mirror of
https://github.com/LCTT/TranslateProject.git
synced 2024-12-29 21:41:00 +08:00
commit
3f0afd5722
@ -1,113 +0,0 @@
|
|||||||
[#]: collector: (lujun9972)
|
|
||||||
[#]: translator: (qhwdw)
|
|
||||||
[#]: reviewer: ( )
|
|
||||||
[#]: publisher: ( )
|
|
||||||
[#]: url: ( )
|
|
||||||
[#]: subject: (How to get started in AI)
|
|
||||||
[#]: via: (https://opensource.com/article/18/12/how-get-started-ai)
|
|
||||||
[#]: author: (Gordon Haff https://opensource.com/users/ghaff)
|
|
||||||
|
|
||||||
How to get started in AI
|
|
||||||
======
|
|
||||||
Before you can begin working in artificial intelligence, you need to acquire some human intelligence.
|
|
||||||
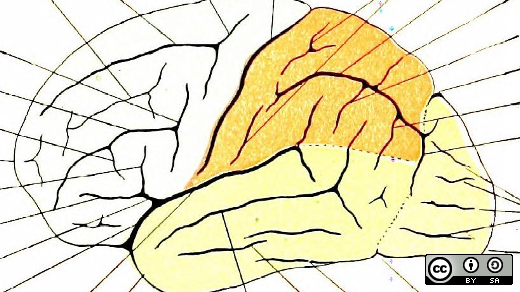
|
|
||||||
|
|
||||||
I've both asked and been asked about the best way to learn more about artificial intelligence (AI). What should I read? What should I watch? I'll get to that. But, first, it's useful to break down this question, given that AI covers a lot of territory.
|
|
||||||
|
|
||||||
One important distinction to draw is between the research side of AI and the applied side. Cassie Kozyrkov of Google [drew this distinction][1] in a talk at the recent O'Reilly Artificial Intelligence Conference in London, and it's a good one.
|
|
||||||
|
|
||||||
Research AI is rather academic in nature and requires a heavy dose of math across a variety of disciplines before you even get to those parts that are specific to AI. This aspect of AI focuses on the algorithms and tools that drive the state of AI forward. For example, what neural network structures might improve vision recognition results? How might we make unsupervised learning a more generally useful approach? Can we find ways to understand better how deep learning pipelines come up with the answers they do?
|
|
||||||
|
|
||||||
Applied AI, on the other hand, is more about using existing tools to obtain useful results. Open source has played a big role here in providing free and often easy-to-use software in a variety of languages. Public cloud providers have also devoted a lot of attention to providing machine learning services, models, and datasets that make the onramp to getting started with AI much simpler than it would be otherwise.
|
|
||||||
|
|
||||||
I'll add at this point that applied AI practitioners shouldn't treat their tools as some sort of black box that spits out answers for mysterious reasons. At a minimum, they need to understand the limits and potential biases of different techniques, models, and data collection approaches. It's just that they don't necessarily need to delve deeply into all the theory underpinning every part of their toolchain.
|
|
||||||
|
|
||||||
Although it's probably less important for working in AI on a day-to-day basis, it's also useful to understand the broader context of AI. It goes beyond the narrow scope of deep learning on neural networks that have been so important to the gains made in reinforcement learning and supervised learning to date. For example, AI is often viewed as a way to augment (rather than replace) human judgment and decisions. But the handoff between machine and human has its own pitfalls.
|
|
||||||
|
|
||||||
With that background, here are some study areas and resources you may find useful.
|
|
||||||
|
|
||||||
### Research AI
|
|
||||||
|
|
||||||
In a lot of respects, a list of resources for research AI mirror those in an undergraduate (or even graduate) computer science program that's focused on AI. The main difference is that the syllabus you draw up may be more interdisciplinary than more traditionally focused university curricula.
|
|
||||||
|
|
||||||
Where you start will depend on your computer science and math background.
|
|
||||||
|
|
||||||
If it's minimal or rusty, but you still want to develop a deep understanding of AI fundamentals, you'll benefit from taking some math courses to start. There are many options on massive online open courses (MOOCs) like the nonprofit [edX][2] platform and [Coursera][3]. (Both platforms charge for certifications, but edX makes all the content available for free to people just auditing the course.)
|
|
||||||
|
|
||||||
Typical foundational courses could include:
|
|
||||||
|
|
||||||
+ [MIT's Calculus courses][22], starting with differentiation
|
|
||||||
+ [Linear Algebra][23] (University of Texas)
|
|
||||||
+ Probability and statistics, such as MIT's [Probability—The Science of Uncertainty and Data][24]
|
|
||||||
|
|
||||||
|
|
||||||
To get deeper into AI from a research perspective, you'll probably want to get into all these areas of mathematics and more. But the above should give you an idea of the general branches of study that are probably most important before delving into machine learning and AI proper.
|
|
||||||
|
|
||||||
In addition to MOOCs, resources such as [MIT OpenCourseWare][4] provide the syllabus and various supporting materials for a wide range of mathematics and computer science courses.
|
|
||||||
|
|
||||||
With the foundations in place, you can move onto more specialized courses in AI proper. Andrew Ng's AI MOOC, from when he was teaching at Stanford, was one of the early courses to popularize the whole online course space. Today, his [Neural Networks and Deep Learning][5] is part of the Deep Learning specialization at Coursera. There are corresponding programs on edX. For example, Columbia offers an [Artificial Intelligence MicroMasters][6].
|
|
||||||
|
|
||||||
In addition to courses, a variety of textbooks and other learning material are also available online. These include:
|
|
||||||
|
|
||||||
* [Neural Networks and Deep Learning][7]
|
|
||||||
* [Deep Learning][8] from MIT Press by Ian Goodfellow and Yoshua Bengio and Aaron Courville
|
|
||||||
|
|
||||||
### Applied AI
|
|
||||||
|
|
||||||
Applied AI is much more focused on using available tools than building new ones. Some appreciation of the mathematical underpinnings, especially statistics, is still useful—arguably even necessary—but you won't be majoring in that aspect of AI to the same degree you would in a research mode.
|
|
||||||
|
|
||||||
Programming is a core skill here. While different programming languages can come into play, a lot of libraries and toolsets—such as [PyTorch][9]—rely on Python, so that's a good skill to have. Especially if you have some level of programming background, MIT's [Introduction to Computer Science and Programming Using Python][10], based on its on-campus 6.001 course, is a good primer. If you're truly new to programming, Charles Severance's [Programming for Everybody (Getting Started with Python)][11] from the University of Michigan doesn't toss you into the deep end of the pool the way the MIT course does.
|
|
||||||
|
|
||||||
[The R programming language][12] is also a useful skill to add to your toolbox. While it's less used in machine learning (ML) per se, it's common for a variety of other data science tasks, and applied AI/ML and data science often blend in practice. For example, many tasks associated with organizing and cleaning data apply equally whatever analysis techniques you'll eventually use. A MOOC sequence like Harvard's [Data Science certificate][13] is an example of a set of courses that provide a good introduction to working with data.
|
|
||||||
|
|
||||||
Another open source software library you're likely to encounter if you do any work with AI is [TensorFlow][14]. It was originally developed by researchers and engineers from the Google Brain team within Google's AI organization. [Google offers a variety of tutorials][15] to get started with TensorFlow using the high-level Keras API. You can run TensorFlow locally as well as online in Google Cloud.
|
|
||||||
|
|
||||||
In general, all of the big public cloud providers offer online datasets and ML services that can be an easy way to get started. However, especially as you move beyond "play" datasets and applications, you need to start thinking seriously about the degree to which you want to be locked into a single provider.
|
|
||||||
|
|
||||||
Datasets for your exploratory learning projects are available from many different sources. In addition to the public cloud providers, [Kaggle][16] is another popular source and also a good learning resource more broadly. Government data is also increasingly available in digital form. The US Federal Government's [Data.gov][17] claims over 300,000 datasets. State and local governments also publish data on everything from restaurant health ratings to dogs' names.
|
|
||||||
|
|
||||||
### Miscellany
|
|
||||||
|
|
||||||
I'll close by noting that AI is a broad topic that isn't just about math, programming, and data. AI as a whole touches many other fields, including cognitive psychology, linguistics, game theory, operations research, and control systems. Indeed, a concern among at least some AI researchers today is that the field has become too fixated on a small number of techniques that have become powerful and interesting only quite recently because of the intersection of processing power and big data. Many longstanding problems in understanding how humans learn and reason remain largely unsolved. Developing at least some appreciation for these broader problem spaces will better enable you to place AI within a broader context.
|
|
||||||
|
|
||||||
One of my favorite examples is the [Humans and Autonomy Lab][18] at Duke. The work in this lab touches on all the challenges of humans working with machines, such as how autopilots can create ["Children of the Magenta"][19] who are unable to take control quickly if the automation fails. A basic brain-science course, such as MIT's [Introduction to Psychology][20], provides some useful context for the relationship between human intelligence and machine intelligence. Another course in a similar vein, but taught by the late Marvin Minsky from MIT's Electrical Engineering and Computer Science department, is [The Society of Mind][21].
|
|
||||||
|
|
||||||
If there's one key challenge to learning about AI, it's not that raw materials and tools aren't readily available. It's that there are so many of them. My objective hasn't been to give you a comprehensive set of pointers. Rather, it's been to both point out the different paths you can take and provide you with some possible starting points. Happy learning!
|
|
||||||
|
|
||||||
--------------------------------------------------------------------------------
|
|
||||||
|
|
||||||
via: https://opensource.com/article/18/12/how-get-started-ai
|
|
||||||
|
|
||||||
作者:[Gordon Haff][a]
|
|
||||||
选题:[lujun9972][b]
|
|
||||||
译者:[译者ID](https://github.com/译者ID)
|
|
||||||
校对:[校对者ID](https://github.com/校对者ID)
|
|
||||||
|
|
||||||
本文由 [LCTT](https://github.com/LCTT/TranslateProject) 原创编译,[Linux中国](https://linux.cn/) 荣誉推出
|
|
||||||
|
|
||||||
[a]: https://opensource.com/users/ghaff
|
|
||||||
[b]: https://github.com/lujun9972
|
|
||||||
[1]: https://www.youtube.com/watch?v=RLtI7r3QUyY
|
|
||||||
[2]: https://www.edx.org/
|
|
||||||
[3]: https://www.coursera.org/
|
|
||||||
[4]: https://ocw.mit.edu/index.htm
|
|
||||||
[5]: https://www.coursera.org/learn/neural-networks-deep-learning
|
|
||||||
[6]: https://www.edx.org/micromasters/columbiax-artificial-intelligence
|
|
||||||
[7]: http://neuralnetworksanddeeplearning.com/
|
|
||||||
[8]: http://www.deeplearningbook.org/
|
|
||||||
[9]: https://pytorch.org/
|
|
||||||
[10]: https://www.edx.org/course/introduction-to-computer-science-and-programming-using-python
|
|
||||||
[11]: https://www.coursera.org/learn/python
|
|
||||||
[12]: https://www.r-project.org/about.html
|
|
||||||
[13]: https://www.edx.org/professional-certificate/harvardx-data-science
|
|
||||||
[14]: https://www.tensorflow.org/
|
|
||||||
[15]: https://www.tensorflow.org/tutorials/
|
|
||||||
[16]: https://www.kaggle.com/
|
|
||||||
[17]: https://www.data.gov/
|
|
||||||
[18]: https://hal.pratt.duke.edu/
|
|
||||||
[19]: https://99percentinvisible.org/episode/children-of-the-magenta-automation-paradox-pt-1/
|
|
||||||
[20]: https://ocw.mit.edu/courses/brain-and-cognitive-sciences/9-00sc-introduction-to-psychology-fall-2011/
|
|
||||||
[21]: https://ocw.mit.edu/courses/electrical-engineering-and-computer-science/6-868j-the-society-of-mind-fall-2011/
|
|
||||||
[22]: https://www.edx.org/course/calculus-1a-differentiation
|
|
||||||
[23]: https://www.edx.org/course/linear-algebra-foundations-to-frontiers
|
|
||||||
[24]: https://courses.edx.org/courses/course-v1:MITx+6.431x+3T2018/course/
|
|
113
translated/tech/20181210 How to get started in AI.md
Normal file
113
translated/tech/20181210 How to get started in AI.md
Normal file
@ -0,0 +1,113 @@
|
|||||||
|
[#]: collector: (lujun9972)
|
||||||
|
[#]: translator: (qhwdw)
|
||||||
|
[#]: reviewer: ()
|
||||||
|
[#]: publisher: ()
|
||||||
|
[#]: url: ()
|
||||||
|
[#]: subject: (How to get started in AI)
|
||||||
|
[#]: via: (https://opensource.com/article/18/12/how-get-started-ai)
|
||||||
|
[#]: author: (Gordon Haff https://opensource.com/users/ghaff)
|
||||||
|
|
||||||
|
学习人工智能如何起步
|
||||||
|
======
|
||||||
|
在你开始从事人工智能之前,你需要先了解人类的智能。
|
||||||
|
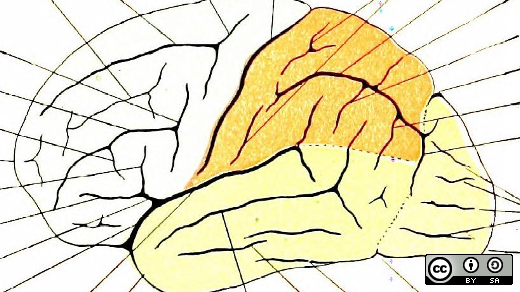
|
||||||
|
|
||||||
|
我曾经问过别人也被别人问过关于学习人工智能最好的方式是什么?我应该去阅读什么?我应该去关注什么?后面我将讲到这些,但是,考虑到人工智能涉及很多领域,我把这个问题分开来讲可能更好理解。
|
||||||
|
|
||||||
|
学习人工智能很重要的一点是区别开研究方面和应用方面。Google 的 Cassie Kozyrkov 在近日于伦敦举行的 O’Reilly 人工智能会议的一个演讲中 [描述了这个区别][1],并且这是一个很好的区别。
|
||||||
|
|
||||||
|
研究人工智能在本质上是学术性的,在你能够获得人工智能的某些细节之前,需要大量的跨各类学科的数学知识。这部分人工智能关注于算法和驱动人工智能发展的工具。比如,什么样的神经网络结构能够改善视觉识别的结果?我们如何使无监督学习成为更有用的方法?我们能否找到一个更好的方法,去理解深度学习流水线是如何得出答案的?
|
||||||
|
|
||||||
|
另一方面,人工智能应用更多是关于使用现有工具去获取有用的结果。开源在这里发挥了一个重要的作用,那就是免费提供了易于使用的、各种语言的软件。公有云提供商也致力于提供大量的机器学习、模型、以及数据集,这使得人工智能入门比其它的要简单的多。
|
||||||
|
|
||||||
|
在这个问题上我想补充一点,那就是人工智能的从业者不应该为了故弄玄虚而将它们的工具搞成只输出答案的黑匣子。至少,他们应该去了解不同技术、模型、和数据采集方法的限制和潜在偏差。但不需要去深入研究他们工具链中每个部分的理论。
|
||||||
|
|
||||||
|
虽然在日常工作中人工智能可能并不那么重要,但理解人工智能的大量的背景知识还是很有用的。人工智能已经超越了神经网络上深度学习的狭窄范围,目前神经网络上的强化学习和监督学习已经取得重要成就。例如,人工智能经常被视为是增强(而不是替代)人类判断和决策的一种方法。但是在机器和人类之间切换还有它自己的缺陷。
|
||||||
|
|
||||||
|
有了这些背景知识,下面是的一些研究领域和资源,你可能发现会很有用。
|
||||||
|
|
||||||
|
### 研究人工智能
|
||||||
|
|
||||||
|
在很多方面,用于人工智能研究的一个资源清单,可以反映出本科(甚至是研究生)的计算机科学项目都是专注于人工智能。最主要的区别是,你起草的教学大纲比起传统的大纲更关注于跨学科。
|
||||||
|
|
||||||
|
你的计算机科学和数学背景知识决定了你的起点。
|
||||||
|
|
||||||
|
如果你的计算机科学和数据背景知识很差或已经荒芜了,但你还希望能够深入了解人工智能的基本原理,那么从一些数学课程开始将会让你受益。MOOCs 上像非盈利的 [edX][2] 平台和 [Coursera][3] 上都有许多可供你选择的课程(这两个平台都对认证收费,但 edX 上所有的课程,对旁听者是全免费的)。
|
||||||
|
|
||||||
|
典型的基础课程包括:
|
||||||
|
|
||||||
|
+ [MIT 的微积分课程][22],从微分开始学习
|
||||||
|
+ [线性代数][23] (德克萨斯大学)
|
||||||
|
+ 概率与统计,比如 MIT 的 [概率 — 不确定性与数据科学][24]
|
||||||
|
|
||||||
|
|
||||||
|
从一个研究的角度去深入人工智能,你可能需要深入所有的这些数据领域,甚至更多。在深入研究机器学习和人工智能之前,上述的内容应该会让你得到一些常见研究分支的大致概念。
|
||||||
|
|
||||||
|
除了 MOOCs 之外,像 [MIT OpenCourseWare][4] 这样的资源也提供了大量的数学和计算机科学课程的大纲和各种支持材料。
|
||||||
|
|
||||||
|
有了这些基础,你就可以学习更专业的人工智能课程了。Andrew Ng 在斯坦福大学教的 “AI MOOC” 就是整个在线课程中最早流行起来的课程之一。今天,他的 [神经网络和深度学习][5] 也是 Coursera 深度学习专业的一部分。在 edX 上也有相关的一些项目,比如,哥伦比亚大学提供的一个 [人工智能 MicroMasters][6]。
|
||||||
|
|
||||||
|
除了课程之外,也可以在网上找到各种范例和其它学习材料。这些包括:
|
||||||
|
|
||||||
|
* [神经网络和深度学习][7]
|
||||||
|
* MIT 的 Ian Goodfellow、Yoshua Bengio、Aaron Courville 出版的 [深度学习][8]
|
||||||
|
|
||||||
|
### 应用人工智能
|
||||||
|
|
||||||
|
人工智能应用更关注于使用可用的工具,而不是去构建新工具。对一些底层的数学,尤其是统计学的了解仍然是非常有用的 — 甚至可以说是必需的 — 但对这些知识的了解程度不像研究人工智能的要求那么高。
|
||||||
|
|
||||||
|
在这里编程是核心技能。虽然可以使用不同的编程语言去做,但是一些库和工具集 — 比如 Python 的 [PyTorch][9],在这方面有很好的专长。尤其是,如果你有一些编程方面的背景知识,MIT 的 [计算机科学入门和使用 Python 编程][10],它是基于 MIT 的 6.001 课程,是一个非常好的启蒙课程。如果你编程零基础,来自密歇根大学的 Charles Severance 的 [人人学编程(Python 使用入门)][11] 是个很好的开端,它不会像 MIT 的课程那样,把你一下子扔进代码的汪洋大海。
|
||||||
|
|
||||||
|
[R 编程语言][12] 也是一个应该增加到你的技能库中的很有用的技能。虽然它在机器学习(ML)中使用的很少,但它在其它数据科学任务中很常见,并且经常与人工智能/机器学习和数据科学的应用实践结合在一起。比如,许多使用原始和数据清洗相关的应用任务,此外还有你最终要使用的诸如此类的分析技术,都将使用到它。一个 MOOC 系列,像 Harvard 的 [数据科学认证][13] 就是一整套课程的一个例子,这些课程介绍了如何去很好地处理数据。
|
||||||
|
|
||||||
|
如果你从事人工智能方面的工作,那么你很可能会遇到的另一个开源软件库就是 [TensorFlow][14]。它最初是由 Google 人工智能团队中的 Google 智慧团队的研发工程师开发的。[Google 提供了许多教程][15] 让你通过高级 Keras API 去开始使用 TensorFlow。你既可以在 Google 云上也可以在本地运行 TensorFlow。
|
||||||
|
|
||||||
|
通常,大的公有云提供商都提供在线数据集和易于使用的机器学习服务。但是,在你开始去 “玩” 数据集和应用之前,你需要考虑清楚,一旦开始选定一个提供商,你将被它们 “锁定” 的程度。
|
||||||
|
|
||||||
|
你的探索学习项目所需的数据集可以从许多不同的源获得。除了公有云提供商之外,[Kaggle][16] 是另一个受欢迎的源,总体来看,它也是一个比较好的学习源。以数字形式提供的政府数据也越来越多了。美国联邦政府的 [Data.gov][17] 声称它提供超过 300,000 的数据集。州和地方政府也发布从餐馆健康评级到狗的名字的所有数据。
|
||||||
|
|
||||||
|
### 研究和应用人工智能兼而有之
|
||||||
|
|
||||||
|
最后我想说明的一点是,人工智能不仅是与数学、编程、和数据有关的一个宽泛的主题。人工智能作为一个综合体涉及到了许多其它的领域,包括心理学、语言学、博弈论、运筹学和控制系统。确实,现在有一些人工智能研究者担心,由于处理能力和大数据的结合,使得该领域过于关注最近才变得强大和有趣的少数几个技术。在了解人类如何学习和推理方面,许多长期存在的问题仍未解决。不管怎样,对这些广泛存在的问题有一个了解,将更好地让你在更广泛的背景中评估人工智能。
|
||||||
|
|
||||||
|
我比较喜欢的其中一个示例是杜克大学的 [人类和自治实验室][18]。这个实验室的工作涉及人类与机器工作所面临的全部挑战,比如,如果自动化设备失效,自动驾驶仪如何设计才能让那些[“洋红色的孩子“][19] 快速取得对飞机的控制。一个基础的大脑科学课程,比如 MIT 的 [心理学导论][20],它提供了关于人类智能和机器智能之间关系的一些很有用的内容。另一个类似的课程是,MIT 电子工程与计算机科学系已故教授 Marvin Minsky 的 [心灵的社会][21]。
|
||||||
|
|
||||||
|
关于学习人工智能,假如说有一个最重要的挑战,那它不是原材料和工具不易获得,而是它们只有这么多。我的目标并不是给你一个全面的指导,相反,而是指出了你可以去学习的不同路径,以及为你提供一些可能的起点。祝你学习愉快!
|
||||||
|
|
||||||
|
--------------------------------------------------------------------------------
|
||||||
|
|
||||||
|
via: https://opensource.com/article/18/12/how-get-started-ai
|
||||||
|
|
||||||
|
作者:[Gordon Haff][a]
|
||||||
|
选题:[lujun9972][b]
|
||||||
|
译者:[qhwdw](https://github.com/qhwdw)
|
||||||
|
校对:[校对者ID](https://github.com/校对者ID)
|
||||||
|
|
||||||
|
本文由 [LCTT](https://github.com/LCTT/TranslateProject) 原创编译,[Linux中国](https://linux.cn/) 荣誉推出
|
||||||
|
|
||||||
|
[a]: https://opensource.com/users/ghaff
|
||||||
|
[b]: https://github.com/lujun9972
|
||||||
|
[1]: https://www.youtube.com/watch?v=RLtI7r3QUyY
|
||||||
|
[2]: https://www.edx.org/
|
||||||
|
[3]: https://www.coursera.org/
|
||||||
|
[4]: https://ocw.mit.edu/index.htm
|
||||||
|
[5]: https://www.coursera.org/learn/neural-networks-deep-learning
|
||||||
|
[6]: https://www.edx.org/micromasters/columbiax-artificial-intelligence
|
||||||
|
[7]: http://neuralnetworksanddeeplearning.com/
|
||||||
|
[8]: http://www.deeplearningbook.org/
|
||||||
|
[9]: https://pytorch.org/
|
||||||
|
[10]: https://www.edx.org/course/introduction-to-computer-science-and-programming-using-python
|
||||||
|
[11]: https://www.coursera.org/learn/python
|
||||||
|
[12]: https://www.r-project.org/about.html
|
||||||
|
[13]: https://www.edx.org/professional-certificate/harvardx-data-science
|
||||||
|
[14]: https://www.tensorflow.org/
|
||||||
|
[15]: https://www.tensorflow.org/tutorials/
|
||||||
|
[16]: https://www.kaggle.com/
|
||||||
|
[17]: https://www.data.gov/
|
||||||
|
[18]: https://hal.pratt.duke.edu/
|
||||||
|
[19]: https://99percentinvisible.org/episode/children-of-the-magenta-automation-paradox-pt-1/
|
||||||
|
[20]: https://ocw.mit.edu/courses/brain-and-cognitive-sciences/9-00sc-introduction-to-psychology-fall-2011/
|
||||||
|
[21]: https://ocw.mit.edu/courses/electrical-engineering-and-computer-science/6-868j-the-society-of-mind-fall-2011/
|
||||||
|
[22]: https://www.edx.org/course/calculus-1a-differentiation
|
||||||
|
[23]: https://www.edx.org/course/linear-algebra-foundations-to-frontiers
|
||||||
|
[24]: https://courses.edx.org/courses/course-v1:MITx+6.431x+3T2018/course/
|
Loading…
Reference in New Issue
Block a user