mirror of
https://github.com/LCTT/TranslateProject.git
synced 2025-01-25 23:11:02 +08:00
TSL&PRF
This commit is contained in:
parent
83edac5ac0
commit
9165f56e32
@ -1,460 +0,0 @@
|
||||
[#]: subject: "Crunch numbers in Python with NumPy"
|
||||
[#]: via: "https://opensource.com/article/21/9/python-numpy"
|
||||
[#]: author: "Ayush Sharma https://opensource.com/users/ayushsharma"
|
||||
[#]: collector: "lujun9972"
|
||||
[#]: translator: "wxy"
|
||||
[#]: reviewer: " "
|
||||
[#]: publisher: " "
|
||||
[#]: url: " "
|
||||
|
||||
Crunch numbers in Python with NumPy
|
||||
======
|
||||
This article discusses installing NumPy, and then creating, reading, and
|
||||
sorting NumPy arrays.
|
||||
![old school calculator][1]
|
||||
|
||||
NumPy, or **Num**erical **Py**thon, is a library that makes it easy to do statistical and set operations on linear series and matrices in Python. It is orders of magnitude faster than Python lists, [which I covered in my notes on Python Data Types][2]. NumPy is used quite frequently in data analysis and scientific calculations.
|
||||
|
||||
I'm going to go over installing NumPy, and then creating, reading, and sorting NumPy arrays. NumPy arrays are also called _ndarrays_, short for **n-dimensional arrays**.
|
||||
|
||||
### Installing NumPy
|
||||
|
||||
Installing the NumPy package is really simple using `pip`, and it can be installed just like you would install any other package.
|
||||
|
||||
|
||||
```
|
||||
pip install numpy
|
||||
```
|
||||
|
||||
With the NumPy package installed, just import it into your Python file.
|
||||
|
||||
|
||||
```
|
||||
import numpy as np
|
||||
```
|
||||
|
||||
Importing `numpy` as `np` is a standard convention, but instead of using `np` you can use any other alias that you want.
|
||||
|
||||
### Why use NumPy? Because it is orders of magnitude faster than Python lists.
|
||||
|
||||
NumPy is orders of magnitude faster than normal Python lists when it comes to handling a large number of values. To see exactly how fast it is, I'm going to first measure the time it takes for `min()` and `max()` operations on a normal Python list.
|
||||
|
||||
I will first create a Python list with 999,999,999 items.
|
||||
|
||||
|
||||
```
|
||||
>>> my_list = range(1, 1000000000)
|
||||
>>> len(my_list)
|
||||
999999999
|
||||
```
|
||||
|
||||
Now I'll measure the time for finding the minimum value in this list.
|
||||
|
||||
|
||||
```
|
||||
>>> start = time.time()
|
||||
>>> min(my_list)
|
||||
1
|
||||
>>> print('Time elapsed in milliseconds: ' + str((time.time() - start) * 1000))
|
||||
Time elapsed in milliseconds: 27007.00879096985
|
||||
```
|
||||
|
||||
That took about 27,007 milliseconds or about **27 seconds**. That’s a long time. Now I'll try to find the time for finding the maximum value.
|
||||
|
||||
|
||||
```
|
||||
>>> start = time.time()
|
||||
>>> max(my_list)
|
||||
999999999
|
||||
>>> print('Time elapsed in milliseconds: ' + str((time.time() - start) * 1000))
|
||||
Time elapsed in milliseconds: 28111.071348190308
|
||||
```
|
||||
|
||||
That took about 28,111 milliseconds, which is about **28 seconds**.
|
||||
|
||||
Now I'll try to find the time to find the minimum and maximum value using NumPy.
|
||||
|
||||
|
||||
```
|
||||
>>> my_list = np.arange(1, 1000000000)
|
||||
>>> len(my_list)
|
||||
999999999
|
||||
>>> start = time.time()
|
||||
>>> my_list.min()
|
||||
1
|
||||
>>> print('Time elapsed in milliseconds: ' + str((time.time() - start) * 1000))
|
||||
Time elapsed in milliseconds: 1151.1778831481934
|
||||
>>>
|
||||
>>> start = time.time()
|
||||
>>> my_list.max()
|
||||
999999999
|
||||
>>> print('Time elapsed in milliseconds: ' + str((time.time() - start) * 1000))
|
||||
Time elapsed in milliseconds: 1114.8970127105713
|
||||
```
|
||||
|
||||
That took about 1151 milliseconds for finding the minimum value, and 1114 milliseconds for finding the maximum value. These are around **1 second**.
|
||||
|
||||
As you can see, using NumPy reduces the time to find the minimum and maximum of a list of around a billion values **from around 28 seconds to 1 second**. This is the power of NumPy.
|
||||
|
||||
### Creating ndarrays using Python lists
|
||||
|
||||
There are several ways to create an ndarray in NumPy.
|
||||
|
||||
You can create an ndarray by using a list of elements.
|
||||
|
||||
|
||||
```
|
||||
>>> my_ndarray = np.array([1, 2, 3, 4, 5])
|
||||
>>> print(my_ndarray)
|
||||
[1 2 3 4 5]
|
||||
```
|
||||
|
||||
With the above ndarray defined, I'll check out a few things. First, the type of the variable defined above is `numpy.ndarray`. This is the type of all NumPy ndarrays.
|
||||
|
||||
|
||||
```
|
||||
>>> type(my_ndarray)
|
||||
<class 'numpy.ndarray'>
|
||||
```
|
||||
|
||||
Another thing to note here would be _shape_. The shape of an ndarray is the length of each dimension of the ndarray. As you can see, the shape of `my_ndarray` is `(5,)`. This means that `my_ndarray` contains one dimension with 5 elements.
|
||||
|
||||
|
||||
```
|
||||
>>> np.shape(my_ndarray)
|
||||
(5,)
|
||||
```
|
||||
|
||||
The number of dimensions in the array is called its _rank_. So the above ndarray has a rank of 1.
|
||||
|
||||
I'll define another ndarray `my_ndarray2` as a multi-dimensional ndarray. What will its shape be then? See below.
|
||||
|
||||
|
||||
```
|
||||
>>> my_ndarray2 = np.array([(1, 2, 3), (4, 5, 6)])
|
||||
>>> np.shape(my_ndarray2)
|
||||
(2, 3)
|
||||
```
|
||||
|
||||
This is a rank 2 ndarray. Another attribute to check is the `dtype`, which is the data type. Checking the `dtype` for our ndarray gives us the following:
|
||||
|
||||
|
||||
```
|
||||
>>> my_ndarray.dtype
|
||||
dtype('int64')
|
||||
```
|
||||
|
||||
The `int64` means that our ndarray is made up of 64-bit integers. NumPy cannot create an ndarray of mixed types, and must contain only one type of element. If you define an ndarray containing a mix of element types, NumPy will automatically typecast all the elements to the highest element type available that can contain all the elements.
|
||||
|
||||
For example, creating a mix of `int`s and `float`s will create a `float64` ndarray.
|
||||
|
||||
|
||||
```
|
||||
>>> my_ndarray2 = np.array([1, 2.0, 3])
|
||||
>>> print(my_ndarray2)
|
||||
[1. 2. 3.]
|
||||
>>> my_ndarray2.dtype
|
||||
dtype('float64')
|
||||
```
|
||||
|
||||
Also, setting one of the elements as `string` will create string ndarray of `dtype` equal to `<U21`, meaning our ndarray contains unicode strings.
|
||||
|
||||
|
||||
```
|
||||
>>> my_ndarray2 = np.array([1, '2', 3])
|
||||
>>> print(my_ndarray2)
|
||||
['1' '2' '3']
|
||||
>>> my_ndarray2.dtype
|
||||
dtype('<U21')
|
||||
```
|
||||
|
||||
The `size` attribute will show the total number of elements that are present in our ndarray.
|
||||
|
||||
|
||||
```
|
||||
>>> my_ndarray = np.array([1, 2, 3, 4, 5])
|
||||
>>> my_ndarray.size
|
||||
5
|
||||
```
|
||||
|
||||
### Creating ndarrays using NumPy methods
|
||||
|
||||
There are several NumPy methods available for creating ndarrays in case you don’t want to create them directly using a list.
|
||||
|
||||
You can use `np.zeros()` to create an ndarray full of zeroes. It takes a shape as a parameter, which is a list containing the number of rows and columns. It can also take an optional `dtype` parameter which is the data type of the ndarray.
|
||||
|
||||
|
||||
```
|
||||
>>> my_ndarray = np.zeros([2,3], dtype=int)
|
||||
>>> print(my_ndarray)
|
||||
[[0 0 0]
|
||||
[0 0 0]]
|
||||
```
|
||||
|
||||
You can use `np.ones()` to create an ndarray full of ones.
|
||||
|
||||
|
||||
```
|
||||
>>> my_ndarray = np.ones([2,3], dtype=int)
|
||||
>>> print(my_ndarray)
|
||||
[[1 1 1]
|
||||
[1 1 1]]
|
||||
```
|
||||
|
||||
You can use `np.full()` to fill an ndarray with a specific value.
|
||||
|
||||
|
||||
```
|
||||
>>> my_ndarray = np.full([2,3], 10, dtype=int)
|
||||
>>> print(my_ndarray)
|
||||
[[10 10 10]
|
||||
[10 10 10]]
|
||||
```
|
||||
|
||||
You can use `np.eye()` to create an identity matrix/ndarray, which is a square matrix with ones all along the main diagonal. A square matrix is a matrix with the same number of rows and columns.
|
||||
|
||||
|
||||
```
|
||||
>>> my_ndarray = np.eye(3, dtype=int)
|
||||
>>> print(my_ndarray)
|
||||
[[1 0 0]
|
||||
[0 1 0]
|
||||
[0 0 1]]
|
||||
```
|
||||
|
||||
You can use `np.diag()` to create a matrix with the specified values along the diagonal, and zeroes in the rest of the matrix.
|
||||
|
||||
|
||||
```
|
||||
>>> my_ndarray = np.diag([10, 20, 30, 40, 50])
|
||||
>>> print(my_ndarray)
|
||||
[[10 0 0 0 0]
|
||||
[ 0 20 0 0 0]
|
||||
[ 0 0 30 0 0]
|
||||
[ 0 0 0 40 0]
|
||||
[ 0 0 0 0 50]]
|
||||
```
|
||||
|
||||
You can use `np.arange()` to create an ndarray with a specific range of values. It is used by specifying a start and end (exclusive) range of integers and a step size.
|
||||
|
||||
|
||||
```
|
||||
>>> my_ndarray = np.arange(1, 20, 3)
|
||||
>>> print(my_ndarray)
|
||||
[ 1 4 7 10 13 16 19]
|
||||
```
|
||||
|
||||
### Reading ndarrays
|
||||
|
||||
The values of an ndarray can be read using indexing, slicing, or boolean indexing.
|
||||
|
||||
#### Reading ndarrays using indexing
|
||||
|
||||
In indexing, you can read the values using the integer indices of the elements of the ndarray, much like you would read a Python list. Just like Python lists, the indices start from zero.
|
||||
|
||||
For example, in the ndarray defined as below:
|
||||
|
||||
|
||||
```
|
||||
>>> my_ndarray = np.arange(1, 20, 3)
|
||||
```
|
||||
|
||||
The fourth value will be `my_ndarray[3]`, or `10`. The last value will be `my_ndarray[-1]`, or `19`.
|
||||
|
||||
|
||||
```
|
||||
>>> my_ndarray = np.arange(1, 20, 3)
|
||||
>>> print(my_ndarray[0])
|
||||
1
|
||||
>>> print(my_ndarray[3])
|
||||
10
|
||||
>>> print(my_ndarray[-1])
|
||||
19
|
||||
>>> print(my_ndarray[5])
|
||||
16
|
||||
>>> print(my_ndarray[6])
|
||||
19
|
||||
```
|
||||
|
||||
#### Reading ndarrays using slicing
|
||||
|
||||
You can also use slicing to read chunks of the ndarray. Slicing works by specifying a start index and an end index using a colon (`:`) operator. Python will then fetch the slice of the ndarray between that start and end index.
|
||||
|
||||
|
||||
```
|
||||
>>> print(my_ndarray[:])
|
||||
[ 1 4 7 10 13 16 19]
|
||||
>>> print(my_ndarray[2:4])
|
||||
[ 7 10]
|
||||
>>> print(my_ndarray[5:6])
|
||||
[16]
|
||||
>>> print(my_ndarray[6:7])
|
||||
[19]
|
||||
>>> print(my_ndarray[:-1])
|
||||
[ 1 4 7 10 13 16]
|
||||
>>> print(my_ndarray[-1:])
|
||||
[19]
|
||||
```
|
||||
|
||||
Slicing creates a reference, or view, of an ndarray. This means that modifying the values in a slice will also change the values of the original ndarray.
|
||||
|
||||
For example:
|
||||
|
||||
|
||||
```
|
||||
>>> my_ndarray[-1:] = 100
|
||||
>>> print(my_ndarray)
|
||||
[ 1 4 7 10 13 16 100]
|
||||
```
|
||||
|
||||
For slicing ndarrays with rank more than 1, the `[row-start-index:row-end-index, column-start-index:column-end-index]` syntax can be used.
|
||||
|
||||
|
||||
```
|
||||
>>> my_ndarray2 = np.array([(1, 2, 3), (4, 5, 6)])
|
||||
>>> print(my_ndarray2)
|
||||
[[1 2 3]
|
||||
[4 5 6]]
|
||||
>>> print(my_ndarray2[0:2,1:3])
|
||||
[[2 3]
|
||||
[5 6]]
|
||||
```
|
||||
|
||||
#### Reading ndarrays using boolean indexing
|
||||
|
||||
Another way to read ndarrays is using boolean indexing. In this method, you specify a filtering condition within square brackets and a section of the ndarray that matches that criteria is returned.
|
||||
|
||||
For example, to get all the values in an ndarray greater than 5, you might specify a boolean indexing operation as `my_ndarray[my_ndarray > 5]`. This operation will return an ndarray that contains all values greater than 5.
|
||||
|
||||
|
||||
```
|
||||
>>> my_ndarray = np.array([1, 2, 3, 4, 5, 6, 7, 8, 9, 10])
|
||||
>>> my_ndarray2 = my_ndarray[my_ndarray > 5]
|
||||
>>> print(my_ndarray2)
|
||||
[ 6 7 8 9 10]
|
||||
```
|
||||
|
||||
For example, to get all the even values in an ndarray, you might use a boolean indexing operation as follows:
|
||||
|
||||
|
||||
```
|
||||
>>> my_ndarray2 = my_ndarray[my_ndarray % 2 == 0]
|
||||
>>> print(my_ndarray2)
|
||||
[ 2 4 6 8 10]
|
||||
```
|
||||
|
||||
And to get all the odd values, you might use this:
|
||||
|
||||
|
||||
```
|
||||
>>> my_ndarray2 = my_ndarray[my_ndarray % 2 == 1]
|
||||
>>> print(my_ndarray2)
|
||||
[1 3 5 7 9]
|
||||
```
|
||||
|
||||
### Vector and scalar arithmetic with ndarrays
|
||||
|
||||
NumPy ndarrays allow vector and scalar arithmetic operations. In vector arithmetic, an element-wise arithmetic operation is performed between two ndarrays. In scalar arithmetic, an arithmetic operation is performed between an ndarray and a constant scalar value.
|
||||
|
||||
Consider the two ndarrays below.
|
||||
|
||||
|
||||
```
|
||||
>>> my_ndarray = np.array([1, 2, 3, 4, 5])
|
||||
>>> my_ndarray2 = np.array([6, 7, 8, 9, 10])
|
||||
```
|
||||
|
||||
If you add the above two ndarrays, it would produce a new ndarray where each element of the two ndarrays would be added. For example, the first element of the resultant ndarray would be the result of adding the first elements of the original ndarrays, and so on.
|
||||
|
||||
|
||||
```
|
||||
>>> print(my_ndarray2 + my_ndarray)
|
||||
[ 7 9 11 13 15]
|
||||
```
|
||||
|
||||
Here, `7` is the sum of `1` and `6`, which are the first two elements of the ndarrays I've added together. Similarly, `15` is the sum of `5` and `10`, which are the last elements.
|
||||
|
||||
Consider the following arithmetic operations:
|
||||
|
||||
|
||||
```
|
||||
>>> print(my_ndarray2 - my_ndarray)
|
||||
[5 5 5 5 5]
|
||||
>>>
|
||||
>>> print(my_ndarray2 * my_ndarray)
|
||||
[ 6 14 24 36 50]
|
||||
>>>
|
||||
>>> print(my_ndarray2 / my_ndarray)
|
||||
[6. 3.5 2.66666667 2.25 2. ]
|
||||
```
|
||||
|
||||
Adding a scalar value to an ndarray has a similar effect—the scalar value is added to all the elements of the ndarray. This is called _broadcasting_.
|
||||
|
||||
|
||||
```
|
||||
>>> print(my_ndarray + 10)
|
||||
[11 12 13 14 15]
|
||||
>>>
|
||||
>>> print(my_ndarray - 10)
|
||||
[-9 -8 -7 -6 -5]
|
||||
>>>
|
||||
>>> print(my_ndarray * 10)
|
||||
[10 20 30 40 50]
|
||||
>>>
|
||||
>>> print(my_ndarray / 10)
|
||||
[0.1 0.2 0.3 0.4 0.5]
|
||||
```
|
||||
|
||||
### Sorting ndarrays
|
||||
|
||||
There are two ways available to sort ndarrays—in-place or out-of-place. In-place sorting sorts and modifies the original ndarray, and out-of-place sorting will return the sorted ndarray but not modify the original one. I'll try out both examples.
|
||||
|
||||
|
||||
```
|
||||
>>> my_ndarray = np.array([3, 1, 2, 5, 4])
|
||||
>>> my_ndarray.sort()
|
||||
>>> print(my_ndarray)
|
||||
[1 2 3 4 5]
|
||||
```
|
||||
|
||||
As you can see, the `sort()` method sorts the ndarray in-place and modifies the original array.
|
||||
|
||||
There is another method called `np.sort()` which sorts the array out of place.
|
||||
|
||||
|
||||
```
|
||||
>>> my_ndarray = np.array([3, 1, 2, 5, 4])
|
||||
>>> print(np.sort(my_ndarray))
|
||||
[1 2 3 4 5]
|
||||
>>> print(my_ndarray)
|
||||
[3 1 2 5 4]
|
||||
```
|
||||
|
||||
As you can see, the `np.sort()` method returns a sorted ndarray but does not modify it.
|
||||
|
||||
### Conclusion
|
||||
|
||||
I've covered quite a bit about NumPy and ndarrays. I talked about creating ndarrays, the different ways of reading them, basic vector and scalar arithmetic, and sorting. There is a lot more to explore with NumPy, including set operations like `union()` and `intersection()`, statistical operations like `min()` and `max()`, etc.
|
||||
|
||||
I hope the examples I demonstrated above were useful. Have fun exploring NumPy.
|
||||
|
||||
* * *
|
||||
|
||||
_This article was originally published on the [author's personal blog][3] and has been adapted with permission._
|
||||
|
||||
--------------------------------------------------------------------------------
|
||||
|
||||
via: https://opensource.com/article/21/9/python-numpy
|
||||
|
||||
作者:[Ayush Sharma][a]
|
||||
选题:[lujun9972][b]
|
||||
译者:[译者ID](https://github.com/译者ID)
|
||||
校对:[校对者ID](https://github.com/校对者ID)
|
||||
|
||||
本文由 [LCTT](https://github.com/LCTT/TranslateProject) 原创编译,[Linux中国](https://linux.cn/) 荣誉推出
|
||||
|
||||
[a]: https://opensource.com/users/ayushsharma
|
||||
[b]: https://github.com/lujun9972
|
||||
[1]: https://opensource.com/sites/default/files/styles/image-full-size/public/lead-images/math_money_financial_calculator_colors.jpg?itok=_yEVTST1 (old school calculator)
|
||||
[2]: https://notes.ayushsharma.in/2018/09/data-types-in-python
|
||||
[3]: https://notes.ayushsharma.in/2018/10/working-with-numpy-in-python
|
425
translated/tech/20210916 Crunch numbers in Python with NumPy.md
Normal file
425
translated/tech/20210916 Crunch numbers in Python with NumPy.md
Normal file
@ -0,0 +1,425 @@
|
||||
[#]: subject: "Crunch numbers in Python with NumPy"
|
||||
[#]: via: "https://opensource.com/article/21/9/python-numpy"
|
||||
[#]: author: "Ayush Sharma https://opensource.com/users/ayushsharma"
|
||||
[#]: collector: "lujun9972"
|
||||
[#]: translator: "wxy"
|
||||
[#]: reviewer: " "
|
||||
[#]: publisher: " "
|
||||
[#]: url: " "
|
||||
|
||||
用 NumPy 在 Python 中处理数字
|
||||
======
|
||||
|
||||
> 这篇文章讨论了安装 NumPy,然后创建、读取和排序 NumPy 数组。
|
||||
|
||||
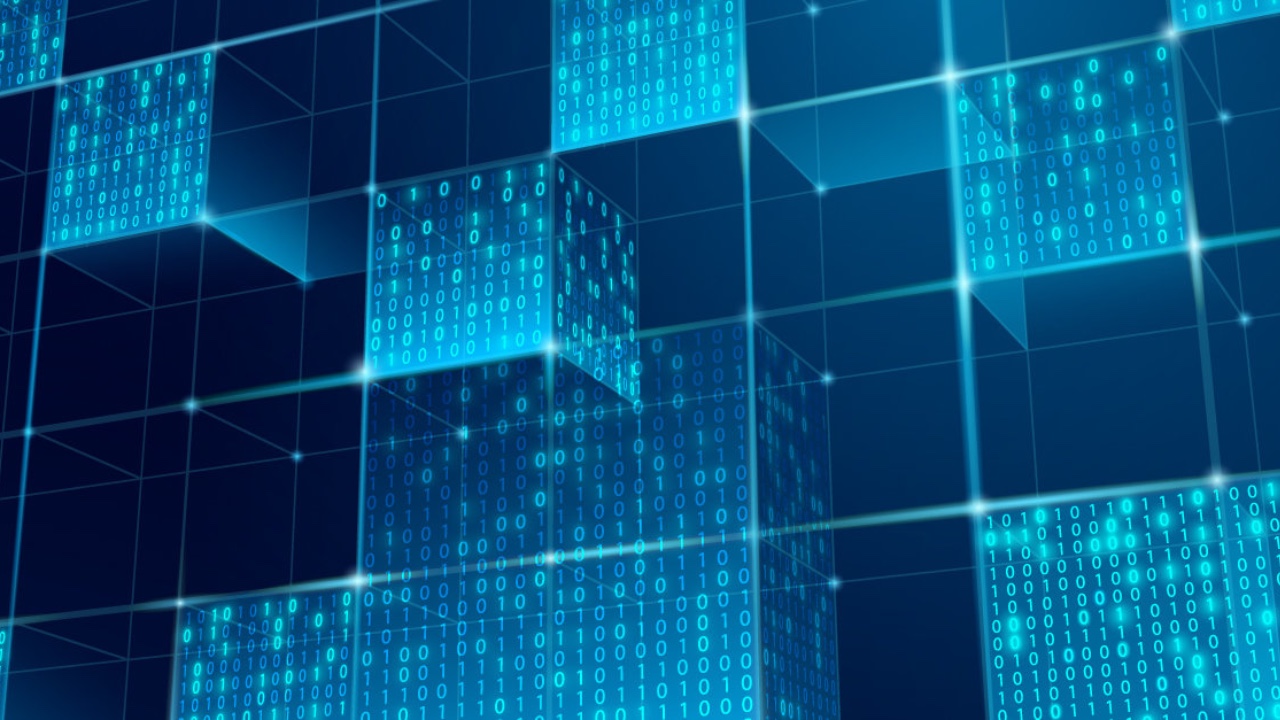
|
||||
|
||||
NumPy(即 **Num**erical **Py**thon)是一个库,它使得在 Python 中对线性数列和矩阵进行统计和集合操作变得容易。[我在 Python 数据类型的笔记中介绍过][2],它比 Python 的列表快几个数量级。NumPy 在数据分析和科学计算中使用得相当频繁。
|
||||
|
||||
我将介绍安装 NumPy,然后创建、读取和排序 NumPy 数组。NumPy 数组也被称为 ndarray,即 N 维数组的缩写。
|
||||
|
||||
### 安装 NumPy
|
||||
|
||||
使用 `pip` 安装 NumPy 包非常简单,可以像安装其他软件包一样进行安装:
|
||||
|
||||
```
|
||||
pip install numpy
|
||||
```
|
||||
|
||||
安装了 NumPy 包后,只需将其导入你的 Python 文件中:
|
||||
|
||||
```
|
||||
import numpy as np
|
||||
```
|
||||
|
||||
将 `numpy` 以 `np` 之名导入是一个标准的惯例,但你可以不使用 `np`,而是使用你想要的任何其他别名。
|
||||
|
||||
### 为什么使用 NumPy? 因为它比 Python 列表要快好几个数量级
|
||||
|
||||
当涉及到处理大量的数值时,NumPy 比普通的 Python 列表快几个数量级。为了看看它到底有多快,我首先测量在普通 Python 列表上进行 `min()` 和 `max()` 操作的时间。
|
||||
|
||||
我将首先创建一个具有 999,999,999 项的 Python 列表:
|
||||
|
||||
```
|
||||
>>> my_list = range(1, 1000000000)
|
||||
>>> len(my_list)
|
||||
999999999
|
||||
```
|
||||
|
||||
现在我将测量在这个列表中找到最小值的时间:
|
||||
|
||||
```
|
||||
>>> start = time.time()
|
||||
>>> min(my_list)
|
||||
1
|
||||
>>> print('Time elapsed in milliseconds: ' + str((time.time() - start) * 1000))
|
||||
Time elapsed in milliseconds: 27007.00879096985
|
||||
```
|
||||
|
||||
这花了大约 27,007 毫秒,也就是大约 **27 秒**。这是个很长的时间。现在我试着找出寻找最大值的时间:
|
||||
|
||||
```
|
||||
>>> start = time.time()
|
||||
>>> max(my_list)
|
||||
999999999
|
||||
>>> print('Time elapsed in milliseconds: ' + str((time.time() - start) * 1000))
|
||||
Time elapsed in milliseconds: 28111.071348190308
|
||||
```
|
||||
|
||||
这花了大约 28,111 毫秒,也就是大约 **28 秒**。
|
||||
|
||||
现在我试试用 NumPy 找到最小值和最大值的时间:
|
||||
|
||||
```
|
||||
>>> my_list = np.arange(1, 1000000000)
|
||||
>>> len(my_list)
|
||||
999999999
|
||||
>>> start = time.time()
|
||||
>>> my_list.min()
|
||||
1
|
||||
>>> print('Time elapsed in milliseconds: ' + str((time.time() - start) * 1000))
|
||||
Time elapsed in milliseconds: 1151.1778831481934
|
||||
>>>
|
||||
>>> start = time.time()
|
||||
>>> my_list.max()
|
||||
999999999
|
||||
>>> print('Time elapsed in milliseconds: ' + str((time.time() - start) * 1000))
|
||||
Time elapsed in milliseconds: 1114.8970127105713
|
||||
```
|
||||
|
||||
找到最小值花了大约 1151 毫秒,找到最大值 1114 毫秒。这大约是 **1 秒**。
|
||||
|
||||
正如你所看到的,使用 NumPy 可以将寻找一个大约有 10 亿个值的列表的最小值和最大值的时间 **从大约 28 秒减少到 1 秒**。这就是 NumPy 的强大之处。
|
||||
|
||||
### 使用 Python 列表创建 ndarray
|
||||
|
||||
有几种方法可以在 NumPy 中创建 ndarray。
|
||||
|
||||
你可以通过使用元素列表来创建一个 ndarray:
|
||||
|
||||
```
|
||||
>>> my_ndarray = np.array([1, 2, 3, 4, 5])
|
||||
>>> print(my_ndarray)
|
||||
[1 2 3 4 5]
|
||||
```
|
||||
|
||||
有了上面的 ndarray 定义,我将检查几件事。首先,上面定义的变量的类型是 `numpy.ndarray`。这是所有 NumPy ndarray 的类型:
|
||||
|
||||
```
|
||||
>>> type(my_ndarray)
|
||||
<class 'numpy.ndarray'>
|
||||
```
|
||||
|
||||
这里要注意的另一件事是 “<ruby>形状<rt>shape</rt></ruby>”。ndarray 的形状是 ndarray 的每个维度的长度。你可以看到,`my_ndarray` 的形状是 `(5,)`。这意味着 `my_ndarray` 包含一个有 5 个元素的维度(轴)。
|
||||
|
||||
```
|
||||
>>> np.shape(my_ndarray)
|
||||
(5,)
|
||||
```
|
||||
|
||||
数组中的维数被称为它的 “<ruby>秩<rt>rank</rt></ruby>”。所以上面的 ndarray 的秩是 1。
|
||||
|
||||
我将定义另一个 ndarray `my_ndarray2` 作为一个多维 ndarray。那么它的形状会是什么呢?请看下面:
|
||||
|
||||
```
|
||||
>>> my_ndarray2 = np.array([(1, 2, 3), (4, 5, 6)])
|
||||
>>> np.shape(my_ndarray2)
|
||||
(2, 3)
|
||||
```
|
||||
|
||||
这是一个秩为 2 的 ndarray。另一个要检查的属性是 `dtype`,也就是数据类型。检查我们的 ndarray 的 `dtype` 可以得到以下结果:
|
||||
|
||||
```
|
||||
>>> my_ndarray.dtype
|
||||
dtype('int64')
|
||||
```
|
||||
|
||||
`int64` 意味着我们的 ndarray 是由 64 位整数组成的。NumPy 不能创建混合类型的 ndarray,必须只包含一种类型的元素。如果你定义了一个包含混合元素类型的 ndarray,NumPy 会自动将所有的元素类型转换为可以包含所有元素的最高元素类型。
|
||||
|
||||
例如,创建一个 `int` 和 `float` 的混合序列将创建一个 `float64` 的 ndarray:
|
||||
|
||||
```
|
||||
>>> my_ndarray2 = np.array([1, 2.0, 3])
|
||||
>>> print(my_ndarray2)
|
||||
[1. 2. 3.]
|
||||
>>> my_ndarray2.dtype
|
||||
dtype('float64')
|
||||
```
|
||||
|
||||
另外,将其中一个元素设置为 `string` 将创建 `dtype` 等于 `<U21` 的字符串 ndarray,意味着我们的 ndarray 包含 unicode 字符串:
|
||||
|
||||
```
|
||||
>>> my_ndarray2 = np.array([1, '2', 3])
|
||||
>>> print(my_ndarray2)
|
||||
['1' '2' '3']
|
||||
>>> my_ndarray2.dtype
|
||||
dtype('<U21')
|
||||
```
|
||||
|
||||
`size` 属性将显示我们的 ndarray 中存在的元素总数:
|
||||
|
||||
```
|
||||
>>> my_ndarray = np.array([1, 2, 3, 4, 5])
|
||||
>>> my_ndarray.size
|
||||
5
|
||||
```
|
||||
|
||||
### 使用 NumPy 方法创建 ndarray
|
||||
|
||||
如果你不想直接使用列表来创建 ndarray,还有几种可以用来创建它的 NumPy 方法。
|
||||
|
||||
你可以使用 `np.zeros()` 来创建一个填满 0 的 ndarray。它需要一个“形状”作为参数,这是一个包含行数和列数的列表。它还可以接受一个可选的 `dtype` 参数,这是 ndarray 的数据类型:
|
||||
|
||||
```
|
||||
>>> my_ndarray = np.zeros([2,3], dtype=int)
|
||||
>>> print(my_ndarray)
|
||||
[[0 0 0]
|
||||
[0 0 0]]
|
||||
```
|
||||
|
||||
你可以使用 `np. ones()` 来创建一个填满 `1` 的 ndarray:
|
||||
|
||||
```
|
||||
>>> my_ndarray = np.ones([2,3], dtype=int)
|
||||
>>> print(my_ndarray)
|
||||
[[1 1 1]
|
||||
[1 1 1]]
|
||||
```
|
||||
|
||||
你可以使用 `np.full()` 来给 ndarray 填充一个特定的值:
|
||||
|
||||
```
|
||||
>>> my_ndarray = np.full([2,3], 10, dtype=int)
|
||||
>>> print(my_ndarray)
|
||||
[[10 10 10]
|
||||
[10 10 10]]
|
||||
```
|
||||
|
||||
你可以使用 `np.eye()` 来创建一个单位矩阵 / ndarray,这是一个沿主对角线都是 `1` 的正方形矩阵。正方形矩阵是一个行数和列数相同的矩阵:
|
||||
|
||||
```
|
||||
>>> my_ndarray = np.eye(3, dtype=int)
|
||||
>>> print(my_ndarray)
|
||||
[[1 0 0]
|
||||
[0 1 0]
|
||||
[0 0 1]]
|
||||
```
|
||||
|
||||
你可以使用 `np.diag()` 来创建一个沿对角线有指定数值的矩阵,而在矩阵的其他部分为 `0`:
|
||||
|
||||
```
|
||||
>>> my_ndarray = np.diag([10, 20, 30, 40, 50])
|
||||
>>> print(my_ndarray)
|
||||
[[10 0 0 0 0]
|
||||
[ 0 20 0 0 0]
|
||||
[ 0 0 30 0 0]
|
||||
[ 0 0 0 40 0]
|
||||
[ 0 0 0 0 50]]
|
||||
```
|
||||
|
||||
你可以使用 `np.range()` 来创建一个具有特定数值范围的 ndarray。它是通过指定一个整数的开始和结束(不包括)范围以及一个步长来创建的:
|
||||
|
||||
```
|
||||
>>> my_ndarray = np.arange(1, 20, 3)
|
||||
>>> print(my_ndarray)
|
||||
[ 1 4 7 10 13 16 19]
|
||||
```
|
||||
|
||||
### 读取 ndarray
|
||||
|
||||
ndarray 的值可以使用索引、分片或布尔索引来读取。
|
||||
|
||||
#### 使用索引读取 ndarray 的值
|
||||
|
||||
在索引中,你可以使用 ndarray 的元素的整数索引来读取数值,就像你读取 Python 列表一样。就像 Python 列表一样,索引从 `0` 开始。
|
||||
|
||||
例如,在定义如下的 ndarray 中:
|
||||
|
||||
```
|
||||
>>> my_ndarray = np.arange(1, 20, 3)
|
||||
```
|
||||
|
||||
第四个值将是 `my_ndarray[3]`,即 `10`。最后一个值是 `my_ndarray[-1]`,即 `19`:
|
||||
|
||||
```
|
||||
>>> my_ndarray = np.arange(1, 20, 3)
|
||||
>>> print(my_ndarray[0])
|
||||
1
|
||||
>>> print(my_ndarray[3])
|
||||
10
|
||||
>>> print(my_ndarray[-1])
|
||||
19
|
||||
>>> print(my_ndarray[5])
|
||||
16
|
||||
>>> print(my_ndarray[6])
|
||||
19
|
||||
```
|
||||
|
||||
#### 使用分片读取 ndarray
|
||||
|
||||
你也可以使用分片来读取 ndarray 的块。分片的工作方式是用冒号(`:`)操作符指定一个开始索引和一个结束索引。然后,Python 将获取该开始和结束索引之间的 ndarray 片断:
|
||||
|
||||
```
|
||||
>>> print(my_ndarray[:])
|
||||
[ 1 4 7 10 13 16 19]
|
||||
>>> print(my_ndarray[2:4])
|
||||
[ 7 10]
|
||||
>>> print(my_ndarray[5:6])
|
||||
[16]
|
||||
>>> print(my_ndarray[6:7])
|
||||
[19]
|
||||
>>> print(my_ndarray[:-1])
|
||||
[ 1 4 7 10 13 16]
|
||||
>>> print(my_ndarray[-1:])
|
||||
[19]
|
||||
```
|
||||
|
||||
分片创建了一个 ndarray 的引用(或视图)。这意味着,修改分片中的值也会改变原始 ndarray 的值。
|
||||
|
||||
比如说:
|
||||
|
||||
```
|
||||
>>> my_ndarray[-1:] = 100
|
||||
>>> print(my_ndarray)
|
||||
[ 1 4 7 10 13 16 100]
|
||||
```
|
||||
|
||||
对于秩超过 1 的 ndarray 的分片,可以使用 `[行开始索引:行结束索引, 列开始索引:列结束索引]` 语法:
|
||||
|
||||
```
|
||||
>>> my_ndarray2 = np.array([(1, 2, 3), (4, 5, 6)])
|
||||
>>> print(my_ndarray2)
|
||||
[[1 2 3]
|
||||
[4 5 6]]
|
||||
>>> print(my_ndarray2[0:2,1:3])
|
||||
[[2 3]
|
||||
[5 6]]
|
||||
```
|
||||
|
||||
#### 使用布尔索引读取 ndarray 的方法
|
||||
|
||||
读取 ndarray 的另一种方法是使用布尔索引。在这种方法中,你在方括号内指定一个过滤条件,然后返回符合该条件的 ndarray 的一个部分。
|
||||
|
||||
例如,为了获得一个 ndarray 中所有大于 5 的值,你可以指定布尔索引操作 `my_ndarray[my_ndarray > 5]`。这个操作将返回一个包含所有大于 5 的值的 ndarray:
|
||||
|
||||
```
|
||||
>>> my_ndarray = np.array([1, 2, 3, 4, 5, 6, 7, 8, 9, 10])
|
||||
>>> my_ndarray2 = my_ndarray[my_ndarray > 5]
|
||||
>>> print(my_ndarray2)
|
||||
[ 6 7 8 9 10]
|
||||
```
|
||||
|
||||
例如,为了获得一个 ndarray 中的所有偶数值,你可以使用如下的布尔索引操作:
|
||||
|
||||
```
|
||||
>>> my_ndarray2 = my_ndarray[my_ndarray % 2 == 0]
|
||||
>>> print(my_ndarray2)
|
||||
[ 2 4 6 8 10]
|
||||
```
|
||||
|
||||
而要得到所有的奇数值,你可以用这个方法:
|
||||
|
||||
```
|
||||
>>> my_ndarray2 = my_ndarray[my_ndarray % 2 == 1]
|
||||
>>> print(my_ndarray2)
|
||||
[1 3 5 7 9]
|
||||
```
|
||||
|
||||
### ndarray 的矢量和标量算术
|
||||
|
||||
NumPy 的 ndarray 允许进行矢量和标量算术操作。在矢量算术中,在两个 ndarray 之间进行一个元素的算术操作。在标量算术中,算术运算是在一个 ndarray 和一个常数标量值之间进行的。
|
||||
|
||||
如下的两个 ndarray:
|
||||
|
||||
```
|
||||
>>> my_ndarray = np.array([1, 2, 3, 4, 5])
|
||||
>>> my_ndarray2 = np.array([6, 7, 8, 9, 10])
|
||||
```
|
||||
|
||||
如果你将上述两个 ndarray 相加,就会产生一个两个 ndarray 的元素相加的新的 ndarray。例如,产生的 ndarray 的第一个元素将是原始 ndarray 的第一个元素相加的结果,以此类推:
|
||||
|
||||
```
|
||||
>>> print(my_ndarray2 + my_ndarray)
|
||||
[ 7 9 11 13 15]
|
||||
```
|
||||
|
||||
这里,`7` 是 `1` 和 `6` 的和,这是我相加的 ndarray 中的前两个元素。同样,`15` 是 `5` 和`10` 之和,是最后一个元素。
|
||||
|
||||
请看以下算术运算:
|
||||
|
||||
```
|
||||
>>> print(my_ndarray2 - my_ndarray)
|
||||
[5 5 5 5 5]
|
||||
>>>
|
||||
>>> print(my_ndarray2 * my_ndarray)
|
||||
[ 6 14 24 36 50]
|
||||
>>>
|
||||
>>> print(my_ndarray2 / my_ndarray)
|
||||
[6. 3.5 2.66666667 2.25 2. ]
|
||||
```
|
||||
|
||||
在 ndarray 中加一个标量值也有类似的效果,标量值被添加到 ndarray 的所有元素中。这被称为“<ruby>广播<rt>broadcasting</rt></ruby>”:
|
||||
|
||||
```
|
||||
>>> print(my_ndarray + 10)
|
||||
[11 12 13 14 15]
|
||||
>>>
|
||||
>>> print(my_ndarray - 10)
|
||||
[-9 -8 -7 -6 -5]
|
||||
>>>
|
||||
>>> print(my_ndarray * 10)
|
||||
[10 20 30 40 50]
|
||||
>>>
|
||||
>>> print(my_ndarray / 10)
|
||||
[0.1 0.2 0.3 0.4 0.5]
|
||||
```
|
||||
|
||||
### ndarray 的排序
|
||||
|
||||
有两种方法可以对 ndarray 进行原地或非原地排序。原地排序会对原始 ndarray 进行排序和修改,而非原地排序会返回排序后的 ndarray,但不会修改原始 ndarray。我将尝试这两个例子:
|
||||
|
||||
```
|
||||
>>> my_ndarray = np.array([3, 1, 2, 5, 4])
|
||||
>>> my_ndarray.sort()
|
||||
>>> print(my_ndarray)
|
||||
[1 2 3 4 5]
|
||||
```
|
||||
|
||||
正如你所看到的,`sort()` 方法对 ndarray 进行原地排序,并修改了原数组。
|
||||
|
||||
还有一个方法叫 `np.sort()`,它对数组进行非原地排序:
|
||||
|
||||
```
|
||||
>>> my_ndarray = np.array([3, 1, 2, 5, 4])
|
||||
>>> print(np.sort(my_ndarray))
|
||||
[1 2 3 4 5]
|
||||
>>> print(my_ndarray)
|
||||
[3 1 2 5 4]
|
||||
```
|
||||
|
||||
正如你所看到的,`np.sort()` 方法返回一个已排序的 ndarray,但没有修改它。
|
||||
|
||||
### 总结
|
||||
|
||||
我已经介绍了很多关于 NumPy 和 ndarray 的内容。我谈到了创建 ndarray,读取它们的不同方法,基本的向量和标量算术,以及排序。NumPy 还有很多东西可以探索,包括像 `union()` 和 `intersection()`这样的集合操作,像 `min()` 和 `max()` 这样的统计操作,等等。
|
||||
|
||||
我希望我上面演示的例子是有用的。祝你在探索 NumPy 时愉快。
|
||||
|
||||
本文最初发表于 [作者的个人博客][3],经授权后改编。
|
||||
|
||||
--------------------------------------------------------------------------------
|
||||
|
||||
via: https://opensource.com/article/21/9/python-numpy
|
||||
|
||||
作者:[Ayush Sharma][a]
|
||||
选题:[lujun9972][b]
|
||||
译者:[wxy](https://github.com/wxy)
|
||||
校对:[wxy](https://github.com/wxy)
|
||||
|
||||
本文由 [LCTT](https://github.com/LCTT/TranslateProject) 原创编译,[Linux中国](https://linux.cn/) 荣誉推出
|
||||
|
||||
[a]: https://opensource.com/users/ayushsharma
|
||||
[b]: https://github.com/lujun9972
|
||||
[1]: https://opensource.com/sites/default/files/styles/image-full-size/public/lead-images/math_money_financial_calculator_colors.jpg?itok=_yEVTST1 (old school calculator)
|
||||
[2]: https://notes.ayushsharma.in/2018/09/data-types-in-python
|
||||
[3]: https://notes.ayushsharma.in/2018/10/working-with-numpy-in-python
|
Loading…
Reference in New Issue
Block a user