mirror of
https://github.com/LCTT/TranslateProject.git
synced 2025-03-24 02:20:09 +08:00
Merge pull request #7097 from Flowsnow/master
翻译完成-20171002 High Dynamic Range Imaging using OpenCV Cpp python.md
This commit is contained in:
commit
33930ee79c
@ -1,423 +0,0 @@
|
||||
translating by flowsnow
|
||||
|
||||
High Dynamic Range (HDR) Imaging using OpenCV (C++/Python)
|
||||
============================================================
|
||||
|
||||
|
||||
|
||||
In this tutorial, we will learn how to create a High Dynamic Range (HDR) image using multiple images taken with different exposure settings. We will share code in both C++ and Python.
|
||||
|
||||
### What is High Dynamic Range (HDR) imaging?
|
||||
|
||||
Most digital cameras and displays capture or display color images as 24-bits matrices. There are 8-bits per color channel and the pixel values are therefore in the range 0 – 255 for each channel. In other words, a regular camera or a display has a limited dynamic range.
|
||||
|
||||
However, the world around us has a very large dynamic range. It can get pitch black inside a garage when the lights are turned off and it can get really bright if you are looking directly at the Sun. Even without considering those extremes, in everyday situations, 8-bits are barely enough to capture the scene. So, the camera tries to estimate the lighting and automatically sets the exposure so that the most interesting aspect of the image has good dynamic range, and the parts that are too dark and too bright are clipped off to 0 and 255 respectively.
|
||||
|
||||
In the Figure below, the image on the left is a normally exposed image. Notice the sky in the background is completely washed out because the camera decided to use a setting where the subject (my son) is properly photographed, but the bright sky is washed out. The image on the right is an HDR image produced by the iPhone.
|
||||
|
||||
[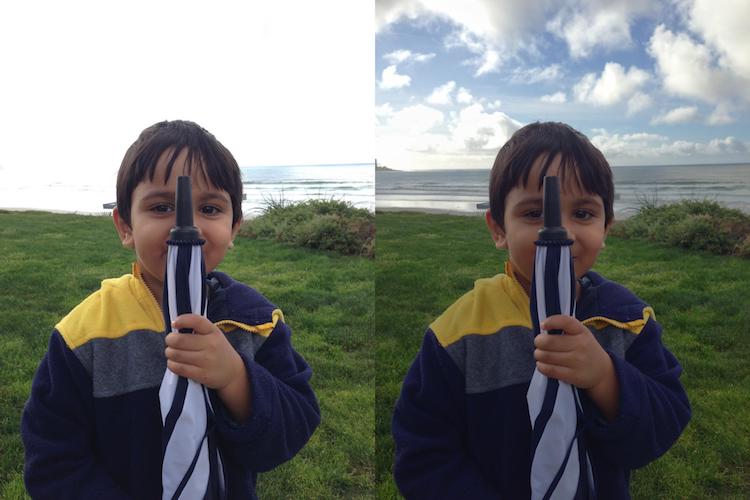][3]
|
||||
|
||||
How does an iPhone capture an HDR image? It actually takes 3 images at three different exposures. The images are taken in quick succession so there is almost no movement between the three shots. The three images are then combined to produce the HDR image. We will see the details in the next section.
|
||||
|
||||
The process of combining different images of the same scene acquired under different exposure settings is called High Dynamic Range (HDR) imaging.
|
||||
|
||||
### How does High Dynamic Range (HDR) imaging work?
|
||||
|
||||
In this section, we will go through the steps of creating an HDR image using OpenCV.
|
||||
|
||||
To easily follow this tutorial, please [download][4] the C++ and Python code and images by clicking [here][5]. If you are interested to learn more about AI, Computer Vision and Machine Learning, please [subscribe][6] to our newsletter.
|
||||
|
||||
### Step 1: Capture multiple images with different exposures
|
||||
|
||||
When we take a picture using a camera, we have only 8-bits per channel to represent the dynamic range ( brightness range ) of the scene. But we can take multiple images of the scene at different exposures by changing the shutter speed. Most SLR cameras have a feature called Auto Exposure Bracketing (AEB) that allows us to take multiple pictures at different exposures with just one press of a button. If you are using an iPhone, you can use this [AutoBracket HDR app][7] and if you are an android user you can try [A Better Camera app][8].
|
||||
|
||||
Using AEB on a camera or an auto bracketing app on the phone, we can take multiple pictures quickly one after the other so the scene does not change. When we use HDR mode in an iPhone, it takes three pictures.
|
||||
|
||||
1. An underexposed image: This image is darker than the properly exposed image. The goal is the capture parts of the image that very bright.
|
||||
|
||||
2. A properly exposed image: This is the regular image the camera would have taken based on the illumination it has estimated.
|
||||
|
||||
3. An overexposed image: This image is brighter than the properly exposed image. The goal is the capture parts of the image that very dark.
|
||||
|
||||
However, if the dynamic range of the scene is very large, we can take more than three pictures to compose the HDR image. In this tutorial, we will use 4 images taken with exposure time 1/30, 0.25, 2.5 and 15 seconds. The thumbnails are shown below.
|
||||
|
||||
[][9]
|
||||
|
||||
The information about the exposure time and other settings used by an SLR camera or a Phone are usually stored in the EXIF metadata of the JPEG file. Check out this [link][10] to see EXIF metadata stored in a JPEG file in Windows and Mac. Alternatively, you can use my favorite command line utility for EXIF called [EXIFTOOL ][11].
|
||||
|
||||
Let’s start by reading in the images are assigning the exposure times
|
||||
|
||||
C++
|
||||
|
||||
```
|
||||
void readImagesAndTimes(vector<Mat> &images, vector<float> ×)
|
||||
{
|
||||
|
||||
int numImages = 4;
|
||||
|
||||
// List of exposure times
|
||||
static const float timesArray[] = {1/30.0f,0.25,2.5,15.0};
|
||||
times.assign(timesArray, timesArray + numImages);
|
||||
|
||||
// List of image filenames
|
||||
static const char* filenames[] = {"img_0.033.jpg", "img_0.25.jpg", "img_2.5.jpg", "img_15.jpg"};
|
||||
for(int i=0; i < numImages; i++)
|
||||
{
|
||||
Mat im = imread(filenames[i]);
|
||||
images.push_back(im);
|
||||
}
|
||||
|
||||
}
|
||||
```
|
||||
|
||||
Python
|
||||
|
||||
```
|
||||
def readImagesAndTimes():
|
||||
# List of exposure times
|
||||
times = np.array([ 1/30.0, 0.25, 2.5, 15.0 ], dtype=np.float32)
|
||||
|
||||
# List of image filenames
|
||||
filenames = ["img_0.033.jpg", "img_0.25.jpg", "img_2.5.jpg", "img_15.jpg"]
|
||||
images = []
|
||||
for filename in filenames:
|
||||
im = cv2.imread(filename)
|
||||
images.append(im)
|
||||
|
||||
return images, times
|
||||
```
|
||||
|
||||
### Step 2: Align Images
|
||||
|
||||
Misalignment of images used in composing the HDR image can result in severe artifacts. In the Figure below, the image on the left is an HDR image composed using unaligned images and the image on the right is one using aligned images. By zooming into a part of the image, shown using red circles, we see severe ghosting artifacts in the left image.
|
||||
|
||||
[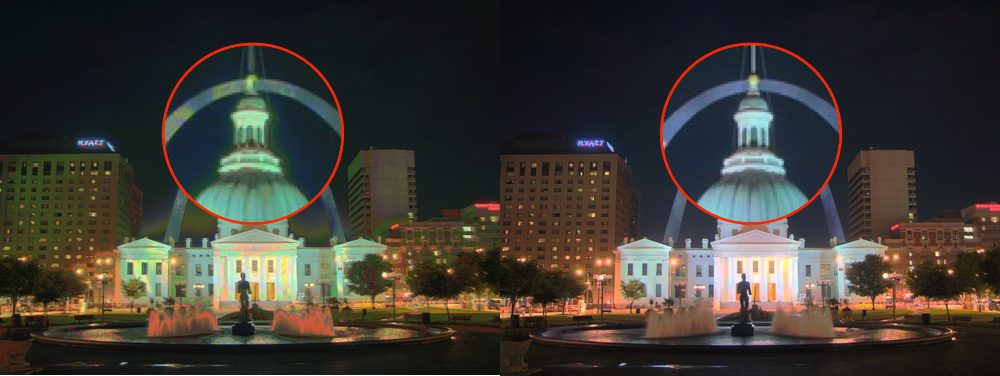][12]
|
||||
|
||||
Naturally, while taking the pictures for creating an HDR image, professional photographer mount the camera on a tripod. They also use a feature called [mirror lockup][13] to reduce additional vibrations. Even then, the images may not be perfectly aligned because there is no way to guarantee a vibration-free environment. The problem of alignment gets a lot worse when images are taken using a handheld camera or a phone.
|
||||
|
||||
Fortunately, OpenCV provides an easy way to align these images using `AlignMTB`. This algorithm converts all the images to median threshold bitmaps (MTB). An MTB for an image is calculated by assigning the value 1 to pixels brighter than median luminance and 0 otherwise. An MTB is invariant to the exposure time. Therefore, the MTBs can be aligned without requiring us to specify the exposure time.
|
||||
|
||||
MTB based alignment is performed using the following lines of code.
|
||||
|
||||
C++
|
||||
|
||||
```
|
||||
// Align input images
|
||||
Ptr<AlignMTB> alignMTB = createAlignMTB();
|
||||
alignMTB->process(images, images);
|
||||
```
|
||||
|
||||
Python
|
||||
|
||||
```
|
||||
# Align input images
|
||||
alignMTB = cv2.createAlignMTB()
|
||||
alignMTB.process(images, images)
|
||||
```
|
||||
|
||||
### Step 3: Recover the Camera Response Function
|
||||
|
||||
The response of a typical camera is not linear to scene brightness. What does that mean? Suppose, two objects are photographed by a camera and one of them is twice as bright as the other in the real world. When you measure the pixel intensities of the two objects in the photograph, the pixel values of the brighter object will not be twice that of the darker object! Without estimating the Camera Response Function (CRF), we will not be able to merge the images into one HDR image.
|
||||
|
||||
What does it mean to merge multiple exposure images into an HDR image?
|
||||
|
||||
Consider just ONE pixel at some location (x,y) of the images. If the CRF was linear, the pixel value would be directly proportional to the exposure time unless the pixel is too dark ( i.e. nearly 0 ) or too bright ( i.e. nearly 255) in a particular image. We can filter out these bad pixels ( too dark or too bright ), and estimate the brightness at a pixel by dividing the pixel value by the exposure time and then averaging this brightness value across all images where the pixel is not bad ( too dark or too bright ). We can do this for all pixels and obtain a single image where all pixels are obtained by averaging “good” pixels.
|
||||
|
||||
But the CRF is not linear and we need to make the image intensities linear before we can merge/average them by first estimating the CRF.
|
||||
|
||||
The good news is that the CRF can be estimated from the images if we know the exposure times for each image. Like many problems in computer vision, the problem of finding the CRF is set up as an optimization problem where the goal is to minimize an objective function consisting of a data term and a smoothness term. These problems usually reduce to a linear least squares problem which are solved using Singular Value Decomposition (SVD) that is part of all linear algebra packages. The details of the CRF recovery algorithm are in the paper titled [Recovering High Dynamic Range Radiance Maps from Photographs][14].
|
||||
|
||||
Finding the CRF is done using just two lines of code in OpenCV using `CalibrateDebevec` or `CalibrateRobertson`. In this tutorial we will use `CalibrateDebevec`
|
||||
|
||||
C++
|
||||
|
||||
```
|
||||
// Obtain Camera Response Function (CRF)
|
||||
Mat responseDebevec;
|
||||
Ptr<CalibrateDebevec> calibrateDebevec = createCalibrateDebevec();
|
||||
calibrateDebevec->process(images, responseDebevec, times);
|
||||
|
||||
```
|
||||
|
||||
Python
|
||||
|
||||
```
|
||||
# Obtain Camera Response Function (CRF)
|
||||
calibrateDebevec = cv2.createCalibrateDebevec()
|
||||
responseDebevec = calibrateDebevec.process(images, times)
|
||||
```
|
||||
|
||||
The figure below shows the CRF recovered using the images for the red, green and blue channels.
|
||||
|
||||
[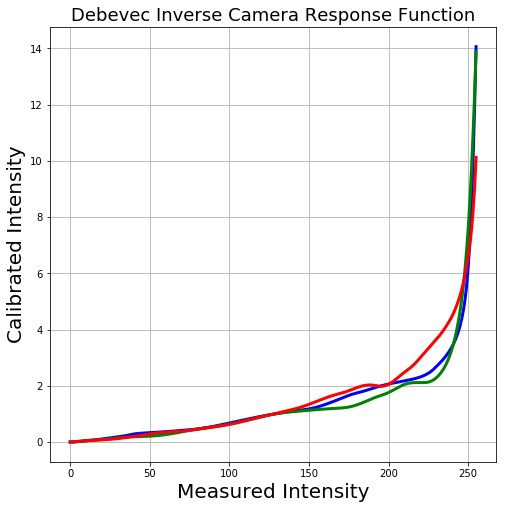][15]
|
||||
|
||||
### Step 4: Merge Images
|
||||
|
||||
Once the CRF has been estimated, we can merge the exposure images into one HDR image using `MergeDebevec`. The C++ and Python code is shown below.
|
||||
|
||||
C++
|
||||
|
||||
```
|
||||
// Merge images into an HDR linear image
|
||||
Mat hdrDebevec;
|
||||
Ptr<MergeDebevec> mergeDebevec = createMergeDebevec();
|
||||
mergeDebevec->process(images, hdrDebevec, times, responseDebevec);
|
||||
// Save HDR image.
|
||||
imwrite("hdrDebevec.hdr", hdrDebevec);
|
||||
```
|
||||
|
||||
Python
|
||||
|
||||
```
|
||||
# Merge images into an HDR linear image
|
||||
mergeDebevec = cv2.createMergeDebevec()
|
||||
hdrDebevec = mergeDebevec.process(images, times, responseDebevec)
|
||||
# Save HDR image.
|
||||
cv2.imwrite("hdrDebevec.hdr", hdrDebevec)
|
||||
```
|
||||
|
||||
The HDR image saved above can be loaded in Photoshop and tonemapped. An example is shown below.
|
||||
|
||||
[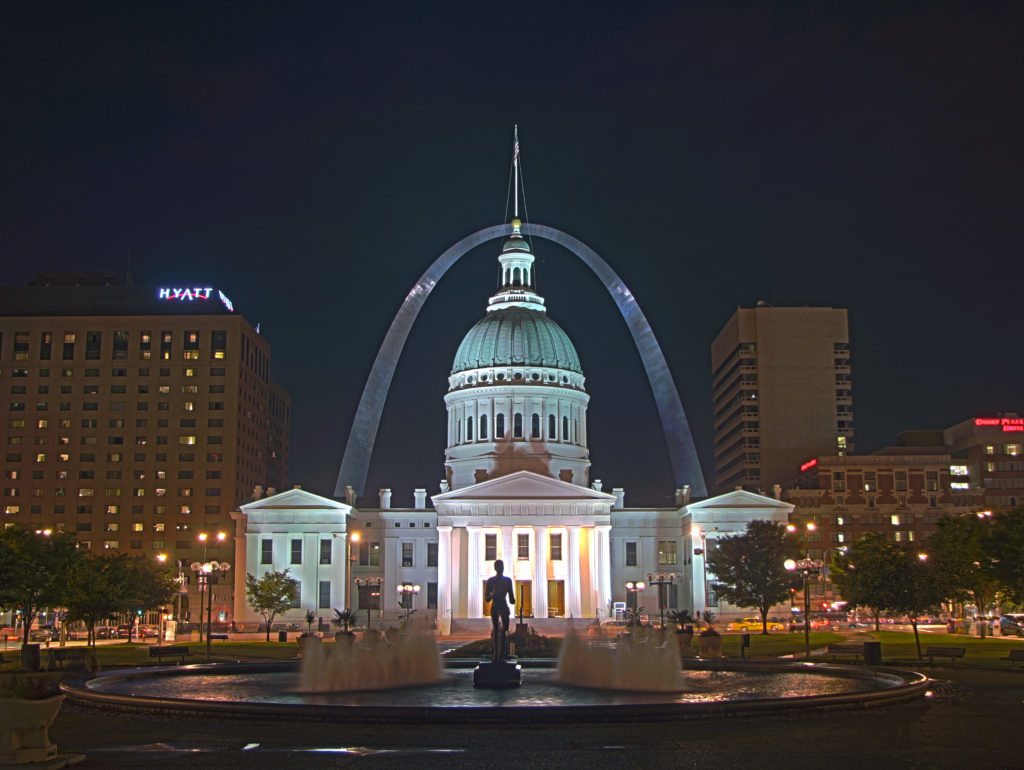][16] HDR Photoshop tone mapping
|
||||
|
||||
### Step 5: Tone mapping
|
||||
|
||||
Now we have merged our exposure images into one HDR image. Can you guess the minimum and maximum pixel values for this image? The minimum value is obviously 0 for a pitch black condition. What is the theoretical maximum value? Infinite! In practice, the maximum value is different for different situations. If the scene contains a very bright light source, we will see a very large maximum value.
|
||||
|
||||
Even though we have recovered the relative brightness information using multiple images, we now have the challenge of saving this information as a 24-bit image for display purposes.
|
||||
|
||||
The process of converting a High Dynamic Range (HDR) image to an 8-bit per channel image while preserving as much detail as possible is called Tone mapping.
|
||||
|
||||
There are several tone mapping algorithms. OpenCV implements four of them. The thing to keep in mind is that there is no right way to do tone mapping. Usually, we want to see more detail in the tonemapped image than in any one of the exposure images. Sometimes the goal of tone mapping is to produce realistic images and often times the goal is to produce surreal images. The algorithms implemented in OpenCV tend to produce realistic and therefore less dramatic results.
|
||||
|
||||
Let’s look at the various options. Some of the common parameters of the different tone mapping algorithms are listed below.
|
||||
|
||||
1. gamma : This parameter compresses the dynamic range by applying a gamma correction. When gamma is equal to 1, no correction is applied. A gamma of less than 1 darkens the image, while a gamma greater than 1 brightens the image.
|
||||
|
||||
2. saturation : This parameter is used to increase or decrease the amount of saturation. When saturation is high, the colors are richer and more intense. Saturation value closer to zero, makes the colors fade away to grayscale.
|
||||
|
||||
3. contrast : Controls the contrast ( i.e. log (maxPixelValue/minPixelValue) ) of the output image.
|
||||
|
||||
Let us explore the four tone mapping algorithms available in OpenCV.
|
||||
|
||||
#### Drago Tonemap
|
||||
|
||||
The parameters for Drago Tonemap are shown below
|
||||
|
||||
```
|
||||
createTonemapDrago
|
||||
(
|
||||
float gamma = 1.0f,
|
||||
float saturation = 1.0f,
|
||||
float bias = 0.85f
|
||||
)
|
||||
```
|
||||
|
||||
Here, bias is the value for bias function in [0, 1] range. Values from 0.7 to 0.9 usually give the best results. The default value is 0.85\. For more technical details, please see this [paper][17].
|
||||
|
||||
The C++ and Python code are shown below. The parameters were obtained by trial and error. The final output is multiplied by 3 just because it gave the most pleasing results.
|
||||
|
||||
C++
|
||||
|
||||
```
|
||||
// Tonemap using Drago's method to obtain 24-bit color image
|
||||
Mat ldrDrago;
|
||||
Ptr<TonemapDrago> tonemapDrago = createTonemapDrago(1.0, 0.7);
|
||||
tonemapDrago->process(hdrDebevec, ldrDrago);
|
||||
ldrDrago = 3 * ldrDrago;
|
||||
imwrite("ldr-Drago.jpg", ldrDrago * 255);
|
||||
```
|
||||
|
||||
Python
|
||||
|
||||
```
|
||||
# Tonemap using Drago's method to obtain 24-bit color image
|
||||
tonemapDrago = cv2.createTonemapDrago(1.0, 0.7)
|
||||
ldrDrago = tonemapDrago.process(hdrDebevec)
|
||||
ldrDrago = 3 * ldrDrago
|
||||
cv2.imwrite("ldr-Drago.jpg", ldrDrago * 255)
|
||||
```
|
||||
|
||||
Result
|
||||
|
||||
[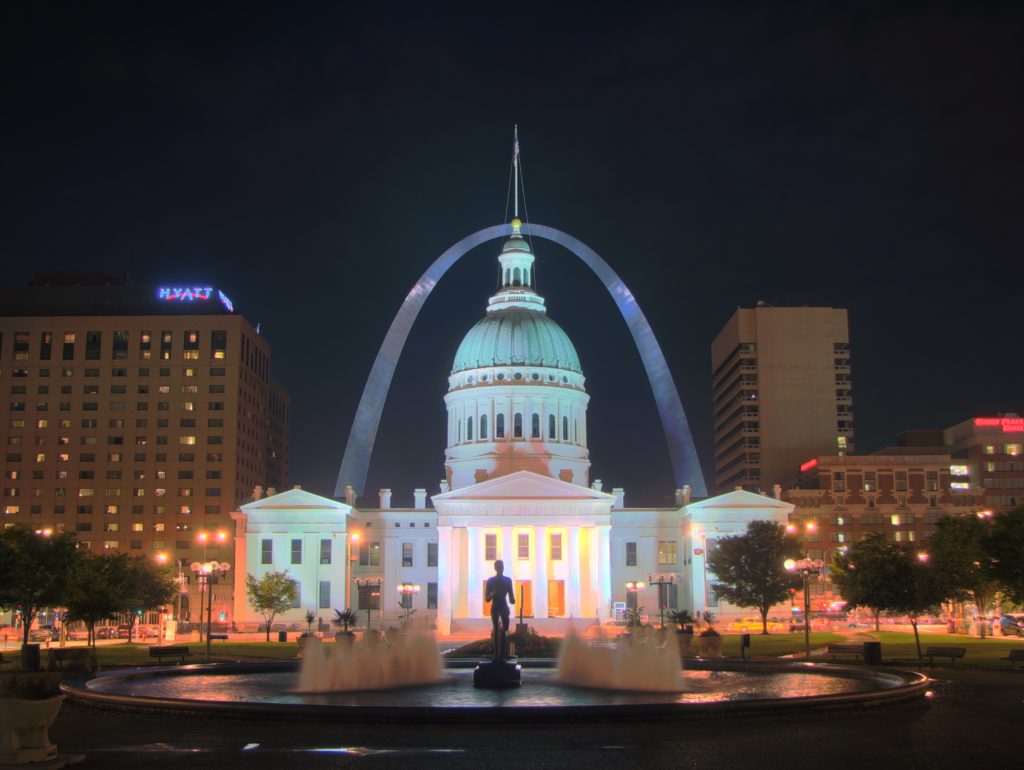][18] HDR tone mapping using Drago’s algorithm
|
||||
|
||||
#### Durand Tonemap
|
||||
|
||||
The parameters for Durand Tonemap are shown below.
|
||||
|
||||
```
|
||||
createTonemapDurand
|
||||
(
|
||||
float gamma = 1.0f,
|
||||
float contrast = 4.0f,
|
||||
float saturation = 1.0f,
|
||||
float sigma_space = 2.0f,
|
||||
float sigma_color = 2.0f
|
||||
);
|
||||
```
|
||||
The algorithm is based on the decomposition of the image into a base layer and a detail layer. The base layer is obtained using an edge-preserving filter called the bilateral filter. sigma_space and sigma_color are the parameters of the bilateral filter that control the amount of smoothing in the spatial and color domains respectively.
|
||||
|
||||
For more details, check out this [paper][19].
|
||||
|
||||
C++
|
||||
|
||||
```
|
||||
// Tonemap using Durand's method obtain 24-bit color image
|
||||
Mat ldrDurand;
|
||||
Ptr<TonemapDurand> tonemapDurand = createTonemapDurand(1.5,4,1.0,1,1);
|
||||
tonemapDurand->process(hdrDebevec, ldrDurand);
|
||||
ldrDurand = 3 * ldrDurand;
|
||||
imwrite("ldr-Durand.jpg", ldrDurand * 255);
|
||||
```
|
||||
Python
|
||||
|
||||
```
|
||||
# Tonemap using Durand's method obtain 24-bit color image
|
||||
tonemapDurand = cv2.createTonemapDurand(1.5,4,1.0,1,1)
|
||||
ldrDurand = tonemapDurand.process(hdrDebevec)
|
||||
ldrDurand = 3 * ldrDurand
|
||||
cv2.imwrite("ldr-Durand.jpg", ldrDurand * 255)
|
||||
```
|
||||
|
||||
Result
|
||||
|
||||
[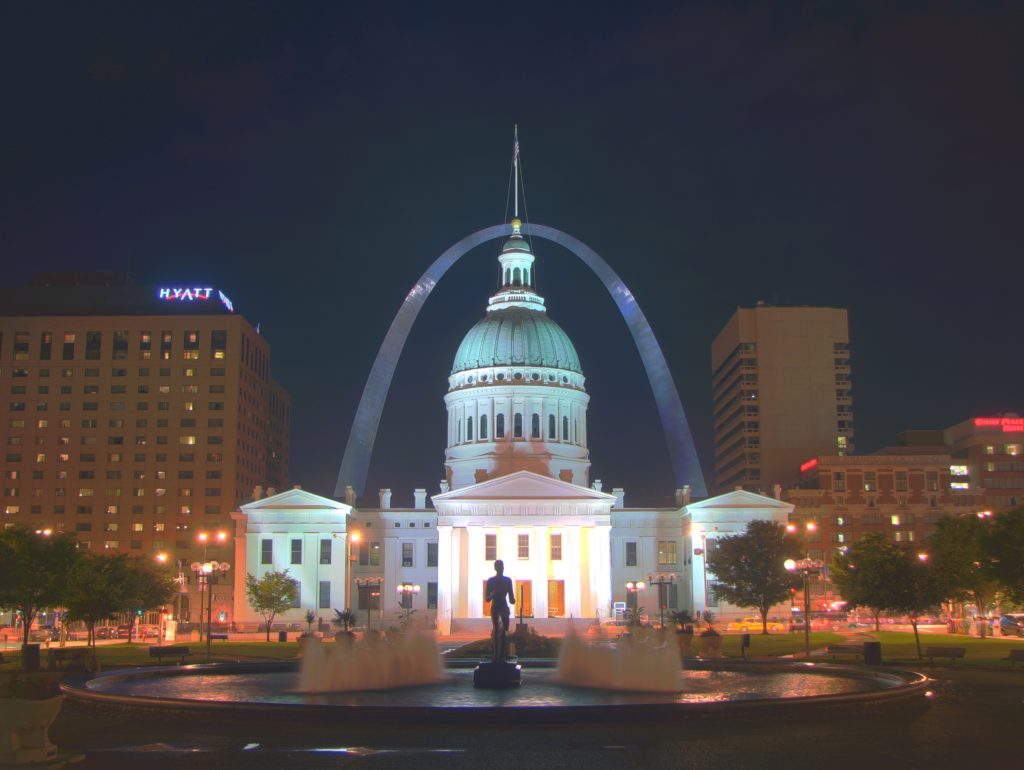][20] HDR tone mapping using Durand’s algorithm
|
||||
|
||||
#### Reinhard Tonemap
|
||||
|
||||
```
|
||||
|
||||
createTonemapReinhard
|
||||
(
|
||||
float gamma = 1.0f,
|
||||
float intensity = 0.0f,
|
||||
float light_adapt = 1.0f,
|
||||
float color_adapt = 0.0f
|
||||
)
|
||||
```
|
||||
|
||||
The parameter intensity should be in the [-8, 8] range. Greater intensity value produces brighter results. light_adapt controls the light adaptation and is in the [0, 1] range. A value of 1 indicates adaptation based only on pixel value and a value of 0 indicates global adaptation. An in-between value can be used for a weighted combination of the two. The parameter color_adapt controls chromatic adaptation and is in the [0, 1] range. The channels are treated independently if the value is set to 1 and the adaptation level is the same for every channel if the value is set to 0\. An in-between value can be used for a weighted combination of the two.
|
||||
|
||||
For more details, check out this [paper][21].
|
||||
|
||||
C++
|
||||
|
||||
```
|
||||
// Tonemap using Reinhard's method to obtain 24-bit color image
|
||||
Mat ldrReinhard;
|
||||
Ptr<TonemapReinhard> tonemapReinhard = createTonemapReinhard(1.5, 0,0,0);
|
||||
tonemapReinhard->process(hdrDebevec, ldrReinhard);
|
||||
imwrite("ldr-Reinhard.jpg", ldrReinhard * 255);
|
||||
```
|
||||
|
||||
Python
|
||||
|
||||
```
|
||||
# Tonemap using Reinhard's method to obtain 24-bit color image
|
||||
tonemapReinhard = cv2.createTonemapReinhard(1.5, 0,0,0)
|
||||
ldrReinhard = tonemapReinhard.process(hdrDebevec)
|
||||
cv2.imwrite("ldr-Reinhard.jpg", ldrReinhard * 255)
|
||||
```
|
||||
|
||||
Result
|
||||
|
||||
[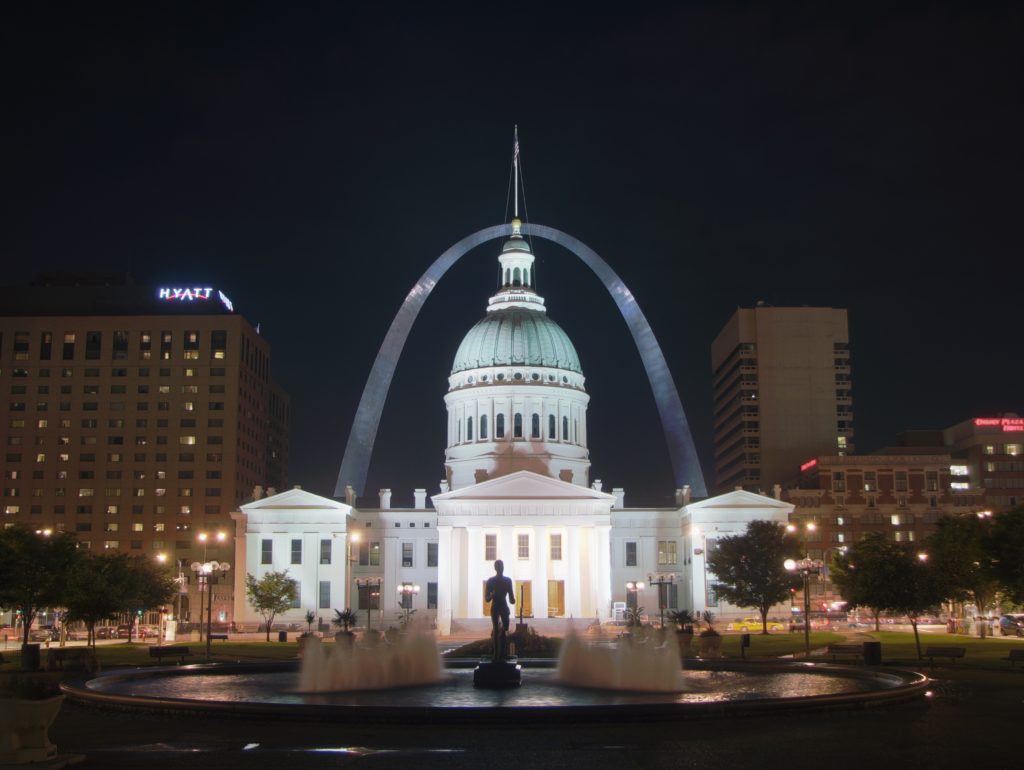][22] HDR tone mapping using Reinhard’s algorithm
|
||||
|
||||
#### Mantiuk Tonemap
|
||||
|
||||
```
|
||||
createTonemapMantiuk
|
||||
(
|
||||
float gamma = 1.0f,
|
||||
float scale = 0.7f,
|
||||
float saturation = 1.0f
|
||||
)
|
||||
```
|
||||
|
||||
The parameter scale is the contrast scale factor. Values from 0.6 to 0.9 produce best results.
|
||||
|
||||
For more details, check out this [paper][23]
|
||||
|
||||
C++
|
||||
|
||||
```
|
||||
// Tonemap using Mantiuk's method to obtain 24-bit color image
|
||||
Mat ldrMantiuk;
|
||||
Ptr<TonemapMantiuk> tonemapMantiuk = createTonemapMantiuk(2.2,0.85, 1.2);
|
||||
tonemapMantiuk->process(hdrDebevec, ldrMantiuk);
|
||||
ldrMantiuk = 3 * ldrMantiuk;
|
||||
imwrite("ldr-Mantiuk.jpg", ldrMantiuk * 255);
|
||||
```
|
||||
|
||||
Python
|
||||
|
||||
```
|
||||
# Tonemap using Mantiuk's method to obtain 24-bit color image
|
||||
tonemapMantiuk = cv2.createTonemapMantiuk(2.2,0.85, 1.2)
|
||||
ldrMantiuk = tonemapMantiuk.process(hdrDebevec)
|
||||
ldrMantiuk = 3 * ldrMantiuk
|
||||
cv2.imwrite("ldr-Mantiuk.jpg", ldrMantiuk * 255)
|
||||
```
|
||||
|
||||
Result
|
||||
|
||||
[][24] HDR Tone mapping using Mantiuk’s algorithm
|
||||
|
||||
### Subscribe & Download Code
|
||||
|
||||
If you liked this article and would like to download code (C++ and Python) and example images used in this post, please [subscribe][25] to our newsletter. You will also receive a free [Computer Vision Resource][26]Guide. In our newsletter, we share OpenCV tutorials and examples written in C++/Python, and Computer Vision and Machine Learning algorithms and news.
|
||||
|
||||
[Subscribe Now][27]
|
||||
|
||||
Image Credits
|
||||
The four exposure images used in this post are licensed under [CC BY-SA 3.0][28] and were downloaded from [Wikipedia’s HDR page][29]. They were photographed by Kevin McCoy.
|
||||
|
||||
--------------------------------------------------------------------------------
|
||||
|
||||
作者简介:
|
||||
|
||||
I am an entrepreneur with a love for Computer Vision and Machine Learning with a dozen years of experience (and a Ph.D.) in the field.
|
||||
|
||||
In 2007, right after finishing my Ph.D., I co-founded TAAZ Inc. with my advisor Dr. David Kriegman and Kevin Barnes. The scalability, and robustness of our computer vision and machine learning algorithms have been put to rigorous test by more than 100M users who have tried our products.
|
||||
|
||||
---------------------------
|
||||
|
||||
via: http://www.learnopencv.com/high-dynamic-range-hdr-imaging-using-opencv-cpp-python/
|
||||
|
||||
作者:[ SATYA MALLICK ][a]
|
||||
译者:[译者ID](https://github.com/译者ID)
|
||||
校对:[校对者ID](https://github.com/校对者ID)
|
||||
|
||||
本文由 [LCTT](https://github.com/LCTT/TranslateProject) 原创编译,[Linux中国](https://linux.cn/) 荣誉推出
|
||||
|
||||
[a]:http://www.learnopencv.com/about/
|
||||
[1]:http://www.learnopencv.com/author/spmallick/
|
||||
[2]:http://www.learnopencv.com/high-dynamic-range-hdr-imaging-using-opencv-cpp-python/#disqus_thread
|
||||
[3]:http://www.learnopencv.com/wp-content/uploads/2017/09/high-dynamic-range-hdr.jpg
|
||||
[4]:http://www.learnopencv.com/wp-content/uploads/2017/10/hdr.zip
|
||||
[5]:http://www.learnopencv.com/wp-content/uploads/2017/10/hdr.zip
|
||||
[6]:https://bigvisionllc.leadpages.net/leadbox/143948b73f72a2%3A173c9390c346dc/5649050225344512/
|
||||
[7]:https://itunes.apple.com/us/app/autobracket-hdr/id923626339?mt=8&ign-mpt=uo%3D8
|
||||
[8]:https://play.google.com/store/apps/details?id=com.almalence.opencam&hl=en
|
||||
[9]:http://www.learnopencv.com/wp-content/uploads/2017/10/hdr-image-sequence.jpg
|
||||
[10]:https://www.howtogeek.com/289712/how-to-see-an-images-exif-data-in-windows-and-macos
|
||||
[11]:https://www.sno.phy.queensu.ca/~phil/exiftool
|
||||
[12]:http://www.learnopencv.com/wp-content/uploads/2017/10/aligned-unaligned-hdr-comparison.jpg
|
||||
[13]:https://www.slrlounge.com/workshop/using-mirror-up-mode-mirror-lockup
|
||||
[14]:http://www.pauldebevec.com/Research/HDR/debevec-siggraph97.pdf
|
||||
[15]:http://www.learnopencv.com/wp-content/uploads/2017/10/camera-response-function.jpg
|
||||
[16]:http://www.learnopencv.com/wp-content/uploads/2017/10/hdr-Photoshop-Tonemapping.jpg
|
||||
[17]:http://resources.mpi-inf.mpg.de/tmo/logmap/logmap.pdf
|
||||
[18]:http://www.learnopencv.com/wp-content/uploads/2017/10/hdr-Drago.jpg
|
||||
[19]:https://people.csail.mit.edu/fredo/PUBLI/Siggraph2002/DurandBilateral.pdf
|
||||
[20]:http://www.learnopencv.com/wp-content/uploads/2017/10/hdr-Durand.jpg
|
||||
[21]:http://citeseerx.ist.psu.edu/viewdoc/download?doi=10.1.1.106.8100&rep=rep1&type=pdf
|
||||
[22]:http://www.learnopencv.com/wp-content/uploads/2017/10/hdr-Reinhard.jpg
|
||||
[23]:http://citeseerx.ist.psu.edu/viewdoc/download?doi=10.1.1.60.4077&rep=rep1&type=pdf
|
||||
[24]:http://www.learnopencv.com/wp-content/uploads/2017/10/hdr-Mantiuk.jpg
|
||||
[25]:https://bigvisionllc.leadpages.net/leadbox/143948b73f72a2%3A173c9390c346dc/5649050225344512/
|
||||
[26]:https://bigvisionllc.leadpages.net/leadbox/143948b73f72a2%3A173c9390c346dc/5649050225344512/
|
||||
[27]:https://bigvisionllc.leadpages.net/leadbox/143948b73f72a2%3A173c9390c346dc/5649050225344512/
|
||||
[28]:https://creativecommons.org/licenses/by-sa/3.0/
|
||||
[29]:https://en.wikipedia.org/wiki/High-dynamic-range_imaging
|
@ -0,0 +1,416 @@
|
||||
使用OpenCV(C ++ / Python)进行高动态范围(HDR)成像
|
||||
============================================================
|
||||
|
||||
在本教程中,我们将学习如何使用由不同曝光设置拍摄的多张图像创建高动态范围(HDR)图像。 我们将以C ++和Python两种形式分享代码。
|
||||
|
||||
### 什么是高动态范围成像?
|
||||
|
||||
大多数数码相机和显示器都是按照24位矩阵捕获或者显示彩色图像。 每个颜色通道有8位,因此每个通道的像素值在0-255范围内。 换句话说,普通的相机或者显示器的动态范围是有限的。
|
||||
|
||||
但是,我们周围世界动态范围极大。 在车库内关灯就会变黑,直接看着太阳就会变得非常亮。 即使不考虑这些极端,在日常情况下,8位的通道勉强可以捕捉到现场场景。 因此,相机会尝试去评估光照并且自动设置曝光,这样图像的感兴趣区就会有良好的动态范围,并且太暗和太亮的部分会被截取,取值为0和255。
|
||||
|
||||
在下图中,左侧的图像是正常曝光的图像。 请注意,由于相机决定使用拍摄主体(我的儿子)的设置,所以背景中的天空已经完全流失了,但是明亮的天空也因此被刷掉了。 右侧的图像是由iPhone生成的HDR图像。
|
||||
|
||||
[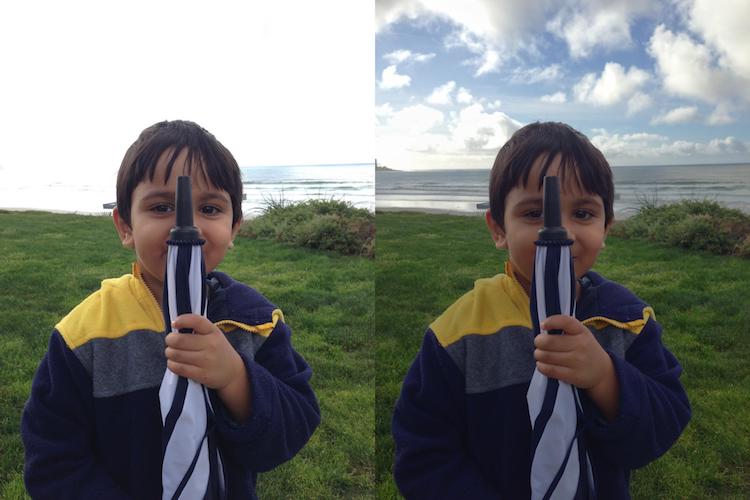][3]
|
||||
|
||||
iPhone是如何拍摄HDR图像的呢? 它实际上采用三种不同的曝光度拍摄了3张图像,3张图像拍摄非常迅速,在3张图像之间几乎没有产生位移。然后组合三幅图像来产生HDR图像。 我们将在下一节看到一些细节。
|
||||
|
||||
将在不同曝光设置下获取的相同场景的不同图像组合的过程称为高动态范围(HDR)成像。
|
||||
|
||||
### 高动态范围(HDR)成像是如何工作的?
|
||||
|
||||
在本节中,我们来看下使用OpenCV创建HDR图像的步骤。
|
||||
|
||||
要想轻松学习本教程,请点击[此处][5][下载][4]C ++和Python代码还有图像。 如果您有兴趣了解更多关于人工智能,计算机视觉和机器学习的信息,请[订阅][6]我们的电子杂志。
|
||||
|
||||
### 第1步:捕获不同曝光度的多张图像
|
||||
|
||||
当我们使用相机拍照时,每个通道只有8位来表示场景的动态范围(亮度范围)。 但是,通过改变快门速度,我们可以在不同的曝光条件下拍摄多个场景图像。 大多数单反相机SLR有一个功能称为自动包围式曝光(AEB),只需按一下按钮,我们就可以在不同的曝光下拍摄多张照片。 如果你正在使用iPhone,你可以使用这个[自动包围式HDR应用程序][7],如果你是一个Android用户,你可以尝试一个[更好的相机应用程序][8]。
|
||||
|
||||
场景没有变化时,在相机上使用自动包围式曝光或在手机上使用自动包围式应用程序,我们可以一张接一张地快速拍摄多张照片。 当我们在iPhone中使用HDR模式时,会拍摄三张照片。
|
||||
|
||||
1. 曝光不足的图像:该图像比正确曝光的图像更暗。 目标是捕捉非常明亮的图像部分。
|
||||
2. 正确曝光的图像:这是相机将根据其估计的照明拍摄的常规图像。
|
||||
3. 曝光过度的图像:该图像比正确曝光的图像更亮。 目标是拍摄非常黑暗的图像部分。
|
||||
|
||||
但是,如果场景的动态范围很大,我们可以拍摄三张以上的图片来合成HDR图像。 在本教程中,我们将使用曝光时间为1/30秒,0.25秒,2.5秒和15秒的4张图像。 缩略图如下所示。
|
||||
|
||||
[][9]
|
||||
|
||||
单反相机或手机的曝光时间和其他设置的信息通常存储在JPEG文件的EXIF元数据中。 查看此[链接][10]可查看Windows和Mac中存储在JPEG文件中的EXIF元数据。 或者,您可以使用我最喜欢的名为[EXIFTOOL][11]的查看EXIF的命令行工具。
|
||||
|
||||
我们先从读取分配到不同曝光时间的图像开始
|
||||
|
||||
C++
|
||||
|
||||
```
|
||||
void readImagesAndTimes(vector<Mat> &images, vector<float> ×)
|
||||
{
|
||||
|
||||
int numImages = 4;
|
||||
|
||||
// 曝光时间列表
|
||||
static const float timesArray[] = {1/30.0f,0.25,2.5,15.0};
|
||||
times.assign(timesArray, timesArray + numImages);
|
||||
|
||||
// 图像文件名称列表
|
||||
static const char* filenames[] = {"img_0.033.jpg", "img_0.25.jpg", "img_2.5.jpg", "img_15.jpg"};
|
||||
for(int i=0; i < numImages; i++)
|
||||
{
|
||||
Mat im = imread(filenames[i]);
|
||||
images.push_back(im);
|
||||
}
|
||||
|
||||
}
|
||||
```
|
||||
|
||||
Python
|
||||
|
||||
```
|
||||
def readImagesAndTimes():
|
||||
# 曝光时间列表
|
||||
times = np.array([ 1/30.0, 0.25, 2.5, 15.0 ], dtype=np.float32)
|
||||
|
||||
# 图像文件名称列表
|
||||
filenames = ["img_0.033.jpg", "img_0.25.jpg", "img_2.5.jpg", "img_15.jpg"]
|
||||
images = []
|
||||
for filename in filenames:
|
||||
im = cv2.imread(filename)
|
||||
images.append(im)
|
||||
|
||||
return images, times
|
||||
```
|
||||
|
||||
### 第2步:对齐图像
|
||||
|
||||
合成HDR图像时使用的图像如果未对齐可能会导致严重的伪影。 在下图中,左侧的图像是使用未对齐的图像组成的HDR图像,右侧的图像是使用对齐的图像的图像。 通过放大图像的一部分,使用红色圆圈显示的,,我们会在左侧图像中看到严重的鬼影。
|
||||
|
||||
[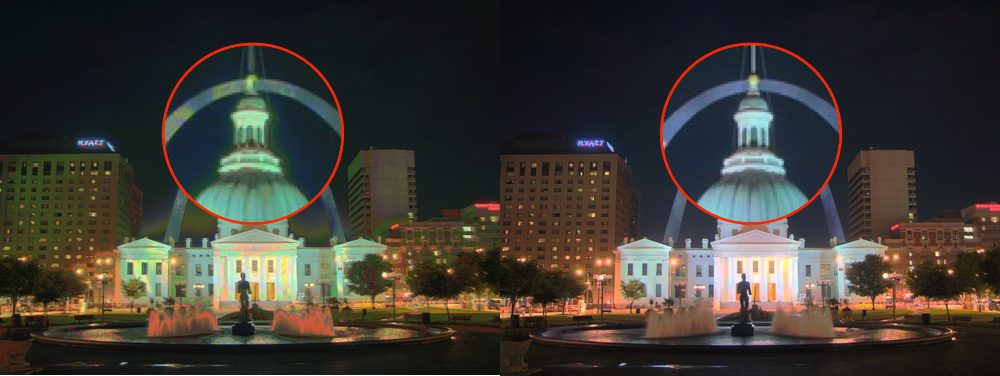][12]
|
||||
|
||||
在拍摄照片制作HDR图像时,专业摄影师自然是将相机安装在三脚架上。 他们还使用称为[镜像锁定][13]功能来减少额外的振动。 即使如此,图像可能仍然没有完美对齐,因为没有办法保证无振动的环境。 使用手持相机或手机拍摄图像时,对齐问题会变得更糟。
|
||||
|
||||
幸运的是,OpenCV提供了一种简单的方法,使用`AlignMTB`对齐这些图像。 该算法将所有图像转换为中值阈值位图(MTB)。 图像的MTB生成方式为将比中值亮度的更亮的分配为1,其余为0。 MTB不随曝光时间的改变而改变。 因此不需要我们指定曝光时间就可以对齐MTB。
|
||||
|
||||
基于MTB的对齐方式的代码如下。
|
||||
|
||||
C++
|
||||
|
||||
```
|
||||
// 对齐输入图像
|
||||
Ptr<AlignMTB> alignMTB = createAlignMTB();
|
||||
alignMTB->process(images, images);
|
||||
```
|
||||
|
||||
Python
|
||||
|
||||
```
|
||||
# 对齐输入图像
|
||||
alignMTB = cv2.createAlignMTB()
|
||||
alignMTB.process(images, images)
|
||||
```
|
||||
|
||||
### 第3步:提取相机响应函数
|
||||
|
||||
典型相机的响应与场景亮度不成线性关系。 那是什么意思呢? 假设有两个物体由同一个相机拍摄,在现实世界中其中一个物体是另一个物体亮度的两倍。 当您测量照片中两个物体的像素亮度时,较亮物体的像素值将不会是较暗物体的两倍。 在不估计相机响应函数(CRF)的情况下,我们将无法将图像合并到一个HDR图像中。
|
||||
|
||||
将多个曝光图像合并为HDR图像意味着什么?
|
||||
|
||||
只考虑图像的某个位置(x,y)一个像素。 如果CRF是线性的,则像素值将直接与曝光时间成比例,除非像素在特定图像中太暗(即接近0)或太亮(即接近255)。 我们可以过滤出这些不好的像素(太暗或太亮),并且将像素值除以曝光时间来估计像素的亮度,然后在像素不差的(太暗或太亮)所有图像上对亮度值取平均。我们可以对所有像素进行这样的处理,并通过对“好”像素进行平均来获得所有像素的单张图像。
|
||||
|
||||
但是CRF不是线性的, 我们需要评估CRF把图像强度变成线性,然后才能合并或者平均它们。
|
||||
|
||||
好消息是,如果我们知道每个图像的曝光时间,则可以从图像估计CRF。 与计算机视觉中的许多问题一样,找到CRF的问题本质是一个最优解问题,其目标是使由数据项和平滑项组成的目标函数最小化。 这些问题通常会降维到线性最小二乘问题,这些问题可以使用奇异值分解(SVD)来解决,奇异值分解是所有线性代数包的一部分。 CRF提取算法的细节在[从照片提取高动态范围辐射图][14]这篇论文中可以找到。
|
||||
|
||||
使用OpenCv的`CalibrateDebevec` 或者`CalibrateRobertson`就可以用2行代码找到CRF。本篇教程中我们使用 `CalibrateDebevec`
|
||||
|
||||
C++
|
||||
|
||||
```
|
||||
// 获取图像响应函数 (CRF)
|
||||
Mat responseDebevec;
|
||||
Ptr<CalibrateDebevec> calibrateDebevec = createCalibrateDebevec();
|
||||
calibrateDebevec->process(images, responseDebevec, times);
|
||||
|
||||
```
|
||||
|
||||
Python
|
||||
|
||||
```
|
||||
# 获取图像响应函数 (CRF)
|
||||
calibrateDebevec = cv2.createCalibrateDebevec()
|
||||
responseDebevec = calibrateDebevec.process(images, times)
|
||||
```
|
||||
|
||||
下图显示了使用红绿蓝通道的图像提取的CRF。
|
||||
|
||||
[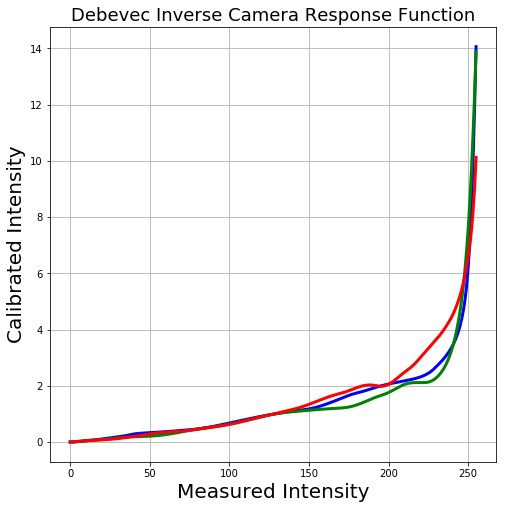][15]
|
||||
|
||||
### 第4步:合并图像
|
||||
|
||||
一旦CRF评估结束,我们可以使用`MergeDebevec`将曝光图像合并成一个HDR图像。 C ++和Python代码如下所示。
|
||||
|
||||
C++
|
||||
|
||||
```
|
||||
// 将图像合并为HDR线性图像
|
||||
Mat hdrDebevec;
|
||||
Ptr<MergeDebevec> mergeDebevec = createMergeDebevec();
|
||||
mergeDebevec->process(images, hdrDebevec, times, responseDebevec);
|
||||
// 保存图像
|
||||
imwrite("hdrDebevec.hdr", hdrDebevec);
|
||||
```
|
||||
|
||||
Python
|
||||
|
||||
```
|
||||
# 将图像合并为HDR线性图像
|
||||
mergeDebevec = cv2.createMergeDebevec()
|
||||
hdrDebevec = mergeDebevec.process(images, times, responseDebevec)
|
||||
# 保存图像
|
||||
cv2.imwrite("hdrDebevec.hdr", hdrDebevec)
|
||||
```
|
||||
|
||||
上面保存的HDR图像可以在Photoshop中加载并进行色调映射。示例图像如下所示。
|
||||
|
||||
[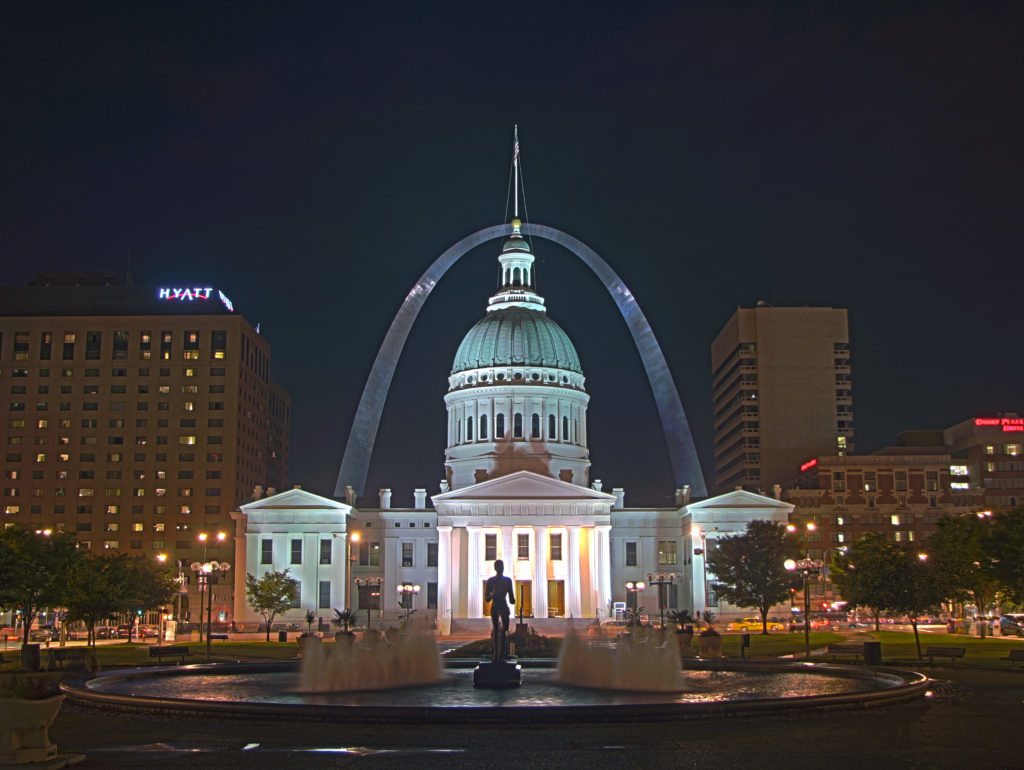][16] HDR Photoshop 色调映射
|
||||
|
||||
### 第5步:色调映射
|
||||
|
||||
现在我们已经将我们的曝光图像合并到一个HDR图像中。 你能猜出这个图像的最小和最大像素值吗? 对于黑色条件,最小值显然为0。 理论最大值是什么? 无限大! 在实践中,不同情况下的最大值是不同的。 如果场景包含非常明亮的光源,那么最大值就会非常大。
|
||||
|
||||
尽管我们已经使用多个图像恢复了相对亮度信息,但是我们现在又面临了新的挑战:将这些信息保存为24位图像用于显示。
|
||||
|
||||
将高动态范围(HDR)图像转换为8位单通道图像的过程称为色调映射。这个过程的同时还需要保留尽可能多的细节。
|
||||
|
||||
有几种色调映射算法。 OpenCV实现了其中的四个。 要记住的是没有一个绝对正确的方法来做色调映射。 通常,我们希望在色调映射图像中看到比任何一个曝光图像更多的细节。 有时色调映射的目标是产生逼真的图像,而且往往是产生超现实图像的目标。 在OpenCV中实现的算法倾向于产生现实的并不那么生动的结果。
|
||||
|
||||
我们来看看各种选项。 以下列出了不同色调映射算法的一些常见参数。
|
||||
|
||||
1. 伽马gamma:该参数通过应用伽马gamma校正来压缩动态范围。 当gamma等于1时,不应用修正。 小于1的伽玛会使图像变暗,而大于1的伽马会使图像变亮。
|
||||
2. 饱和度saturation:该参数用于增加或减少饱和度。 饱和度高时,色彩更丰富,更浓。 饱和度值接近零,使颜色逐渐消失为灰度。
|
||||
3. 对比度contrast:控制输出图像的对比度(即log (maxPixelValue/minPixelValue))。
|
||||
|
||||
让我们来探索OpenCV中可用的四种色调映射算法。
|
||||
|
||||
#### Drago 色调映射
|
||||
|
||||
Drago 色调映射的参数如下所示
|
||||
|
||||
```
|
||||
createTonemapDrago
|
||||
(
|
||||
float gamma = 1.0f,
|
||||
float saturation = 1.0f,
|
||||
float bias = 0.85f
|
||||
)
|
||||
```
|
||||
|
||||
这里,bias是[0,1]范围内偏差函数的值。 从0.7到0.9的值通常效果较好。 默认值是0.85。 有关更多技术细节,请参阅这篇[论文][17]。
|
||||
|
||||
C ++和Python代码如下所示。 参数是通过反复试验获得的。 最后的结果乘以3只是因为它给出了最令人满意的结果。
|
||||
|
||||
C++
|
||||
|
||||
```
|
||||
// 使用Drago色调映射算法获得24位彩色图像
|
||||
Mat ldrDrago;
|
||||
Ptr<TonemapDrago> tonemapDrago = createTonemapDrago(1.0, 0.7);
|
||||
tonemapDrago->process(hdrDebevec, ldrDrago);
|
||||
ldrDrago = 3 * ldrDrago;
|
||||
imwrite("ldr-Drago.jpg", ldrDrago * 255);
|
||||
```
|
||||
|
||||
Python
|
||||
|
||||
```
|
||||
# 使用Drago色调映射算法获得24位彩色图像
|
||||
tonemapDrago = cv2.createTonemapDrago(1.0, 0.7)
|
||||
ldrDrago = tonemapDrago.process(hdrDebevec)
|
||||
ldrDrago = 3 * ldrDrago
|
||||
cv2.imwrite("ldr-Drago.jpg", ldrDrago * 255)
|
||||
```
|
||||
|
||||
结果如下
|
||||
|
||||
[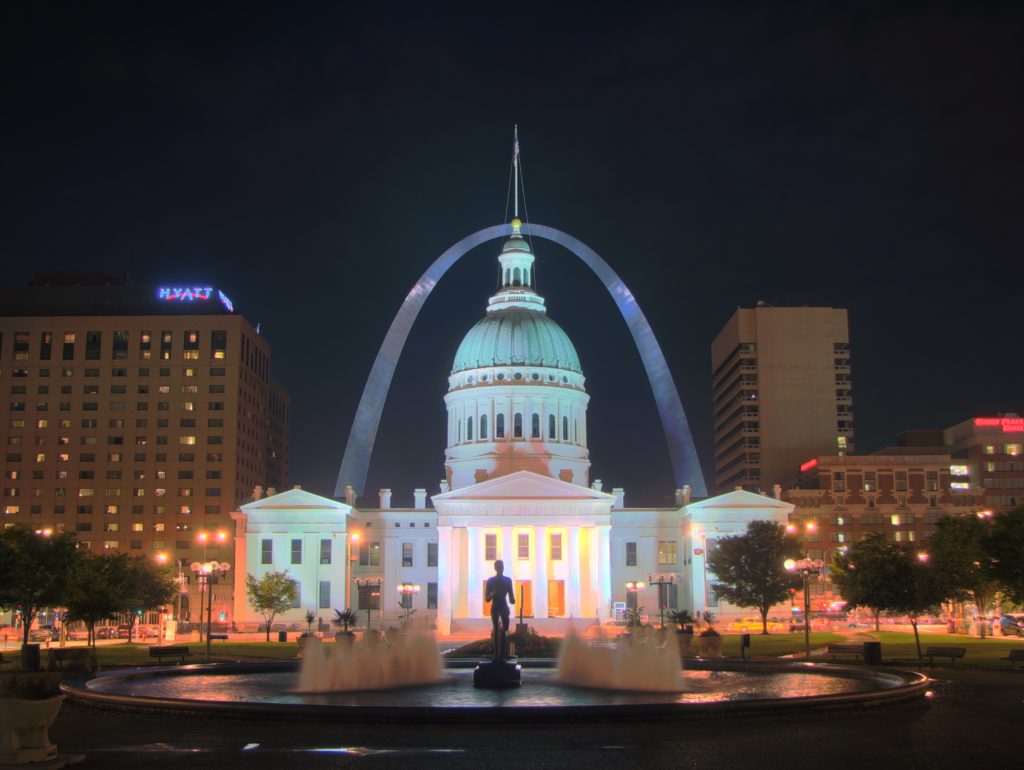][18] 使用Drago算法的HDR色调映射
|
||||
|
||||
#### Durand 色调映射
|
||||
|
||||
Durand 色调映射的参数如下所示
|
||||
|
||||
```
|
||||
createTonemapDurand
|
||||
(
|
||||
float gamma = 1.0f,
|
||||
float contrast = 4.0f,
|
||||
float saturation = 1.0f,
|
||||
float sigma_space = 2.0f,
|
||||
float sigma_color = 2.0f
|
||||
);
|
||||
```
|
||||
该算法基于将图像分解为基础层和细节层。 使用称为双边滤波器的边缘保留滤波器来获得基本层。 sigma_space和sigma_color是双边滤波器的参数,分别控制空间域和彩色域中的平滑量。
|
||||
|
||||
有关更多详细信息,请查看这篇[论文][19]。
|
||||
|
||||
C++
|
||||
|
||||
```
|
||||
// 使用Durand色调映射算法获得24位彩色图像
|
||||
Mat ldrDurand;
|
||||
Ptr<TonemapDurand> tonemapDurand = createTonemapDurand(1.5,4,1.0,1,1);
|
||||
tonemapDurand->process(hdrDebevec, ldrDurand);
|
||||
ldrDurand = 3 * ldrDurand;
|
||||
imwrite("ldr-Durand.jpg", ldrDurand * 255);
|
||||
```
|
||||
Python
|
||||
|
||||
```
|
||||
# 使用Durand色调映射算法获得24位彩色图像
|
||||
tonemapDurand = cv2.createTonemapDurand(1.5,4,1.0,1,1)
|
||||
ldrDurand = tonemapDurand.process(hdrDebevec)
|
||||
ldrDurand = 3 * ldrDurand
|
||||
cv2.imwrite("ldr-Durand.jpg", ldrDurand * 255)
|
||||
```
|
||||
|
||||
结果如下
|
||||
|
||||
[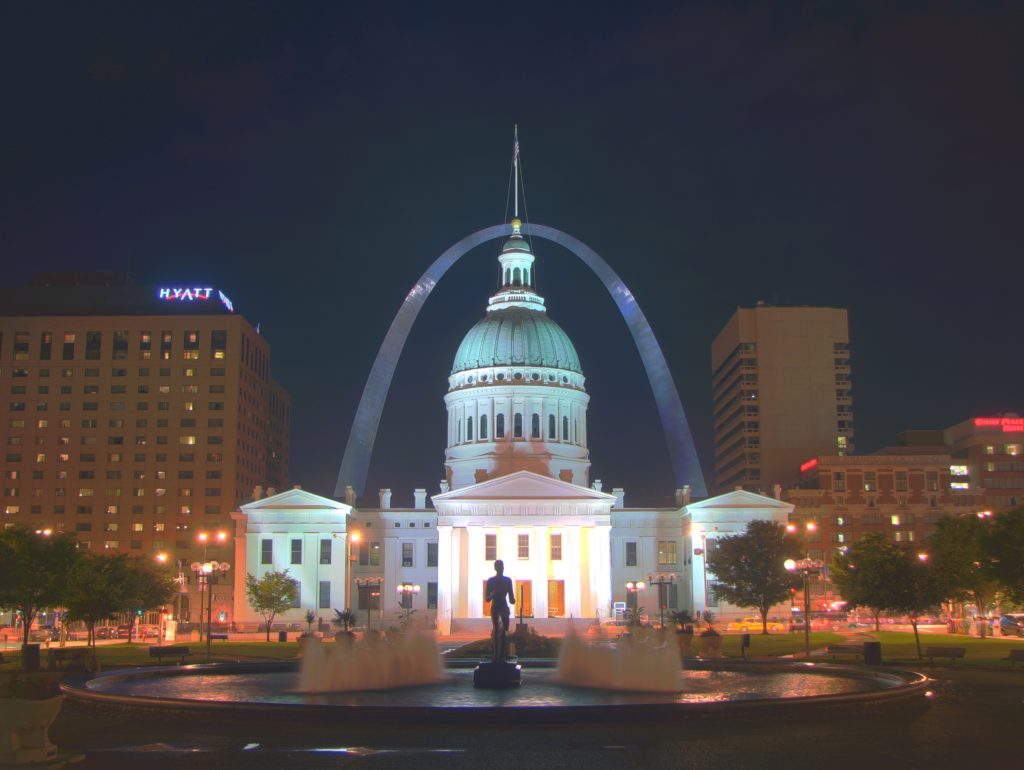][20] 使用Durand算法的HDR色调映射
|
||||
|
||||
#### Reinhard 色调映射
|
||||
|
||||
```
|
||||
|
||||
createTonemapReinhard
|
||||
(
|
||||
float gamma = 1.0f,
|
||||
float intensity = 0.0f,
|
||||
float light_adapt = 1.0f,
|
||||
float color_adapt = 0.0f
|
||||
)
|
||||
```
|
||||
|
||||
intensity 参数应在[-8, 8]范围内。 更高的亮度值会产生更明亮的结果。 light_adapt控制灯光,范围为[0, 1]。 值1表示仅基于像素值的自适应,而值0表示全局自适应。 中间值可以用于两者的加权组合。 参数color_adapt控制色彩,范围为[0, 1]。 如果值被设置为1,则通道被独立处理,如果该值被设置为0,则每个通道的适应级别相同。中间值可以用于两者的加权组合。
|
||||
|
||||
有关更多详细信息,请查看这篇[论文][21]。
|
||||
|
||||
C++
|
||||
|
||||
```
|
||||
// 使用Reinhard色调映射算法获得24位彩色图像
|
||||
Mat ldrReinhard;
|
||||
Ptr<TonemapReinhard> tonemapReinhard = createTonemapReinhard(1.5, 0,0,0);
|
||||
tonemapReinhard->process(hdrDebevec, ldrReinhard);
|
||||
imwrite("ldr-Reinhard.jpg", ldrReinhard * 255);
|
||||
```
|
||||
|
||||
Python
|
||||
|
||||
```
|
||||
# 使用Reinhard色调映射算法获得24位彩色图像
|
||||
tonemapReinhard = cv2.createTonemapReinhard(1.5, 0,0,0)
|
||||
ldrReinhard = tonemapReinhard.process(hdrDebevec)
|
||||
cv2.imwrite("ldr-Reinhard.jpg", ldrReinhard * 255)
|
||||
```
|
||||
|
||||
结果如下
|
||||
|
||||
[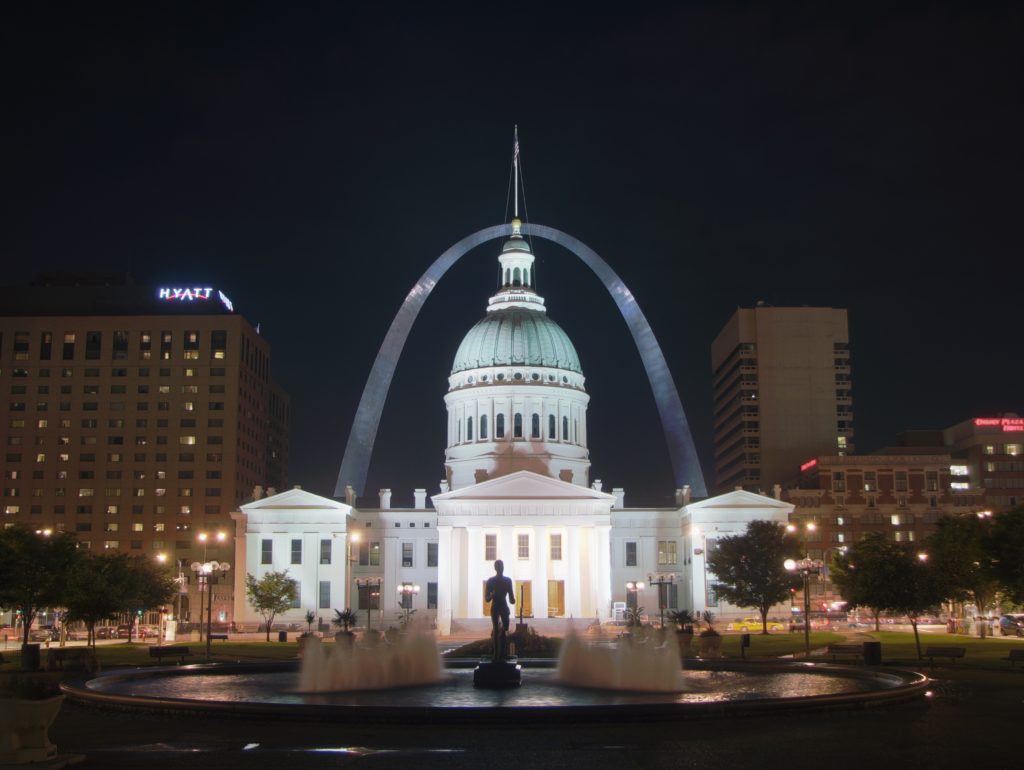][22] 使用Reinhard算法的HDR色调映射
|
||||
|
||||
#### Mantiuk 色调映射
|
||||
|
||||
```
|
||||
createTonemapMantiuk
|
||||
(
|
||||
float gamma = 1.0f,
|
||||
float scale = 0.7f,
|
||||
float saturation = 1.0f
|
||||
)
|
||||
```
|
||||
|
||||
参数scale是对比度比例因子。 从0.7到0.9的值通常效果较好
|
||||
|
||||
有关更多详细信息,请查看这篇[论文][23]。
|
||||
|
||||
C++
|
||||
|
||||
```
|
||||
// 使用Mantiuk色调映射算法获得24位彩色图像
|
||||
Mat ldrMantiuk;
|
||||
Ptr<TonemapMantiuk> tonemapMantiuk = createTonemapMantiuk(2.2,0.85, 1.2);
|
||||
tonemapMantiuk->process(hdrDebevec, ldrMantiuk);
|
||||
ldrMantiuk = 3 * ldrMantiuk;
|
||||
imwrite("ldr-Mantiuk.jpg", ldrMantiuk * 255);
|
||||
```
|
||||
|
||||
Python
|
||||
|
||||
```
|
||||
# 使用Mantiuk色调映射算法获得24位彩色图像
|
||||
tonemapMantiuk = cv2.createTonemapMantiuk(2.2,0.85, 1.2)
|
||||
ldrMantiuk = tonemapMantiuk.process(hdrDebevec)
|
||||
ldrMantiuk = 3 * ldrMantiuk
|
||||
cv2.imwrite("ldr-Mantiuk.jpg", ldrMantiuk * 255)
|
||||
```
|
||||
|
||||
结果如下
|
||||
|
||||
[][24] 使用Mantiuk算法的HDR色调映射
|
||||
|
||||
### 订阅然后下载代码
|
||||
|
||||
如果你喜欢这篇文章,并希望下载本文中使用的代码(C ++和Python)和示例图片,请[订阅][25]我们的电子杂志。 您还将获得免费的[计算机视觉资源][26]指南。 在我们的电子杂志中,我们分享了用C ++还有Python编写的OpenCV教程和例子,以及计算机视觉和机器学习的算法和新闻。
|
||||
|
||||
[点此订阅][27]
|
||||
|
||||
图像学分
|
||||
|
||||
本文中使用的四个曝光图像获得[CC BY-SA 3.0][28]许可,并从[维基百科的HDR页面][29]下载。 图像由Kevin McCoy拍摄。
|
||||
|
||||
--------------------------------------------------------------------------------
|
||||
|
||||
作者简介:
|
||||
|
||||
我是一位热爱计算机视觉和机器学习的企业家,拥有十多年的实践经验(还有博士学位)。
|
||||
|
||||
2007年,在完成博士学位之后,我和我的顾问David Kriegman博士还有Kevin Barnes共同创办了TAAZ公司.。 我们的计算机视觉和机器学习算法的可扩展性和鲁棒性已经经过了试用了我们产品的超过1亿的用户的严格测试。
|
||||
|
||||
---------------------------
|
||||
|
||||
via: http://www.learnopencv.com/high-dynamic-range-hdr-imaging-using-opencv-cpp-python/
|
||||
|
||||
作者:[SATYA MALLICK ][a]
|
||||
译者:[Flowsnow](https://github.com/Flowsnow)
|
||||
校对:[校对者ID](https://github.com/校对者ID)
|
||||
|
||||
本文由 [LCTT](https://github.com/LCTT/TranslateProject) 原创编译,[Linux中国](https://linux.cn/) 荣誉推出
|
||||
|
||||
[a]:http://www.learnopencv.com/about/
|
||||
[1]:http://www.learnopencv.com/author/spmallick/
|
||||
[2]:http://www.learnopencv.com/high-dynamic-range-hdr-imaging-using-opencv-cpp-python/#disqus_thread
|
||||
[3]:http://www.learnopencv.com/wp-content/uploads/2017/09/high-dynamic-range-hdr.jpg
|
||||
[4]:http://www.learnopencv.com/wp-content/uploads/2017/10/hdr.zip
|
||||
[5]:http://www.learnopencv.com/wp-content/uploads/2017/10/hdr.zip
|
||||
[6]:https://bigvisionllc.leadpages.net/leadbox/143948b73f72a2%3A173c9390c346dc/5649050225344512/
|
||||
[7]:https://itunes.apple.com/us/app/autobracket-hdr/id923626339?mt=8&amp;ign-mpt=uo%3D8
|
||||
[8]:https://play.google.com/store/apps/details?id=com.almalence.opencam&amp;hl=en
|
||||
[9]:http://www.learnopencv.com/wp-content/uploads/2017/10/hdr-image-sequence.jpg
|
||||
[10]:https://www.howtogeek.com/289712/how-to-see-an-images-exif-data-in-windows-and-macos
|
||||
[11]:https://www.sno.phy.queensu.ca/~phil/exiftool
|
||||
[12]:http://www.learnopencv.com/wp-content/uploads/2017/10/aligned-unaligned-hdr-comparison.jpg
|
||||
[13]:https://www.slrlounge.com/workshop/using-mirror-up-mode-mirror-lockup
|
||||
[14]:http://www.pauldebevec.com/Research/HDR/debevec-siggraph97.pdf
|
||||
[15]:http://www.learnopencv.com/wp-content/uploads/2017/10/camera-response-function.jpg
|
||||
[16]:http://www.learnopencv.com/wp-content/uploads/2017/10/hdr-Photoshop-Tonemapping.jpg
|
||||
[17]:http://resources.mpi-inf.mpg.de/tmo/logmap/logmap.pdf
|
||||
[18]:http://www.learnopencv.com/wp-content/uploads/2017/10/hdr-Drago.jpg
|
||||
[19]:https://people.csail.mit.edu/fredo/PUBLI/Siggraph2002/DurandBilateral.pdf
|
||||
[20]:http://www.learnopencv.com/wp-content/uploads/2017/10/hdr-Durand.jpg
|
||||
[21]:http://citeseerx.ist.psu.edu/viewdoc/download?doi=10.1.1.106.8100&amp;rep=rep1&amp;type=pdf
|
||||
[22]:http://www.learnopencv.com/wp-content/uploads/2017/10/hdr-Reinhard.jpg
|
||||
[23]:http://citeseerx.ist.psu.edu/viewdoc/download?doi=10.1.1.60.4077&amp;rep=rep1&amp;type=pdf
|
||||
[24]:http://www.learnopencv.com/wp-content/uploads/2017/10/hdr-Mantiuk.jpg
|
||||
[25]:https://bigvisionllc.leadpages.net/leadbox/143948b73f72a2%3A173c9390c346dc/5649050225344512/
|
||||
[26]:https://bigvisionllc.leadpages.net/leadbox/143948b73f72a2%3A173c9390c346dc/5649050225344512/
|
||||
[27]:https://bigvisionllc.leadpages.net/leadbox/143948b73f72a2%3A173c9390c346dc/5649050225344512/
|
||||
[28]:https://creativecommons.org/licenses/by-sa/3.0/
|
||||
[29]:https://en.wikipedia.org/wiki/High-dynamic-range_imaging
|
Loading…
Reference in New Issue
Block a user