mirror of
https://github.com/LCTT/TranslateProject.git
synced 2024-12-26 21:30:55 +08:00
Merge pull request #11248 from ChiZelin/pr-2018-1118
[Translated]Monitoring database health and behavior: Which metrics ma…
This commit is contained in:
commit
7ab64da5a4
@ -1,83 +0,0 @@
|
||||
[translating by ChiZelin]
|
||||
Monitoring database health and behavior: Which metrics matter?
|
||||
======
|
||||
Monitoring your database can be overwhelming or seem not important. Here's how to do it right.
|
||||

|
||||
|
||||
We don’t talk about our databases enough. In this age of instrumentation, we monitor our applications, our infrastructure, and even our users, but we sometimes forget that our database deserves monitoring, too. That’s largely because most databases do their job so well that we simply trust them to do it. Trust is great, but confirmation of our assumptions is even better.
|
||||
|
||||
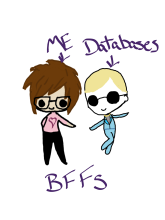
|
||||
|
||||
### Why monitor your databases?
|
||||
|
||||
There are plenty of reasons to monitor your databases, most of which are the same reasons you'd monitor any other part of your systems: Knowing what’s going on in the various components of your applications makes you a better-informed developer who makes smarter decisions.
|
||||
|
||||
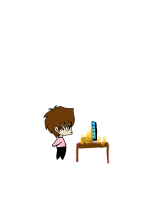
|
||||
|
||||
More specifically, databases are great indicators of system health and behavior. Odd behavior in the database can point to problem areas in your applications. Alternately, when there’s odd behavior in your application, you can use database metrics to help expedite the debugging process.
|
||||
|
||||
### The problem
|
||||
|
||||
The slightest investigation reveals one problem with monitoring databases: Databases have a lot of metrics. "A lot" is an understatement—if you were Scrooge McDuck, you could swim through all of the metrics available. If this were Wrestlemania, the metrics would be folding chairs. Monitoring them all doesn’t seem practical, so how do you decide which metrics to monitor?
|
||||
|
||||
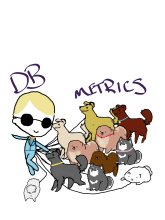
|
||||
|
||||
### The solution
|
||||
|
||||
The best way to start monitoring databases is to identify some foundational, database-agnostic metrics. These metrics create a great start to understanding the lives of your databases.
|
||||
|
||||
### Throughput: How much is the database doing?
|
||||
|
||||
The easiest way to start monitoring a database is to track the number of requests the database receives. We have high expectations for our databases; we expect them to store data reliably and handle all of the queries we throw at them, which could be one massive query a day or millions of queries from users all day long. Throughput can tell you which of those is true.
|
||||
|
||||
You can also group requests by type (reads, writes, server-side, client-side, etc.) to begin analyzing the traffic.
|
||||
|
||||
### Execution time: How long does it take the database to do its job?
|
||||
|
||||
This metric seems obvious, but it often gets overlooked. You don’t just want to know how many requests the database received, but also how long the database spent on each request. It’s important to approach execution time with context, though: What's slow for a time-series database like InfluxDB isn’t the same as what's slow for a relational database like MySQL. Slow in InfluxDB might mean milliseconds, whereas MySQL’s default value for its `SLOW_QUERY` variable is ten seconds.
|
||||
|
||||
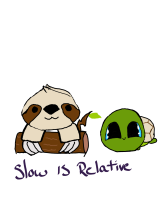
|
||||
|
||||
Monitoring execution time is not the same thing as improving execution time, so beware of the temptation to spend time on optimizations if you have other problems in your app to fix.
|
||||
|
||||
### Concurrency: How many jobs is the database doing at the same time?
|
||||
|
||||
Once you know how many requests the database is handling and how long each one takes, you need to add a layer of complexity to start getting real value from these metrics.
|
||||
|
||||
If the database receives ten requests and each one takes ten seconds to complete, is the database busy for 100 seconds, ten seconds—or somewhere in between? The number of concurrent tasks changes the way the database’s resources are used. When you consider things like the number of connections and threads, you’ll start to get a fuller picture of your database metrics.
|
||||
|
||||
Concurrency can also affect latency, which includes not only the time it takes for the task to be completed (execution time) but also the time the task needs to wait before it’s handled.
|
||||
|
||||
### Utilization: What percentage of the time was the database busy?
|
||||
|
||||
Utilization is a culmination of throughput, execution time, and concurrency to determine how often the database was available—or alternatively, how often the database was too busy to respond to a request.
|
||||
|
||||
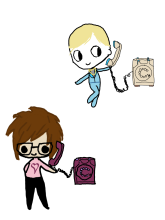
|
||||
|
||||
This metric is particularly useful for determining the overall health and performance of your database. If it’s available to respond to requests only 80% of the time, you can reallocate resources, work on optimization, or otherwise make changes to get closer to high availability.
|
||||
|
||||
### The good news
|
||||
|
||||
It can seem overwhelming to monitor and analyze, especially because most of us aren’t database experts and we may not have time to devote to understanding these metrics. But the good news is that most of this work is already done for us. Many databases have an internal performance database (Postgres: pg_stats, CouchDB: Runtime_Statistics, InfluxDB: _internal, etc.), which is designed by database engineers to monitor the metrics that matter for that particular database. You can see things as broad as the number of slow queries or as detailed as the average microseconds each event in the database takes.
|
||||
|
||||
### Conclusion
|
||||
|
||||
Databases create enough metrics to keep us all busy for a long time, and while the internal performance databases are full of useful information, it’s not always clear which metrics you should care about. Start with throughput, execution time, concurrency, and utilization, which provide enough information for you to start understanding the patterns in your database.
|
||||
|
||||
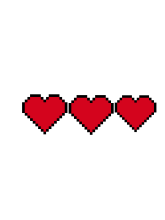
|
||||
|
||||
Are you monitoring your databases? Which metrics have you found to be useful? Tell me about it!
|
||||
|
||||
--------------------------------------------------------------------------------
|
||||
|
||||
via: https://opensource.com/article/18/10/database-metrics-matter
|
||||
|
||||
作者:[Katy Farmer][a]
|
||||
选题:[lujun9972][b]
|
||||
译者:[译者ID](https://github.com/译者ID)
|
||||
校对:[校对者ID](https://github.com/校对者ID)
|
||||
|
||||
本文由 [LCTT](https://github.com/LCTT/TranslateProject) 原创编译,[Linux中国](https://linux.cn/) 荣誉推出
|
||||
|
||||
[a]: https://opensource.com/users/thekatertot
|
||||
[b]: https://github.com/lujun9972
|
@ -0,0 +1,82 @@
|
||||
监测数据库的健康和行为: 有哪些重要指标?
|
||||
======
|
||||
对数据库的监测可能过于困难或者没有监测到关键点。本文将讲述如何正确的监测数据库。
|
||||

|
||||
|
||||
我们没有足够的讨论数据库。在这个充满监测仪器的时代,我们监测我们的应用程序、基础设施、甚至我们的用户,但有时忘记我们的数据库也值得被监测。这很大程度是因为数据库表现的很好,以至于我们单纯地信任它能把任务完成的很好。信任固然重要,但能够证明它的表现确实如我们所期待的那样就更好了。
|
||||
|
||||
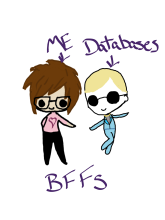
|
||||
|
||||
### 为什么监测你的数据库?
|
||||
|
||||
监测数据库的原因有很多,其中大多数原因与监测系统的任何其他部分的原因相同:了解应用程序的各个组件中发生的什么,会让你成为更了解情况的,能够做出明智决策的开发人员。
|
||||
|
||||
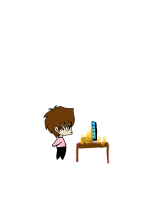
|
||||
|
||||
更具体地说,数据库是系统健康和行为的重要标志。数据库中的异常行为能够指出应用程序中出现问题的区域。另外,当应用程序中有异常行为时,你可以利用数据库的指标来迅速完成排除故障的过程。
|
||||
|
||||
### 问题
|
||||
|
||||
最轻微的调查揭示了监测数据库的一个问题:数据库有很多指标。说“很多”只是轻描淡写,如果你是Scrooge McDuck,你可以浏览所有可用的指标。如果这是Wrestlemania,那么指标就是折叠椅。监测所有指标似乎并不实用,那么你如何决定要监测哪些指标?
|
||||
|
||||
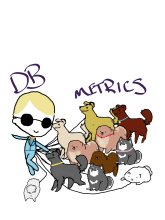
|
||||
|
||||
### 解决方案
|
||||
|
||||
开始监测数据库的最好方式是认识一些基础的数据库指标。这些指标为理解数据库的行为创造了良好的开端。
|
||||
|
||||
### 吞吐量:数据库做了多少?
|
||||
|
||||
开始检测数据库的最好方法是跟踪它所接到请求的数量。我们对数据库有较高期望;期望它能稳定的存储数据,并处理我们抛给它的所有查询,这些查询可能是一天一次大规模查询,或者是来自用户一天到晚的数百万次查询。吞吐量可以告诉我们数据库是否如我们期望的那样工作。
|
||||
|
||||
你也可以将请求安照类型(读,写,服务器端,客户端等)分组,以开始分析流量。
|
||||
|
||||
### 执行时间:数据库完成工作需要多长时间?
|
||||
|
||||
这个指标看起来很明显,但往往被忽视了。 你不仅想知道数据库收到了多少请求,还想知道数据库在每个请求上花费了多长时间。 然而,参考上下文来讨论执行时间非常重要:像InfluxDB这样的时间序列数据库中的慢与像MySQL这样的关系型数据库中的慢不一样。InfluxDB中的慢可能意味着毫秒,而MySQL的“SLOW_QUERY”变量的默认值是10秒。
|
||||
|
||||
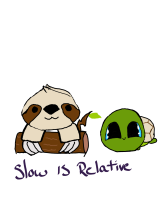
|
||||
|
||||
监测执行时间和提高执行时间不一样,所以如果你的应用程序中有其他问题需要修复,那么请注意在优化上花费时间的诱惑。
|
||||
|
||||
### 并发性:数据库同时做了多少工作?
|
||||
|
||||
一旦你知道数据库正在处理多少请求以及每个请求需要多长时间,你就需要添加一层复杂性以开始从这些指标中获得实际值。
|
||||
|
||||
如果数据库接收到十个请求,并且每个请求需要十秒钟来完成,那么数据库是否忙碌了100秒、10秒,或者介于两者之间?并发任务的数量改变了数据库资源的使用方式。当你考虑连接和线程的数量等问题时,你将开始对数据库指标有更全面的了解。
|
||||
|
||||
并发性还能影响延迟,这不仅包括任务完成所需的时间(执行时间),还包括任务在处理之前需要等待的时间。
|
||||
|
||||
### 利用率:数据库繁忙的时间百分比是多少?
|
||||
|
||||
利用率是由吞吐量、执行时间和并发性的峰值所确定的数据库可用的频率,或者数据库太忙而不能响应请求的频率。
|
||||
|
||||
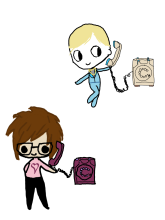
|
||||
|
||||
该指标对于确定数据库的整体健康和性能特别有用。如果只能在80%的时间内响应请求,则可以重新分配资源、进行优化工作,或者进行更改以更接近高可用性。
|
||||
|
||||
### 好消息
|
||||
|
||||
监测和分析似乎非常困难,特别是因为我们大多数人不是数据库专家,我们可能没有时间去理解这些指标。但好消息是,大部分的工作已经为我们做好了。许多数据库都有一个内部性能数据库(Postgres:pg_stats、CouchDB:Runtime_.、InfluxDB:_internal等),数据库工程师设计该数据库来监测与该特定数据库有关的指标。你可以看到像慢速查询的数量一样广泛的内容,或者像数据库中每个事件的平均微秒一样详细的内容。
|
||||
|
||||
### 结论
|
||||
|
||||
数据库创建了足够的指标以使我们需要长时间研究,虽然内部性能数据库充满了有用的信息,但并不总是使你清楚应该关注哪些指标。 从吞吐量,执行时间,并发性和利用率开始,它们为你提供了足够的信息,使你可以开始了解你的数据库中的情况。
|
||||
|
||||
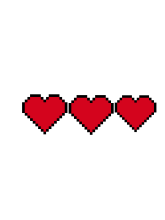
|
||||
|
||||
你在监视你的数据库吗?你发现哪些指标有用?告诉我吧!
|
||||
|
||||
--------------------------------------------------------------------------------
|
||||
|
||||
via: https://opensource.com/article/18/10/database-metrics-matter
|
||||
|
||||
作者:[Katy Farmer][a]
|
||||
选题:[lujun9972][b]
|
||||
译者:[ChiZelin](https://github.com/ChiZelin)
|
||||
校对:[校对者ID](https://github.com/校对者ID)
|
||||
|
||||
本文由 [LCTT](https://github.com/LCTT/TranslateProject) 原创编译,[Linux中国](https://linux.cn/) 荣誉推出
|
||||
|
||||
[a]: https://opensource.com/users/thekatertot
|
||||
[b]: https://github.com/lujun9972
|
Loading…
Reference in New Issue
Block a user