mirror of
https://github.com/LCTT/TranslateProject.git
synced 2025-01-13 22:30:37 +08:00
translated
This commit is contained in:
parent
f52358ca0d
commit
87fb3dc8cc
@ -1,258 +0,0 @@
|
|||||||
heguangzhi Translating
|
|
||||||
|
|
||||||
9 obscure Python libraries for data science
|
|
||||||
======
|
|
||||||
Go beyond pandas, scikit-learn, and matplotlib and learn some new tricks for doing data science in Python.
|
|
||||||
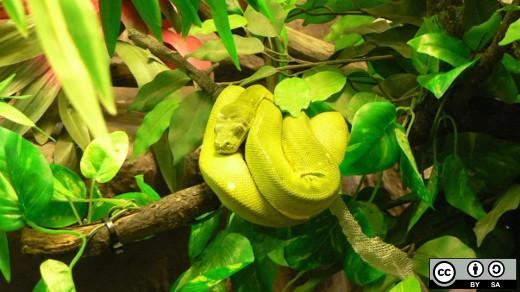
|
|
||||||
Python is an amazing language. In fact, it's one of the fastest growing programming languages in the world. It has time and again proved its usefulness both in developer job roles and data science positions across industries. The entire ecosystem of Python and its libraries makes it an apt choice for users (beginners and advanced) all over the world. One of the reasons for its success and popularity is its set of robust libraries that make it so dynamic and fast.
|
|
||||||
|
|
||||||
In this article, we will look at some of the Python libraries for data science tasks other than the commonly used ones like **pandas, scikit-learn** , and **matplotlib**. Although libraries like **pandas and scikit-learn** are the ones that come to mind for machine learning tasks, it's always good to learn about other Python offerings in this field.
|
|
||||||
|
|
||||||
### Wget
|
|
||||||
|
|
||||||
Extracting data, especially from the web, is one of a data scientist's vital tasks. [Wget][1] is a free utility for non-interactive downloading files from the web. It supports HTTP, HTTPS, and FTP protocols, as well as retrieval through HTTP proxies. Since it is non-interactive, it can work in the background even if the user isn't logged in. So the next time you want to download a website or all the images from a page, **wget** will be there to assist.
|
|
||||||
|
|
||||||
#### Installation
|
|
||||||
|
|
||||||
```
|
|
||||||
$ pip install wget
|
|
||||||
```
|
|
||||||
|
|
||||||
#### Example
|
|
||||||
|
|
||||||
```
|
|
||||||
import wget
|
|
||||||
url = 'http://www.futurecrew.com/skaven/song_files/mp3/razorback.mp3'
|
|
||||||
|
|
||||||
filename = wget.download(url)
|
|
||||||
100% [................................................] 3841532 / 3841532
|
|
||||||
|
|
||||||
filename
|
|
||||||
'razorback.mp3'
|
|
||||||
```
|
|
||||||
|
|
||||||
### Pendulum
|
|
||||||
|
|
||||||
For people who get frustrated when working with date-times in Python, **[Pendulum][2]** is here. It is a Python package to ease **datetime** manipulations. It is a drop-in replacement for Python's native class. Refer to the [documentation][3] for in-depth information.
|
|
||||||
|
|
||||||
#### Installation
|
|
||||||
|
|
||||||
```
|
|
||||||
$ pip install pendulum
|
|
||||||
```
|
|
||||||
|
|
||||||
#### Example
|
|
||||||
|
|
||||||
```
|
|
||||||
import pendulum
|
|
||||||
|
|
||||||
dt_toronto = pendulum.datetime(2012, 1, 1, tz='America/Toronto')
|
|
||||||
dt_vancouver = pendulum.datetime(2012, 1, 1, tz='America/Vancouver')
|
|
||||||
|
|
||||||
print(dt_vancouver.diff(dt_toronto).in_hours())
|
|
||||||
|
|
||||||
3
|
|
||||||
```
|
|
||||||
|
|
||||||
### Imbalanced-learn
|
|
||||||
|
|
||||||
Most classification algorithms work best when the number of samples in each class is almost the same (i.e., balanced). But real-life cases are full of imbalanced datasets, which can have a bearing upon the learning phase and the subsequent prediction of machine learning algorithms. Fortunately, the **[imbalanced-learn][4]** library was created to address this issue. It is compatible with [**scikit-learn**][5] and is part of **[scikit-learn-contrib][6]** projects. Try it the next time you encounter imbalanced datasets.
|
|
||||||
|
|
||||||
#### Installation
|
|
||||||
|
|
||||||
```
|
|
||||||
pip install -U imbalanced-learn
|
|
||||||
|
|
||||||
# or
|
|
||||||
|
|
||||||
conda install -c conda-forge imbalanced-learn
|
|
||||||
```
|
|
||||||
|
|
||||||
#### Example
|
|
||||||
|
|
||||||
For usage and examples refer to the [documentation][7].
|
|
||||||
|
|
||||||
### FlashText
|
|
||||||
|
|
||||||
Cleaning text data during natural language processing (NLP) tasks often requires replacing keywords in or extracting keywords from sentences. Usually, such operations can be accomplished with regular expressions, but they can become cumbersome if the number of terms to be searched runs into the thousands.
|
|
||||||
|
|
||||||
Python's **[FlashText][8]** module, which is based upon the [FlashText algorithm][9], provides an apt alternative for such situations. The best part of FlashText is the runtime is the same irrespective of the number of search terms. You can read more about it in the [documentation][10].
|
|
||||||
|
|
||||||
#### Installation
|
|
||||||
|
|
||||||
```
|
|
||||||
$ pip install flashtext
|
|
||||||
```
|
|
||||||
|
|
||||||
#### Examples
|
|
||||||
|
|
||||||
##### **Extract keywords:**
|
|
||||||
|
|
||||||
```
|
|
||||||
from flashtext import KeywordProcessor
|
|
||||||
keyword_processor = KeywordProcessor()
|
|
||||||
|
|
||||||
# keyword_processor.add_keyword(<unclean name>, <standardised name>)
|
|
||||||
|
|
||||||
keyword_processor.add_keyword('Big Apple', 'New York')
|
|
||||||
keyword_processor.add_keyword('Bay Area')
|
|
||||||
keywords_found = keyword_processor.extract_keywords('I love Big Apple and Bay Area.')
|
|
||||||
|
|
||||||
keywords_found
|
|
||||||
['New York', 'Bay Area']
|
|
||||||
```
|
|
||||||
|
|
||||||
**Replace keywords:**
|
|
||||||
|
|
||||||
```
|
|
||||||
keyword_processor.add_keyword('New Delhi', 'NCR region')
|
|
||||||
|
|
||||||
new_sentence = keyword_processor.replace_keywords('I love Big Apple and new delhi.')
|
|
||||||
|
|
||||||
new_sentence
|
|
||||||
'I love New York and NCR region.'
|
|
||||||
```
|
|
||||||
|
|
||||||
For more examples, refer to the [usage][11] section in the documentation.
|
|
||||||
|
|
||||||
### FuzzyWuzzy
|
|
||||||
|
|
||||||
The name sounds weird, but **[FuzzyWuzzy][12]** is a very helpful library when it comes to string matching. It can easily implement operations like string comparison ratios, token ratios, etc. It is also handy for matching records kept in different databases.
|
|
||||||
|
|
||||||
#### Installation
|
|
||||||
|
|
||||||
```
|
|
||||||
$ pip install fuzzywuzzy
|
|
||||||
```
|
|
||||||
|
|
||||||
#### Example
|
|
||||||
|
|
||||||
```
|
|
||||||
from fuzzywuzzy import fuzz
|
|
||||||
from fuzzywuzzy import process
|
|
||||||
|
|
||||||
# Simple Ratio
|
|
||||||
|
|
||||||
fuzz.ratio("this is a test", "this is a test!")
|
|
||||||
97
|
|
||||||
|
|
||||||
# Partial Ratio
|
|
||||||
fuzz.partial_ratio("this is a test", "this is a test!")
|
|
||||||
100
|
|
||||||
```
|
|
||||||
|
|
||||||
More examples can be found in FuzzyWuzzy's [GitHub repo.][12]
|
|
||||||
|
|
||||||
### PyFlux
|
|
||||||
|
|
||||||
Time-series analysis is one of the most frequently encountered problems in machine learning. **[PyFlux][13]** is an open source library in Python that was explicitly built for working with time-series problems. The library has an excellent array of modern time-series models, including but not limited to **ARIMA** , **GARCH** , and **VAR** models. In short, PyFlux offers a probabilistic approach to time-series modeling. It's worth trying out.
|
|
||||||
|
|
||||||
#### Installation
|
|
||||||
|
|
||||||
```
|
|
||||||
pip install pyflux
|
|
||||||
```
|
|
||||||
|
|
||||||
#### Example
|
|
||||||
|
|
||||||
Please refer to the [documentation][14] for usage and examples.
|
|
||||||
|
|
||||||
### IPyvolume
|
|
||||||
|
|
||||||
Communicating results is an essential aspect of data science, and visualizing results offers a significant advantage. **[**IPyvolume**][15]** is a Python library to visualize 3D volumes and glyphs (e.g., 3D scatter plots) in the Jupyter notebook with minimal configuration and effort. However, it is currently in the pre-1.0 stage. A good analogy would be something like this: IPyvolume's **volshow** is to 3D arrays what matplotlib's **imshow** is to 2D arrays. You can read more about it in the [documentation][16].
|
|
||||||
|
|
||||||
#### Installation
|
|
||||||
|
|
||||||
```
|
|
||||||
Using pip
|
|
||||||
$ pip install ipyvolume
|
|
||||||
|
|
||||||
Conda/Anaconda
|
|
||||||
$ conda install -c conda-forge ipyvolume
|
|
||||||
```
|
|
||||||
|
|
||||||
#### Examples
|
|
||||||
|
|
||||||
**Animation:**
|
|
||||||
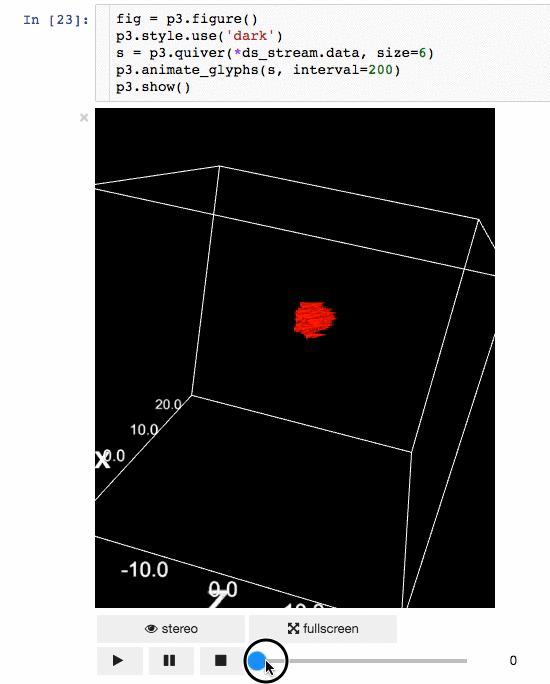
|
|
||||||
|
|
||||||
**Volume rendering:**
|
|
||||||
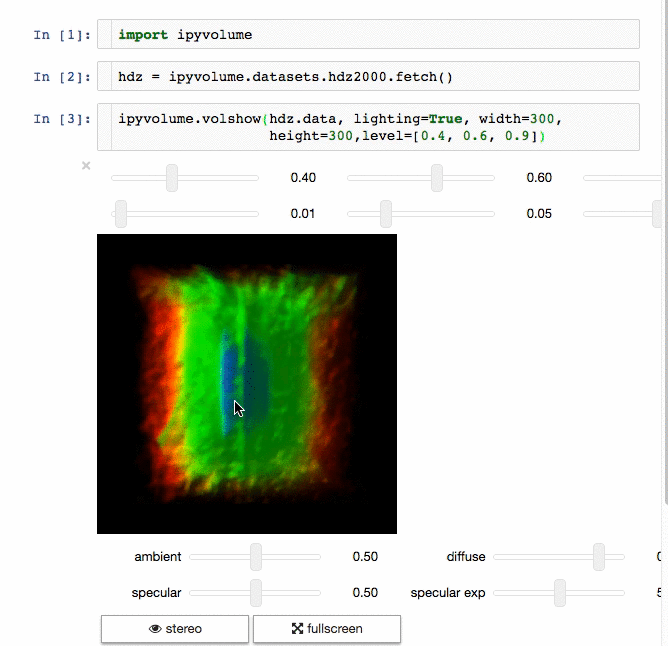
|
|
||||||
|
|
||||||
### Dash
|
|
||||||
|
|
||||||
**[Dash][17]** is a productive Python framework for building web applications. It is written on top of Flask, Plotly.js, and React.js and ties modern UI elements like drop-downs, sliders, and graphs to your analytical Python code without the need for JavaScript. Dash is highly suitable for building data visualization apps that can be rendered in the web browser. Consult the [user guide][18] for more details.
|
|
||||||
|
|
||||||
#### Installation
|
|
||||||
|
|
||||||
```
|
|
||||||
pip install dash==0.29.0 # The core dash backend
|
|
||||||
pip install dash-html-components==0.13.2 # HTML components
|
|
||||||
pip install dash-core-components==0.36.0 # Supercharged components
|
|
||||||
pip install dash-table==3.1.3 # Interactive DataTable component (new!)
|
|
||||||
```
|
|
||||||
|
|
||||||
#### Example
|
|
||||||
|
|
||||||
The following example shows a highly interactive graph with drop-down capabilities. As the user selects a value in the drop-down, the application code dynamically exports data from Google Finance into a Pandas DataFrame.
|
|
||||||
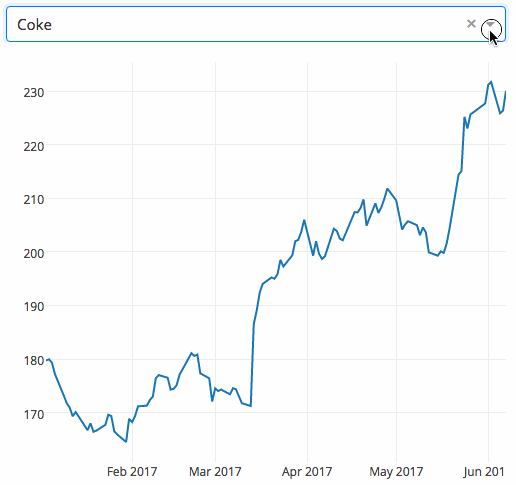
|
|
||||||
|
|
||||||
### Gym
|
|
||||||
|
|
||||||
**[Gym][19]** from [OpenAI][20] is a toolkit for developing and comparing reinforcement learning algorithms. It is compatible with any numerical computation library, such as TensorFlow or Theano. The Gym library is a collection of test problems, also called environments, that you can use to work out your reinforcement-learning algorithms. These environments have a shared interface, which allows you to write general algorithms.
|
|
||||||
|
|
||||||
#### Installation
|
|
||||||
|
|
||||||
```
|
|
||||||
pip install gym
|
|
||||||
```
|
|
||||||
|
|
||||||
#### Example
|
|
||||||
|
|
||||||
The following example will run an instance of the environment **[CartPole-v0][21]** for 1,000 timesteps, rendering the environment at each step.
|
|
||||||
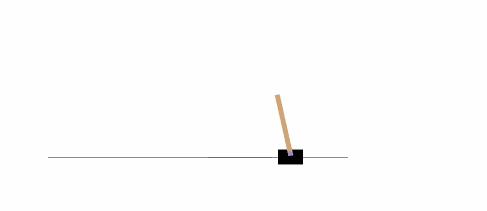
|
|
||||||
|
|
||||||
You can read about [other environments][22] on the Gym website.
|
|
||||||
|
|
||||||
### Conclusion
|
|
||||||
|
|
||||||
These are my picks for useful, but little-known Python libraries for data science. If you know another one to add to this list, please mention it in the comments below.
|
|
||||||
|
|
||||||
This was originally published on the [Analytics Vidhya][23] Medium channel and is reprinted with permission.
|
|
||||||
|
|
||||||
--------------------------------------------------------------------------------
|
|
||||||
|
|
||||||
via: https://opensource.com/article/18/11/python-libraries-data-science
|
|
||||||
|
|
||||||
作者:[Parul Pandey][a]
|
|
||||||
选题:[lujun9972][b]
|
|
||||||
译者:[译者ID](https://github.com/译者ID)
|
|
||||||
校对:[校对者ID](https://github.com/校对者ID)
|
|
||||||
|
|
||||||
本文由 [LCTT](https://github.com/LCTT/TranslateProject) 原创编译,[Linux中国](https://linux.cn/) 荣誉推出
|
|
||||||
|
|
||||||
[a]: https://opensource.com/users/parul-pandey
|
|
||||||
[b]: https://github.com/lujun9972
|
|
||||||
[1]: https://pypi.org/project/wget/
|
|
||||||
[2]: https://github.com/sdispater/pendulum
|
|
||||||
[3]: https://pendulum.eustace.io/docs/#installation
|
|
||||||
[4]: https://github.com/scikit-learn-contrib/imbalanced-learn
|
|
||||||
[5]: http://scikit-learn.org/stable/
|
|
||||||
[6]: https://github.com/scikit-learn-contrib
|
|
||||||
[7]: http://imbalanced-learn.org/en/stable/api.html
|
|
||||||
[8]: https://github.com/vi3k6i5/flashtext
|
|
||||||
[9]: https://arxiv.org/abs/1711.00046
|
|
||||||
[10]: https://flashtext.readthedocs.io/en/latest/
|
|
||||||
[11]: https://flashtext.readthedocs.io/en/latest/#usage
|
|
||||||
[12]: https://github.com/seatgeek/fuzzywuzzy
|
|
||||||
[13]: https://github.com/RJT1990/pyflux
|
|
||||||
[14]: https://pyflux.readthedocs.io/en/latest/index.html
|
|
||||||
[15]: https://github.com/maartenbreddels/ipyvolume
|
|
||||||
[16]: https://ipyvolume.readthedocs.io/en/latest/?badge=latest
|
|
||||||
[17]: https://github.com/plotly/dash
|
|
||||||
[18]: https://dash.plot.ly/
|
|
||||||
[19]: https://github.com/openai/gym
|
|
||||||
[20]: https://openai.com/
|
|
||||||
[21]: https://gym.openai.com/envs/CartPole-v0
|
|
||||||
[22]: https://gym.openai.com/
|
|
||||||
[23]: https://medium.com/analytics-vidhya/python-libraries-for-data-science-other-than-pandas-and-numpy-95da30568fad
|
|
@ -0,0 +1,268 @@
|
|||||||
|
|
||||||
|
9个不为人知晓的Python数据科学库
|
||||||
|
======
|
||||||
|
|
||||||
|
除了 pandas 、scikit-learn 和 matplotlib,还要学习一些用 Python 进行数据科学的新技巧。
|
||||||
|
|
||||||
|
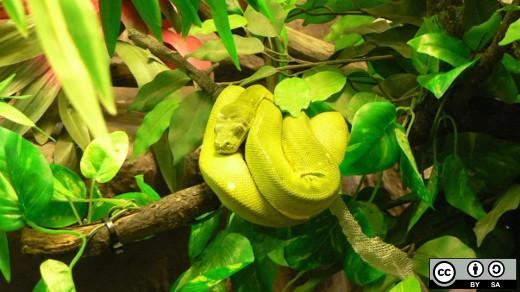
|
||||||
|
|
||||||
|
|
||||||
|
Python 是一种令人惊叹的语言。事实上,它是世界上增长最快的编程语言之一。它一次又一次地证明了它在各个行业的开发者和数据科学者中的作用。Python 及其库的整个生态系统使其成为全世界用户(初学者和高级用户)的恰当选择。它成功和受欢迎的原因之一是它的一组强大的库,使它如此动态和快速。
|
||||||
|
|
||||||
|
在本文中,我们将看到 Python 库中的一些数据科学工具,而不是那些常用的工具,如 **pandas,scikit-learn** ,和 **matplotlib** 。虽然像 **pandas,scikit-learn** 这样的库是机器学习中最常想到的,但是了解这个领域的其他 Python 产品库也是非常有帮助的。
|
||||||
|
|
||||||
|
### Wget
|
||||||
|
|
||||||
|
提取数据,尤其是从网络中提取数据,是数据科学家的重要任务之一。[Wget][1] 是一个免费的工具,用于从网络上非交互式下载文件。它支持 HTTP、HTTPS 和 FTP 协议,以及通过 HTTP 代理进行检索。因为它是非交互式的,所以即使用户没有登录,它也可以在后台工作。所以下次你想下载一个网站或者网页上的所有图片,**wget** 会提供帮助。
|
||||||
|
|
||||||
|
#### 安装
|
||||||
|
|
||||||
|
```
|
||||||
|
$ pip install wget
|
||||||
|
```
|
||||||
|
|
||||||
|
#### 例子
|
||||||
|
|
||||||
|
```
|
||||||
|
import wget
|
||||||
|
url = 'http://www.futurecrew.com/skaven/song_files/mp3/razorback.mp3'
|
||||||
|
|
||||||
|
filename = wget.download(url)
|
||||||
|
100% [................................................] 3841532 / 3841532
|
||||||
|
|
||||||
|
filename
|
||||||
|
'razorback.mp3'
|
||||||
|
```
|
||||||
|
|
||||||
|
### 钟摆
|
||||||
|
|
||||||
|
对于在 Python 中处理时间感到沮丧的人来说, **[Pendulum][2]** 库是很有帮助的。这是一个 Python 包,可以简化 **datetime** 操作。它是 Python 原生类的一个替换。有关详细信息,请参阅[documentation][3]。
|
||||||
|
|
||||||
|
|
||||||
|
#### 安装
|
||||||
|
|
||||||
|
```
|
||||||
|
$ pip install pendulum
|
||||||
|
```
|
||||||
|
|
||||||
|
#### 例子
|
||||||
|
|
||||||
|
```
|
||||||
|
import pendulum
|
||||||
|
|
||||||
|
dt_toronto = pendulum.datetime(2012, 1, 1, tz='America/Toronto')
|
||||||
|
dt_vancouver = pendulum.datetime(2012, 1, 1, tz='America/Vancouver')
|
||||||
|
|
||||||
|
print(dt_vancouver.diff(dt_toronto).in_hours())
|
||||||
|
|
||||||
|
3
|
||||||
|
```
|
||||||
|
|
||||||
|
### 不平衡学习
|
||||||
|
|
||||||
|
当每个类别中的样本数几乎相同(即平衡)时,大多数分类算法会工作得最好。但是现实生活中的案例中充满了不平衡的数据集,这可能会影响到机器学习算法的学习和后续预测。幸运的是, **[imbalanced-learn][4]** 库就是为了解决这个问题而创建的。它与[**scikit-learn**][5] 兼容,并且是 **[scikit-learn-contrib][6]** 项目的一部分。下次遇到不平衡的数据集时,可以尝试一下。
|
||||||
|
|
||||||
|
#### 安装
|
||||||
|
|
||||||
|
```
|
||||||
|
pip install -U imbalanced-learn
|
||||||
|
|
||||||
|
# or
|
||||||
|
|
||||||
|
conda install -c conda-forge imbalanced-learn
|
||||||
|
```
|
||||||
|
|
||||||
|
#### 例子
|
||||||
|
|
||||||
|
有关用法和示例,请参阅 [documentation][7] 。
|
||||||
|
|
||||||
|
|
||||||
|
### 闪光灯文字
|
||||||
|
|
||||||
|
在自然语言处理( NLP )任务中清理文本数据通常需要替换句子中的关键词或从句子中提取关键词。通常,这种操作可以用正则表达式来完成,但是如果要搜索的术语数达到数千个,它们可能会变得很麻烦。
|
||||||
|
|
||||||
|
Python的 **[FlashText][8]** 模块,基于 [FlashText algorithm][9]算法,为这种情况提供了一个合适的替代方案。FlashText 的最佳部分是运行时间与搜索项的数量无关。你可以在 [documentation][10] 中读到更多关于它的信息。
|
||||||
|
|
||||||
|
#### 安装
|
||||||
|
|
||||||
|
```
|
||||||
|
$ pip install flashtext
|
||||||
|
```
|
||||||
|
|
||||||
|
#### 例子
|
||||||
|
|
||||||
|
##### **提取关键词:**
|
||||||
|
|
||||||
|
```
|
||||||
|
from flashtext import KeywordProcessor
|
||||||
|
keyword_processor = KeywordProcessor()
|
||||||
|
|
||||||
|
# keyword_processor.add_keyword(<unclean name>, <standardised name>)
|
||||||
|
|
||||||
|
keyword_processor.add_keyword('Big Apple', 'New York')
|
||||||
|
keyword_processor.add_keyword('Bay Area')
|
||||||
|
keywords_found = keyword_processor.extract_keywords('I love Big Apple and Bay Area.')
|
||||||
|
|
||||||
|
keywords_found
|
||||||
|
['New York', 'Bay Area']
|
||||||
|
```
|
||||||
|
|
||||||
|
**替代关键词:**
|
||||||
|
|
||||||
|
```
|
||||||
|
keyword_processor.add_keyword('New Delhi', 'NCR region')
|
||||||
|
|
||||||
|
new_sentence = keyword_processor.replace_keywords('I love Big Apple and new delhi.')
|
||||||
|
|
||||||
|
new_sentence
|
||||||
|
'I love New York and NCR region.'
|
||||||
|
```
|
||||||
|
|
||||||
|
For more examples, refer to the [usage][11] section in the documentation.
|
||||||
|
|
||||||
|
有关更多示例,请参阅文档中的 [usage][11] 一节。
|
||||||
|
|
||||||
|
### 模糊处理
|
||||||
|
|
||||||
|
这个名字听起来很奇怪,但是 **[FuzzyWuzzy][12]** 在字符串匹配方面是一个非常有用的库。它可以很容易地实现字符串比较、令牌比较等操作。对于匹配保存在不同数据库中的记录也很方便。
|
||||||
|
|
||||||
|
#### 安装
|
||||||
|
|
||||||
|
```
|
||||||
|
$ pip install fuzzywuzzy
|
||||||
|
```
|
||||||
|
|
||||||
|
#### 例子
|
||||||
|
|
||||||
|
```
|
||||||
|
from fuzzywuzzy import fuzz
|
||||||
|
from fuzzywuzzy import process
|
||||||
|
|
||||||
|
# 简单的匹配率
|
||||||
|
|
||||||
|
fuzz.ratio("this is a test", "this is a test!")
|
||||||
|
97
|
||||||
|
|
||||||
|
# 部分的匹配率
|
||||||
|
fuzz.partial_ratio("this is a test", "this is a test!")
|
||||||
|
100
|
||||||
|
```
|
||||||
|
|
||||||
|
更多的例子可以在 FuzzyWuzy 的 [GitHub repo.][12]得到。
|
||||||
|
|
||||||
|
### PyFlux
|
||||||
|
|
||||||
|
时间序列分析是机器学习中最常遇到的问题之一。**[PyFlux][13]** 是Python中的开源库,专门为处理时间序列问题而构建的。该库拥有一系列优秀的现代时间序列模型,包括但不限于 **ARIMA** 、 **GARCH** ,、以及**VAR**模型。简而言之,PyFlux 为时间序列建模提供了一种概率方法。这值得一试。
|
||||||
|
|
||||||
|
#### 安装
|
||||||
|
|
||||||
|
```
|
||||||
|
pip install pyflux
|
||||||
|
```
|
||||||
|
|
||||||
|
#### 例子
|
||||||
|
|
||||||
|
有关用法和示例,请参阅 [documentation][14]。
|
||||||
|
|
||||||
|
### IPyvolume
|
||||||
|
|
||||||
|
|
||||||
|
交流结果是数据科学的一个重要方面,可视化结果提供了显著优势。 **[**IPyvolume**][15]** 是一个Python库,用于在Jupyter笔记本中可视化3D体积和字形(例如3D散点图),配置和工作量极小。然而,它目前处于1.0之前的阶段。一个很好的类比是这样的: IPyVolumee **volshow** 是3D阵列,Matplotlib 的**imshow** 是2D阵列。你可以在 [documentation][16] 中读到更多关于它的信息。
|
||||||
|
|
||||||
|
#### 安装
|
||||||
|
|
||||||
|
```
|
||||||
|
Using pip
|
||||||
|
$ pip install ipyvolume
|
||||||
|
|
||||||
|
Conda/Anaconda
|
||||||
|
$ conda install -c conda-forge ipyvolume
|
||||||
|
```
|
||||||
|
|
||||||
|
#### 例子
|
||||||
|
|
||||||
|
**Animation:**
|
||||||
|
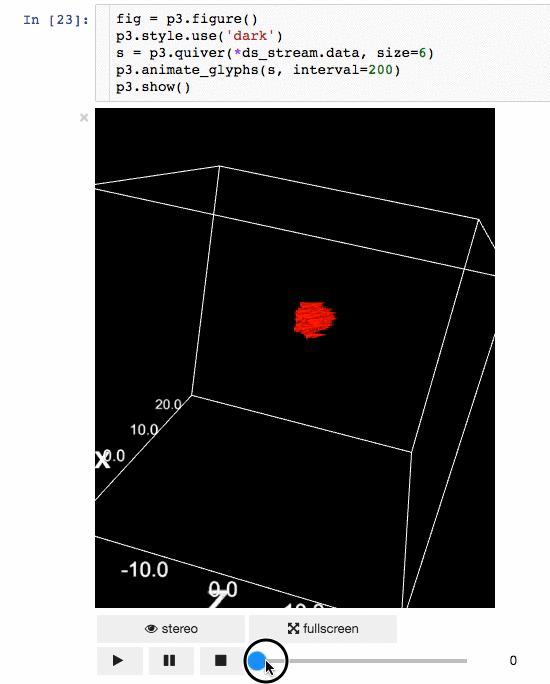
|
||||||
|
|
||||||
|
**Volume rendering:**
|
||||||
|
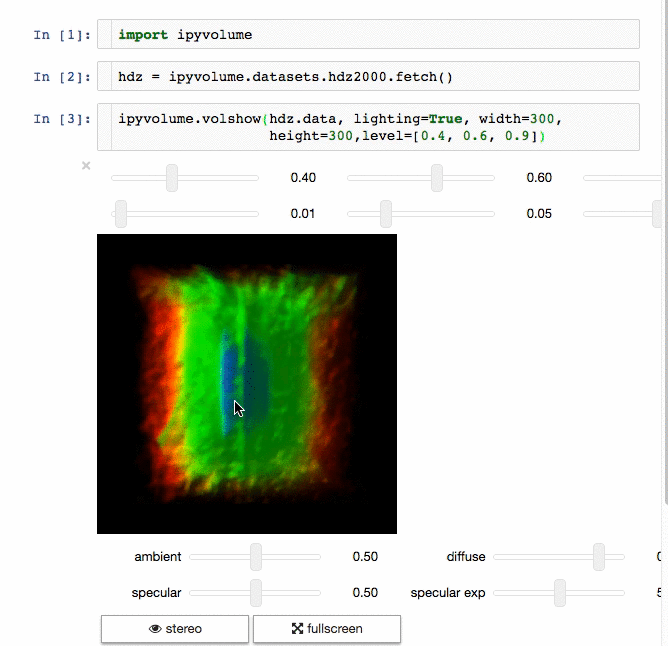
|
||||||
|
|
||||||
|
### Dash
|
||||||
|
|
||||||
|
**[Dash][17]** 是一个用于构建 Web 应用程序的高效 Python 框架。它写在Flask、Plotty.js和Response.js 的顶部,将下拉菜单、滑块和图形等流行 UI 元素与分析 Python 代码联系起来,而不需要JavaScript。Dash 非常适合构建可在 Web 浏览器中呈现的数据可视化应用程序。有关详细信息,请参阅 [user guide][18] 。
|
||||||
|
|
||||||
|
#### 安装
|
||||||
|
|
||||||
|
```
|
||||||
|
pip install dash==0.29.0 # The core dash backend
|
||||||
|
pip install dash-html-components==0.13.2 # HTML components
|
||||||
|
pip install dash-core-components==0.36.0 # Supercharged components
|
||||||
|
pip install dash-table==3.1.3 # Interactive DataTable component (new!)
|
||||||
|
```
|
||||||
|
|
||||||
|
#### 例子
|
||||||
|
|
||||||
|
|
||||||
|
下面的示例显示了一个具有下拉功能的高度交互的图表。当用户在下拉列表中选择一个值时,应用程序代码将数据从Google Finance 动态导出到 Pandas 数据框架中。
|
||||||
|
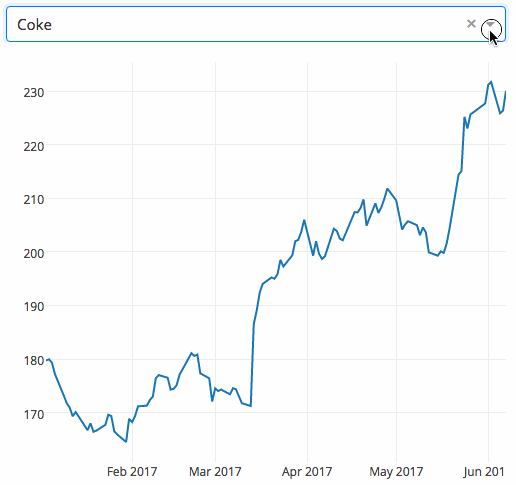
|
||||||
|
|
||||||
|
### Gym
|
||||||
|
|
||||||
|
从[OpenAI][20] 而来的 **[Gym][19]** 是开发和比较强化学习算法的工具包。它与任何数值计算库兼容,如TensorFlow 或Theano 。Gym 是一个测试问题的集合,也称为环境,你可以用它来制定你的强化学习算法。这些环境有一个共享接口,允许您编写通用算法。
|
||||||
|
|
||||||
|
#### 安装
|
||||||
|
|
||||||
|
```
|
||||||
|
pip install gym
|
||||||
|
```
|
||||||
|
|
||||||
|
#### 例子
|
||||||
|
|
||||||
|
|
||||||
|
|
||||||
|
以下示例将在 **[CartPole-v0][21]** 环境中,运行1,000次,在每一步渲染环境。
|
||||||
|
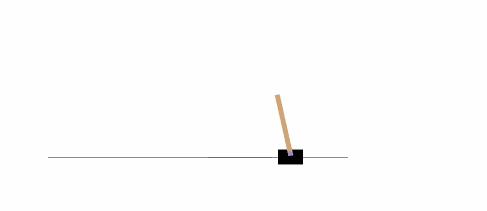
|
||||||
|
|
||||||
|
You can read about [other environments][22] on the Gym website.
|
||||||
|
你可以在 Gym 网站上读到其他的 [other environments][22] 。
|
||||||
|
|
||||||
|
### 结论
|
||||||
|
|
||||||
|
这些是我挑选的有用但鲜为人知的数据科学 Python 库。如果你知道另一个要添加到这个列表中,请在下面的评论中提及。
|
||||||
|
这本书最初发表在 [Analytics Vidhya][23] 的媒体频道上,并经许可转载。
|
||||||
|
|
||||||
|
|
||||||
|
via: https://opensource.com/article/18/11/python-libraries-data-science
|
||||||
|
|
||||||
|
作者:[Parul Pandey][a]
|
||||||
|
选题:[lujun9972][b]
|
||||||
|
译者:[heguangzhi](https://github.com/heguangzhi)
|
||||||
|
校对:[校对者ID](https://github.com/校对者ID)
|
||||||
|
|
||||||
|
本文由 [LCTT](https://github.com/LCTT/TranslateProject) 原创编译,[Linux中国](https://linux.cn/) 荣誉推出
|
||||||
|
|
||||||
|
[a]: https://opensource.com/users/parul-pandey
|
||||||
|
[b]: https://github.com/lujun9972
|
||||||
|
[1]: https://pypi.org/project/wget/
|
||||||
|
[2]: https://github.com/sdispater/pendulum
|
||||||
|
[3]: https://pendulum.eustace.io/docs/#installation
|
||||||
|
[4]: https://github.com/scikit-learn-contrib/imbalanced-learn
|
||||||
|
[5]: http://scikit-learn.org/stable/
|
||||||
|
[6]: https://github.com/scikit-learn-contrib
|
||||||
|
[7]: http://imbalanced-learn.org/en/stable/api.html
|
||||||
|
[8]: https://github.com/vi3k6i5/flashtext
|
||||||
|
[9]: https://arxiv.org/abs/1711.00046
|
||||||
|
[10]: https://flashtext.readthedocs.io/en/latest/
|
||||||
|
[11]: https://flashtext.readthedocs.io/en/latest/#usage
|
||||||
|
[12]: https://github.com/seatgeek/fuzzywuzzy
|
||||||
|
[13]: https://github.com/RJT1990/pyflux
|
||||||
|
[14]: https://pyflux.readthedocs.io/en/latest/index.html
|
||||||
|
[15]: https://github.com/maartenbreddels/ipyvolume
|
||||||
|
[16]: https://ipyvolume.readthedocs.io/en/latest/?badge=latest
|
||||||
|
[17]: https://github.com/plotly/dash
|
||||||
|
[18]: https://dash.plot.ly/
|
||||||
|
[19]: https://github.com/openai/gym
|
||||||
|
[20]: https://openai.com/
|
||||||
|
[21]: https://gym.openai.com/envs/CartPole-v0
|
||||||
|
[22]: https://gym.openai.com/
|
||||||
|
[23]: https://medium.com/analytics-vidhya/python-libraries-for-data-science-other-than-pandas-and-numpy-95da30568fad
|
Loading…
Reference in New Issue
Block a user