mirror of
https://github.com/LCTT/TranslateProject.git
synced 2025-03-27 02:30:10 +08:00
translated (#10281)
This commit is contained in:
parent
6fab8d3a65
commit
32b77557cd
@ -1,244 +0,0 @@
|
||||
ucasFL translating
|
||||
|
||||
Top 3 Python libraries for data science
|
||||
======
|
||||
Turn Python into a scientific data analysis and modeling tool with these libraries.
|
||||
|
||||

|
||||
|
||||
Python's many attractions—such as efficiency, code readability, and speed—have made it the go-to programming language for data science enthusiasts. Python is usually the preferred choice for data scientists and machine learning experts who want to escalate the functionalities of their applications. (For example, Andrey Bulezyuk used the Python programming language to create an amazing [machine learning application][1].)
|
||||
|
||||
Because of its extensive usage, Python has a huge number of libraries that make it easier for data scientists to complete complicated tasks without many coding hassles. Here are the top 3 Python libraries for data science; check them out if you want to kickstart your career in the field.
|
||||
|
||||
### 1\. NumPy
|
||||
|
||||
[NumPy][2] (short for Numerical Python) is one of the top libraries equipped with useful resources to help data scientists turn Python into a powerful scientific analysis and modelling tool. The popular open source library is available under the BSD license. It is the foundational Python library for performing tasks in scientific computing. NumPy is part of a bigger Python-based ecosystem of open source tools called SciPy.
|
||||
|
||||
The library empowers Python with substantial data structures for effortlessly performing multi-dimensional arrays and matrices calculations. Besides its uses in solving linear algebra equations and other mathematical calculations, NumPy is also used as a versatile multi-dimensional container for different types of generic data.
|
||||
|
||||
Furthermore, it integrates flawlessly with other programming languages like C/C++ and Fortran. The versatility of the NumPy library allows it to easily and swiftly coalesce with an extensive range of databases and tools. For example, let's see how NumPy (abbreviated **np** ) can be used for multiplying two matrices.
|
||||
|
||||
Let's start by importing the library (we'll be using the Jupyter notebook for these examples).
|
||||
|
||||
```
|
||||
import numpy as np
|
||||
```
|
||||
|
||||
Next, let's use the **eye()** function to generate an identity matrix with the stipulated dimensions.
|
||||
|
||||
```
|
||||
matrix_one = np.eye(3)
|
||||
matrix_one
|
||||
```
|
||||
|
||||
Here is the output:
|
||||
|
||||
```
|
||||
array([[1., 0., 0.],
|
||||
[0., 1., 0.],
|
||||
[0., 0., 1.]])
|
||||
```
|
||||
|
||||
Let's generate another 3x3 matrix.
|
||||
|
||||
We'll use the **arange([starting number], [stopping number])** function to arrange numbers. Note that the first parameter in the function is the initial number to be listed and the last number is not included in the generated results.
|
||||
|
||||
Also, the **reshape()** function is applied to modify the dimensions of the originally generated matrix into the desired dimension. For the matrices to be "multiply-able," they should be of the same dimension.
|
||||
|
||||
```
|
||||
matrix_two = np.arange(1,10).reshape(3,3)
|
||||
matrix_two
|
||||
```
|
||||
|
||||
Here is the output:
|
||||
|
||||
```
|
||||
array([[1, 2, 3],
|
||||
[4, 5, 6],
|
||||
[7, 8, 9]])
|
||||
```
|
||||
|
||||
Let's use the **dot()** function to multiply the two matrices.
|
||||
|
||||
```
|
||||
matrix_multiply = np.dot(matrix_one, matrix_two)
|
||||
|
||||
matrix_multiply
|
||||
|
||||
```
|
||||
|
||||
Here is the output:
|
||||
|
||||
```
|
||||
array([[1., 2., 3.],
|
||||
[4., 5., 6.],
|
||||
[7., 8., 9.]])
|
||||
```
|
||||
|
||||
Great!
|
||||
|
||||
We managed to multiply two matrices without using vanilla Python.
|
||||
|
||||
Here is the entire code for this example:
|
||||
|
||||
```
|
||||
import numpy as np
|
||||
#generating a 3 by 3 identity matrix
|
||||
matrix_one = np.eye(3)
|
||||
matrix_one
|
||||
#generating another 3 by 3 matrix for multiplication
|
||||
matrix_two = np.arange(1,10).reshape(3,3)
|
||||
matrix_two
|
||||
#multiplying the two arrays
|
||||
matrix_multiply = np.dot(matrix_one, matrix_two)
|
||||
matrix_multiply
|
||||
```
|
||||
|
||||
### 2\. Pandas
|
||||
|
||||
[Pandas][3] is another great library that can enhance your Python skills for data science. Just like NumPy, it belongs to the family of SciPy open source software and is available under the BSD free software license.
|
||||
|
||||
Pandas offers versatile and powerful tools for munging data structures and performing extensive data analysis. The library works well with incomplete, unstructured, and unordered real-world data—and comes with tools for shaping, aggregating, analyzing, and visualizing datasets.
|
||||
|
||||
There are three types of data structures in this library:
|
||||
|
||||
* Series: single-dimensional, homogeneous array
|
||||
* DataFrame: two-dimensional with heterogeneously typed columns
|
||||
* Panel: three-dimensional, size-mutable array
|
||||
|
||||
|
||||
|
||||
For example, let's see how the Panda Python library (abbreviated **pd** ) can be used for performing some descriptive statistical calculations.
|
||||
|
||||
Let's start by importing the library.
|
||||
|
||||
```
|
||||
import pandas as pd
|
||||
```
|
||||
|
||||
Let's create a dictionary of series.
|
||||
|
||||
```
|
||||
d = {'Name':pd.Series(['Alfrick','Michael','Wendy','Paul','Dusan','George','Andreas',
|
||||
'Irene','Sagar','Simon','James','Rose']),
|
||||
'Years of Experience':pd.Series([5,9,1,4,3,4,7,9,6,8,3,1]),
|
||||
'Programming Language':pd.Series(['Python','JavaScript','PHP','C++','Java','Scala','React','Ruby','Angular','PHP','Python','JavaScript'])
|
||||
}
|
||||
```
|
||||
|
||||
Let's create a DataFrame.
|
||||
|
||||
```
|
||||
df = pd.DataFrame(d)
|
||||
```
|
||||
|
||||
Here is a nice table of the output:
|
||||
|
||||
```
|
||||
Name Programming Language Years of Experience
|
||||
0 Alfrick Python 5
|
||||
1 Michael JavaScript 9
|
||||
2 Wendy PHP 1
|
||||
3 Paul C++ 4
|
||||
4 Dusan Java 3
|
||||
5 George Scala 4
|
||||
6 Andreas React 7
|
||||
7 Irene Ruby 9
|
||||
8 Sagar Angular 6
|
||||
9 Simon PHP 8
|
||||
10 James Python 3
|
||||
11 Rose JavaScript 1
|
||||
```
|
||||
|
||||
Here is the entire code for this example:
|
||||
|
||||
```
|
||||
import pandas as pd
|
||||
#creating a dictionary of series
|
||||
d = {'Name':pd.Series(['Alfrick','Michael','Wendy','Paul','Dusan','George','Andreas',
|
||||
'Irene','Sagar','Simon','James','Rose']),
|
||||
'Years of Experience':pd.Series([5,9,1,4,3,4,7,9,6,8,3,1]),
|
||||
'Programming Language':pd.Series(['Python','JavaScript','PHP','C++','Java','Scala','React','Ruby','Angular','PHP','Python','JavaScript'])
|
||||
}
|
||||
|
||||
#Create a DataFrame
|
||||
df = pd.DataFrame(d)
|
||||
print(df)
|
||||
```
|
||||
|
||||
### 3\. Matplotlib
|
||||
|
||||
[Matplotlib][4] is also part of the SciPy core packages and offered under the BSD license. It is a popular Python scientific library used for producing simple and powerful visualizations. You can use the Python framework for data science for generating creative graphs, charts, histograms, and other shapes and figures—without worrying about writing many lines of code. For example, let's see how the Matplotlib library can be used to create a simple bar chart.
|
||||
|
||||
Let's start by importing the library.
|
||||
|
||||
```
|
||||
from matplotlib import pyplot as plt
|
||||
```
|
||||
|
||||
Let's generate values for both the x-axis and the y-axis.
|
||||
|
||||
```
|
||||
x = [2, 4, 6, 8, 10]
|
||||
y = [10, 11, 6, 7, 4]
|
||||
```
|
||||
|
||||
Let's call the function for plotting the bar chart.
|
||||
|
||||
```
|
||||
plt.bar(x,y)
|
||||
```
|
||||
|
||||
Let's show the plot.
|
||||
|
||||
```
|
||||
plt.show()
|
||||
```
|
||||
|
||||
Here is the bar chart:
|
||||
|
||||
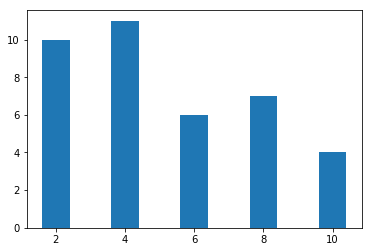
|
||||
|
||||
Here is the entire code for this example:
|
||||
|
||||
```
|
||||
#importing Matplotlib Python library
|
||||
from matplotlib import pyplot as plt
|
||||
#same as import matplotlib.pyplot as plt
|
||||
|
||||
#generating values for x-axis
|
||||
x = [2, 4, 6, 8, 10]
|
||||
|
||||
#generating vaues for y-axis
|
||||
y = [10, 11, 6, 7, 4]
|
||||
|
||||
#calling function for plotting the bar chart
|
||||
plt.bar(x,y)
|
||||
|
||||
#showing the plot
|
||||
plt.show()
|
||||
```
|
||||
|
||||
### Wrapping up
|
||||
|
||||
The Python programming language has always done a good job in data crunching and preparation, but less so for complicated scientific data analysis and modeling. The top Python frameworks for [data science][5] help fill this gap, allowing you to carry out complex mathematical computations and create sophisticated models that make sense of your data.
|
||||
|
||||
Which other Python data-mining libraries do you know? What's your experience with them? Please share your comments below.
|
||||
|
||||
--------------------------------------------------------------------------------
|
||||
|
||||
via: https://opensource.com/article/18/9/top-3-python-libraries-data-science
|
||||
|
||||
作者:[Dr.Michael J.Garbade][a]
|
||||
选题:[lujun9972](https://github.com/lujun9972)
|
||||
译者:[译者ID](https://github.com/译者ID)
|
||||
校对:[校对者ID](https://github.com/校对者ID)
|
||||
|
||||
本文由 [LCTT](https://github.com/LCTT/TranslateProject) 原创编译,[Linux中国](https://linux.cn/) 荣誉推出
|
||||
|
||||
[a]: https://opensource.com/users/drmjg
|
||||
[1]: https://www.liveedu.tv/andreybu/REaxr-machine-learning-model-python-sklearn-kera/oPGdP-machine-learning-model-python-sklearn-kera/
|
||||
[2]: http://www.numpy.org/
|
||||
[3]: http://pandas.pydata.org/
|
||||
[4]: https://matplotlib.org/
|
||||
[5]: https://www.liveedu.tv/guides/data-science/
|
@ -0,0 +1,246 @@
|
||||
3 个用于数据科学的顶级 Python 库
|
||||
======
|
||||
|
||||
>使用这些库把 Python 变成一个科学数据分析和建模工具。
|
||||
|
||||
![][7]
|
||||
|
||||
Python 的许多特性,比如开发效率、代码可读性、速度等使之成为了数据科学爱好者的首选编程语言。对于想要升级应用程序功能的数据科学家和机器学习专家来说,Python 通常是最好的选择(比如,Andrey Bulezyuk 使用 Python 语言创造了一个优秀的[机器学习应用程序][1])。
|
||||
|
||||
由于 Python 的广泛使用,因此它拥有大量的库,使得数据科学家能够很容易地完成复杂的任务,而且不会遇到许多编码困难。下面列出 3 个用于数据科学的顶级 Python 库。如果你想在数据科学这一领域开始你的职业生涯,就去了解一下它们吧。
|
||||
|
||||
### NumPy
|
||||
|
||||
[NumPy][2](数值 Python 的简称)是其中一个顶级数据科学库,它拥有许多有用的资源,从而帮助数据科学家把 Python 变成一个强大的科学分析和建模工具。NumPy 是在 BSD 许可证的许可下开源的,它是在科学计算中执行任务的基础 Python 库。SciPy 是一个更大的基于 Python 生态系统的开源工具,而 NumPy 是 SciPy 非常重要的一部分。
|
||||
|
||||
NumPy 为 Python 提供了大量数据结构,从而能够轻松地执行多维数组和矩阵运算。除了用于求解线性代数方程和其它数学计算之外,NumPy 还可以用做不同类型通用数据的多维容器。
|
||||
|
||||
此外,NumPy 还可以和其他编程语言无缝集成,比如 C/C++ 和 Fortran。NumPy 的多功能性使得它可以简单而快速地与大量数据库和工具结合。比如,让我们来看一下如何使用 NumPy(缩写成 `np`)来实现两个矩阵的乘法运算。
|
||||
|
||||
我们首先导入 NumPy 库(在这些例子中,我将使用 Jupyter notebook):
|
||||
|
||||
```
|
||||
import numpy as np
|
||||
```
|
||||
|
||||
接下来,使用 `eye()` 函数来生成指定维数的单位矩阵:
|
||||
|
||||
```
|
||||
matrix_one = np.eye(3)
|
||||
matrix_one
|
||||
```
|
||||
|
||||
输出如下:
|
||||
|
||||
```
|
||||
array([[1., 0., 0.],
|
||||
[0., 1., 0.],
|
||||
[0., 0., 1.]])
|
||||
```
|
||||
|
||||
让我们生成另一个 3x3 矩阵。
|
||||
|
||||
我们使用 `arange([starting number], [stopping number])` 函数来排列数字。注意,函数中的第一个参数是需要列出的初始数字,而后一个数字不包含在生成的结果中。
|
||||
|
||||
另外,使用 `reshape()` 函数把原始生成的矩阵的维度改成我们需要的维度。为了使两个矩阵“可乘”,它们需要有相同的维度。
|
||||
|
||||
```
|
||||
matrix_two = np.arange(1,10).reshape(3,3)
|
||||
matrix_two
|
||||
```
|
||||
|
||||
Here is the output:
|
||||
输出如下:
|
||||
|
||||
```
|
||||
array([[1, 2, 3],
|
||||
[4, 5, 6],
|
||||
[7, 8, 9]])
|
||||
```
|
||||
|
||||
接下来,使用 `dot()` 函数将两个矩阵相乘。
|
||||
|
||||
```
|
||||
matrix_multiply = np.dot(matrix_one, matrix_two)
|
||||
|
||||
matrix_multiply
|
||||
|
||||
```
|
||||
|
||||
相乘后的输出如下:
|
||||
|
||||
```
|
||||
array([[1., 2., 3.],
|
||||
[4., 5., 6.],
|
||||
[7., 8., 9.]])
|
||||
```
|
||||
|
||||
太好了!
|
||||
|
||||
我们成功使用 NumPy 完成了两个矩阵的相乘,而不是使用<ruby>普通冗长<rt>vanilla</rt></ruby>的 Python 代码。
|
||||
|
||||
下面是这个例子的完整代码:
|
||||
|
||||
```
|
||||
import numpy as np
|
||||
#生成一个 3x3 单位矩阵
|
||||
matrix_one = np.eye(3)
|
||||
matrix_one
|
||||
#生成另一个 3x3 矩阵以用来做乘法运算
|
||||
matrix_two = np.arange(1,10).reshape(3,3)
|
||||
matrix_two
|
||||
#将两个矩阵相乘
|
||||
matrix_multiply = np.dot(matrix_one, matrix_two)
|
||||
matrix_multiply
|
||||
```
|
||||
|
||||
### Pandas
|
||||
|
||||
[Pandas][3] 是另一个可以提高你的 Python 数据科学技能的优秀库。就和 NumPy 一样,它属于 SciPy 开源软件家族,可以在 BSD 免费许可证许可下使用。
|
||||
|
||||
Pandas 提供了多功能并且很强大的工具用于管理数据结构和执行大量数据分析。该库能够很好的处理不完整、非结构化和无序的真实世界数据,并且提供了用于整形、聚合、分析和可视化数据集的工具
|
||||
|
||||
Pandas 中有三种类型的数据结构:
|
||||
|
||||
* Series: 一维、相同数据类型的数组
|
||||
* DataFrame: 二维异型矩阵
|
||||
* Panel: 三维大小可变数组
|
||||
|
||||
|
||||
|
||||
例如,我们来看一下如何使用 Panda 库(缩写成 `pd`)来执行一些描述性统计计算。
|
||||
|
||||
首先导入该库:
|
||||
|
||||
```
|
||||
import pandas as pd
|
||||
```
|
||||
|
||||
然后,创建一个<ruby>序列<rt>series</rt></ruby>字典:
|
||||
|
||||
```
|
||||
d = {'Name':pd.Series(['Alfrick','Michael','Wendy','Paul','Dusan','George','Andreas',
|
||||
'Irene','Sagar','Simon','James','Rose']),
|
||||
'Years of Experience':pd.Series([5,9,1,4,3,4,7,9,6,8,3,1]),
|
||||
'Programming Language':pd.Series(['Python','JavaScript','PHP','C++','Java','Scala','React','Ruby','Angular','PHP','Python','JavaScript'])
|
||||
}
|
||||
```
|
||||
|
||||
接下来,再创建一个<ruby>数据框<rt>DataFrame</rt></ruby>:
|
||||
|
||||
```
|
||||
df = pd.DataFrame(d)
|
||||
```
|
||||
|
||||
输出是一个非常规整的表:
|
||||
|
||||
```
|
||||
Name Programming Language Years of Experience
|
||||
0 Alfrick Python 5
|
||||
1 Michael JavaScript 9
|
||||
2 Wendy PHP 1
|
||||
3 Paul C++ 4
|
||||
4 Dusan Java 3
|
||||
5 George Scala 4
|
||||
6 Andreas React 7
|
||||
7 Irene Ruby 9
|
||||
8 Sagar Angular 6
|
||||
9 Simon PHP 8
|
||||
10 James Python 3
|
||||
11 Rose JavaScript 1
|
||||
```
|
||||
|
||||
下面是这个例子的完整代码:
|
||||
|
||||
```
|
||||
import pandas as pd
|
||||
#创建一个序列字典
|
||||
d = {'Name':pd.Series(['Alfrick','Michael','Wendy','Paul','Dusan','George','Andreas',
|
||||
'Irene','Sagar','Simon','James','Rose']),
|
||||
'Years of Experience':pd.Series([5,9,1,4,3,4,7,9,6,8,3,1]),
|
||||
'Programming Language':pd.Series(['Python','JavaScript','PHP','C++','Java','Scala','React','Ruby','Angular','PHP','Python','JavaScript'])
|
||||
}
|
||||
|
||||
#创建一个数据框
|
||||
df = pd.DataFrame(d)
|
||||
print(df)
|
||||
```
|
||||
|
||||
### Matplotlib
|
||||
|
||||
[Matplotlib][4] 也是 Scipy 核心包的一部分,并且在 BSD 许可证下可用。它是一个非常流行的科学库,用于实现简单而强大的可视化。你可以使用这个 Python 数据科学框架来生成曲线图、柱状图、直方图以及各种不同形状的图表,并且不用担心需要写很多行的代码。例如,我们来看一下如何使用 Matplotlib 库来生成一个简单的柱状图。
|
||||
|
||||
首先导入该库:
|
||||
|
||||
```
|
||||
from matplotlib import pyplot as plt
|
||||
```
|
||||
|
||||
然后生成 x 轴和 y 轴的数值:
|
||||
|
||||
```
|
||||
x = [2, 4, 6, 8, 10]
|
||||
y = [10, 11, 6, 7, 4]
|
||||
```
|
||||
|
||||
接下来,调用函数来绘制柱状图:
|
||||
|
||||
```
|
||||
plt.bar(x,y)
|
||||
```
|
||||
|
||||
最后,显示图表:
|
||||
|
||||
```
|
||||
plt.show()
|
||||
```
|
||||
|
||||
柱状图如下:
|
||||
|
||||
![][6]
|
||||
|
||||
下面是这个例子的完整代码:
|
||||
|
||||
```
|
||||
#导入 Matplotlib 库
|
||||
from matplotlib import pyplot as plt
|
||||
#和 import matplotlib.pyplot as plt 一样
|
||||
|
||||
#生成 x 轴的数值
|
||||
x = [2, 4, 6, 8, 10]
|
||||
|
||||
#生成 y 轴的数值
|
||||
y = [10, 11, 6, 7, 4]
|
||||
|
||||
#调用函数来绘制柱状图
|
||||
plt.bar(x,y)
|
||||
|
||||
#显示图表
|
||||
plt.show()
|
||||
```
|
||||
|
||||
### 总结
|
||||
|
||||
Python 编程语言非常擅长数据处理和准备,但是在科学数据分析和建模方面就没有那么优秀了。幸好有这些用于[数据科学][5]的顶级 Python 框架填补了这一空缺,从而你能够进行复杂的数学计算以及创建复杂模型,进而让数据变得更有意义。
|
||||
|
||||
你还知道其它的 Python 数据挖掘库吗?你的使用经验是什么样的?请在下面的评论中和我们分享。
|
||||
|
||||
--------------------------------------------------------------------------------
|
||||
|
||||
via: https://opensource.com/article/18/9/top-3-python-libraries-data-science
|
||||
|
||||
作者:[Dr.Michael J.Garbade][a]
|
||||
选题:[lujun9972](https://github.com/lujun9972)
|
||||
译者:[ucasFL](https://github.com/ucasFL)
|
||||
校对:[校对者ID](https://github.com/校对者ID)
|
||||
|
||||
本文由 [LCTT](https://github.com/LCTT/TranslateProject) 原创编译,[Linux中国](https://linux.cn/) 荣誉推出
|
||||
|
||||
[a]: https://opensource.com/users/drmjg
|
||||
[1]: https://www.liveedu.tv/andreybu/REaxr-machine-learning-model-python-sklearn-kera/oPGdP-machine-learning-model-python-sklearn-kera/
|
||||
[2]: http://www.numpy.org/
|
||||
[3]: http://pandas.pydata.org/
|
||||
[4]: https://matplotlib.org/
|
||||
[5]: https://www.liveedu.tv/guides/data-science/
|
||||
[6]: https://opensource.com/sites/default/files/uploads/matplotlib_barchart.png
|
||||
[7]: https://opensource.com/sites/default/files/styles/image-full-size/public/lead-images/data_metrics_analytics_desktop_laptop.png?itok=9QXd7AUr
|
Loading…
Reference in New Issue
Block a user