mirror of
https://github.com/LCTT/TranslateProject.git
synced 2025-01-25 23:11:02 +08:00
Update and rename sources/tech/20160511 An introduction to data processing with Cassandra and Spark.md to translated/tech/20160511 An introduction to data processing with Cassandra and Spark.md
This commit is contained in:
parent
2ebc5a38e9
commit
47c03d6c30
@ -1,51 +0,0 @@
|
||||
Translating KevinSJ
|
||||
An introduction to data processing with Cassandra and Spark
|
||||
==============================================================
|
||||
|
||||

|
||||
|
||||
|
||||
There's been a huge surge of interest around the Apache Cassandra database due to the increasing uptime and performance demands of modern cloud applications.
|
||||
|
||||
So, what is Apache Cassandra? A distributed OLTP database built for high availability and linear scalability. When people ask what Cassandra is used for, think about the type of system you want close to the customer. This is ultimately the system that our users interact with. Applications that must always be available: product catalogs, IoT, medical systems, and mobile applications. In these categories downtime can mean loss of revenue or even more dire outcomes depending on your specific use case. Netflix was one of the earliest adopters of this project, which was open sourced in 2008, and their contributions, along with successes, put it on the radar of the masses.
|
||||
|
||||
Cassandra became a top level Apache Software Foundation project in 2010 and has been riding the wave in popularity since then. Now even knowledge in Cassandra gets you serious returns in the job market. It's both crazy and awesome to consider a NoSQL and open source technology could perform this sort of disruption next to the giants of enterprise SQL. This begs the question, what makes it so popular?
|
||||
|
||||
Cassandra has the ability to be always on in spite of massive hardware and network failures by utilizing a design first widely discussed in [the Dynamo paper from Amazon][1]. By using a peer to peer model, with no single point of failure, we can survive rack failure and even complete network partitions. We can deal with an entire data center failure without impacting our customer's experience. A distributed system that plans for failure is a properly planned distributed system, because frankly, failures are just going to happen. With Cassandra, we accept that cruel fact of life, and bake it into the database's architecture and functionality.
|
||||
|
||||
We know what you’re thinking, "But, I’m coming from a relational background, isn't this going to be a daunting transition?" The answer is somewhat yes and no. Data modeling with Cassandra will feel familiar to developers coming from the relational world. We use tables to model our data, and CQL, the Cassandra Query Language, to query the database. However, unlike SQL, Cassandra supports more complex data structures such as nested and user defined types. For instance, instead of creating a dedicated table to store likes on a cat photo, we can store that data in a collection with the photo itself enabling faster, sequential lookups. That's expressed very naturally in CQL. In our photo table we may want to track the name, URL, and the people that liked the photo.
|
||||
|
||||
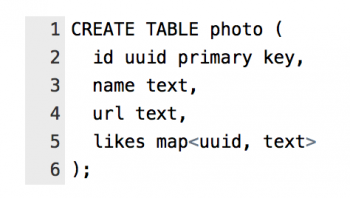
|
||||
|
||||
In a high performance system milliseconds matter for both user experience and for customer retention. Expensive JOIN operations limit our ability to scale out by adding unpredictable network calls. By denormalizing our data so it can be fetched in as few requests as possible, we profit from the trend of decreasing costs in disk space and in return get predictable, high performance applications. We embrace the concept of denormalization with Cassandra because it offers a pretty appealing tradeoff.
|
||||
|
||||
We're obviously not just limited to storing likes on cat photos. Cassandra is a optimized for high write throughput. This makes it the perfect solution for big data applications where we’re constantly ingesting data. Time series and IoT use cases are growing at a steady rate in both demand and appearance in the market, and we're continuously finding ways to utilize the data we collect to improve our technological application.
|
||||
|
||||
This brings us to the next step, we've talked about storing our data in a modern, cost-effective fashion, but how do we get even more horsepower? Meaning, once we've collected all that data, what do we do with it? How can we analyze hundreds of terabytes efficiently? How can we react to information we're receiving in real-time, making decisions in seconds rather than hours? Enter Apache Spark.
|
||||
|
||||
Spark is the next step in the evolution of big data processing. Hadoop and MapReduce were revolutionary projects, giving the big data world an opportunity to crunch all the data we've collected. Spark takes our big data analysis to the next level by drastically improving performance and massively decreasing code complexity. Through Spark, we can perform massive batch processing calculations, react quickly to stream processing, make smart decisions through machine learning, and understand complex, recursive relationships through graph traversals. It’s not just about offering your customers a fast and reliable connection to their application (which is what Cassandra offers), it's also about being able to leverage insights from the data Cassandra stores to make more intelligent business decisions and better cater to customer needs.
|
||||
|
||||
You can check out the [Spark-Cassandra Connector][2] (open source) and give it a shot. To learn more about both technologies, we highly recommend the free self-paced courses on [DataStax Academy][3].
|
||||
|
||||
Have fun digging in and learning some killer new technology! If you want to learn more, check out our [OSCON tutorial][4], with a hands on exploration into the worlds of both Cassandra and Spark.
|
||||
|
||||
We also love taking questions on Twitter, so give us a shout and we’ll try to help: [Dani][5] and [Jon][6].
|
||||
|
||||
--------------------------------------------------------------------------------
|
||||
|
||||
via: https://opensource.com/life/16/5/basics-cassandra-and-spark-data-processing
|
||||
|
||||
作者:[Jon Haddad][a],[Dani Traphagen][b]
|
||||
译者:[译者ID](https://github.com/译者ID)
|
||||
校对:[校对者ID](https://github.com/校对者ID)
|
||||
|
||||
本文由 [LCTT](https://github.com/LCTT/TranslateProject) 原创编译,[Linux中国](https://linux.cn/) 荣誉推出
|
||||
|
||||
[a]: https://twitter.com/rustyrazorblade
|
||||
[b]: https://opensource.com/users/dtrapezoid
|
||||
[1]: http://www.allthingsdistributed.com/files/amazon-dynamo-sosp2007.pdf
|
||||
[2]: https://github.com/datastax/spark-cassandra-connector
|
||||
[3]: https://academy.datastax.com/
|
||||
[4]: http://conferences.oreilly.com/oscon/open-source-us/public/schedule/detail/49162
|
||||
[5]: https://twitter.com/dtrapezoid
|
||||
[6]: https://twitter.com/rustyrazorblade
|
@ -0,0 +1,49 @@
|
||||
Cassandra 和 Spark 数据处理入门
|
||||
==============================================================
|
||||
|
||||

|
||||
|
||||
Apache Cassandra 数据库近来引起了很多的兴趣,这主要源于现代云端软件对于可用性及性能方面的要求。
|
||||
|
||||
那么,Apache Cassandra 是什么?它是一种为高可用性及线性可扩展性优化的分布式的联机交易处理 (OLTP) 数据库。当人们想知道 Cassandra 的用途时,可以想想你想要的离客户近的系统。这j最终是我们的用户进行交互的系统。需要保证实时可用的程序:产品目录,IoT,医疗系统,以及移动应用。对这些程序而言,下线时间意味着利润降低甚至导致其他更坏的结果。Netfilix 是这个于2008年开源的项目的早期使用者,他们对此项目的贡献以及带来的成功让这个项目名声大噪。
|
||||
|
||||
Cassandra 于2010年成为了 Apache 软件基金会的顶级项目,在这之后就开始变得流行。现在,只要你有 Cassadra 的相关知识,找工作时就能轻松不少。光是想想一个 NoSQL 语言和开源技术能达到如此企业级 SQL 的高度就觉得这是十分疯狂而又不可思议的。这引出了一个问题。是什么让它如此的流行?
|
||||
|
||||
因为采用了首先在[亚马逊发表的 Dynamo 论文][1]提出的设计,Cassandra 有能力在大规模的硬件及网络故障时保持实时在线。由于采用了点对点模式,在没有单点故障的情况下,我们能幸免于机架故障甚至完全网络分区。我们能在不影响用户体验的前提下处理数据中心故障。一个能考虑到故障的分布式系统才是一个没有后顾之忧的分布式系统,因为老实说,故障是迟早会发生的。有了 Cassandra, 我们可疑直面残酷的生活并将之融入数据库的结构和功能中。
|
||||
|
||||
|
||||
|
||||
我们能猜到你现在在想什么,“但我只有关系数据库相关背景,难道这样的转变不会很困难吗?"这问题的答案介于是和不是之间。使用 Cassandra 建立数据模型对有关系数据库背景的开发者而言是轻车熟路。我们使用表格来建立数据模型,并使用 CQL 或者 Cassandra 查询语言来查询数据库。然而,与 SQL 不同的是,Cassandra 支持更加复杂的数据结构,例如多重和用户自定义类型。举个例子,当要储存对一个小猫照片的点赞数目时,我们可以将整个数据储存在一个包含照片本身的集合之中从而获得更快的顺序查找而不是建立一个独立的表。这样的表述在 CQL 中十分的自然。在我们照片表中,我们需要记录名字,URL以及给此照片点赞过的人。
|
||||
|
||||
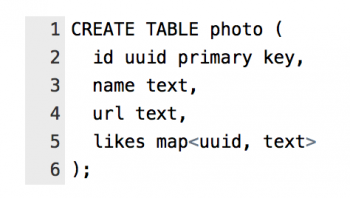
|
||||
|
||||
在一个高性能系统中,毫秒对用户体验和客户保留都能产生影响。昂贵的 JOIN 制约了我们通过增加不可预见的网络调用而扩容的能力。当我们将数据反规范化使其能在尽可能少的请求中被获取到时,我们即可从磁盘空间花费的降低中获益并获得可预测的,高性能应用。我们将反规范化同 Cassandra 一同介绍是因为它提供了很有吸引力的的折衷方案。
|
||||
|
||||
很明显,我们不会局限于对于小猫照片的点赞数量。Canssandra 是一款个为并发高写入优化的方案。这使其成为需要时常吞吐数据的大数据应用的理想解决方案。市场上的时序和 IoT 的使用场景正在以稳定的速度在需求和亮相方面增加,我们也在不断探寻优化我们所收集到的数据以求提升我们的技术应用(注:这句翻的非常别扭,求校队)
|
||||
|
||||
|
||||
这就引出了我们的下一步,我们已经提到了如何以一种现代的,性价比高的方式储存数据,但我们应该如何获得更多的马力呢?具体而言,当我们收集到了所需的数据,我们应该怎样处理呢?如何才能有效的分析几百 TB 的数据呢?如何才能在实时的对我们所收集到的信息进行反馈并在几秒而不是几小时的时间利作出决策呢?Apache Spark 将给我们答案。
|
||||
|
||||
|
||||
Spark 是大数据变革中的下一步。 Hadoop 和 MapReduce 都是革命性的产品,他们让大数据界获得了分析所有我们所取得的数据的机会。Spark 对性能的大幅提升及对代码复杂度的大幅降低则将大数据分析提升到了另一个高度。通过 Spark,我们能大批量的处理计算,对流处理进行快速反映,通过机器学习作出决策并理解通过对图的遍历理解复杂的递归关系。这并非只是为你的客户提供与快捷可靠的应用程序连接(Cassandra 已经提供了这样的功能),这更是能一探 Canssandra 所储存的数据并作出更加合理的商业决策同时更好地满足客户需求。
|
||||
|
||||
你可以看看 [Spark-Cassandra Connector][2] (open source) 并动手试试。若想了解更多关于这两种技术的信息,我们强烈推荐名为 [DataStax Academy][3] 的自学课程
|
||||
|
||||
--------------------------------------------------------------------------------
|
||||
|
||||
via: https://opensource.com/life/16/5/basics-cassandra-and-spark-data-processing
|
||||
|
||||
作者:[Jon Haddad][a],[Dani Traphagen][b]
|
||||
译者:[KevinSJ](https://github.com/KevinSJ)
|
||||
校对:[校对者ID](https://github.com/校对者ID)
|
||||
|
||||
本文由 [LCTT](https://github.com/LCTT/TranslateProject) 原创编译,[Linux中国](https://linux.cn/) 荣誉推出
|
||||
|
||||
[a]: https://twitter.com/rustyrazorblade
|
||||
[b]: https://opensource.com/users/dtrapezoid
|
||||
[1]: http://www.allthingsdistributed.com/files/amazon-dynamo-sosp2007.pdf
|
||||
[2]: https://github.com/datastax/spark-cassandra-connector
|
||||
[3]: https://academy.datastax.com/
|
||||
[4]: http://conferences.oreilly.com/oscon/open-source-us/public/schedule/detail/49162
|
||||
[5]: https://twitter.com/dtrapezoid
|
||||
[6]: https://twitter.com/rustyrazorblade
|
Loading…
Reference in New Issue
Block a user