mirror of
https://github.com/LCTT/TranslateProject.git
synced 2025-02-03 23:40:14 +08:00
Merge remote-tracking branch 'LCTT/master'
This commit is contained in:
commit
37dc32e7e7
@ -1,29 +1,30 @@
|
||||
[#]: collector: (lujun9972)
|
||||
[#]: translator: (geekpi)
|
||||
[#]: reviewer: ( )
|
||||
[#]: publisher: ( )
|
||||
[#]: url: ( )
|
||||
[#]: reviewer: (wxy)
|
||||
[#]: publisher: (wxy)
|
||||
[#]: url: (https://linux.cn/article-11879-1.html)
|
||||
[#]: subject: (Use this open source tool to get your local weather forecast)
|
||||
[#]: via: (https://opensource.com/article/20/1/open-source-weather-forecast)
|
||||
[#]: author: (Kevin Sonney https://opensource.com/users/ksonney)
|
||||
|
||||
使用这个开源工具获取本地天气预报
|
||||
======
|
||||
在我们的 20 个使用开源提升生产力的系列的第十三篇文章中使用 wego 来了解出门前你是否要需要外套、雨伞或者防晒霜。
|
||||
![Sky with clouds and grass][1]
|
||||
|
||||
> 在我们的 20 个使用开源提升生产力的系列的第十三篇文章中使用 wego 来了解出门前你是否要需要外套、雨伞或者防晒霜。
|
||||
|
||||
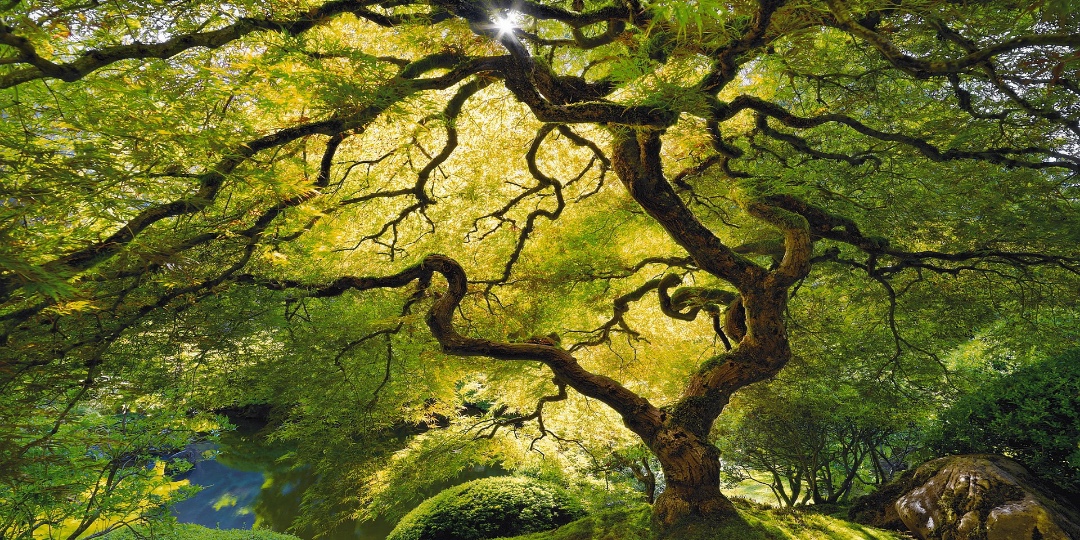
|
||||
|
||||
去年,我在 19 天里给你介绍了 19 个新(对你而言)的生产力工具。今年,我换了一种方式:使用你在使用或者还没使用的工具,构建一个使你可以在新一年更加高效的环境。
|
||||
|
||||
### 使用 wego 了解天气
|
||||
|
||||
过去十年我对我的职业最满意的地方之一是大多数时候是远程工作。尽管现实情况是我很多时候是在家里办公,但我可以在世界上任何地方工作。缺点是,离家时我会根据天气做出一些决定。在我居住的地方,”晴朗“可以表示从”酷热“、”低于零度“到”一小时内会小雨“。能够了解实际情况和快速预测非常有用。
|
||||
过去十年我对我的职业最满意的地方之一是大多数时候是远程工作。尽管现实情况是我很多时候是在家里办公,但我可以在世界上任何地方工作。缺点是,离家时我会根据天气做出一些决定。在我居住的地方,“晴朗”可以表示从“酷热”、“低于零度”到“一小时内会小雨”。能够了解实际情况和快速预测非常有用。
|
||||
|
||||
![Wego][2]
|
||||
|
||||
[Wego][3] 是用 Go 编写的程序,可以获取并显示你的当地天气。如果你愿意,它甚至可以用闪亮的 ASCII 艺术效果进行渲染。
|
||||
|
||||
要安装 wego,你需要确保在系统上安装了[Go][4]。之后,你可以使用 **go get** 命令获取最新版本。你可能还想将 **~/go/bin** 目录添加到路径中:
|
||||
|
||||
要安装 `wego`,你需要确保在系统上安装了[Go][4]。之后,你可以使用 `go get` 命令获取最新版本。你可能还想将 `~/go/bin` 目录添加到路径中:
|
||||
|
||||
```
|
||||
go get -u github.com/schachmat/wego
|
||||
@ -31,11 +32,9 @@ export PATH=~/go/bin:$PATH
|
||||
wego
|
||||
```
|
||||
|
||||
首次运行时,wego 会报告缺失 API 密钥。现在你需要决定一个后端。默认后端是 [Forecast.io][5],它是 [Dark Sky][6]的一部分。Wego还支持 [OpenWeatherMap][7] 和 [WorldWeatherOnline][8]。我更喜欢 OpenWeatherMap,因此我将在此向你展示如何设置。
|
||||
|
||||
你需要在 OpenWeatherMap 中[注册 API 密钥][9]。注册是免费的,尽管免费的 API 密钥限制了一天可以查询的数量,但这对于普通用户来说应该没问题。得到 API 密钥后,将它放到 **~/.wegorc** 文件中。现在可以填写你的位置、语言以及使用公制、英制(英国/美国)还是国际单位制(SI)。OpenWeatherMap 可通过名称、邮政编码、坐标和 ID 确定位置,这是我喜欢它的原因之一。
|
||||
|
||||
首次运行时,`wego` 会报告缺失 API 密钥。现在你需要决定一个后端。默认后端是 [Forecast.io][5],它是 [Dark Sky][6]的一部分。`wego` 还支持 [OpenWeatherMap][7] 和 [WorldWeatherOnline][8]。我更喜欢 OpenWeatherMap,因此我将在此向你展示如何设置。
|
||||
|
||||
你需要在 OpenWeatherMap 中[注册 API 密钥][9]。注册是免费的,尽管免费的 API 密钥限制了一天可以查询的数量,但这对于普通用户来说应该没问题。得到 API 密钥后,将它放到 `~/.wegorc` 文件中。现在可以填写你的位置、语言以及使用公制、英制(英国/美国)还是国际单位制(SI)。OpenWeatherMap 可通过名称、邮政编码、坐标和 ID 确定位置,这是我喜欢它的原因之一。
|
||||
|
||||
```
|
||||
# wego configuration for OEM
|
||||
@ -53,16 +52,15 @@ owm-lang=en
|
||||
units=imperial
|
||||
```
|
||||
|
||||
现在,在命令行运行 **wego** 将显示接下来三天的当地天气。
|
||||
现在,在命令行运行 `wego` 将显示接下来三天的当地天气。
|
||||
|
||||
Wego 还可以输出 JSON 以便程序使用,还可显示 emoji。你可以使用 **-f** 参数或在 **.wegorc** 文件中指定前端。
|
||||
`wego` 还可以输出 JSON 以便程序使用,还可显示 emoji。你可以使用 `-f` 参数或在 `.wegorc` 文件中指定前端。
|
||||
|
||||
![Wego at login][10]
|
||||
|
||||
如果你想在每次打开 shell 或登录主机时查看天气,只需将 wego 添加到 **~/.bashrc**(我这里是 **~/.zshrc**)即可。
|
||||
|
||||
[wttr.in][11] 项目是 wego 上的基于 Web 的封装。它提供了一些其他显示选项,并且可以在同名网站上看到。关于 wttr.in 的一件很酷的事情是,你可以使用 **curl** 获取一行天气信息。我有一个名为 **get_wttr** 的 shell 函数,用于获取当前简化的预报信息。
|
||||
如果你想在每次打开 shell 或登录主机时查看天气,只需将 wego 添加到 `~/.bashrc`(我这里是 `~/.zshrc`)即可。
|
||||
|
||||
[wttr.in][11] 项目是 wego 上的基于 Web 的封装。它提供了一些其他显示选项,并且可以在同名网站上看到。关于 wttr.in 的一件很酷的事情是,你可以使用 `curl` 获取一行天气信息。我有一个名为 `get_wttr` 的 shell 函数,用于获取当前简化的预报信息。
|
||||
|
||||
```
|
||||
get_wttr() {
|
||||
@ -81,7 +79,7 @@ via: https://opensource.com/article/20/1/open-source-weather-forecast
|
||||
作者:[Kevin Sonney][a]
|
||||
选题:[lujun9972][b]
|
||||
译者:[geekpi](https://github.com/geekpi)
|
||||
校对:[校对者ID](https://github.com/校对者ID)
|
||||
校对:[wxy](https://github.com/wxy)
|
||||
|
||||
本文由 [LCTT](https://github.com/LCTT/TranslateProject) 原创编译,[Linux中国](https://linux.cn/) 荣誉推出
|
||||
|
@ -1,5 +1,5 @@
|
||||
[#]: collector: (lujun9972)
|
||||
[#]: translator: ( )
|
||||
[#]: translator: (chenmu-kk )
|
||||
[#]: reviewer: ( )
|
||||
[#]: publisher: ( )
|
||||
[#]: url: ( )
|
||||
|
@ -1,113 +0,0 @@
|
||||
[#]: collector: (lujun9972)
|
||||
[#]: translator: (zhangxiangping)
|
||||
[#]: reviewer: ( )
|
||||
[#]: publisher: ( )
|
||||
[#]: url: ( )
|
||||
[#]: subject: (12 open source tools for natural language processing)
|
||||
[#]: via: (https://opensource.com/article/19/3/natural-language-processing-tools)
|
||||
[#]: author: (Dan Barker https://opensource.com/users/barkerd427)
|
||||
|
||||
12 open source tools for natural language processing
|
||||
======
|
||||
|
||||
Take a look at a dozen options for your next NLP application.
|
||||
|
||||
![Chat bubbles][1]
|
||||
|
||||
Natural language processing (NLP), the technology that powers all the chatbots, voice assistants, predictive text, and other speech/text applications that permeate our lives, has evolved significantly in the last few years. There are a wide variety of open source NLP tools out there, so I decided to survey the landscape to help you plan your next voice- or text-based application.
|
||||
|
||||
For this review, I focused on tools that use languages I'm familiar with, even though I'm not familiar with all the tools. (I didn't find a great selection of tools in the languages I'm not familiar with anyway.) That said, I excluded tools in three languages I am familiar with, for various reasons.
|
||||
|
||||
The most obvious language I didn't include might be R, but most of the libraries I found hadn't been updated in over a year. That doesn't always mean they aren't being maintained well, but I think they should be getting updates more often to compete with other tools in the same space. I also chose languages and tools that are most likely to be used in production scenarios (rather than academia and research), and I have mostly used R as a research and discovery tool.
|
||||
|
||||
I was also surprised to see that the Scala libraries are fairly stagnant. It has been a couple of years since I last used Scala, when it was pretty popular. Most of the libraries haven't been updated since that time—or they've only had a few updates.
|
||||
|
||||
Finally, I excluded C++. This is mostly because it's been many years since I last wrote in C++, and the organizations I've worked in have not used C++ for NLP or any data science work.
|
||||
|
||||
### Python tools
|
||||
|
||||
#### Natural Language Toolkit (NLTK)
|
||||
|
||||
It would be easy to argue that [Natural Language Toolkit (NLTK)][2] is the most full-featured tool of the ones I surveyed. It implements pretty much any component of NLP you would need, like classification, tokenization, stemming, tagging, parsing, and semantic reasoning. And there's often more than one implementation for each, so you can choose the exact algorithm or methodology you'd like to use. It also supports many languages. However, it represents all data in the form of strings, which is fine for simple constructs but makes it hard to use some advanced functionality. The documentation is also quite dense, but there is a lot of it, as well as [a great book][3]. The library is also a bit slow compared to other tools. Overall, this is a great toolkit for experimentation, exploration, and applications that need a particular combination of algorithms.
|
||||
|
||||
#### SpaCy
|
||||
|
||||
[SpaCy][4] is probably the main competitor to NLTK. It is faster in most cases, but it only has a single implementation for each NLP component. Also, it represents everything as an object rather than a string, which simplifies the interface for building applications. This also helps it integrate with many other frameworks and data science tools, so you can do more once you have a better understanding of your text data. However, SpaCy doesn't support as many languages as NLTK. It does have a simple interface with a simplified set of choices and great documentation, as well as multiple neural models for various components of language processing and analysis. Overall, this is a great tool for new applications that need to be performant in production and don't require a specific algorithm.
|
||||
|
||||
#### TextBlob
|
||||
|
||||
[TextBlob][5] is kind of an extension of NLTK. You can access many of NLTK's functions in a simplified manner through TextBlob, and TextBlob also includes functionality from the Pattern library. If you're just starting out, this might be a good tool to use while learning, and it can be used in production for applications that don't need to be overly performant. Overall, TextBlob is used all over the place and is great for smaller projects.
|
||||
|
||||
#### Textacy
|
||||
|
||||
This tool may have the best name of any library I've ever used. Say "[Textacy][6]" a few times while emphasizing the "ex" and drawing out the "cy." Not only is it great to say, but it's also a great tool. It uses SpaCy for its core NLP functionality, but it handles a lot of the work before and after the processing. If you were planning to use SpaCy, you might as well use Textacy so you can easily bring in many types of data without having to write extra helper code.
|
||||
|
||||
#### PyTorch-NLP
|
||||
|
||||
[PyTorch-NLP][7] has been out for just a little over a year, but it has already gained a tremendous community. It is a great tool for rapid prototyping. It's also updated often with the latest research, and top companies and researchers have released many other tools to do all sorts of amazing processing, like image transformations. Overall, PyTorch is targeted at researchers, but it can also be used for prototypes and initial production workloads with the most advanced algorithms available. The libraries being created on top of it might also be worth looking into.
|
||||
|
||||
### Node tools
|
||||
|
||||
#### Retext
|
||||
|
||||
[Retext][8] is part of the [unified collective][9]. Unified is an interface that allows multiple tools and plugins to integrate and work together effectively. Retext is one of three syntaxes used by the unified tool; the others are Remark for markdown and Rehype for HTML. This is a very interesting idea, and I'm excited to see this community grow. Retext doesn't expose a lot of its underlying techniques, but instead uses plugins to achieve the results you might be aiming for with NLP. It's easy to do things like checking spelling, fixing typography, detecting sentiment, or making sure text is readable with simple plugins. Overall, this is an excellent tool and community if you just need to get something done without having to understand everything in the underlying process.
|
||||
|
||||
#### Compromise
|
||||
|
||||
[Compromise][10] certainly isn't the most sophisticated tool. If you're looking for the most advanced algorithms or the most complete system, this probably isn't the right tool for you. However, if you want a performant tool that has a wide breadth of features and can function on the client side, you should take a look at Compromise. Overall, its name is accurate in that the creators compromised on functionality and accuracy by focusing on a small package with much more specific functionality that benefits from the user understanding more of the context surrounding the usage.
|
||||
|
||||
#### Natural
|
||||
|
||||
[Natural][11] includes most functions you might expect in a general NLP library. It is mostly focused on English, but some other languages have been contributed, and the community is open to additional contributions. It supports tokenizing, stemming, classification, phonetics, term frequency–inverse document frequency, WordNet, string similarity, and some inflections. It might be most comparable to NLTK, in that it tries to include everything in one package, but it is easier to use and isn't necessarily focused around research. Overall, this is a pretty full library, but it is still in active development and may require additional knowledge of underlying implementations to be fully effective.
|
||||
|
||||
#### Nlp.js
|
||||
|
||||
[Nlp.js][12] is built on top of several other NLP libraries, including Franc and Brain.js. It provides a nice interface into many components of NLP, like classification, sentiment analysis, stemming, named entity recognition, and natural language generation. It also supports quite a few languages, which is helpful if you plan to work in something other than English. Overall, this is a great general tool with a simplified interface into several other great tools. This will likely take you a long way in your applications before you need something more powerful or more flexible.
|
||||
|
||||
### Java tools
|
||||
|
||||
#### OpenNLP
|
||||
|
||||
[OpenNLP][13] is hosted by the Apache Foundation, so it's easy to integrate it into other Apache projects, like Apache Flink, Apache NiFi, and Apache Spark. It is a general NLP tool that covers all the common processing components of NLP, and it can be used from the command line or within an application as a library. It also has wide support for multiple languages. Overall, OpenNLP is a powerful tool with a lot of features and ready for production workloads if you're using Java.
|
||||
|
||||
#### StanfordNLP
|
||||
|
||||
[Stanford CoreNLP][14] is a set of tools that provides statistical NLP, deep learning NLP, and rule-based NLP functionality. Many other programming language bindings have been created so this tool can be used outside of Java. It is a very powerful tool created by an elite research institution, but it may not be the best thing for production workloads. This tool is dual-licensed with a special license for commercial purposes. Overall, this is a great tool for research and experimentation, but it may incur additional costs in a production system. The Python implementation might also interest many readers more than the Java version. Also, one of the best Machine Learning courses is taught by a Stanford professor on Coursera. [Check it out][15] along with other great resources.
|
||||
|
||||
#### CogCompNLP
|
||||
|
||||
[CogCompNLP][16], developed by the University of Illinois, also has a Python library with similar functionality. It can be used to process text, either locally or on remote systems, which can remove a tremendous burden from your local device. It provides processing functions such as tokenization, part-of-speech tagging, chunking, named-entity tagging, lemmatization, dependency and constituency parsing, and semantic role labeling. Overall, this is a great tool for research, and it has a lot of components that you can explore. I'm not sure it's great for production workloads, but it's worth trying if you plan to use Java.
|
||||
|
||||
* * *
|
||||
|
||||
What are your favorite open source tools and libraries for NLP? Please share in the comments—especially if there's one I didn't include.
|
||||
|
||||
--------------------------------------------------------------------------------
|
||||
|
||||
via: https://opensource.com/article/19/3/natural-language-processing-tools
|
||||
|
||||
作者:[Dan Barker (Community Moderator)][a]
|
||||
选题:[lujun9972][b]
|
||||
译者:[zhangxiangping](https://github.com/zhangxiangping)
|
||||
校对:[校对者ID](https://github.com/校对者ID)
|
||||
|
||||
本文由 [LCTT](https://github.com/LCTT/TranslateProject) 原创编译,[Linux中国](https://linux.cn/) 荣誉推出
|
||||
|
||||
[a]: https://opensource.com/users/barkerd427
|
||||
[b]: https://github.com/lujun9972
|
||||
[1]: https://opensource.com/sites/default/files/styles/image-full-size/public/lead-images/talk_chat_communication_team.png?itok=CYfZ_gE7 (Chat bubbles)
|
||||
[2]: http://www.nltk.org/
|
||||
[3]: http://www.nltk.org/book_1ed/
|
||||
[4]: https://spacy.io/
|
||||
[5]: https://textblob.readthedocs.io/en/dev/
|
||||
[6]: https://readthedocs.org/projects/textacy/
|
||||
[7]: https://pytorchnlp.readthedocs.io/en/latest/
|
||||
[8]: https://www.npmjs.com/package/retext
|
||||
[9]: https://unified.js.org/
|
||||
[10]: https://www.npmjs.com/package/compromise
|
||||
[11]: https://www.npmjs.com/package/natural
|
||||
[12]: https://www.npmjs.com/package/node-nlp
|
||||
[13]: https://opennlp.apache.org/
|
||||
[14]: https://stanfordnlp.github.io/CoreNLP/
|
||||
[15]: https://opensource.com/article/19/2/learn-data-science-ai
|
||||
[16]: https://github.com/CogComp/cogcomp-nlp
|
@ -1,5 +1,5 @@
|
||||
[#]: collector: (lujun9972)
|
||||
[#]: translator: ( )
|
||||
[#]: translator: (zhangxiangping)
|
||||
[#]: reviewer: ( )
|
||||
[#]: publisher: ( )
|
||||
[#]: url: ( )
|
||||
@ -264,7 +264,7 @@ via: https://opensource.com/article/19/7/python-google-natural-language-api
|
||||
|
||||
作者:[JR Oakes][a]
|
||||
选题:[lujun9972][b]
|
||||
译者:[译者ID](https://github.com/译者ID)
|
||||
译者:[zhangxiangping](https://github.com/zhangxiangping)
|
||||
校对:[校对者ID](https://github.com/校对者ID)
|
||||
|
||||
本文由 [LCTT](https://github.com/LCTT/TranslateProject) 原创编译,[Linux中国](https://linux.cn/) 荣誉推出
|
||||
|
@ -1,5 +1,5 @@
|
||||
[#]: collector: (lujun9972)
|
||||
[#]: translator: ( )
|
||||
[#]: translator: (HankChow)
|
||||
[#]: reviewer: ( )
|
||||
[#]: publisher: ( )
|
||||
[#]: url: ( )
|
||||
|
@ -0,0 +1,111 @@
|
||||
[#]: collector: (lujun9972)
|
||||
[#]: translator: (zhangxiangping)
|
||||
[#]: reviewer: ( )
|
||||
[#]: publisher: ( )
|
||||
[#]: url: ( )
|
||||
[#]: subject: (12 open source tools for natural language processing)
|
||||
[#]: via: (https://opensource.com/article/19/3/natural-language-processing-tools)
|
||||
[#]: author: (Dan Barker https://opensource.com/users/barkerd427)
|
||||
|
||||
12种自然语言处理的开源工具
|
||||
======
|
||||
|
||||
看看可以用在你自己NLP应用中的十几个工具吧。
|
||||
|
||||
![Chat bubbles][1]
|
||||
|
||||
在过去的几年里,自然语言处理(NLP)推动了聊天机器人、语音助手、文本预测,这些在我们的日常生活中常用的语音或文本应用程技术的发展。目前有着各种各样开源的NLP工具,所以我决定调查一下当前开源的NLP工具来帮助您制定您开发下一个基于语音或文本的应用程序的计划。
|
||||
|
||||
我将从我所熟悉的编程语言出发来介绍这些工具,尽管我对这些工具不是很熟悉(我没有在我不熟悉的语言中找工具)。也就是说,出于各种原因,我排除了三种我熟悉的语言中的工具。
|
||||
|
||||
R语言是没有被包含在内的,因为我发现的大多数库都有一年多没有更新了。这并不总是意味着他们没有得到很好的维护,但我认为他们应该得到更多的更新,以便和同一领域的其他工具竞争。我还选择了最有可能在生产场景中使用的语言和工具(而不是在学术界和研究中使用),虽然我主要是使用R作为研究和发现工具。
|
||||
|
||||
我发现Scala的很多库都没有更新了。我上次使用Scala已经有好几年了,当时它非常流行。但是大多数库从那个时候就再没有更新过,或者只有少数一些有更新。
|
||||
|
||||
最后,我排除了C++。这主要是因为我在的公司很久没有使用C++来进行NLP或者任何数据科学的工作。
|
||||
|
||||
### Python工具
|
||||
#### Natural Language Toolkit (NLTK)
|
||||
|
||||
[Natural Language Toolkit (NLTK)][2]是我调研的所有工具中功能最完善的一个。它完美地实现了自然语言处理中多数功能组件,比如分类,令牌化,词干化,标注,分词和语义推理。每一种方法都有多种不同的实现方式,所以你可以选择具体的算法和方式去使用它。同时,它也支持不同语言。然而,它将所有的数据都表示为字符串的形式,对于一些简单的数据结构来说可能很方便,但是如果要使用一些高级的功能来说就可能有点困难。它的使用文档有点复杂,但也有很多其他人编写的使用文档,比如[a great book][3]。和其他的工具比起来,这个工具库的运行速度有点慢。但总的来说,这个工具包非常不错,可以用于需要具体算法组合的实验,探索和实际应用当中。
|
||||
|
||||
#### SpaCy
|
||||
|
||||
[SpaCy][4]是NLTK的主要竞争者。在大多数情况下都比NLTK的速度更快,但是SpaCy对自然语言处理的功能组件只有单一实现。SpaCy把所有的东西都表示为一个对象而不是字符串,这样就能够为构建应用简化接口。这也方便它能够集成多种框架和数据科学的工具,使得你更容易理解你的文本数据。然而,SpaCy不像NLTK那样支持多种语言。它对每个接口都有一些简单的选项和文档,包括用于语言处理和分析各种组件的多种神经网络模型。总的来说,如果创造一个新的应用的生产过程中不需要使用特定的算法的话,这是一个很不错的工具。
|
||||
|
||||
#### TextBlob
|
||||
|
||||
[TextBlob][5]是NLTK的一个扩展库。你可以通过TextBlob用一种更简单的方式来使用NLTK的功能,TextBlob也包括了Pattern库中的功能。如果你刚刚开始学习,这将会是一个不错的工具可以用于生产对性能要求不太高的应用。TextBlob适用于任何场景,但是对小型项目会更加合适。
|
||||
|
||||
#### Textacy
|
||||
|
||||
这个工具是我用过的名字最好听的。读"[Textacy][6]" 时先发出"ex"再发出"cy"。它不仅仅是名字好,同时它本身也是一个很不错的工具。它使用SpaCy作为它自然语言处理核心功能,但它在处理过程的前后做了很多工作。如果你想要使用SpaCy,你可以先使用Textacy,从而不用去多写额外的附加代码你就可以处理不同种类的数据。
|
||||
|
||||
#### PyTorch-NLP
|
||||
|
||||
[PyTorch-NLP][7]才出现短短的一年,但它已经有一个庞大的社区了。它适用于快速原型开发。当公司或者研究人员推出很多其他工具去完成新奇的处理任务,比如图像转换,它就会被更新。PyTorch的目标用户是研究人员,但它也能用于原型开发,或在最开始的生产任务中使用最好的算法。基于此基础上的创建的库也是值得研究的。
|
||||
|
||||
### 节点工具
|
||||
|
||||
#### Retext
|
||||
|
||||
[Retext][8]是[unified collective][9]的一部分。Unified是一个接口,能够集成不同的工具和插件以便他们能够高效的工作。Retext是unified工具集三个中的一个,另外的两个分别是用于markdown编辑的Remark和用于HTML处理的Rehype。这是一个非常有趣的想法,我很高兴看到这个社区的发展。Retext没有暴露过多的底层技术,更多的是使用插件去完成你在NLP任务中想要做的事情。拼写检查,固定排版,情绪检测和可读性分析都可以用简单的插件来完成。如果你不想了解底层处理技术又想完成你的任务的话,这个工具和社区是一个不错的选择。
|
||||
|
||||
#### Compromise
|
||||
|
||||
如果你在找拥有最高级的功能和最复杂的系统的工具的话,[Compromise][10]不是你的选择。 然而,如果你想要一个性能好,应用广泛,还能在客户端运行的工具的话,Compromise值得一试。实际上,它的名字是准确的,因为作者更关注更具体功能的小软件包,而在功能性和准确性上做出了牺牲,这些功能得益于用户对使用环境的理解。
|
||||
|
||||
#### Natural
|
||||
|
||||
[Natural][11]包含了一般自然语言处理库所具有的大多数功能。它主要是处理英文文本,但也包括一些其他语言,它的社区也支持额外的语言。它能够进行令牌化,词干化,分类,语音处理,词频-逆文档频率计算(TF-IDF),WordNet,字符相似度计算和一些变换。它和NLTK有的一比,因为它想要把所有东西都包含在一个包里头,使用方便但是可能不太适合专注的研究。总的来说,这是一个不错的功能齐全的库,目前仍在开发但可能需要对底层实现有更多的了解才能完更有效。
|
||||
|
||||
#### Nlp.js
|
||||
|
||||
[Nlp.js][12]是在其他几个NLP库上开发的,包括Franc和Brain.js。它提供了一个能很好支持NLP组件的接口,比如分类,情感分析,词干化,命名实体识别和自然语言生成。它也支持一些其他语言,在你处理除了英语之外的语言时也能提供一些帮助。总之,它是一个不错的通用工具,能够提供简单的接口去调用其他工具。在你需要更强大或更灵活的工具之前,这个工具可能会在你的应用程序中用上很长一段时间。
|
||||
|
||||
### Java工具
|
||||
#### OpenNLP
|
||||
|
||||
[OpenNLP][13]是由Apache基金会维护的,所以它可以很方便地集成到其他Apache项目中,比如Apache Flink,Apache NiFi和Apache Spark。这是一个通用的NLP工具,包含了所有NLP组件中的通用功能,可以通过命令行或者以包的形式导入到应用中来使用它。它也支持很多种语言。OpenNLP是一个很高效的工具,包含了很多特性,如果你用Java开发生产的话,它是个很好的选择。
|
||||
|
||||
#### StanfordNLP
|
||||
|
||||
[Stanford CoreNLP][14]是一个工具集,提供了基于统计的,基于深度学习和基于规则的NLP功能。这个工具也有许多其他编程语言的版本,所以可以脱离Java来使用。它是由高水平的研究机构创建的一个高效的工具,但在生产环境中可能不是最好的。此工具具有双重许可,并具有可以用于商业目的的特殊许可。总之,在研究和实验中它是一个很棒的工具,但在生产系统中可能会带来一些额外的开销。比起Java版本来说,读者可能对它的Python版本更感兴趣。斯坦福教授在Coursera上教的最好的机器学习课程之一,[点此][15]访问其他不错的资源。
|
||||
|
||||
#### CogCompNLP
|
||||
|
||||
[CogCompNLP][16]由伊利诺斯大学开发的一个工具,它也有一个相似功能的Python版本事项。它可以用于处理文本,包括本地处理和远程处理,能够极大地缓解你本地设备的压力。它提供了很多处理函数,比如令牌化,词性分析,标注,断句,命名实体标注,词型还原,依存分析和语义角色标注。它是一个很好的研究工具,你可以自己探索它的不同功能。我不确定它是否适合生产环境,但如果你使用Java的话,它值得一试。
|
||||
|
||||
* * *
|
||||
|
||||
你最喜欢的开源的NLP工具和库是什么?请在评论区分享文中没有提到的工具。
|
||||
|
||||
--------------------------------------------------------------------------------
|
||||
|
||||
via: https://opensource.com/article/19/3/natural-language-processing-tools
|
||||
|
||||
作者:[Dan Barker (Community Moderator)][a]
|
||||
选题:[lujun9972][b]
|
||||
译者:[zxp](https://github.com/zhangxiangping)
|
||||
校对:[校对者ID](https://github.com/校对者ID)
|
||||
|
||||
本文由 [LCTT](https://github.com/LCTT/TranslateProject) 原创编译,[Linux中国](https://linux.cn/) 荣誉推出
|
||||
|
||||
[a]: https://opensource.com/users/barkerd427
|
||||
[b]: https://github.com/lujun9972
|
||||
[1]: https://opensource.com/sites/default/files/styles/image-full-size/public/lead-images/talk_chat_communication_team.png?itok=CYfZ_gE7 (Chat bubbles)
|
||||
[2]: http://www.nltk.org/
|
||||
[3]: http://www.nltk.org/book_1ed/
|
||||
[4]: https://spacy.io/
|
||||
[5]: https://textblob.readthedocs.io/en/dev/
|
||||
[6]: https://readthedocs.org/projects/textacy/
|
||||
[7]: https://pytorchnlp.readthedocs.io/en/latest/
|
||||
[8]: https://www.npmjs.com/package/retext
|
||||
[9]: https://unified.js.org/
|
||||
[10]: https://www.npmjs.com/package/compromise
|
||||
[11]: https://www.npmjs.com/package/natural
|
||||
[12]: https://www.npmjs.com/package/node-nlp
|
||||
[13]: https://opennlp.apache.org/
|
||||
[14]: https://stanfordnlp.github.io/CoreNLP/
|
||||
[15]: https://opensource.com/article/19/2/learn-data-science-ai
|
||||
[16]: https://github.com/CogComp/cogcomp-nlp
|
Loading…
Reference in New Issue
Block a user