mirror of
https://github.com/LCTT/TranslateProject.git
synced 2025-01-13 22:30:37 +08:00
Merge pull request #2008 from coloka/master
【翻译完成】20141112 How to Debug CPU Regressions Using Flame Graphs.md
This commit is contained in:
commit
424da1b9fb
@ -1,155 +0,0 @@
|
||||
翻译中 by coloka
|
||||
How to Debug CPU Regressions Using Flame Graphs
|
||||
================================================================================
|
||||
How quickly can you debug a CPU performance regression? If your environment is complex and changing quickly, this becomes challenging with existing tools. If it takes a week to root cause a regression, the code may have changed multiple times, and now you have new regressions to debug.
|
||||
|
||||
Debugging CPU usage is easy in most cases, thanks to [CPU flame graphs][1]. To debug regressions, I would load before and after flame graphs in separate browser tabs, and then blink between them like searching for [Pluto][2]. It got the job done, but I wondered about a better way.
|
||||
|
||||
Introducing **red/blue differential flame graphs**:
|
||||
|
||||
<p><object data="http://www.brendangregg.com/blog/images/2014/zfs-flamegraph-diff.svg" type="image/svg+xml" width=720 height=296>
|
||||
<img src="http://www.brendangregg.com/blog/images/2014/zfs-flamegraph-diff.svg" width=720 />
|
||||
</object></p>
|
||||
|
||||
This is an interactive SVG (direct [link][3]). The color shows **red for growth**, and **blue for reductions**.
|
||||
|
||||
The size and shape of the flame graph is the same as a CPU flame graph for the second profile (y-axis is stack depth, x-axis is population, and the width of each frame is proportional to its presence in the profile; the top edge is what's actually running on CPU, and everything beneath it is ancestry.)
|
||||
|
||||
In this example, a workload saw a CPU increase after a system update. Here's the CPU flame graph ([SVG][4]):
|
||||
|
||||
<p><object data="http://www.brendangregg.com/blog/images/2014/zfs-flamegraph-after.svg" type="image/svg+xml" width=720 height=296>
|
||||
<img src="http://www.brendangregg.com/blog/images/2014/zfs-flamegraph-after.svg" width=720 />
|
||||
</object></p>
|
||||
|
||||
Normally, the colors are picked at random to differentiate frames and towers. Red/blue differential flame graphs use color to show the difference between two profiles.
|
||||
|
||||
The deflate_slow() code and children were running more in the second profile, highlighted earlier as red frames. The cause was that ZFS compression was enabled in the system update, which it wasn't previously.
|
||||
|
||||
While this makes for a clear example, I didn't really need a differential flame graph for this one. Imagine tracking down subtle regressions, of less than 5%, and where the code is also more complex.
|
||||
|
||||
### Red/Blue Differential Flame Graphs ###
|
||||
|
||||
I've had many discussions about this for years, and finally wrote an implementation that I hope makes sense. It works like this:
|
||||
|
||||
1. Take stack profile 1.
|
||||
1. Take stack profile 2.
|
||||
1. Generate a flame graph using 2. (This sets the width of all frames using profile 2.)
|
||||
1. Colorize the flame graph using the "2 - 1" delta. If a frame appeared more times in 2, it is red, less times, it is blue. The saturation is relative to the delta.
|
||||
|
||||
The intent is for use with before & after profiles, such as for **non-regression testing** or benchmarking code changes. The flame graph is drawn using the "after" profile (such that the frame widths show the current CPU consumption), and then colorized by the delta to show how we got there.
|
||||
|
||||
The colors show the difference that function directly contributed (eg, being on-CPU), not its children.
|
||||
|
||||
### Generation ###
|
||||
|
||||
I've pushed a simple implementation to github (see [FlameGraph][5]), which includes a new program, difffolded.pl. To show how it works, here are the steps using Linux [perf_events][6] (you can use other profilers).
|
||||
|
||||
#### Collect profile 1: ####
|
||||
|
||||
# perf record -F 99 -a -g -- sleep 30
|
||||
# perf script > out.stacks1
|
||||
|
||||
#### Some time later (or after a code change), collect profile 2: ####
|
||||
|
||||
# perf record -F 99 -a -g -- sleep 30
|
||||
# perf script > out.stacks2
|
||||
|
||||
#### Now fold these profile files, and generate a differential flame graph: ####
|
||||
|
||||
$ git clone --depth 1 http://github.com/brendangregg/FlameGraph
|
||||
$ cd FlameGraph
|
||||
$ ./stackcollapse-perf.pl ../out.stacks1 > out.folded1
|
||||
$ ./stackcollapse-perf.pl ../out.stacks2 > out.folded2
|
||||
$ ./difffolded.pl out.folded1 out.folded2 | ./flamegraph.pl > diff2.svg
|
||||
|
||||
difffolded.pl operates on the "folded" style of stack profiles, which are generated by the stackcollapse collection of tools (see the files in [FlameGraph][7]). It emits a three column output, with the folded stack trace and two value columns, one for each profile. Eg:
|
||||
|
||||
func_a;func_b;func_c 31 33
|
||||
[...]
|
||||
|
||||
This would mean the stack composed of "func_a()->func_b()->func_c()" was seen 31 times in profile 1, and in 33 times in profile 2. If flamegraph.pl is handed this three column input, it will automatically generate a red/blue differential flame graph.
|
||||
|
||||
### Options ###
|
||||
|
||||
Some options you'll want to know about:
|
||||
|
||||
**difffolded.pl -n**: This normalizes the first profile count to match the second. If you don't do this, and take profiles at different times of day, then all the stack counts will naturally differ due to varied load. Everything will look red if the load increased, or blue if load decreased. The -n option balances the first profile, so you get the full red/blue spectrum.
|
||||
|
||||
**difffolded.pl -x**: This strips hex addresses. Sometimes profilers can't translate addresses into symbols, and include raw hex addresses. If these addresses differ between profiles, then they'll be shown as differences, when in fact the executed function was the same. Fix with -x.
|
||||
|
||||
**flamegraph.pl --negate**: Inverts the red/blue scale. See the next section.
|
||||
|
||||
### Negation ###
|
||||
|
||||
While my red/blue differential flame graphs are useful, there is a problem: if code paths vanish completely in the second profile, then there's nothing to color blue. You'll be looking at the current CPU usage, but missing information on how we got there.
|
||||
|
||||
One solution is to reverse the order of the profiles and draw a negated flame graph differential. Eg:
|
||||
|
||||
<p><object data="http://www.brendangregg.com/blog/images/2014/zfs-flamegraph-negated.svg" type="image/svg+xml" width=720 height=296>
|
||||
<img src="http://www.brendangregg.com/blog/images/2014/zfs-flamegraph-negated.svg" width=720 />
|
||||
</object></p>
|
||||
|
||||
Now the widths show the first profile, and the colors show what will happen. The blue highlighting on the right shows we're about to spend a lot less time in the CPU idle path. (Note that I usually filter out cpu_idle from the folded files, by including a grep -v cpu_idle.)
|
||||
|
||||
This also highlights the vanishing code problem (or rather, doesn't highlight), as since compression wasn't enabled in the "before" profile, there is nothing to color red.
|
||||
|
||||
This was generated using:
|
||||
|
||||
$ ./difffolded.pl out.folded2 out.folded1 | ./flamegraph.pl --negate > diff1.svg
|
||||
|
||||
Which, along with the earlier diff2.svg, gives us:
|
||||
|
||||
- **diff1.svg**: widths show the before profile, colored by what WILL happen
|
||||
- **diff2.svg**: widths show the after profile, colored by what DID happen
|
||||
|
||||
If I were to automate this for non-regression testing, I'd generate and show both side by side.
|
||||
|
||||
### CPI Flame Graphs ###
|
||||
|
||||
I first used this code for my [CPI flame graphs][8], where instead of doing a difference between two profiles, I showed the difference between CPU cycles and stall cycles, which highlights what the CPUs were doing.
|
||||
|
||||
### Other Differential Flame Graphs ###
|
||||
|
||||
[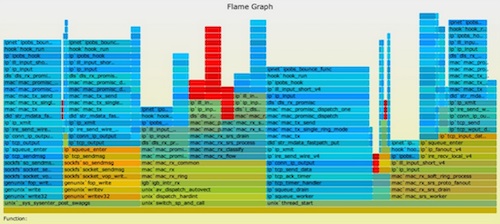][9]
|
||||
|
||||
There's other ways flame graph differentials can be done. [Robert Mustacchi][10] experimented with [differentials][11] a while ago, and used an approach similar to a colored code review: only the difference is shown, colored red for added (increased) code paths, and blue for removed (decreased) code paths. The key difference is that the frame widths are now relative to the size of the difference only. An example is on the right. It's a good idea, but in practice I found it a bit weird, and hard to follow without the bigger picture context: a standard flame graph showing the full profile.
|
||||
|
||||
[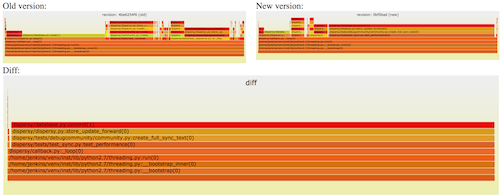][12]
|
||||
|
||||
Cor-Paul Bezemer has created [flamegraphdiff][13], which shows the profile difference using three flame graphs at the same time: the standard before and after flame graphs, and then a differential flame graph where the widths show the difference. See the [example][14]. You can mouse-over frames in the differential, which highlights frames in all profiles. This solves the context problem, since you can see the standard flame graph profiles.
|
||||
|
||||
My red/blue flame graphs, Robert's hue differential, and Cor-Paul's triple-view, all have their strengths. These could be combined: the top two flame graphs in Cor-Paul's view could be my diff1.svg and diff2.svg. Then the bottom flame graph colored using Robert's approach. For consistency, the bottom flame graph could use the same palette range as mine: blue->white->red.
|
||||
|
||||
Flame graphs are spreading, and are now used by many companies. I wouldn't be surprised if there were already other implementations of flame graph differentials I didn't know about. (Leave a comment!)
|
||||
|
||||
### Conclusion ###
|
||||
|
||||
If you have problems with performance regressions, red/blue differential flame graphs may be the quickest way to find the root cause. These take a normal flame graph and then use colors to show the difference between two profiles: red for greater samples, and blue for fewer. The size and shape of the flame graph shows the current ("after") profile, so that you can easily see where the samples are based on the widths, and then the colors show how we got there: the profile difference.
|
||||
|
||||
These differential flame graphs could also be generated by a nightly non-regression test suite, so that performance regressions can be quickly debugged after the fact.
|
||||
|
||||
--------------------------------------------------------------------------------
|
||||
|
||||
via: http://www.brendangregg.com/blog/2014-11-09/differential-flame-graphs.html
|
||||
|
||||
作者:[Brendan Gregg][a]
|
||||
译者:[译者ID](https://github.com/译者ID)
|
||||
校对:[校对者ID](https://github.com/校对者ID)
|
||||
|
||||
本文由 [LCTT](https://github.com/LCTT/TranslateProject) 原创翻译,[Linux中国](http://linux.cn/) 荣誉推出
|
||||
|
||||
[a]:http://www.linux.com/community/forums/person/60160
|
||||
[1]:http://www.brendangregg.com/FlameGraphs/cpuflamegraphs.html
|
||||
[2]:http://en.wikipedia.org/wiki/Planets_beyond_Neptune#Discovery_of_Pluto
|
||||
[3]:http://www.brendangregg.com/blog/images/2014/zfs-flamegraph-diff.svg
|
||||
[4]:http://www.brendangregg.com/blog/images/2014/zfs-flamegraph-after.svg
|
||||
[5]:https://github.com/brendangregg/FlameGraph
|
||||
[6]:http://www.brendangregg.com/perf.html
|
||||
[7]:https://github.com/brendangregg/FlameGraph
|
||||
[8]:http://www.brendangregg.com/blog/2014-10-31/cpi-flame-graphs.html
|
||||
[9]:http://www.slideshare.net/brendangregg/blazing-performance-with-flame-graphs/167
|
||||
[10]:http://dtrace.org/blogs/rm
|
||||
[11]:http://www.slideshare.net/brendangregg/blazing-performance-with-flame-graphs/167
|
||||
[12]:https://github.com/corpaul/flamegraphdiff
|
||||
[13]:http://corpaul.github.io/flamegraphdiff/
|
||||
[14]:http://corpaul.github.io/flamegraphdiff/demos/dispersy/dispersy_diff.html
|
@ -1,3 +1,4 @@
|
||||
翻译中 by coloka
|
||||
How to visualize memory usage on Linux
|
||||
================================================================================
|
||||
Lack of sufficient physical memory can significantly hamper the performance of Linux desktop and server environments. When your desktop is sluggish, one of the first things to do is to free up RAMs. Memory usage is even more critical in multi-user shared hosting or mission-critical server environments, where different users or application threads constantly compete for more memory.
|
||||
|
@ -0,0 +1,149 @@
|
||||
使用火焰图分析CPU性能回退问题
|
||||
================================================================================
|
||||
你能快速定位CPU性能回退的问题么? 如果你的工作环境非常复杂且变化快速,那么使用现有的工具是来定位这类问题是很具有挑战性的。当你花掉数周时间把根因找到时,代码已经又变更了好几轮,新的性能问题又冒了出来。
|
||||
|
||||
辛亏有了[CPU火焰图][1](flame graphs),CPU使用率的问题一般都比较好定位。但要处理性能回退问题,就要在修改前后的火焰图间,不断切换对比,来找出问题所在,这感觉就是像在太阳系中搜寻冥王星。虽然,这种方法可以解决问题,但我觉得应该会有更好的办法。
|
||||
|
||||
所以,下面就隆重介绍**红/蓝差分火焰图(red/blue differential flame graphs)**:
|
||||
|
||||
<p><object data="http://www.brendangregg.com/blog/images/2014/zfs-flamegraph-diff.svg" type="image/svg+xml" width=720 height=296>
|
||||
<img src="http://www.brendangregg.com/blog/images/2014/zfs-flamegraph-diff.svg" width=720 />
|
||||
</object></p>
|
||||
|
||||
上面是一副交互式SVG格式图片([链接][3])。图中使用了两种颜色来表示状态,**红色表示增长**,**蓝色表示衰减**。
|
||||
|
||||
这张火焰图中各火焰的形状和大小都是和第二次抓取的profile文件对应的CPU火焰图是相同的。(其中,y轴表示栈的深度,x轴表示样本的总数,栈帧的宽度表示了profile文件中该函数出现的比例,最顶层表示正在运行的函数,再往下就是调用它的栈)
|
||||
|
||||
在下面这个案例展示了,在系统升级后,一个工作负载的CPU使用率上升了。 下面是对应的CPU火焰图([SVG格式][4])
|
||||
|
||||
<p><object data="http://www.brendangregg.com/blog/images/2014/zfs-flamegraph-after.svg" type="image/svg+xml" width=720 height=296>
|
||||
<img src="http://www.brendangregg.com/blog/images/2014/zfs-flamegraph-after.svg" width=720 />
|
||||
</object></p>
|
||||
|
||||
通常,在标准的火焰图中栈帧和栈塔的颜色是随机选择的。 而在红/蓝差分火焰图中,使用不同的颜色来表示两个profile文件中的差异部分。
|
||||
|
||||
在第二个profile中deflate_slow()函数以及它后续调用的函数运行的次数要比前一次更多,所以在上图中这个栈帧被标为了红色。可以看出问题的原因是ZFS的压缩功能被使能了,而在系统升级前这项功能是关闭的。
|
||||
|
||||
这个例子过于简单,我甚至可以不用差分火焰图也能分析出来。但想象一下,如果是在分析一个微小的性能下降,比如说小于5%,而且代码也更加复杂的时候,问题就为那么好处理了。
|
||||
|
||||
### 红/蓝差分火焰图 ###
|
||||
|
||||
这个事情我已经讨论了好几年了,最终我自己编写了一个我个人认为有价值的实现。它的工作原理是这样的:
|
||||
|
||||
1. 抓取修改前的堆栈profile1文件
|
||||
1. 抓取修改后的堆栈profile2文件
|
||||
1. 使用profile2来生成火焰图。(这样栈帧的宽度就是以profile2文件为基准的)
|
||||
1. 使用“2 - 1”的差异来对火焰图重新上色。上色的原则是,如果栈帧在profile2中出现出现的次数更多,则标为红色,否则标为蓝色。色彩是根据修改前后的差异来填充的。
|
||||
|
||||
这样做的目的是,同时使用了修改前后的profile文件进行对比,在进行功能验证测试或者评估代码修改对性能的影响时,会非常有用。新的火焰图是基于修改后的profile文件生成(所以栈帧的宽度仍然显示了当前的CPU消耗),通过颜色的对比,就可以了解到系统性能差异的原因。
|
||||
|
||||
只有对性能产生直接影响的函数才会标注颜色(比如说,正在运行的函数),它所调用的子函数不会重复标注。
|
||||
|
||||
### 生成红/蓝差分火焰图 ###
|
||||
|
||||
我已经把一个简单的代码实现推送到github上(见[火焰图][5]),其中新增了一个程序脚本,difffolded.pl。为了展示工具是如何工作的,用Linux [perf_events][6] 来演示一下操作步骤。(你也可以使用其他profiler)
|
||||
|
||||
#### 抓取修改前的profile 1文件: ####
|
||||
|
||||
# perf record -F 99 -a -g -- sleep 30
|
||||
# perf script > out.stacks1
|
||||
|
||||
#### 一段时间后 (或者程序代码修改后), 抓取profile 2文件: ####
|
||||
|
||||
# perf record -F 99 -a -g -- sleep 30
|
||||
# perf script > out.stacks2
|
||||
|
||||
#### 现在将 profile 文件进行折叠(fold), 再生成差分火焰图: ####
|
||||
|
||||
$ git clone --depth 1 http://github.com/brendangregg/FlameGraph
|
||||
$ cd FlameGraph
|
||||
$ ./stackcollapse-perf.pl ../out.stacks1 > out.folded1
|
||||
$ ./stackcollapse-perf.pl ../out.stacks2 > out.folded2
|
||||
$ ./difffolded.pl out.folded1 out.folded2 | ./flamegraph.pl > diff2.svg
|
||||
|
||||
difffolded.p只能对“折叠”过的堆栈profile文件进行操作,折叠操作是由前面的stackcollapse系列脚本完成的。(见链接[火焰图][7])。 脚本共输出3列数据,其中一列代表折叠的调用栈,另两列为修改前后profile文件的统计数据。
|
||||
|
||||
func_a;func_b;func_c 31 33
|
||||
[...]
|
||||
|
||||
在上面的例子中"func_a()->func_b()->func_c()" 代表调用栈,这个调用栈在profile1文件中共出现了31次,在profile2文件中共出现了33次。然后,使用flamegraph.pl脚本处理这3列数据,会自动生成一张红/蓝差分火焰图。
|
||||
|
||||
### 其他选项 ###
|
||||
再介绍一些有用的选项:
|
||||
**difffolded.pl -n**:这个选项会把两个profile文件中的数据规范化,使其能相互匹配上。如果你不这样做,抓取到所有栈的统计值肯定会不相同,因为抓取的时间和CPU负载都不同。这样的话,看上去要么就是一片红(负载增加),要么就是一片蓝(负载下降)。-n选项对第一个profile文件进行了平衡,这样你就可以得到完整红/蓝图谱。
|
||||
|
||||
**difffolded.pl -x**: 这个选项会把16进制的地址删掉。 profiler时常会无法将地址转换为符号,这样的话栈里就会有16进制地址。如果这个地址在两个profile文件中不同,这两个栈就会认为是不同的栈,而实际上它们是相同的。遇到这样的问题就用-x选项搞定。
|
||||
|
||||
**flamegraph.pl --negate**: 用于颠倒红/蓝配色。 在下面的章节中,会用到这个功能。
|
||||
|
||||
### 不足之处 ###
|
||||
虽然我的红/蓝差分火焰图很有用,但实际上还是有一个问题:如果一个代码执行路径完全消失了,那么在火焰图中就找不到地方来标注蓝色。你只能看到当前的CPU使用情况,而不知道为什么会变成这样。
|
||||
|
||||
一个办法是,将对比顺序颠倒,画一个相反的差分火焰图。例如:
|
||||
|
||||
<p><object data="http://www.brendangregg.com/blog/images/2014/zfs-flamegraph-negated.svg" type="image/svg+xml" width=720 height=296>
|
||||
<img src="http://www.brendangregg.com/blog/images/2014/zfs-flamegraph-negated.svg" width=720 />
|
||||
</object></p>
|
||||
|
||||
上面的火焰图是以修改前的profile文件为基准,颜色表达了将要发生的情况。右边使用蓝色高亮显示的部分,从中可以看出修改后CPU Idle消耗的CPU时间会变少。(其实,我通常会把cpu_idle给过滤掉,使用命令行grep -v cpu_idle)
|
||||
|
||||
图中把消失的代码也突显了出来(或者应该是说,没有突显),因为修改前并没有使能压缩功能,所以它没有出现在修改前的profile文件了,也就没有了被表为红色的部分。
|
||||
|
||||
下面是对应的命令行:
|
||||
|
||||
$ ./difffolded.pl out.folded2 out.folded1 | ./flamegraph.pl --negate > diff1.svg
|
||||
|
||||
这样,把前面生成diff2.svg一并使用,我们就能得到:
|
||||
|
||||
- **diff1.svg**: 宽度是以修改前profile文件为基准, 颜色表明将要发生的情况
|
||||
- **diff2.svg**: 宽度以修改后的profile文件为基准,颜色表明已经发生的情况
|
||||
|
||||
如果是在做功能验证测试,我会同时生成这两张图。
|
||||
|
||||
### CPI 火焰图 ###
|
||||
这些脚本开始是被使用在[CPI火焰图][8]的分析上。与比较修改前后的profile文件不同,在分析CPI火焰图时,可以分析CPU工作周期与停顿周期的差异变化,这样可以凸显出CPU的工作状态来。
|
||||
|
||||
### 其他的差分火焰图 ###
|
||||
|
||||
[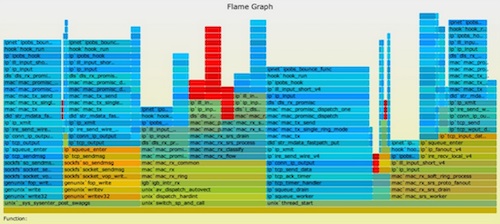][9]
|
||||
|
||||
也有其他人做过类似的工作。[Robert Mustacchi][10]在不久前也做了一些尝试,他使用的方法类似于代码检视时的标色风格:只显示了差异的部分,红色表示新增(上升)的代码路径,蓝色表示删除(下降)的代码路径。一个关键的差别是栈帧的宽度只体现了差异的样本数。右边是一个例子。这个是个很好的主意,但在实际使用中会感觉有点奇怪,因为缺失了完整profile文件的上下文作为背景,这张图显得有些难以理解。
|
||||
|
||||
[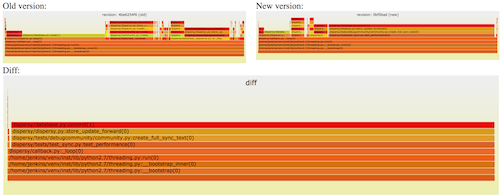][12]
|
||||
Cor-Paul Bezemer也制作了一种差分显示方法[flamegraphdiff][13],他同时将3张火焰图放在同一张图中,修改前后的标准火焰图各一张,下面再补充了一张差分火焰图,但栈帧宽度也是差异的样本数。 上图是一个[例子][14]。在差分图中将鼠标移到栈帧上,3张图中同一栈帧都会被高亮显示。这种方法中补充了两张标准的火焰图,因此解决了上下文的问题。
|
||||
|
||||
我们3人的差分火焰图,都各有所长。三者可以结合起来使用:Cor-Paul方法中上方的两张图,可以用我的diff1.svg 和 diff2.svg。下方的火焰图可以用Robert的方式。为保持一致性,下方的火焰图可以用我的着色方式:蓝->白->红。
|
||||
|
||||
火焰图正在广泛传播中,现在很多公司都在使用它。如果大家知道有其他的实现差分火焰图的方式,我也不会感到惊讶。(请在评论中告诉我)
|
||||
|
||||
### 结论 ###
|
||||
|
||||
如果你遇到了性能回退问题,红/蓝差分火焰图是找到根因的最快方式。这种方式抓取了两张普通的火焰图,然后进行对比,并对差异部分进行标色:红色表示上升,蓝色表示下降。 差分火焰图是以当前(“修改后”)的profile文件作为基准,形状和大小都保持不变。因此你通过色彩的差异就能够很直观的找到差异部分,且可以看出为什么会有这样的差异。
|
||||
|
||||
差分火焰图可以应用到项目的每日构建中,这样性能回退的问题就可以及时地被发现和修正。
|
||||
|
||||
--------------------------------------------------------------------------------
|
||||
|
||||
via: http://www.brendangregg.com/blog/2014-11-09/differential-flame-graphs.html
|
||||
|
||||
作者:[Brendan Gregg][a]
|
||||
译者:[coloka](https://github.com/coloka)
|
||||
校对:[校对者ID](https://github.com/校对者ID)
|
||||
|
||||
本文由 [LCTT](https://github.com/LCTT/TranslateProject) 原创翻译,[Linux中国](http://linux.cn/) 荣誉推出
|
||||
|
||||
[a]:http://www.linux.com/community/forums/person/60160
|
||||
[1]:http://www.brendangregg.com/FlameGraphs/cpuflamegraphs.html
|
||||
[2]:http://en.wikipedia.org/wiki/Planets_beyond_Neptune#Discovery_of_Pluto
|
||||
[3]:http://www.brendangregg.com/blog/images/2014/zfs-flamegraph-diff.svg
|
||||
[4]:http://www.brendangregg.com/blog/images/2014/zfs-flamegraph-after.svg
|
||||
[5]:https://github.com/brendangregg/FlameGraph
|
||||
[6]:http://www.brendangregg.com/perf.html
|
||||
[7]:https://github.com/brendangregg/FlameGraph
|
||||
[8]:http://www.brendangregg.com/blog/2014-10-31/cpi-flame-graphs.html
|
||||
[9]:http://www.slideshare.net/brendangregg/blazing-performance-with-flame-graphs/167
|
||||
[10]:http://dtrace.org/blogs/rm
|
||||
[11]:http://www.slideshare.net/brendangregg/blazing-performance-with-flame-graphs/167
|
||||
[12]:https://github.com/corpaul/flamegraphdiff
|
||||
[13]:http://corpaul.github.io/flamegraphdiff/
|
||||
[14]:http://corpaul.github.io/flamegraphdiff/demos/dispersy/dispersy_diff.html
|
Loading…
Reference in New Issue
Block a user