mirror of
https://github.com/LCTT/TranslateProject.git
synced 2025-03-03 01:10:13 +08:00
Merge pull request #12055 from MjSeven/tornado
20180614 An introduction to the Tornado Python web app framework.md 翻译完毕
This commit is contained in:
commit
633e178b86
@ -1,590 +0,0 @@
|
||||
[#]: collector: (lujun9972)
|
||||
[#]: translator: (MjSeven)
|
||||
[#]: reviewer: ( )
|
||||
[#]: publisher: ( )
|
||||
[#]: subject: (An introduction to the Tornado Python web app framework)
|
||||
[#]: via: (https://opensource.com/article/18/6/tornado-framework)
|
||||
[#]: author: (Nicholas Hunt-Walker https://opensource.com/users/nhuntwalker)
|
||||
[#]: url: ( )
|
||||
|
||||
An introduction to the Tornado Python web app framework
|
||||
======
|
||||
In the third part in a series comparing Python frameworks, learn about Tornado, built to handle asynchronous processes.
|
||||
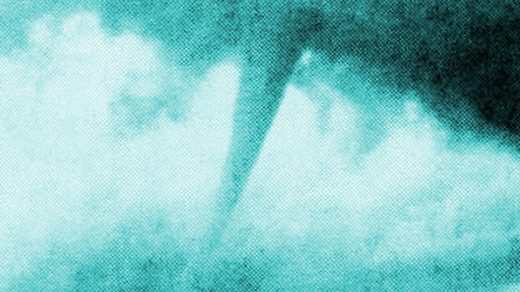
|
||||
|
||||
In the first two articles in this four-part series comparing different Python web frameworks, we've covered the [Pyramid][1] and [Flask][2] web frameworks. We've built the same app twice and seen the similarities and differences between a complete DIY framework and a framework with a few more batteries included.
|
||||
|
||||
Now let's look at a somewhat different option: [the Tornado framework][3]. Tornado is, for the most part, as bare-bones as Flask, but with a major difference: Tornado is built specifically to handle asynchronous processes. That special sauce isn't terribly useful in the app we're building in this series, but we'll see where we can use it and how it works in a more general situation.
|
||||
|
||||
Let's continue the pattern we set in the first two articles and start by tackling the setup and config.
|
||||
|
||||
### Tornado startup and configuration
|
||||
|
||||
If you've been following along with this series, what we do first shouldn't come as much of a surprise.
|
||||
|
||||
```
|
||||
$ mkdir tornado_todo
|
||||
$ cd tornado_todo
|
||||
$ pipenv install --python 3.6
|
||||
$ pipenv shell
|
||||
(tornado-someHash) $ pipenv install tornado
|
||||
```
|
||||
|
||||
Create a `setup.py` for installing our application:
|
||||
|
||||
```
|
||||
(tornado-someHash) $ touch setup.py
|
||||
# setup.py
|
||||
from setuptools import setup, find_packages
|
||||
|
||||
requires = [
|
||||
'tornado',
|
||||
'tornado-sqlalchemy',
|
||||
'psycopg2',
|
||||
]
|
||||
|
||||
setup(
|
||||
name='tornado_todo',
|
||||
version='0.0',
|
||||
description='A To-Do List built with Tornado',
|
||||
author='<Your name>',
|
||||
author_email='<Your email>',
|
||||
keywords='web tornado',
|
||||
packages=find_packages(),
|
||||
install_requires=requires,
|
||||
entry_points={
|
||||
'console_scripts': [
|
||||
'serve_app = todo:main',
|
||||
],
|
||||
},
|
||||
)
|
||||
```
|
||||
|
||||
Because Tornado doesn't require any external configuration, we can dive right into writing the Python code that'll run our application. Let's make our inner `todo` directory and fill it with the first few files we'll need.
|
||||
|
||||
```
|
||||
todo/
|
||||
__init__.py
|
||||
models.py
|
||||
views.py
|
||||
```
|
||||
|
||||
Like Flask and Pyramid, Tornado has some central configuration that will go in `__init__.py`. From `tornado.web`, we'll import the `Application` object. This will handle the hookups for routing and views, including our database (when we get there) and any extra settings needed to run our Tornado app.
|
||||
|
||||
```
|
||||
# __init__.py
|
||||
from tornado.httpserver import HTTPServer
|
||||
from tornado.options import define, options
|
||||
from tornado.web import Application
|
||||
|
||||
define('port', default=8888, help='port to listen on')
|
||||
|
||||
def main():
|
||||
"""Construct and serve the tornado application."""
|
||||
app = Application()
|
||||
http_server = HTTPServer(app)
|
||||
http_server.listen(options.port)
|
||||
```
|
||||
|
||||
When we use the `define` function, we end up creating attributes on the `options` object. Anything that goes in the position of the first argument will be the attribute's name, and what's assigned to the `default` keyword argument will be the value of that attribute.
|
||||
|
||||
As an example, if we name the attribute `potato` instead of `port`, we can access its value via `options.potato`.
|
||||
|
||||
Calling `listen` on the `HTTPServer` doesn't start the server yet. We must do one more step to have a working application that can listen for requests and return responses. We need an input-output loop. Thankfully, Tornado comes with that out of the box in the form of `tornado.ioloop.IOLoop`.
|
||||
|
||||
```
|
||||
# __init__.py
|
||||
from tornado.httpserver import HTTPServer
|
||||
from tornado.ioloop import IOLoop
|
||||
from tornado.options import define, options
|
||||
from tornado.web import Application
|
||||
|
||||
define('port', default=8888, help='port to listen on')
|
||||
|
||||
def main():
|
||||
"""Construct and serve the tornado application."""
|
||||
app = Application()
|
||||
http_server = HTTPServer(app)
|
||||
http_server.listen(options.port)
|
||||
print('Listening on http://localhost:%i' % options.port)
|
||||
IOLoop.current().start()
|
||||
```
|
||||
|
||||
I like some kind of a `print` statement somewhere that tells me when I'm serving my application, but that's me. You could do without the `print` line if you so chose.
|
||||
|
||||
We begin our I/O loop with `IOLoop.current().start()`. Let's talk a little more about input, output, and asynchronicity.
|
||||
|
||||
### The basics of async in Python and the I/O loop
|
||||
|
||||
Allow me to preface by saying that I am absolutely, positively, surely, and securely not an expert in asynchronous programming. As with all things I write, what follows stems from the limits of my understanding of the concept. As I am human, it may be deeply, deeply flawed.
|
||||
|
||||
The main concerns of an asynchronous program are:
|
||||
|
||||
* How is data coming in?
|
||||
* How is data going out?
|
||||
* When can some procedure be left to run without consuming my full attention?
|
||||
|
||||
|
||||
|
||||
Due to the [global interpreter lock][4] (GIL), Python is—by design—a [single-threaded][5] language. For every task a Python program must execute, the full attention of its thread of execution is on that task for the duration of that task. Our HTTP server is written in Python. Thus, when data (e.g., an HTTP request) is received, the server's sole focus is that incoming data. This means that, in most cases, whatever procedures need to run in handling and processing that data will completely consume your server's thread of execution, blocking other potential data from being received until your server finishes whatever it needed to do.
|
||||
|
||||
In many cases this isn't too problematic; a typical web request-response cycle will take only fractions of a second. Along with that, the sockets that HTTP servers are built from can maintain a backlog of incoming requests to be handled. So, if a request comes in while that socket is handling something else, chances are it'll just wait in line a bit before being addressed. For a low to intermediate traffic site, a fraction of a second isn't that big of a deal, and you can use multiple deployed instances along with a load-balancer like [NGINX][6] to distribute traffic for the larger request loads.
|
||||
|
||||
What if, however, your average response time takes more than a fraction of a second? What if you use data from the incoming request to start some long-running process like a machine-learning algorithm or some massive database query? Now, your single-threaded web server starts to accumulate an unaddressable backlog of requests, some of which will get dropped due to simply timing out. This is not an option, especially if you want your service to be seen as reliable on a regular basis.
|
||||
|
||||
In comes the asynchronous Python program. It's important to keep in mind that because it's written in Python, the program is still a single-threaded process. Anything that would block execution in a synchronous program, unless specifically flagged, will still block execution in an asynchronous one.
|
||||
|
||||
When it's structured correctly, however, your asynchronous Python program can "shelve" long-running tasks whenever you designate that a certain function should have the ability to do so. Your async controller can then be alerted when the shelved tasks are complete and ready to resume, managing their execution only when needed without completely blocking the handling of new input.
|
||||
|
||||
That was somewhat jargony, so let's demonstrate with a human example.
|
||||
|
||||
#### Bringing it home
|
||||
|
||||
I often find myself trying to get multiple chores done at home with little time to do them. On a given day, that backlog of chores may look like:
|
||||
|
||||
* Cook a meal (20 min. prep, 40 min. cook)
|
||||
* Wash dishes (60 min.)
|
||||
* Wash and dry laundry (30 min. wash, 90 min. dry per load)
|
||||
* Vacuum floors (30 min.)
|
||||
|
||||
|
||||
|
||||
If I were acting as a traditional, synchronous program, I'd be doing each task myself, by hand. Each task would require my full attention to complete before I could consider handling anything else, as nothing would get done without my active attention. So my sequence of execution might look like:
|
||||
|
||||
1. Focus fully on preparing and cooking the meal, including waiting around for food to just… cook (60 min.).
|
||||
2. Transfer dirty dishes to sink (65 min. elapsed).
|
||||
3. Wash all the dishes (125 min. elapsed).
|
||||
4. Start laundry with my full focus on that, including waiting around for the washing machine to finish, then transferring laundry to the dryer, and waiting for the dryer to finish (250 min. elapsed).
|
||||
5. Vacuum the floors (280 min. elapsed).
|
||||
|
||||
|
||||
|
||||
That's 4 hours and 40 minutes to complete my chores from end-to-end.
|
||||
|
||||
Instead of working hard, I should work smart like an asynchronous program. My home is full of machines that can do my work for me without my continuous effort. Meanwhile, I can switch my attention to what may actively need it right now.
|
||||
|
||||
My execution sequence might instead look like:
|
||||
|
||||
1. Load clothes into and start the washing machine (5 min.).
|
||||
2. While the washing machine is running, prep food (25 min. elapsed).
|
||||
3. After prepping food, start cooking food (30 min. elapsed).
|
||||
4. While the food is cooking, move clothes from the washing machine into the dryer and start dryer (35 min. elapsed).
|
||||
5. While dryer is running and food is still cooking, vacuum the floors (65 min. elapsed).
|
||||
6. After vacuuming the floors, take food off the stove and load the dishwasher (70 min. elapsed).
|
||||
7. Run the dishwasher (130 min. when done).
|
||||
|
||||
|
||||
|
||||
Now I'm down to 2 hours and 10 minutes. Even if I allow more time for switching between jobs (10-20 more minutes total), I'm still down to about half the time I would've spent if I'd waited to perform each task in sequential order. This is the power of structuring your program to be asynchronous.
|
||||
|
||||
#### So where does the I/O loop come in?
|
||||
|
||||
An asynchronous Python program works by taking in data from some external source (input) and, should the process require it, offloading that data to some external worker (output) for processing. When that external process finishes, the main Python program is alerted. The program then picks up the result of that external processing (input) and continues on its merry way.
|
||||
|
||||
Whenever that data isn't actively in the hands of the main Python program, that main program is freed to work on just about anything else. This includes awaiting completely new inputs (e.g., HTTP requests) and handling the results of long-running processes (e.g., results of machine-learning algorithms, long-running database queries). The main program, while still single-threaded, becomes event-driven, triggered into action for specific occurrences handled by the program. The main worker that listens for those events and dictates how they should be handled is the I/O loop.
|
||||
|
||||
We traveled a long road to get to this nugget of an explanation, I know, but what I'm hoping to communicate here is that it's not magic, nor is it some type of complex parallel processing or multi-threaded work. The global interpreter lock is still in place; any long-running process within the main program will still block anything else from happening. The program is also still single-threaded; however, by externalizing tedious work, we conserve the attention of that thread to only what it needs to be attentive to.
|
||||
|
||||
This is kind of like my asynchronous chores above. When my attention is fully necessary for prepping food, that's all I'm doing. However, when I can get the stove to do work for me by cooking my food, and the dishwasher to wash my dishes, and the washing machine and dryer to handle my laundry, my attention is freed to work on other things. When I am alerted that one of my long-running tasks is finished and ready to be handled once again, if my attention is free, I can pick up the results of that task and do whatever needs to be done with it next.
|
||||
|
||||
### Tornado routes and views
|
||||
|
||||
Despite having gone through all the trouble of talking about async in Python, we're going to hold off on using it for a bit and first write a basic Tornado view.
|
||||
|
||||
Unlike the function-based views we've seen in the Flask and Pyramid implementations, Tornado's views are all class-based. This means we'll no longer use individual, standalone functions to dictate how requests are handled. Instead, the incoming HTTP request will be caught and assigned to be an attribute of our defined class. Its methods will then handle the corresponding request types.
|
||||
|
||||
Let's start with a basic view that prints "Hello, World" to the screen. Every class-based view we construct for our Tornado app must inherit from the `RequestHandler` object found in `tornado.web`. This will set up all the ground-level logic that we'll need (but don't want to write) to take in a request and construct a properly formatted HTTP response.
|
||||
|
||||
```
|
||||
from tornado.web import RequestHandler
|
||||
|
||||
class HelloWorld(RequestHandler):
|
||||
"""Print 'Hello, world!' as the response body."""
|
||||
|
||||
def get(self):
|
||||
"""Handle a GET request for saying Hello World!."""
|
||||
self.write("Hello, world!")
|
||||
```
|
||||
|
||||
Because we're looking to handle a `GET` request, we declare (really override) the `get` method. Instead of returning anything, we provide text or a JSON-serializable object to be written to the response body with `self.write`. After that, we let the `RequestHandler` take on the rest of the work that must be done before a response can be sent.
|
||||
|
||||
As it stands, this view has no actual connection to the Tornado application itself. We have to go back into `__init__.py` and update the `main` function a bit. Here's the new hotness:
|
||||
|
||||
```
|
||||
# __init__.py
|
||||
from tornado.httpserver import HTTPServer
|
||||
from tornado.ioloop import IOLoop
|
||||
from tornado.options import define, options
|
||||
from tornado.web import Application
|
||||
from todo.views import HelloWorld
|
||||
|
||||
define('port', default=8888, help='port to listen on')
|
||||
|
||||
def main():
|
||||
"""Construct and serve the tornado application."""
|
||||
app = Application([
|
||||
('/', HelloWorld)
|
||||
])
|
||||
http_server = HTTPServer(app)
|
||||
http_server.listen(options.port)
|
||||
print('Listening on http://localhost:%i' % options.port)
|
||||
IOLoop.current().start()
|
||||
```
|
||||
|
||||
#### What'd we do?
|
||||
|
||||
We imported the `HelloWorld` view from the `views.py` file into `__init__.py` at the top of the script. Then we added a list of route-view pairs as the first argument to the instantiation to `Application`. Whenever we want to declare a route in our application, it must be tied to a view. You can use the same view for multiple routes if you want, but there must always be a view for every route.
|
||||
|
||||
We can make sure this all works by running our app with the `serve_app` command we enabled in the `setup.py`. Check `http://localhost:8888/` and see that it says "Hello, world!"
|
||||
|
||||
Of course, there's more we can and will do in this space, but let's move on to models.
|
||||
|
||||
### Connecting the database
|
||||
|
||||
If we want to hold onto data, we need to connect a database. Like with Flask, we'll be using a framework-specific variant of SQLAlchemy called [tornado-sqlalchemy][7].
|
||||
|
||||
Why use this instead of just the bare [SQLAlchemy][8]? Well, `tornado-sqlalchemy` has all the goodness of straightforward SQLAlchemy, so we can still declare models with a common `Base` as well as use all the column data types and relationships to which we've grown accustomed. Alongside what we already know from habit, `tornado-sqlalchemy` provides an accessible async pattern for its database-querying functionality specifically to work with Tornado's existing I/O loop.
|
||||
|
||||
We set the stage by adding `tornado-sqlalchemy` and `psycopg2` to `setup.py` to the list of required packages and reinstall the package. In `models.py`, we declare our models. This step looks pretty much exactly like what we've already seen in Flask and Pyramid, so I'll skip the full-class declarations and just put up the necessaries of the `Task` model.
|
||||
|
||||
```
|
||||
# this is not the complete models.py, but enough to see the differences
|
||||
from tornado_sqlalchemy import declarative_base
|
||||
|
||||
Base = declarative_base
|
||||
|
||||
class Task(Base):
|
||||
# and so on, because literally everything's the same...
|
||||
```
|
||||
|
||||
We still have to connect `tornado-sqlalchemy` to the actual application. In `__init__.py`, we'll be defining the database and integrating it into the application.
|
||||
|
||||
```
|
||||
# __init__.py
|
||||
from tornado.httpserver import HTTPServer
|
||||
from tornado.ioloop import IOLoop
|
||||
from tornado.options import define, options
|
||||
from tornado.web import Application
|
||||
from todo.views import HelloWorld
|
||||
|
||||
# add these
|
||||
import os
|
||||
from tornado_sqlalchemy import make_session_factory
|
||||
|
||||
define('port', default=8888, help='port to listen on')
|
||||
factory = make_session_factory(os.environ.get('DATABASE_URL', ''))
|
||||
|
||||
def main():
|
||||
"""Construct and serve the tornado application."""
|
||||
app = Application([
|
||||
('/', HelloWorld)
|
||||
],
|
||||
session_factory=factory
|
||||
)
|
||||
http_server = HTTPServer(app)
|
||||
http_server.listen(options.port)
|
||||
print('Listening on http://localhost:%i' % options.port)
|
||||
IOLoop.current().start()
|
||||
```
|
||||
|
||||
Much like the session factory we passed around in Pyramid, we can use `make_session_factory` to take in a database URL and produce an object whose sole purpose is to provide connections to the database for our views. We then tie it into our application by passing the newly created `factory` into the `Application` object with the `session_factory` keyword argument.
|
||||
|
||||
Finally, initializing and managing the database will look the same as it did for Flask and Pyramid (i.e., separate DB management script, working with respect to the `Base` object, etc.). It'll look so similar that I'm not going to reproduce it here.
|
||||
|
||||
### Revisiting views
|
||||
|
||||
Hello, World is always nice for learning the basics, but we need some real, application-specific views.
|
||||
|
||||
Let's start with the info view.
|
||||
|
||||
```
|
||||
# views.py
|
||||
import json
|
||||
from tornado.web import RequestHandler
|
||||
|
||||
class InfoView(RequestHandler):
|
||||
"""Only allow GET requests."""
|
||||
SUPPORTED_METHODS = ["GET"]
|
||||
|
||||
def set_default_headers(self):
|
||||
"""Set the default response header to be JSON."""
|
||||
self.set_header("Content-Type", 'application/json; charset="utf-8"')
|
||||
|
||||
def get(self):
|
||||
"""List of routes for this API."""
|
||||
routes = {
|
||||
'info': 'GET /api/v1',
|
||||
'register': 'POST /api/v1/accounts',
|
||||
'single profile detail': 'GET /api/v1/accounts/<username>',
|
||||
'edit profile': 'PUT /api/v1/accounts/<username>',
|
||||
'delete profile': 'DELETE /api/v1/accounts/<username>',
|
||||
'login': 'POST /api/v1/accounts/login',
|
||||
'logout': 'GET /api/v1/accounts/logout',
|
||||
"user's tasks": 'GET /api/v1/accounts/<username>/tasks',
|
||||
"create task": 'POST /api/v1/accounts/<username>/tasks',
|
||||
"task detail": 'GET /api/v1/accounts/<username>/tasks/<id>',
|
||||
"task update": 'PUT /api/v1/accounts/<username>/tasks/<id>',
|
||||
"delete task": 'DELETE /api/v1/accounts/<username>/tasks/<id>'
|
||||
}
|
||||
self.write(json.dumps(routes))
|
||||
```
|
||||
|
||||
So what changed? Let's go from the top down.
|
||||
|
||||
The `SUPPORTED_METHODS` class attribute was added. This will be an iterable of only the request methods that are accepted by this view. Any other method will return a [405][9] status code. When we made the `HelloWorld` view, we didn't specify this, mostly out of laziness. Without this class attribute, this view would respond to any request trying to access the route tied to the view.
|
||||
|
||||
The `set_default_headers` method is declared, which sets the default headers of the outgoing HTTP response. We declare this here to ensure that any response we send back has a `"Content-Type"` of `"application/json"`.
|
||||
|
||||
We added `json.dumps(some_object)` to the argument of `self.write` because it makes it easy to construct the content for the body of the outgoing response.
|
||||
|
||||
Now that's done, and we can go ahead and connect it to the home route in `__init__.py`.
|
||||
|
||||
```
|
||||
# __init__.py
|
||||
from tornado.httpserver import HTTPServer
|
||||
from tornado.ioloop import IOLoop
|
||||
from tornado.options import define, options
|
||||
from tornado.web import Application
|
||||
from todo.views import InfoView
|
||||
|
||||
# add these
|
||||
import os
|
||||
from tornado_sqlalchemy import make_session_factory
|
||||
|
||||
define('port', default=8888, help='port to listen on')
|
||||
factory = make_session_factory(os.environ.get('DATABASE_URL', ''))
|
||||
|
||||
def main():
|
||||
"""Construct and serve the tornado application."""
|
||||
app = Application([
|
||||
('/', InfoView)
|
||||
],
|
||||
session_factory=factory
|
||||
)
|
||||
http_server = HTTPServer(app)
|
||||
http_server.listen(options.port)
|
||||
print('Listening on http://localhost:%i' % options.port)
|
||||
IOLoop.current().start()
|
||||
```
|
||||
|
||||
As we know, more views and routes will need to be written. Each one will get dropped into the `Application` route listing as needed. Each will also need a `set_default_headers` method. On top of that, we'll create our `send_response`method, whose job it will be to package our response along with any custom status codes we want to set for a given response. Since each one will need both methods, we can create a base class containing them that each of our views can inherit from. That way, we have to write them only once.
|
||||
|
||||
```
|
||||
# views.py
|
||||
import json
|
||||
from tornado.web import RequestHandler
|
||||
|
||||
class BaseView(RequestHandler):
|
||||
"""Base view for this application."""
|
||||
|
||||
def set_default_headers(self):
|
||||
"""Set the default response header to be JSON."""
|
||||
self.set_header("Content-Type", 'application/json; charset="utf-8"')
|
||||
|
||||
def send_response(self, data, status=200):
|
||||
"""Construct and send a JSON response with appropriate status code."""
|
||||
self.set_status(status)
|
||||
self.write(json.dumps(data))
|
||||
```
|
||||
|
||||
For a view like the `TaskListView` we'll soon write, we'll also need a connection to the database. We'll need `tornado_sqlalchemy`'s `SessionMixin` to add a database session within every view class. We can fold that into the `BaseView` so that, by default, every view inheriting from it has access to a database session.
|
||||
|
||||
```
|
||||
# views.py
|
||||
import json
|
||||
from tornado_sqlalchemy import SessionMixin
|
||||
from tornado.web import RequestHandler
|
||||
|
||||
class BaseView(RequestHandler, SessionMixin):
|
||||
"""Base view for this application."""
|
||||
|
||||
def set_default_headers(self):
|
||||
"""Set the default response header to be JSON."""
|
||||
self.set_header("Content-Type", 'application/json; charset="utf-8"')
|
||||
|
||||
def send_response(self, data, status=200):
|
||||
"""Construct and send a JSON response with appropriate status code."""
|
||||
self.set_status(status)
|
||||
self.write(json.dumps(data))
|
||||
```
|
||||
|
||||
As long as we're modifying this `BaseView` object, we should address a quirk that will come up when we consider data being posted to this API.
|
||||
|
||||
When Tornado (as of v.4.5) consumes data from a client and organizes it for use in the application, it keeps all the incoming data as bytestrings. However, all the code here assumes Python 3, so the only strings that we want to work with are Unicode strings. We can add another method to this `BaseView` class whose job it will be to convert the incoming data to Unicode before using it anywhere else in the view.
|
||||
|
||||
If we want to convert this data before we use it in a proper view method, we can override the view class's native `prepare` method. Its job is to run before the view method runs. If we override the `prepare` method, we can set some logic to run that'll do the bytestring-to-Unicode conversion whenever a request is received.
|
||||
|
||||
```
|
||||
# views.py
|
||||
import json
|
||||
from tornado_sqlalchemy import SessionMixin
|
||||
from tornado.web import RequestHandler
|
||||
|
||||
class BaseView(RequestHandler, SessionMixin):
|
||||
"""Base view for this application."""
|
||||
|
||||
def prepare(self):
|
||||
self.form_data = {
|
||||
key: [val.decode('utf8') for val in val_list]
|
||||
for key, val_list in self.request.arguments.items()
|
||||
}
|
||||
|
||||
def set_default_headers(self):
|
||||
"""Set the default response header to be JSON."""
|
||||
self.set_header("Content-Type", 'application/json; charset="utf-8"')
|
||||
|
||||
def send_response(self, data, status=200):
|
||||
"""Construct and send a JSON response with appropriate status code."""
|
||||
self.set_status(status)
|
||||
self.write(json.dumps(data))
|
||||
```
|
||||
|
||||
If there's any data coming in, it'll be found within the `self.request.arguments` dictionary. We can access that data by key and convert its contents (always a list) to Unicode. Because this is a class-based view instead of a function-based view, we can store the modified data as an instance attribute to be used later. I called it `form_data` here, but it can just as easily be called `potato`. The point is that we can store data that has been submitted to the application.
|
||||
|
||||
### Asynchronous view methods
|
||||
|
||||
Now that we've built our `BaseView`, we can build the `TaskListView` that will inherit from it.
|
||||
|
||||
As you can probably tell from the section heading, this is where all that talk about asynchronicity comes in. The `TaskListView` will handle `GET` requests for returning a list of tasks and `POST` requests for creating new tasks given some form data. Let's first look at the code to handle the `GET` request.
|
||||
|
||||
```
|
||||
# all the previous imports
|
||||
import datetime
|
||||
from tornado.gen import coroutine
|
||||
from tornado_sqlalchemy import as_future
|
||||
from todo.models import Profile, Task
|
||||
|
||||
# the BaseView is above here
|
||||
class TaskListView(BaseView):
|
||||
"""View for reading and adding new tasks."""
|
||||
SUPPORTED_METHODS = ("GET", "POST",)
|
||||
|
||||
@coroutine
|
||||
def get(self, username):
|
||||
"""Get all tasks for an existing user."""
|
||||
with self.make_session() as session:
|
||||
profile = yield as_future(session.query(Profile).filter(Profile.username == username).first)
|
||||
if profile:
|
||||
tasks = [task.to_dict() for task in profile.tasks]
|
||||
self.send_response({
|
||||
'username': profile.username,
|
||||
'tasks': tasks
|
||||
})
|
||||
```
|
||||
|
||||
The first major piece here is the `@coroutine` decorator, imported from `tornado.gen`. Any Python callable that has a portion that acts out of sync with the normal flow of the call stack is effectively a "co-routine"; a routine that can run alongside other routines. In the example of my household chores, pretty much every chore was a co-routine. Some were blocking routines (e.g., vacuuming the floor), but that routine simply blocked my ability to start or attend to anything else. It didn't block any of the other routines that were already set in motion from continuing.
|
||||
|
||||
Tornado offers a number of ways to build an app that take advantage of co-routines, including allowing us to set locks on function calls, conditions for synchronizing asynchronous routines, and a system for manually modifying the events that control the I/O loop.
|
||||
|
||||
The only way the `@coroutine` decorator is used here is to allow the `get` method to farm out the SQL query as a background process and resume once the query is complete, while not blocking the Tornado I/O loop from handling other sources of incoming data. That is all that's "asynchronous" about this implementation: out-of-band database queries. Clearly if we wanted to showcase the magic and wonder of an async web app, a To-Do List isn't the way.
|
||||
|
||||
But hey, that's what we're building, so let's see how our method takes advantage of that `@coroutine` decorator. The `SessionMixin` that was, well, mixed into the `BaseView` declaration added two handy, database-aware attributes to our view class: `session` and `make_session`. They're similarly named and accomplish fairly similar goals.
|
||||
|
||||
The `self.session` attribute is a session with an eye on the database. At the end of the request-response cycle, just before the view sends a response back to the client, any changes that have been made to the database are committed, and the session is closed.
|
||||
|
||||
`self.make_session` is a context manager and generator, building and returning a brand new session object on the fly. That first `self.session` object still exists; `make_session` creates a new one anyway. The `make_session` generator also has baked into itself the logic for committing and closing the session it creates as soon as its context (i.e., indentation level) ends.
|
||||
|
||||
If you inspect the source code, there is no difference between the type of object assigned to `self.session` and the type of object generated by `self.make_session`. The difference is in how they're managed.
|
||||
|
||||
With the `make_session` context manager, the generated session belongs only to the context, beginning and ending within that context. You can open, modify, commit, and close multiple database sessions within the same view with the `make_session` context manager.
|
||||
|
||||
`self.session` is much simpler, with the session already opened by the time you get to your view method and committing before the response is sent back to the client.
|
||||
|
||||
Although the [read the docs snippet][10] and the [the PyPI example][11] both specify the use of the context manager, there's nothing about either the `self.session` object or the `session` generated by `self.make_session` that is inherently asynchronous. The point where we start thinking about the async behavior built into `tornado-sqlalchemy` comes when we initiate a query.
|
||||
|
||||
The `tornado-sqlalchemy` package provides us with the `as_future` function. The job of `as_future` is to wrap the query constructed by the `tornado-sqlalchemy` session and yield its return value. If the view method is decorated with `@coroutine`, then using this `yield as_future(query)` pattern will now make your wrapped query an asynchronous background process. The I/O loop takes over, awaiting the return value of the query and the resolution of the `future` object created by `as_future`.
|
||||
|
||||
To have access to the result from `as_future(query)`, you must `yield` from it. Otherwise, you get only an unresolved generator object and can do nothing with the query.
|
||||
|
||||
Everything else in this view method is pretty much par for the course, mirroring what we've already seen in Flask and Pyramid.
|
||||
|
||||
The `post` method will look fairly similar. For the sake of consistency, let's see how the `post` method looks and how it handles the `self.form_data` that was constructed with the `BaseView`.
|
||||
|
||||
```
|
||||
@coroutine
|
||||
def post(self, username):
|
||||
"""Create a new task."""
|
||||
with self.make_session() as session:
|
||||
profile = yield as_future(session.query(Profile).filter(Profile.username == username).first)
|
||||
if profile:
|
||||
due_date = self.form_data['due_date'][0]
|
||||
task = Task(
|
||||
name=self.form_data['name'][0],
|
||||
note=self.form_data['note'][0],
|
||||
creation_date=datetime.now(),
|
||||
due_date=datetime.strptime(due_date, '%d/%m/%Y %H:%M:%S') if due_date else None,
|
||||
completed=self.form_data['completed'][0],
|
||||
profile_id=profile.id,
|
||||
profile=profile
|
||||
)
|
||||
session.add(task)
|
||||
self.send_response({'msg': 'posted'}, status=201)
|
||||
```
|
||||
|
||||
As I said, it's about what we'd expect:
|
||||
|
||||
* The same query pattern as we saw with the `get` method
|
||||
* The construction of an instance of a new `Task` object, populated with data from `form_data`
|
||||
* The adding (but not committing because it's handled by the context manager!) of the new `Task` object to the database session
|
||||
* The sending of a response back to the client
|
||||
|
||||
|
||||
|
||||
And thus we have the basis for our Tornado web app. Everything else (e.g., database management and more views for a more complete app) is effectively the same as what we've already seen in the Flask and Pyramid apps.
|
||||
|
||||
### Thoughts about using the right tool for the right job
|
||||
|
||||
What we're starting to see as we continue to move through these web frameworks is that they can all effectively handle the same problems. For something like this To-Do List, any framework can do the job. However, some web frameworks are more appropriate for certain jobs than other ones, depending on what "more appropriate" means for you and your needs.
|
||||
|
||||
While Tornado is clearly capable of handling the same job that Pyramid or Flask can handle, to use it for an app like this is effectively a waste. It's like using a car to travel one block from home. Yes it can do the job of "travel," but short trips aren't why you choose to use a car over a bike or just your feet.
|
||||
|
||||
Per the documentation, Tornado is billed as "a Python web framework and asynchronous networking library." There are few like it in the Python web framework ecosystem. If the job you're trying to accomplish requires (or would benefit significantly from) asynchronicity in any way, shape, or form, use Tornado. If your application needs to handle multiple, long-lived connections while not sacrificing much in performance, choose Tornado. If your application is many applications in one and needs to be thread-aware for the accurate handling of data, reach for Tornado. That's where it works best.
|
||||
|
||||
Use your car to do "car things." Use other modes of transportation to do everything else.
|
||||
|
||||
### Going forward and a little perspective check
|
||||
|
||||
Speaking of using the right tool for the right job, keep in mind the scope and scale, both present and future, of your application when choosing your framework. Up to this point we've only looked at frameworks meant for small to midsized web applications. The next and final installment of this series will cover one of the most popular Python frameworks, Django, meant for big applications that might grow bigger. Again, while it technically can and will handle the To-Do List problem, keep in mind that it's not really what the framework is for. We'll still put it through its paces to show how an application can be built with it, but we have to keep in mind the intent of the framework and how that's reflected in its architecture:
|
||||
|
||||
* **Flask:** Meant for small, simple projects; makes it easy for us to construct views and connect them to routes quickly; can be encapsulated in a single file without much fuss
|
||||
* **Pyramid:** Meant for projects that may grow; contains a fair bit of configuration to get up and running; separate realms of application components can easily be divided and built out to arbitrary depth without losing sight of the central application
|
||||
* **Tornado:** Meant for projects benefiting from precise and deliberate I/O control; allows for co-routines and easily exposes methods that can control how requests are received/responses are sent and when those operations occur
|
||||
* **Django:** (As we'll see) meant for big things that may get bigger; large ecosystem of add-ons and mods; very opinionated in its configuration and management in order to keep all the disparate parts in line
|
||||
|
||||
|
||||
|
||||
Whether you've been reading since the first post in this series or joined a little later, thanks for reading! Please feel free to leave questions or comments. I'll see you next time with hands full of Django.
|
||||
|
||||
### Huge shout-out to the Python BDFL
|
||||
|
||||
I must give credit where credit is due. Massive thanks are owed to [Guido van Rossum][12] for more than just creating my favorite programming language.
|
||||
|
||||
During [PyCascades 2018][13], I was fortunate not only to give the talk this article series is based on, but also to be invited to the speakers' dinner. I got to sit next to Guido the whole night and pepper him with questions. One of those questions was how in the world async worked in Python, and he, without a bit of fuss, spent time explaining it to me in a way that I could start to grasp the concept. He later [tweeted to me][14] a spectacular resource for learning async with Python that I subsequently read three times over three months, then wrote this post. You're an awesome guy, Guido!
|
||||
|
||||
--------------------------------------------------------------------------------
|
||||
|
||||
via: https://opensource.com/article/18/6/tornado-framework
|
||||
|
||||
作者:[Nicholas Hunt-Walker][a]
|
||||
选题:[lujun9972][b]
|
||||
译者:[译者ID](https://github.com/译者ID)
|
||||
校对:[校对者ID](https://github.com/校对者ID)
|
||||
|
||||
本文由 [LCTT](https://github.com/LCTT/TranslateProject) 原创编译,[Linux中国](https://linux.cn/) 荣誉推出
|
||||
|
||||
[a]: https://opensource.com/users/nhuntwalker
|
||||
[b]: https://github.com/lujun9972
|
||||
[1]: https://opensource.com/article/18/5/pyramid-framework
|
||||
[2]: https://opensource.com/article/18/4/flask
|
||||
[3]: https://tornado.readthedocs.io/en/stable/
|
||||
[4]: https://realpython.com/python-gil/
|
||||
[5]: https://en.wikipedia.org/wiki/Thread_(computing)
|
||||
[6]: https://www.nginx.com/
|
||||
[7]: https://tornado-sqlalchemy.readthedocs.io/en/latest/
|
||||
[8]: https://www.sqlalchemy.org/
|
||||
[9]: https://en.wikipedia.org/wiki/List_of_HTTP_status_codes#4xx_Client_errors
|
||||
[10]: https://tornado-sqlalchemy.readthedocs.io/en/latest/#usage
|
||||
[11]: https://pypi.org/project/tornado-sqlalchemy/#description
|
||||
[12]: https://www.twitter.com/gvanrossum
|
||||
[13]: https://www.pycascades.com
|
||||
[14]: https://twitter.com/gvanrossum/status/956186585493458944
|
@ -0,0 +1,580 @@
|
||||
[#]: collector: (lujun9972)
|
||||
[#]: translator: (MjSeven)
|
||||
[#]: reviewer: ( )
|
||||
[#]: publisher: ( )
|
||||
[#]: subject: (An introduction to the Tornado Python web app framework)
|
||||
[#]: via: (https://opensource.com/article/18/6/tornado-framework)
|
||||
[#]: author: (Nicholas Hunt-Walker https://opensource.com/users/nhuntwalker)
|
||||
[#]: url: ( )
|
||||
|
||||
Python Web 框架 Tornado 简介
|
||||
======
|
||||
|
||||
在比较 Python 框架的系列文章的第三部分中,我们来了解 Tornado,它是为处理异步进程而构建的。
|
||||
|
||||
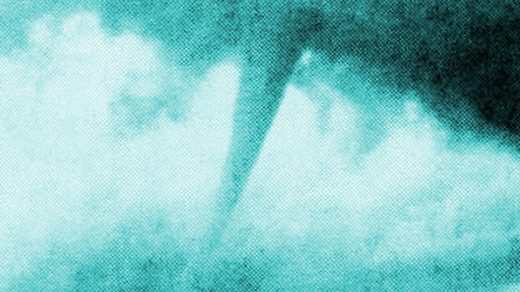
|
||||
|
||||
在这个由四部分组成的系列文章的前两篇中,我们介绍了 [Pyramid][1] 和 [Flask][2] Web 框架。我们已经构建了两次相同的应用程序,看到了一个完全的 DIY 框架和包含更多电池的框架之间的异同。
|
||||
|
||||
现在让我们来看看另一个稍微不同的选项:[Tornado 框架][3]。Tornado 在很大程度上与 Flask 一样简单,但有一个主要区别:Tornado 是专门为处理异步进程而构建的。在我们本系列所构建的应用程序中,这种特殊的酱料(译者注:这里意思是 Tornado 的异步功能)并不是非常有用,但我们将看到在哪里可以使用它,以及它在更一般的情况下是如何工作的。
|
||||
|
||||
让我们继续前两篇文章中设置的流程,首先从处理设置和配置。
|
||||
|
||||
### Tornado 启动和配置
|
||||
|
||||
如果你一直关注这个系列,那么第一步应该对你来说习以为常。
|
||||
```
|
||||
$ mkdir tornado_todo
|
||||
$ cd tornado_todo
|
||||
$ pipenv install --python 3.6
|
||||
$ pipenv shell
|
||||
(tornado-someHash) $ pipenv install tornado
|
||||
```
|
||||
|
||||
创建一个 `setup.py` 文件来安装我们的应用程序相关的东西:
|
||||
```
|
||||
(tornado-someHash) $ touch setup.py
|
||||
# setup.py
|
||||
from setuptools import setup, find_packages
|
||||
|
||||
requires = [
|
||||
'tornado',
|
||||
'tornado-sqlalchemy',
|
||||
'psycopg2',
|
||||
]
|
||||
|
||||
setup(
|
||||
name='tornado_todo',
|
||||
version='0.0',
|
||||
description='A To-Do List built with Tornado',
|
||||
author='<Your name>',
|
||||
author_email='<Your email>',
|
||||
keywords='web tornado',
|
||||
packages=find_packages(),
|
||||
install_requires=requires,
|
||||
entry_points={
|
||||
'console_scripts': [
|
||||
'serve_app = todo:main',
|
||||
],
|
||||
},
|
||||
)
|
||||
```
|
||||
|
||||
因为 Tornado 不需要任何外部配置,所以我们可以直接编写 Python 代码来让程序运行。让我们创建 `todo` 目录,并用需要的前几个文件填充它。
|
||||
```
|
||||
todo/
|
||||
__init__.py
|
||||
models.py
|
||||
views.py
|
||||
```
|
||||
|
||||
就像 Flask 和 Pyramid 一样,Tornado 也有一些基本配置,将放在 `__init__.py` 中。从 `tornado.web` 中,我们将导入 `Application` 对象,它将处理路由和视图的连接,包括数据库(当我们谈到那里时再说)以及运行 Tornado 应用程序所需的其它额外设置。
|
||||
|
||||
```
|
||||
# __init__.py
|
||||
from tornado.httpserver import HTTPServer
|
||||
from tornado.options import define, options
|
||||
from tornado.web import Application
|
||||
|
||||
define('port', default=8888, help='port to listen on')
|
||||
|
||||
def main():
|
||||
"""Construct and serve the tornado application."""
|
||||
app = Application()
|
||||
http_server = HTTPServer(app)
|
||||
http_server.listen(options.port)
|
||||
```
|
||||
|
||||
当我们使用 `define` 函数时,我们最终会在 `options` 对象上创建属性。第一个参数位置的任何内容都将是属性的名称,分配给 `default` 关键字参数的内容将是该属性的值。
|
||||
|
||||
例如,如果我们将属性命名为 `potato` 而不是 `port`,我们可以通过 `options.potato` 访问它的值。
|
||||
|
||||
在 `HTTPServer` 上调用 `listen` 并不会启动服务器。我们必须再做一步,找一个可以监听请求并返回响应的工作应用程序,我们需要一个输入输出循环。幸运的是,Tornado 以 `tornado.ioloop.IOLoop` 的形式提供了开箱即用的功能。
|
||||
|
||||
```
|
||||
# __init__.py
|
||||
from tornado.httpserver import HTTPServer
|
||||
from tornado.ioloop import IOLoop
|
||||
from tornado.options import define, options
|
||||
from tornado.web import Application
|
||||
|
||||
define('port', default=8888, help='port to listen on')
|
||||
|
||||
def main():
|
||||
"""Construct and serve the tornado application."""
|
||||
app = Application()
|
||||
http_server = HTTPServer(app)
|
||||
http_server.listen(options.port)
|
||||
print('Listening on http://localhost:%i' % options.port)
|
||||
IOLoop.current().start()
|
||||
```
|
||||
|
||||
我喜欢某种形式的 `print` 声明,告诉我什么时候应用程序正在提供服务,我就是这样子。如果你愿意,可以不使用 `print`。
|
||||
|
||||
我们以 `IOLoop.current().start()` 开始我们的 I/O 循环。让我们进一步讨论输入,输出和异步性。
|
||||
|
||||
### Python 中的异步和 I/O 循环的基础知识
|
||||
|
||||
请允许我提前说明,我绝对,肯定,肯定并且安心地说不是异步编程方面的专家。就像我写的所有内容一样,接下来的内容源于我对这个概念的理解的局限性。因为我是人,可能有很深很深的缺陷。
|
||||
|
||||
异步程序的主要问题是:
|
||||
|
||||
* 数据如何进来?
|
||||
* 数据如何出去?
|
||||
* 什么时候可以在不占用我全部注意力情况下运行某个过程?
|
||||
|
||||
由于[全局解释器锁][4](GIL),Python 被设计为一种单线程语言。对于 Python 程序必须执行的每个任务,其线程执行的全部注意力都集中在该任务的持续时间内。我们的 HTTP 服务器是用 Python 编写的,因此,当接收到数据(如 HTTP 请求)时,服务器的唯一关心的是传入的数据。这意味着,在大多数情况下,无论是程序需要运行还是处理数据,程序都将完全消耗服务器的执行线程,阻止接收其它可能的数据,直到服务器完成它需要做的事情。
|
||||
|
||||
在许多情况下,这不是太成问题。典型的 Web 请求,响应周期只需要几分之一秒。除此之外,构建 HTTP 服务器的套接字可以维护待处理的传入请求的积压。因此,如果请求在该套接字处理其它内容时进入,则它很可能只是在处理之前稍微排队等待一会。对于低到中等流量的站点,几分之一秒的时间并不是什么大问题,你可以使用多个部署的实例以及 [NGINX][6] 等负载均衡器来为更大的请求负载分配流量。
|
||||
|
||||
但是,如果你的平均响应时间超过一秒钟,该怎么办?如果你使用来自传入请求的数据来启动一些长时间的过程(如机器学习算法或某些海量数据库查询),该怎么办?现在,你的单线程 Web 服务器开始累积一个无法寻址的积压请求,其中一些请求会因为超时而被丢弃。这不是一种选择,特别是如果你希望你的服务在一段时间内是可靠的。
|
||||
|
||||
异步 Python 程序登场。重要的是要记住因为它是用 Python 编写的,所以程序仍然是一个单线程进程。除非特别标记,否则在异步程序中仍然会阻塞执行。
|
||||
|
||||
但是,当异步程序结构正确时,只要你指定某个函数应该具有这样的能力,你的异步 Python 程序就可以“搁置”长时间运行的任务。然后,当搁置的任务完成并准备好恢复时,异步控制器会收到报告,只要在需要时管理它们的执行,而不会完全阻塞对新输入的处理。
|
||||
|
||||
这有点夸张,所以让我们用一个人类的例子来证明。
|
||||
|
||||
### 带回家吧
|
||||
|
||||
我经常发现自己在家里试图完成很多家务,但没有多少时间来做它们。在某一天,积压的家务可能看起来像:
|
||||
|
||||
* 做饭(20 分钟准备,40 分钟烹饪)
|
||||
* 洗碗(60 分钟)
|
||||
* 洗涤并擦干衣物(30 分钟洗涤,每次干燥 90 分钟)
|
||||
* 真空清洗地板(30 分钟)
|
||||
|
||||
如果我是一个传统的同步程序,我会亲自完成每项任务。在我考虑处理任何其他事情之前,每项任务都需要我全神贯注地完成。因为如果没有我的全力关注,什么事情都完成不了。所以我的执行顺序可能如下:
|
||||
|
||||
1. 完全专注于准备和烹饪食物,包括等待食物烹饪(60 分钟)
|
||||
2. 将脏盘子移到水槽中(65 分钟过去了)
|
||||
3. 清洗所有盘子(125 分钟过去了)
|
||||
4. 开始完全专注于洗衣服,包括等待洗衣机洗完,然后将衣物转移到烘干机,再等烘干机完成( 250 分钟过去了)
|
||||
5. 对地板进行真空吸尘(280 分钟了)
|
||||
|
||||
从头到尾完成所有事情花费了 4 小时 40 分钟。
|
||||
|
||||
我应该像异步程序一样聪明地工作,而不是努力工作。我的家里到处都是可以为我工作的机器,而不用我一直努力工作。同时,现在我可以将注意力转移真正需要的东西上。
|
||||
|
||||
我的执行顺序可能看起来像:
|
||||
|
||||
1. 将衣物放入洗衣机并启动它(5 分钟)
|
||||
2. 在洗衣机运行时,准备食物(25 分钟过去了)
|
||||
3. 准备好食物后,开始烹饪食物(30 分钟过去了)
|
||||
4. 在烹饪食物时,将衣物从洗衣机移到烘干机机中开始烘干(35 分钟过去了)
|
||||
5. 当烘干机运行中,且食物仍在烹饪时,对地板进行真空吸尘(65 分钟过去了)
|
||||
6. 吸尘后,将食物从炉子中取出并装盘子入洗碗机(70 分钟过去了)
|
||||
7. 运行洗碗机(130 分钟完成)
|
||||
|
||||
现在花费的时间下降到 2 小时 10 分钟。即使我允许在作业之间切换花费更多时间(总共 10-20 分钟)。如果我等待着按顺序执行每项任务,我花费的时间仍然只有一半左右。这就是将程序构造为异步的强大功能。
|
||||
|
||||
#### 那么 I/O 循环在哪里?
|
||||
|
||||
一个异步 Python 程序的工作方式是从某个外部源(输入)获取数据,如果某个进程需要,则将该数据转移到某个外部工作者(输出)进行处理。当外部进程完成时,Python 主程序会收到提醒,然后程序获取外部处理(输入)的结果,并继续这样其乐融融的方式。
|
||||
|
||||
当数据不在 Python 主程序手中时,主程序就会被释放来处理其它任何事情。包括等待全新的输入(如 HTTP 请求)和处理长时间运行的进程的结果(如机器学习算法的结果,长时间运行的数据库查询)。主程序虽仍然是单线程的,但成了事件驱动的,它对程序处理的特定事件会触发动作。监听这些事件并指示应如何处理它们的主要是 I/O 循环在工作。
|
||||
|
||||
我知道,我们走了很长的路才得到这个重要的解释,但我希望在这里传达的是,它不是魔术,也不是某种复杂的并行处理或多线程工作。全局解释器锁仍然存在,主程序中任何长时间运行的进程仍然会阻塞其它任何事情的进行,该程序仍然是单线程的。然而,通过将繁琐的工作外部化,我们可以将线程的注意力集中在它需要注意的地方。
|
||||
|
||||
这有点像我上面的异步任务。当我的注意力完全集中在准备食物上时,它就是我所能做的一切。然而,当我能让炉子帮我做饭,洗碗机帮我洗碗,洗衣机和烘干机帮我洗衣服时,我的注意力就会被释放出来,去做其它事情。当我被提醒,我的一个长时间运行的任务已经完成并准备再次处理时,如果我的注意力是空闲的,我可以获取该任务的结果,并对其做下一步需要做的任何事情。
|
||||
|
||||
### Tornado 路由和视图
|
||||
|
||||
尽管经历了在 Python 中讨论异步的所有麻烦,我们还是决定暂不使用它。先来编写一个基本的 Tornado 视图。
|
||||
|
||||
与我们在 Flask 和 Pyramid 实现中看到的基于函数的视图不同,Tornado 的视图都是基于类的。这意味着我们将不在使用单独的,独立的函数来规定如何处理请求。相反,传入的 HTTP 请求将被捕获并将其分配为我们定义的类的一个属性。然后,它的方法将处理相应的请求类型。
|
||||
|
||||
让我们从一个基本的视图开始,即在屏幕上打印 "Hello, World"。我们为 Tornado 应用程序构造的每个基于类的视图都必须继承 `tornado.web` 中的 `RequestHandler` 对象。这将设置我们需要(但不想写)的所有底层逻辑来接收请求,同时构造正确格式的 HTTP 响应。
|
||||
|
||||
```
|
||||
from tornado.web import RequestHandler
|
||||
|
||||
class HelloWorld(RequestHandler):
|
||||
"""Print 'Hello, world!' as the response body."""
|
||||
|
||||
def get(self):
|
||||
"""Handle a GET request for saying Hello World!."""
|
||||
self.write("Hello, world!")
|
||||
```
|
||||
|
||||
因为我们要处理 `GET` 请求,所以我们声明(实际上是重写) `get` 方法。我们提供文本或 JSON 可序列化对象,用 `self.write` 写入响应体。之后,我们让 `RequestHandler` 来做在发送响应之前必须完成的其它工作。
|
||||
|
||||
就目前而言,此视图与 Tornado 应用程序本身并没有实际连接。我们必须回到 `__init__.py`,并稍微更新 `main` 函数。以下是新的内容:
|
||||
|
||||
```
|
||||
# __init__.py
|
||||
from tornado.httpserver import HTTPServer
|
||||
from tornado.ioloop import IOLoop
|
||||
from tornado.options import define, options
|
||||
from tornado.web import Application
|
||||
from todo.views import HelloWorld
|
||||
|
||||
define('port', default=8888, help='port to listen on')
|
||||
|
||||
def main():
|
||||
"""Construct and serve the tornado application."""
|
||||
app = Application([
|
||||
('/', HelloWorld)
|
||||
])
|
||||
http_server = HTTPServer(app)
|
||||
http_server.listen(options.port)
|
||||
print('Listening on http://localhost:%i' % options.port)
|
||||
IOLoop.current().start()
|
||||
```
|
||||
|
||||
#### 我们做了什么
|
||||
|
||||
我们将 `views.py` 文件中的 `HelloWorld` 视图导入到脚本 `__init__.py` 的顶部。然后我们添加了一个路由-视图对应的列表,作为 `Application` 实例化的第一个参数。每当我们想要在应用程序中声明一个路由时,它必须绑定到一个视图。如果需要,可以对多个路由使用相同的视图,但每个路由必须有一个视图。
|
||||
|
||||
我们可以通过在 `setup.py` 中启用的 `serve_app` 命令来运行应用程序,从而确保这一切都能正常工作。查看 `http://localhost:8888/` 并看到它显示 "Hello, world!"。
|
||||
|
||||
当然,在这个领域中我们还能做更多,也将做更多,但现在让我们来讨论模型吧。
|
||||
|
||||
### 连接数据库
|
||||
|
||||
如果我们想要保留数据,我们需要连接数据库。与 Flask 一样,我们将使用一个特定于框架的 SQLAchemy 变体,名为 [tornado-sqlalchemy][7]。
|
||||
|
||||
为什么要使用它而不是 [SQLAlchemy][8] 呢?好吧,其实 `tornado-sqlalchemy` 具有简单 SQLAlchemy 的所有优点,因此我们仍然可以使用通用的 `Base` 声明模型,并使用我们习以为常的所有列数据类型和关系。除了我们已经从习惯中了解到的,`tornado-sqlalchemy` 还为其数据库查询功能提供了一种可访问的异步模式,专门用于与 Tornado 现有的 I/O 循环一起工作。
|
||||
|
||||
我们通过将 `tornado-sqlalchemy` 和 `psycopg2` 添加到 `setup.py` 到所需包的列表并重新安装包来创建环境。在 `models.py` 中,我们声明了模型。这一步看起来与我们在 Flask 和 Pyramid 中已经看到的完全一样,所以我将跳过全部声明,只列出了 `Task` 模型的必要部分。
|
||||
|
||||
```
|
||||
# 这不是完整的 models.py, 但是足够看到不同点
|
||||
from tornado_sqlalchemy import declarative_base
|
||||
|
||||
Base = declarative_base
|
||||
|
||||
class Task(Base):
|
||||
# 等等,因为剩下的几乎所有的东西都一样 ...
|
||||
```
|
||||
|
||||
我们仍然需要将 `tornado-sqlalchemy` 连接到实际应用程序。在 `__init__.py` 中,我们将定义数据库并将其集成到应用程序中。
|
||||
|
||||
```
|
||||
# __init__.py
|
||||
from tornado.httpserver import HTTPServer
|
||||
from tornado.ioloop import IOLoop
|
||||
from tornado.options import define, options
|
||||
from tornado.web import Application
|
||||
from todo.views import HelloWorld
|
||||
|
||||
# add these
|
||||
import os
|
||||
from tornado_sqlalchemy import make_session_factory
|
||||
|
||||
define('port', default=8888, help='port to listen on')
|
||||
factory = make_session_factory(os.environ.get('DATABASE_URL', ''))
|
||||
|
||||
def main():
|
||||
"""Construct and serve the tornado application."""
|
||||
app = Application([
|
||||
('/', HelloWorld)
|
||||
],
|
||||
session_factory=factory
|
||||
)
|
||||
http_server = HTTPServer(app)
|
||||
http_server.listen(options.port)
|
||||
print('Listening on http://localhost:%i' % options.port)
|
||||
IOLoop.current().start()
|
||||
```
|
||||
|
||||
就像我们在 Pyramid 中传递的会话工厂一样,我们可以使用 `make_session_factory` 来接收数据库 URL 并生成一个对象,这个对象的唯一目的是为视图提供到数据库的连接。然后我们将新创建的 `factory` 传递给 `Application` 对象,并使用 `session_factory` 关键字参数将它绑定到应用程序中。
|
||||
|
||||
最后,初始化和管理数据库与 Flask 和 Pyramid 相同(即,单独的 DB 管理脚本,与 `Base` 对象一起工作等)。它看起来很相似,所以在这里我就不介绍了。
|
||||
|
||||
### 回顾视图
|
||||
|
||||
Hello,World 总是适合学习基础知识,但我们需要一些真实的,特定应用程序的视图。
|
||||
|
||||
让我们从 info 视图开始。
|
||||
|
||||
```
|
||||
# views.py
|
||||
import json
|
||||
from tornado.web import RequestHandler
|
||||
|
||||
class InfoView(RequestHandler):
|
||||
"""只允许 GET 请求"""
|
||||
SUPPORTED_METHODS = ["GET"]
|
||||
|
||||
def set_default_headers(self):
|
||||
"""设置默认响应头为 json 格式的"""
|
||||
self.set_header("Content-Type", 'application/json; charset="utf-8"')
|
||||
|
||||
def get(self):
|
||||
"""列出这个 API 的路由"""
|
||||
routes = {
|
||||
'info': 'GET /api/v1',
|
||||
'register': 'POST /api/v1/accounts',
|
||||
'single profile detail': 'GET /api/v1/accounts/<username>',
|
||||
'edit profile': 'PUT /api/v1/accounts/<username>',
|
||||
'delete profile': 'DELETE /api/v1/accounts/<username>',
|
||||
'login': 'POST /api/v1/accounts/login',
|
||||
'logout': 'GET /api/v1/accounts/logout',
|
||||
"user's tasks": 'GET /api/v1/accounts/<username>/tasks',
|
||||
"create task": 'POST /api/v1/accounts/<username>/tasks',
|
||||
"task detail": 'GET /api/v1/accounts/<username>/tasks/<id>',
|
||||
"task update": 'PUT /api/v1/accounts/<username>/tasks/<id>',
|
||||
"delete task": 'DELETE /api/v1/accounts/<username>/tasks/<id>'
|
||||
}
|
||||
self.write(json.dumps(routes))
|
||||
```
|
||||
|
||||
有什么改变吗?让我们从上往下看。
|
||||
|
||||
我们添加了 `SUPPORTED_METHODS` 类属性,它是一个可迭代对象,代表这个视图所接受的请求方法,其他任何方法都将返回一个 [405][9] 状态码。当我们创建 `HelloWorld` 视图时,我们没有指定它,主要是当时有点懒。如果没有这个类属性,此视图将响应任何试图绑定到该视图的路由的请求。
|
||||
|
||||
我们声明了 `set_default_headers` 方法,它设置 HTTP 响应的默认头。我们在这里声明它,以确保我们返回的任何响应都有一个 `"Content-Type"` 是 `"application/json"` 类型。
|
||||
|
||||
我们将 `json.dumps(some_object)` 添加到 `self.write` 的参数中,因为它可以很容易地构建响应主体的内容。
|
||||
|
||||
现在已经完成了,我们可以继续将它连接到 `__init__.py` 中的主路由。
|
||||
|
||||
```
|
||||
# __init__.py
|
||||
from tornado.httpserver import HTTPServer
|
||||
from tornado.ioloop import IOLoop
|
||||
from tornado.options import define, options
|
||||
from tornado.web import Application
|
||||
from todo.views import InfoView
|
||||
|
||||
# 添加这些
|
||||
import os
|
||||
from tornado_sqlalchemy import make_session_factory
|
||||
|
||||
define('port', default=8888, help='port to listen on')
|
||||
factory = make_session_factory(os.environ.get('DATABASE_URL', ''))
|
||||
|
||||
def main():
|
||||
"""Construct and serve the tornado application."""
|
||||
app = Application([
|
||||
('/', InfoView)
|
||||
],
|
||||
session_factory=factory
|
||||
)
|
||||
http_server = HTTPServer(app)
|
||||
http_server.listen(options.port)
|
||||
print('Listening on http://localhost:%i' % options.port)
|
||||
IOLoop.current().start()
|
||||
```
|
||||
|
||||
我们知道,还需要编写更多的视图和路由。每个都会根据需要放入 `Application` 路由列表中,每个视图还需要一个 `set_default_headers` 方法。在此基础上,我们还将创建 `send_response` 方法,它的作用是将响应与我们想要给响应设置的任何自定义状态码打包在一起。由于每个视图都需要这两个方法,因此我们可以创建一个包含它们的基类,这样每个视图都可以继承基类。这样,我们只需要编写一次。
|
||||
|
||||
```
|
||||
# views.py
|
||||
import json
|
||||
from tornado.web import RequestHandler
|
||||
|
||||
class BaseView(RequestHandler):
|
||||
"""Base view for this application."""
|
||||
|
||||
def set_default_headers(self):
|
||||
"""Set the default response header to be JSON."""
|
||||
self.set_header("Content-Type", 'application/json; charset="utf-8"')
|
||||
|
||||
def send_response(self, data, status=200):
|
||||
"""Construct and send a JSON response with appropriate status code."""
|
||||
self.set_status(status)
|
||||
self.write(json.dumps(data))
|
||||
```
|
||||
|
||||
对于我们即将编写的 `TaskListView` 这样的视图,我们还需要一个到数据库的连接。我们需要 `tornado_sqlalchemy` 中的 `SessionMixin` 在每个视图类中添加一个数据库会话。我们可以将它放在 `BaseView` 中,这样,默认情况下,从它继承的每个视图都可以访问数据库会话。
|
||||
|
||||
```
|
||||
# views.py
|
||||
import json
|
||||
from tornado_sqlalchemy import SessionMixin
|
||||
from tornado.web import RequestHandler
|
||||
|
||||
class BaseView(RequestHandler, SessionMixin):
|
||||
"""Base view for this application."""
|
||||
|
||||
def set_default_headers(self):
|
||||
"""Set the default response header to be JSON."""
|
||||
self.set_header("Content-Type", 'application/json; charset="utf-8"')
|
||||
|
||||
def send_response(self, data, status=200):
|
||||
"""Construct and send a JSON response with appropriate status code."""
|
||||
self.set_status(status)
|
||||
self.write(json.dumps(data))
|
||||
```
|
||||
|
||||
只要我们修改 `BaseView` 对象,在将数据发布到这个 API 时,我们就应该定位到这里。
|
||||
|
||||
当 Tornado(从 v.4.5 开始)使用来自客户端的数据并将其组织起来到应用程序中使用时,它会将所有传入数据视为字节串。但是,这里的所有代码都假设使用 Python 3,因此我们希望使用的唯一字符串是 Unicode 字符串。我们可以为这个 `BaseView` 类添加另一个方法,它的工作是将输入数据转换为 Unicode,然后再在视图的其他地方使用。
|
||||
|
||||
如果我们想要在正确的视图方法中使用它之前转换这些数据,我们可以重写视图类的原生 `prepare` 方法。它的工作是在视图方法运行前运行。如果我们重写 `prepare` 方法,我们可以设置一些逻辑来运行,每当收到请求时,这些逻辑就会执行字节串到 Unicode 的转换。
|
||||
|
||||
```
|
||||
# views.py
|
||||
import json
|
||||
from tornado_sqlalchemy import SessionMixin
|
||||
from tornado.web import RequestHandler
|
||||
|
||||
class BaseView(RequestHandler, SessionMixin):
|
||||
"""Base view for this application."""
|
||||
|
||||
def prepare(self):
|
||||
self.form_data = {
|
||||
key: [val.decode('utf8') for val in val_list]
|
||||
for key, val_list in self.request.arguments.items()
|
||||
}
|
||||
|
||||
def set_default_headers(self):
|
||||
"""Set the default response header to be JSON."""
|
||||
self.set_header("Content-Type", 'application/json; charset="utf-8"')
|
||||
|
||||
def send_response(self, data, status=200):
|
||||
"""Construct and send a JSON response with appropriate status code."""
|
||||
self.set_status(status)
|
||||
self.write(json.dumps(data))
|
||||
```
|
||||
|
||||
如果有任何数据进入,它将在 `self.request.arguments` 字典中找到。我们可以通过键访问该数据库,并将其内容(始终是列表)转换为 Unicode。因为这是基于类的视图而不是基于函数的,所以我们可以将修改后的数据存储为一个实例属性,以便以后使用。我在这里称它为 `form_data`,但它也可以被称为 `potato`。关键是我们可以存储提交给应用程序的数据。
|
||||
|
||||
### 异步视图方法
|
||||
|
||||
现在我们已经构建了 `BaseaView`,我们可以构建 `TaskListView` 了,它会继承 `BaseaView`。
|
||||
|
||||
正如你可以从章节标题中看到的那样,以下是所有关于异步性的讨论。`TaskListView` 将处理返回任务列表的 `GET` 请求和用户给定一些表单数据来创建新任务的 `POST` 请求。让我们首先来看看处理 `GET` 请求的代码。
|
||||
|
||||
```
|
||||
# all the previous imports
|
||||
import datetime
|
||||
from tornado.gen import coroutine
|
||||
from tornado_sqlalchemy import as_future
|
||||
from todo.models import Profile, Task
|
||||
|
||||
# the BaseView is above here
|
||||
class TaskListView(BaseView):
|
||||
"""View for reading and adding new tasks."""
|
||||
SUPPORTED_METHODS = ("GET", "POST",)
|
||||
|
||||
@coroutine
|
||||
def get(self, username):
|
||||
"""Get all tasks for an existing user."""
|
||||
with self.make_session() as session:
|
||||
profile = yield as_future(session.query(Profile).filter(Profile.username == username).first)
|
||||
if profile:
|
||||
tasks = [task.to_dict() for task in profile.tasks]
|
||||
self.send_response({
|
||||
'username': profile.username,
|
||||
'tasks': tasks
|
||||
})
|
||||
```
|
||||
|
||||
这里的第一个主要部分是 `@coroutine` 装饰器,它从 `tornado.gen` 导入。任何具有与调用堆栈的正常流程不同步的部分的 Python 可调用实际上是“协程”。一个可以与其它协程一起运行的协程。在我的家务劳动的例子中,几乎所有的家务活都是一个共同的例行协程。有些人阻止了例行协程(例如,给地板吸尘),但这种例行协程只会阻碍我开始或关心其它任何事情的能力。它没有阻止已经启动的任何其他协程继续进行。
|
||||
|
||||
Tornado 提供了许多方法来构建一个利用协程的应用程序,包括允许我们设置函数调用锁,同步异步协程的条件,以及手动修改控制 I/O 循环的事件系统。
|
||||
|
||||
这里使用 `@coroutine` 装饰器的唯一条件是允许 `get` 方法将 SQL 查询作为后台进程,并在查询完成后恢复,同时不阻止 Tornado I/O 循环去处理其他传入的数据源。这就是关于此实现的所有“异步”:带外数据库查询。显然,如果我们想要展示异步 Web 应用程序的魔力和神奇,那么一个任务列表就不是好的展示方式。
|
||||
|
||||
但是,这就是我们正在构建的,所以让我们来看看方法如何利用 `@coroutine` 装饰器。`SessionMixin` 混合到 `BaseView` 声明中,为我们的视图类添加了两个方便的,支持数据库的属性:`session` 和 `make_session`。它们的名字相似,实现的目标也相当相似。
|
||||
|
||||
`self.session` 属性是一个关注数据库的会话。在请求-响应周期结束时,在视图将响应发送回客户端之前,任何对数据库的更改都被提交,并关闭会话。
|
||||
|
||||
`self.make_session` 是一个上下文管理器和生成器,可以动态构建和返回一个全新的会话对象。第一个 `self.session` 对象仍然存在。无论如何,反正 `make_session` 会创建一个新的。`make_session` 生成器还为其自身提供了一个功能,用于在其上下文(即缩进级别)结束时提交和关闭它创建的会话。
|
||||
|
||||
如果你查看源代码,则赋值给 `self.session` 的对象类型与 `self.make_session` 生成的对象类型之间没有区别,不同之处在于它们是如何被管理的。
|
||||
|
||||
使用 `make_session` 上下文管理器,生成的会话仅属于上下文,在该上下文中开始和结束。你可以使用 `make_session` 上下文管理器在同一个视图中打开,修改,提交以及关闭多个数据库会话。
|
||||
|
||||
`self.session` 要简单得多,当你进入视图方法时会话已经打开,在响应被发送回客户端之前会话就已提交。
|
||||
|
||||
虽然[读取文档片段][10]和 [PyPI 示例][11]都说明了上下文管理器的使用,但是没有说明 `self.session` 对象或由 `self.make_session` 生成的 `session` 本质上是不是异步的。当我们启动查询时,我们开始考虑内置于 `tornado-sqlalchemy` 中的异步行为。
|
||||
|
||||
`tornado-sqlalchemy` 包为我们提供了 `as_future` 函数。它的工作是装饰 `tornado-sqlalchemy` 会话构造的查询并 yield 其返回值。如果视图方法用 `@coroutine` 装饰,那么使用 `yield as_future(query)` 模式将使封装的查询成为一个异步后台进程。I/O 循环会接管等待查询的返回值和 `as_future` 创建的 `future` 对象的解析。
|
||||
|
||||
要访问 `as_future(query)` 的结果,你必须从它 `yield`。否则,你只能获得一个未解析的生成器对象,并且无法对查询执行任何操作。
|
||||
|
||||
这个视图方法中的其他所有内容都与之前课堂上的类似,与我们在 Flask 和 Pyramid 中看到的内容类似。
|
||||
|
||||
`post` 方法看起来非常相似。为了保持一致性,让我们看一下 `post` 方法以及它如何处理用 `BaseView` 构造的 `self.form_data`。
|
||||
|
||||
```
|
||||
@coroutine
|
||||
def post(self, username):
|
||||
"""Create a new task."""
|
||||
with self.make_session() as session:
|
||||
profile = yield as_future(session.query(Profile).filter(Profile.username == username).first)
|
||||
if profile:
|
||||
due_date = self.form_data['due_date'][0]
|
||||
task = Task(
|
||||
name=self.form_data['name'][0],
|
||||
note=self.form_data['note'][0],
|
||||
creation_date=datetime.now(),
|
||||
due_date=datetime.strptime(due_date, '%d/%m/%Y %H:%M:%S') if due_date else None,
|
||||
completed=self.form_data['completed'][0],
|
||||
profile_id=profile.id,
|
||||
profile=profile
|
||||
)
|
||||
session.add(task)
|
||||
self.send_response({'msg': 'posted'}, status=201)
|
||||
```
|
||||
|
||||
正如我所说,这是我们所期望的:
|
||||
|
||||
* 与我们在 `get` 方法中看到的查询模式相同
|
||||
* 构造一个新的 `Task` 对象的实例,用 `form_data` 的数据填充
|
||||
* 添加新的 `Task` 对象(但不提交,因为它由上下文管理器处理!)到数据库会话
|
||||
* 将响应发送给客户端
|
||||
|
||||
这样我们就有了 Tornado web 应用程序的基础。其他内容(例如,数据库管理和更多完整应用程序的视图)实际上与我们在 Flask 和 Pyramid 应用程序中看到的相同。
|
||||
|
||||
### 关于使用合适的工具完成合适的工作的一点想法
|
||||
|
||||
在我们继续浏览这些 Web 框架时,我们开始看到它们都可以有效地处理相同的问题。对于像这样的待办事项列表,任何框架都可以完成这项任务。但是,有些 Web 框架比其它框架更适合某些工作,这具体取决于对你来说什么“更合适”和你的需求。
|
||||
|
||||
虽然 Tornado 显然能够处理 Pyramid 或 Flask 可以处理的相同工作,但将它用于这样的应用程序实际上是一种浪费,这就像开车从家走一个街区(to 校正:这里意思应该是从家开始走一个街区只需步行即可)。是的,它可以完成“旅行”的工作,但短途旅行不是你选择汽车而不是自行车或者使用双脚的原因。
|
||||
|
||||
根据文档,Tornado 被称为 “Python Web 框架和异步网络库”。在 Python Web 框架生态系统中很少有人喜欢它。如果你尝试完成的工作需要(或将从中获益)以任何方式,形状或形式的异步性,使用 Tornado。如果你的应用程序需要处理多个长期连接,同时又不想牺牲太多性能,选择 Tornado。如果你的应用程序是多个应用程序,并且需要线程感知以准确处理数据,使用 Tornado。这是它最有效的地方。
|
||||
|
||||
用你的汽车做“汽车的事情”,使用其他交通工具做其他事情。
|
||||
|
||||
### 向前看,进行一些深度检查
|
||||
|
||||
谈到使用合适的工具来完成合适的工作,在选择框架时,请记住应用程序的范围和规模,包括现在和未来。到目前为止,我们只研究了适用于中小型 Web 应用程序的框架。本系列的下一篇也是最后一篇将介绍最受欢迎的 Python 框架之一 Django,它适用于可能会变得更大的大型应用程序。同样,尽管它在技术上能够并且将会处理待办事项列表问题,但请记住,这不是它的真正用途。我们仍然会通过它来展示如何使用它来构建应用程序,但我们必须牢记框架的意图以及它是如何反映在架构中的:
|
||||
|
||||
* **Flask:** 适用于小型,简单的项目。它可以使我们轻松地构建视图并将它们快速连接到路由,它可以简单地封装在一个文件中。
|
||||
|
||||
* **Pyramid:** 适用于可能增长的项目。它包含一些配置来启动和运行。应用程序组件的独立领域可以很容易地划分并构建到任意深度,而不会忽略中央应用程序。
|
||||
|
||||
* **Tornado:** 适用于受益于精确和有意识的 I/O 控制的项目。它允许协程,并轻松公开可以控制如何接收请求或发送响应以及何时发生这些操作的方法。
|
||||
|
||||
* **Django:**(我们将会看到)意味着可能会变得更大的东西。它有着非常庞大的生态系统,包括大量插件和模块。它非常有主见的配置和管理,以保持所有不同部分在同一条线上。
|
||||
|
||||
无论你是从本系列的第一篇文章开始阅读,还是稍后才加入的,都要感谢阅读!请随意留下问题或意见。下次再见时,我手里会拿着 Django。
|
||||
|
||||
### 感谢 Python BDFL
|
||||
|
||||
我必须把功劳归于它应得的地方,非常感谢 [Guido van Rossum][12],不仅仅是因为他创造了我最喜欢的编程语言。
|
||||
|
||||
在 [PyCascades 2018][13] 期间,我很幸运的不仅给了基于这个文章系列的演讲,而且还被邀请参加了演讲者的晚宴。整个晚上我都坐在 Guido 旁边,不停地问他问题。其中一个问题是,在 Python 中异步到底是如何工作的,但他没有一点大惊小怪,而是花时间向我解释,让我开始理解这个概念。他后来[推特给我][14]发了一条消息:是用于学习异步 Python 的广阔资源。我随后在三个月内阅读了三次,然后写了这篇文章。你真是一个非常棒的人,Guido!
|
||||
|
||||
--------------------------------------------------------------------------------
|
||||
|
||||
via: https://opensource.com/article/18/6/tornado-framework
|
||||
|
||||
作者:[Nicholas Hunt-Walker][a]
|
||||
选题:[lujun9972][b]
|
||||
译者:[MjSeven](https://github.com/MjSeven)
|
||||
校对:[校对者ID](https://github.com/校对者ID)
|
||||
|
||||
本文由 [LCTT](https://github.com/LCTT/TranslateProject) 原创编译,[Linux中国](https://linux.cn/) 荣誉推出
|
||||
|
||||
[a]: https://opensource.com/users/nhuntwalker
|
||||
[b]: https://github.com/lujun9972
|
||||
[1]: https://opensource.com/article/18/5/pyramid-framework
|
||||
[2]: https://opensource.com/article/18/4/flask
|
||||
[3]: https://tornado.readthedocs.io/en/stable/
|
||||
[4]: https://realpython.com/python-gil/
|
||||
[5]: https://en.wikipedia.org/wiki/Thread_(computing)
|
||||
[6]: https://www.nginx.com/
|
||||
[7]: https://tornado-sqlalchemy.readthedocs.io/en/latest/
|
||||
[8]: https://www.sqlalchemy.org/
|
||||
[9]: https://en.wikipedia.org/wiki/List_of_HTTP_status_codes#4xx_Client_errors
|
||||
[10]: https://tornado-sqlalchemy.readthedocs.io/en/latest/#usage
|
||||
[11]: https://pypi.org/project/tornado-sqlalchemy/#description
|
||||
[12]: https://www.twitter.com/gvanrossum
|
||||
[13]: https://www.pycascades.com
|
||||
[14]: https://twitter.com/gvanrossum/status/956186585493458944
|
Loading…
Reference in New Issue
Block a user