
#### Jaeger
|
||||
|
||||
[Jaeger][1] is a newer project from Uber Technologies that the [CNCF][13] has since adopted as an Incubating project. It is written in Golang, so you don’t have to worry about having dependencies installed on the host or any overhead of interpreters or language virtual machines. Similar to Zipkin, Jaeger also supports Cassandra and ElasticSearch as scalable storage backends. Jaeger is also fully compatible with the OpenTracing standard.
|
||||
|
||||
Jaeger’s architecture is similar to Zipkin, with clients (reporters), collectors, a query service, and a web UI, but it also has an agent on each host that locally aggregates the data. The agent receives data over a UDP connection, which it batches and sends to a collector. The collector receives that data in the form of the [Thrift][14] protocol and stores that data in Cassandra or ElasticSearch. The query service can access the data store directly and provide that information to the web UI.
|
||||
|
||||
By default, a user won’t get all the traces from the Jaeger clients. The system samples 0.1% (1 in 1,000) of traces that pass through each client. Keeping and transmitting all traces would be a bit overwhelming to most systems. However, this can be increased or decreased by configuring the agents, which the client consults with for its configuration. This sampling isn’t completely random, though, and it’s getting better. Jaeger uses probabilistic sampling, which tries to make an educated guess at whether a new trace should be sampled or not. [Adaptive sampling is on its roadmap][15], which will improve the sampling algorithm by adding additional context for making decisions.
|
||||
|
||||
#### Appdash
|
||||
|
||||
[Appdash][16] is a distributed tracing system written in Golang, like Jaeger. It was created by [Sourcegraph][17] based on Google’s Dapper and Twitter’s Zipkin. Similar to Jaeger and Zipkin, Appdash supports the OpenTracing standard; this was a later addition and requires a component that is different from the default component. This adds risk and complexity.
|
||||
|
||||
At a high level, Appdash’s architecture consists mostly of three components: a client, a local collector, and a remote collector. There’s not a lot of documentation, so this description comes from testing the system and reviewing the code. The client in Appdash gets added to your code. Appdash provides Python, Golang, and Ruby implementations, but OpenTracing libraries can be used with Appdash’s OpenTracing implementation. The client collects the spans and sends them to the local collector. The local collector then sends the data to a centralized Appdash server running its own local collector, which is the remote collector for all other nodes in the system.
|
||||
|
||||
--------------------------------------------------------------------------------
|
||||
|
||||
via: https://opensource.com/article/18/9/distributed-tracing-tools
|
||||
|
||||
作者:[Dan Barker][a]
|
||||
选题:[lujun9972](https://github.com/lujun9972)
|
||||
译者:[译者ID](https://github.com/译者ID)
|
||||
校对:[校对者ID](https://github.com/校对者ID)
|
||||
|
||||
本文由 [LCTT](https://github.com/LCTT/TranslateProject) 原创编译,[Linux中国](https://linux.cn/) 荣誉推出
|
||||
|
||||
[a]: https://opensource.com/users/barkerd427
|
||||
[1]: https://www.jaegertracing.io/
|
||||
[2]: https://www.youtube.com/watch?v=T8BbeqZ0Rls
|
||||
[3]: http://opentracing.io/
|
||||
[4]: https://zipkin.io/
|
||||
[5]: https://www.datadoghq.com/
|
||||
[6]: https://www.instana.com/
|
||||
[7]: https://opencensus.io/
|
||||
[8]: https://research.google.com/archive/papers/dapper-2010-1.pdf
|
||||
[9]: https://thrift.apache.org/
|
||||
[10]: https://zipkin.io/pages/community.html
|
||||
[11]: https://github.com/openzipkin/brave
|
||||
[12]: https://cloud.spring.io/spring-cloud-sleuth/
|
||||
[13]: https://www.cncf.io/
|
||||
[14]: https://en.wikipedia.org/wiki/Apache_Thrift
|
||||
[15]: https://www.jaegertracing.io/docs/roadmap/#adaptive-sampling
|
||||
[16]: https://github.com/sourcegraph/appdash
|
||||
[17]: https://about.sourcegraph.com/
|
@ -0,0 +1,88 @@
|
||||
三个开源的分布式追踪工具
|
||||
======
|
||||
|
||||
这几个工具对复杂软件系统中的实时事件做了可视化,能帮助你快速发现性能问题。
|
||||
|
||||

|
||||
|
||||
分布式追踪系统能够从头到尾地追踪分布式系统中的请求,跨越多个应用、服务、数据库以及像代理这样的中间件。它能帮助你更深入地理解系统中到底发生了什么。追踪系统以图形化的方式,展示出每个已知步骤以及某个请求在每个步骤上的耗时。
|
||||
|
||||
用户可以通过这些展示来判断系统的哪个环节有延迟或阻塞,当请求失败时,运维和开发人员可以看到准确的问题源头,而不需要去测试整个系统,比如用二叉查找树的方法去定位问题。在开发迭代的过程中,追踪系统还能够展示出可能引起性能变化的环节。通过异常行为的警告自动地感知到性能在退化,总是比客户告诉你要好。
|
||||
|
||||
追踪是怎么工作的呢?给每个请求分配一个特殊 ID,这个 ID 通常会插入到请求头部中。它唯一标识了对应的事务。一般把事务叫做 trace,trace 是抽象整个事务的概念。每一个 trace 由 span 组成,span 代表着一次请求中真正执行的操作,比如一次服务调用,一次数据库请求等。每一个 span 也有自己唯一的 ID。span 之下也可以创建子 span,子 span 可以有多个父 span。
|
||||
|
||||
当一次事务(或者说 trace)运行过之后,就可以在追踪系统的表示层上搜索了。有几个工具可以用作表示层,我们下文会讨论,不过,我们先看下面的图,它是我在 [Istio walkthrough][2] 视频教程中提到的 [Jaeger][1] 界面,展示了单个 trace 中的多个 span。很明显,这个图能让你一目了然地对事务有更深的了解。
|
||||
|
||||
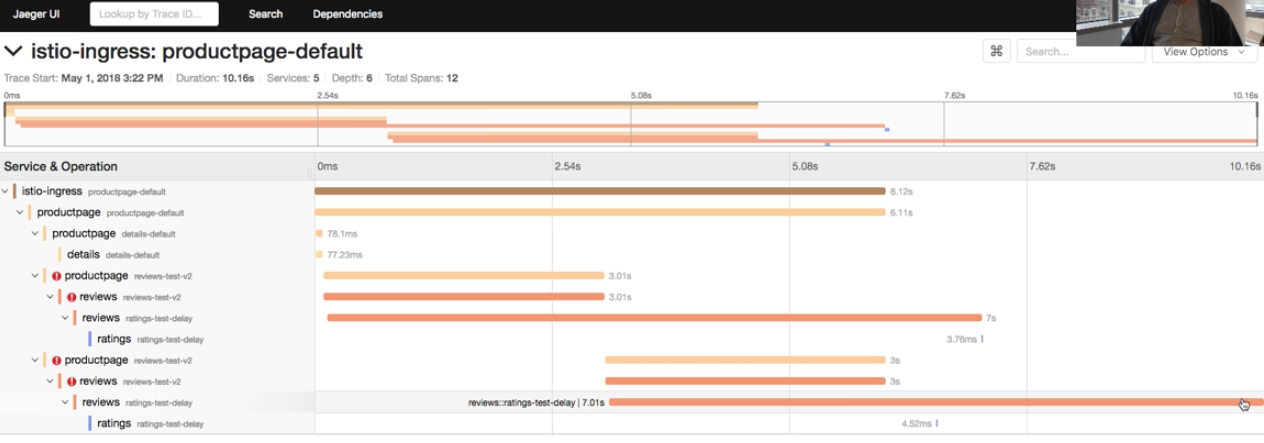
|
||||
|
||||
这个 demo 使用了 Istio 内置的 OpenTracing 实现,所以我甚至不需要修改自己的应用代码就可以获得追踪数据。我也用到了 Jaeger,它是兼容 OpenTracing 的。
|
||||
|
||||
那么 OpenTracing 到底是什么呢?我们来看看。
|
||||
|
||||
### OpenTracing API
|
||||
|
||||
[OpenTracing][3] 是源自 [Zipkin][4] 的规范,以提供跨平台兼容性。它提供了对厂商中立的 API,用来向应用程序添加追踪功能并将追踪数据发送到分布式的追踪系统。按照 OpenTracing 规范编写的库,可以被任何兼容 OpenTracing 的系统使用。采用这个开放标准的开源工具有 Zipkin,Jaeger,和 Appdash 等。甚至像 [Datadog][5] 和 [Instana][6] 这种付费工具也在采用。因为现在 OpenTracing 已经无处不在,这样的趋势有望继续发展下去。
|
||||
|
||||
### OpenCensus
|
||||
|
||||
OpenTracing 已经说过了,可 [OpenCensus][7] 又是什么呢?它在搜索结果中老是出现。它是一个和 OpenTracing 完全不同或者互补的竞争标准吗?
|
||||
|
||||
这个问题的答案取决于你的提问对象。我先尽我所能地解释一下他们的不同(按照我的理解):OpenCensus 更加全面或者说它包罗万象。OpenTracing 专注于建立开放的 API 和规范,而不是为每一种开发语言和追踪系统都提供开放的实现。OpenCensus 不仅提供规范,还提供开发语言的实现,和连接协议,而且它不仅只做追踪,还引入了额外的度量指标,这些一般不在分布式追踪系统的职责范围。
|
||||
|
||||
使用 OpenCensus,我们能够在运行着应用程序的主机上查看追踪数据,但它也有个可插拔的导出器系统,用于导出数据到中心聚合器。目前 OpenCensus 团队提供的导出器包括 Zipkin,Prometheus,Jaeger,Stackdriver,Datadog 和 SignalFx,不过任何人都可以创建一个导出器。
|
||||
|
||||
依我看这两者有很多重叠的部分,没有哪个一定比另外一个好,但是重要的是,要知道它们做什么事情和不做什么事情。OpenTracing 主要是一个规范,具体的实现和独断的设计由其他人来做。OpenCensus 更加独断地为本地组件提供了全面的解决方案,但是仍然需要其他系统做远程的聚合。
|
||||
|
||||
### 可选工具
|
||||
|
||||
#### Zipkin
|
||||
|
||||
Zipkin 是最早出现的这类工具之一。 谷歌在 2010 年发表了介绍其内部追踪系统 Dapper 的[论文][8],Twitter 以此为基础开发了 Zipkin。Zipkin 的开发语言 Java,用 Cassandra 或 ElasticSearch 作为可扩展的存储后端,这些选择能满足大部分公司的需求。Zipkin 支持的最低 Java 版本是 Java 6,它也使用了 [Thrift][9] 的二进制通信协议,Thrift 在 Twitter 的系统中很流行,现在作为 Apache 项目在托管。
|
||||
|
||||
这个系统包括上报器(客户端),数据收集器,查询服务和一个 web 界面。Zipkin 只传输一个带事务上下文的 trace ID 来告知接收者追踪的进行,所以说在生产环境中是安全的。每一个客户端收集到的数据,会异步地传输到数据收集器。收集器把这些 span 的数据存到数据库,web 界面负责用可消费的格式展示这些数据给用户。客户端传输数据到收集器有三种方式:HTTP,Kafka 和 Scribe。
|
||||
|
||||
[Zipkin 社区][10] 还提供了 [Brave][11],一个跟 Zipkin 兼容的 Java 客户端的实现。由于 Brave 没有任何依赖,所以它不会拖累你的项目,也不会使用跟你们公司标准不兼容的库来搞乱你的项目。除 Brave 之外,还有很多其他的 Zipkin 客户端实现,因为 Zipkin 和 OpenTracing 标准是兼容的,所以这些实现也能用到其他的分布式追踪系统中。流行的 Spring 框架中一个叫 [Spring Cloud Sleuth][12] 的分布式追踪组件,它和 Zipkin 是兼容的。
|
||||
|
||||
#### Jaeger
|
||||
|
||||
[Jaeger][1] 来自 Uber,是一个比较新的项目,[CNCF][13] (云原生计算基金会)已经把 Jaeger 托管为孵化项目。Jaeger 使用 Golang 开发,因此你不用担心在服务器上安装依赖的问题,也不用担心开发语言的解释器或虚拟机的开销。和 Zipkin 类似,Jaeger 也支持用 Cassandra 和 ElasticSearch 做可扩展的存储后端。Jaeger 也完全兼容 OpenTracing 标准。
|
||||
|
||||
Jaeger 的架构跟 Zipkin 很像,有客户端(上报器),数据收集器,查询服务和一个 web 界面,不过它还有一个在各个服务器上运行着的代理,负责在服务器本地做数据聚合。代理通过一个 UDP 连接接收数据,然后分批处理,发送到数据收集器。收集器接收到的数据是 [Thrift][14] 协议的格式,它把数据存到 Cassandra 或者 ElasticSearch 中。查询服务能直接访问数据库,并给 web 界面提供所需的信息。
|
||||
|
||||
默认情况下,Jaeger 客户端不会采集所有的追踪数据,只抽样了 0.1% 的( 1000 个采 1 个)追踪数据。对大多数系统来说,保留所有的追踪数据并传输的话就太多了。不过,通过配置代理可以调整这个值,客户端会从代理获取自己的配置。这个抽样并不是完全随机的,并且正在变得越来越好。Jaeger 使用概率抽样,试图对是否应该对新踪迹进行抽样进行有根据的猜测。 自适应采样已经在[路线图][15],它将通过添加额外的,能够帮助做决策的上下文,来改进采样算法。
|
||||
|
||||
#### Appdash
|
||||
|
||||
[Appdash][16] 也是一个用 Golang 写的分布式追踪系统,和 Jaeger 一样。Appdash 是 [Sourcegraph][17] 公司基于谷歌的 Dapper 和 Twitter 的 Zipkin 开发的。同样的,它也支持 Opentracing 标准,不过这是后来添加的功能,依赖了一个与默认组件不同的组件,因此增加了风险和复杂度。
|
||||
|
||||
从高层次来看,Appdash 的架构主要有三个部分:客户端,本地收集器和远程收集器。因为没有很多文档,所以这个架构描述是基于对系统的测试以及查看源码。写代码时需要把 Appdash 的客户端添加进来。 Appdash 提供了 Python,Golang 和 Ruby 的实现,不过 OpenTracing 库可以与 Appdash 的 OpenTracing 实现一起使用。 客户端收集 span 数据,并将它们发送到本地收集器。然后,本地收集器将数据发送到中心的 Appdash 服务器,这个服务器上运行着自己的本地收集器,它的本地收集器是其他所有节点的远程收集器。
|
||||
|
||||
--------------------------------------------------------------------------------
|
||||
|
||||
via: https://opensource.com/article/18/9/distributed-tracing-tools
|
||||
|
||||
作者:[Dan Barker][a]
|
||||
选题:[lujun9972](https://github.com/lujun9972)
|
||||
译者:[belitex](https://github.com/belitex)
|
||||
校对:[校对者ID](https://github.com/校对者ID)
|
||||
|
||||
本文由 [LCTT](https://github.com/LCTT/TranslateProject) 原创编译,[Linux中国](https://linux.cn/) 荣誉推出
|
||||
|
||||
[a]: https://opensource.com/users/barkerd427
|
||||
[1]: https://www.jaegertracing.io/
|
||||
[2]: https://www.youtube.com/watch?v=T8BbeqZ0Rls
|
||||
[3]: http://opentracing.io/
|
||||
[4]: https://zipkin.io/
|
||||
[5]: https://www.datadoghq.com/
|
||||
[6]: https://www.instana.com/
|
||||
[7]: https://opencensus.io/
|
||||
[8]: https://research.google.com/archive/papers/dapper-2010-1.pdf
|
||||
[9]: https://thrift.apache.org/
|
||||
[10]: https://zipkin.io/pages/community.html
|
||||
[11]: https://github.com/openzipkin/brave
|
||||
[12]: https://cloud.spring.io/spring-cloud-sleuth/
|
||||
[13]: https://www.cncf.io/
|
||||
[14]: https://en.wikipedia.org/wiki/Apache_Thrift
|
||||
[15]: https://www.jaegertracing.io/docs/roadmap/#adaptive-sampling
|
||||
[16]: https://github.com/sourcegraph/appdash
|
||||
[17]: https://about.sourcegraph.com/
|
Loading…
Reference in New Issue
Block a user