mirror of
https://github.com/LCTT/TranslateProject.git
synced 2024-12-26 21:30:55 +08:00
Merge remote-tracking branch 'LCTT/master'
This commit is contained in:
commit
23590588cb
@ -1,40 +1,38 @@
|
||||
Android 9.0 概览
|
||||
======
|
||||
|
||||
> 第九代 Android 带来了更令人满意的用户体验。
|
||||
|
||||

|
||||
|
||||
我们来谈论一下 Android。尽管 Android 只是一款内核经过修改的 Linux,但经过多年的发展,Android 开发者们(或许包括正在阅读这篇文章的你)已经为这个平台的演变做出了很多值得称道的贡献。当然,可能很多人都已经知道,但我们还是要说,Android 并不完全开源,当你使用 Google 服务的时候,就已经接触到闭源的部分了。Google Play 商店就是其中之一,它不是一个开放的服务,不过这与 Android 是否开源没有太直接的联系,而是为了让你享用到美味、营养、高效、省电的馅饼(注:Android 9.0 代号为 Pie)。
|
||||
我们来谈论一下 Android。尽管 Android 只是一款内核经过修改的 Linux,但经过多年的发展,Android 开发者们(或许包括正在阅读这篇文章的你)已经为这个平台的演变做出了很多值得称道的贡献。当然,可能很多人都已经知道,但我们还是要说,Android 并不完全开源,当你使用 Google 服务的时候,就已经接触到闭源的部分了。Google Play 商店就是其中之一,它不是一个开放的服务。不过无论 Android 开源与否,这就是一个美味、营养、高效、省电的馅饼(LCTT 译注:Android 9.0 代号为 Pie)。
|
||||
|
||||
我在我的 Essential PH-1 手机上运行了 Android 9.0(我真的很喜欢这款手机,也很了解这家公司的境况并不好)。在我自己体验了一段时间之后,我认为它是会被大众接受的。那么 Android 9.0 到底好在哪里呢?下面我们就来深入探讨一下。我们的出发点是用户的角度,而不是开发人员的角度,因此我也不会深入探讨太底层的方面。
|
||||
我在我的 Essential PH-1 手机上运行了 Android 9.0(我真的很喜欢这款手机,也知道这家公司的境况并不好)。在我自己体验了一段时间之后,我认为它是会被大众接受的。那么 Android 9.0 到底好在哪里呢?下面我们就来深入探讨一下。我们的出发点是用户的角度,而不是开发人员的角度,因此我也不会深入探讨太底层的方面。
|
||||
|
||||
### 手势操作
|
||||
|
||||
Android 系统在新的手势操作方面投入了很多,但实际体验却不算太好。这个功能确实引起了我的兴趣。在这个功能发布之初,大家都对它了解甚少,纷纷猜测它会不会让用户使用多点触控的手势来浏览 Android 界面?又或者会不会是一个完全颠覆人们认知的东西?
|
||||
|
||||
实际上,手势操作比大多数人设想的要更加微妙和简单,因为很多功能都浓缩到了 Home 键上。打开手势操作功能之后,Recent 键的功能就合并到 Home 键上了。因此,如果需要查看最近打开的应用程序,就不能简单地通过 Recent 键来查看,而应该从 Home 键向上轻扫一下。(图1)
|
||||
实际上,手势操作比大多数人设想的要更加微妙而简单,因为很多功能都浓缩到了 Home 键上。打开手势操作功能之后,Recent 键的功能就合并到 Home 键上了。因此,如果需要查看最近打开的应用程序,就不能简单地通过 Recent 键来查看,而应该从 Home 键向上轻扫一下。(图 1)
|
||||
|
||||
![Android Pie][2]
|
||||
|
||||
图 1:Android 9.0 中的”最近的应用程序“界面。
|
||||
*图 1:Android 9.0 中的”最近的应用程序“界面。*
|
||||
|
||||
另一个不同的地方是 App Drawer。类似于查看最近打开的应用,需要在 Home 键向上滑动才能打开 App Drawer。
|
||||
|
||||
而后退按钮则没有去掉。在应用程序需要用到后退功能时,它就会出现在屏幕的左下方。有时候即使应用程序自己带有后退按钮,Android 的后退按钮也会出现。
|
||||
而后退按钮则没有去掉。在应用程序需要用到后退功能时,它就会出现在主屏幕的左下方。有时候即使应用程序自己带有后退按钮,Android 的后退按钮也会出现。
|
||||
|
||||
当然,如果你不喜欢使用手势操作,也可以禁用这个功能。只需要按照下列步骤操作:
|
||||
|
||||
|
||||
1. 打开”设置“
|
||||
|
||||
2. 向下滑动并进入 系统 > 手势
|
||||
|
||||
2. 向下滑动并进入“系统 > 手势”
|
||||
3. 从 Home 键向上滑动
|
||||
|
||||
4. 将 On/Off 滑块(图2)滑动至 Off 位置
|
||||
4. 将 On/Off 滑块(图 2)滑动至 Off 位置
|
||||
|
||||

|
||||
|
||||
图 2:关闭手势操作。
|
||||
*图 2:关闭手势操作。*
|
||||
|
||||
### 电池寿命
|
||||
|
||||
@ -42,25 +40,19 @@ Android 系统在新的手势操作方面投入了很多,但实际体验却不
|
||||
|
||||
对于这个功能的唯一一个警告是,如果人工智能出现问题并导致电池电量过早耗尽,就只能通过恢复出厂设置来解决这个问题了。尽管有这样的缺陷,在电池续航时间方面,Android 9.0 也比 Android 8.0 有所改善。
|
||||
|
||||
### 分屏功能
|
||||
### 分屏功能的变化
|
||||
|
||||
分屏对于 Android 来说不是一个新功能,但在 Android 9.0 上,它的使用方式和以往相比略有不同,而且只对于手势操作有影响,不使用手势操作的用户不受影响。要在 Android 9.0 上使用分屏功能,需要按照下列步骤操作:
|
||||
|
||||
1. 从 Home 键向上滑动,打开“最近的应用程序”。
|
||||
2. 找到需要放置在屏幕顶部的应用程序。
|
||||
3. 长按应用程序顶部的图标以显示新的弹出菜单。(图 3)
|
||||
4. 点击分屏,应用程序会在屏幕的上半部分打开。
|
||||
5. 找到要打开的第二个应用程序,然后点击它添加到屏幕的下半部分。
|
||||
|
||||
![Adding an app][5]
|
||||
|
||||
图 3:在 Android 9.0 上将应用添加到分屏模式中。
|
||||
|
||||
[Used with permission][3]
|
||||
|
||||
1. 从 Home 键向上滑动,打开“最近的应用程序”。
|
||||
|
||||
2. 找到需要放置在屏幕顶部的应用程序。
|
||||
|
||||
3. 长按应用程序顶部的图标以显示新的弹出菜单。(图 3)
|
||||
|
||||
4. 点击分屏,应用程序会在屏幕的上半部分打开。
|
||||
|
||||
5. 找到要打开的第二个应用程序,然后点击它添加到屏幕的下半部分。
|
||||
*图 3:在 Android 9.0 上将应用添加到分屏模式中。*
|
||||
|
||||
使用分屏功能关闭应用程序的方法和原来保持一致。
|
||||
|
||||
@ -72,7 +64,7 @@ Android 系统在新的手势操作方面投入了很多,但实际体验却不
|
||||
|
||||
![Actions][7]
|
||||
|
||||
图 4:Android 应用操作。
|
||||
*图 4:Android 应用操作。*
|
||||
|
||||
### 声音控制
|
||||
|
||||
@ -82,17 +74,17 @@ Android 9.0 这次优化针对的是设备上快速控制声音的按钮。如
|
||||
|
||||
![Sound control][9]
|
||||
|
||||
图 5:Android 9.0 上的声音控制。
|
||||
*图 5:Android 9.0 上的声音控制。*
|
||||
|
||||
### 屏幕截图
|
||||
|
||||
由于我要撰写关于 Android 的文章,所以我会常常需要进行屏幕截图。而 Android 9.0 有意向我最喜欢的更新,就是分享屏幕截图。Android 9.0 可以在截取屏幕截图后,直接共享、编辑,或者删除不喜欢的截图,而不需要像以前一样打开 Google 相册、找到要共享的屏幕截图、打开图像然后共享图像。
|
||||
由于我要撰写关于 Android 的文章,所以我会常常需要进行屏幕截图。而 Android 9.0 有一项我最喜欢的更新,就是分享屏幕截图。Android 9.0 可以在截取屏幕截图后,直接共享、编辑,或者删除不喜欢的截图,而不需要像以前一样打开 Google 相册、找到要共享的屏幕截图、打开图像然后共享图像。
|
||||
|
||||
如果你想分享屏幕截图,只需要在截图后等待弹出菜单,点击分享(图 6),从标准的 Android 分享菜单中分享即可。
|
||||
|
||||
![Sharing ][11]
|
||||
|
||||
图 6:共享屏幕截图变得更加容易。
|
||||
|
||||
如果你想分享屏幕截图,只需要在截图后等待弹出菜单,点击分享(图 6),从标准的 Android 分享菜单中分享即可。
|
||||
*图 6:共享屏幕截图变得更加容易。*
|
||||
|
||||
### 更令人满意的 Android 体验
|
||||
|
||||
@ -105,7 +97,7 @@ via: https://www.linux.com/learn/2018/10/overview-android-pie
|
||||
作者:[Jack Wallen][a]
|
||||
选题:[lujun9972][b]
|
||||
译者:[HankChow](https://github.com/HankChow)
|
||||
校对:[校对者ID](https://github.com/校对者ID)
|
||||
校对:[wxy](https://github.com/wxy)
|
||||
|
||||
本文由 [LCTT](https://github.com/LCTT/TranslateProject) 原创编译,[Linux中国](https://linux.cn/) 荣誉推出
|
||||
|
@ -1,43 +0,0 @@
|
||||
translating---geekpi
|
||||
|
||||
Gathering project requirements using the Open Decision Framework
|
||||
======
|
||||
|
||||
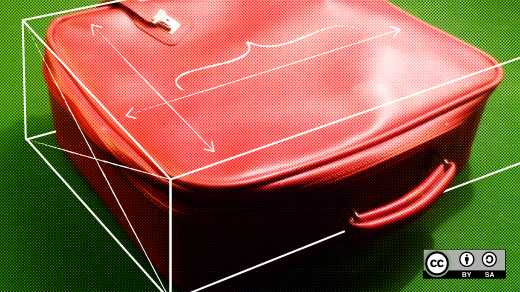
|
||||
|
||||
It's no secret that clear, concise, and measurable requirements lead to more successful projects. A study about large scale projects by [McKinsey & Company in conjunction with the University of Oxford][1] revealed that "on average, large IT projects run 45 percent over budget and 7 percent over time, while delivering 56 percent less value than predicted." The research also showed that some of the causes for this failure were "fuzzy business objectives, out-of-sync stakeholders, and excessive rework."
|
||||
|
||||
Business analysts often find themselves constructing these requirements through ongoing conversations. To do this, they must engage multiple stakeholders and ensure that engaged participants provide clear business objectives. This leads to less rework and more projects with a higher rate of success.
|
||||
|
||||
And they can do it in an open and inclusive way.
|
||||
|
||||
### A framework for success
|
||||
|
||||
One tool for increasing project success rate is the [Open Decision Framework][2]. The Open Decision Framework is an resource that can help users make more effective decisions in organizations that embrace [open principles][3]. The framework stresses three primary principles: being transparent, being inclusive, and being customer-centric.
|
||||
|
||||
**Transparent**. Many times, developers and product designers assume they know how stakeholders use a particular tool or piece of software. But these assumptions are often incorrect and lead to misconceptions about what stakeholders actually need. Practicing transparency when having discussions with developers and business owners is imperative. Development teams need to see not only the "sunny day" scenario but also the challenges that stakeholders face with certain tools or processes. Ask questions such as: "What steps must be done manually?" and "Is this tool performing as you expect?" This provides a shared understanding of the problem and a common baseline for discussion.
|
||||
|
||||
|
||||
**Inclusive**. It is vitally important for business analysts to look at body language and visual cues when gathering requirements. If someone is sitting with arms crossed or rolling their eyes, then it's a clear indication that they do not feel heard. A BA must encourage open communication by reaching out to those that don't feel heard and giving them the opportunity to be heard. Prior to starting the session, lay down ground rules that make the place safe for all to speak their opinions and to share their thoughts. Listen to the feedback provided and respond politely when feedback is offered. Diverse opinions and collaborative problem solving will bring exciting ideas to the session.
|
||||
|
||||
**Customer-centric**. The first step to being customer-centric is to recognize the customer. Who is benefiting from this change, update, or development? Early in the project, conduct a stakeholder mapping to help determine the key stakeholders, their roles in the project, and the ways they fit into the big picture. Involving the right customers and assuring that their needs are met will lead to more successful requirements being identified, more realistic (real-life) tests being conducted, and, ultimately, a successful delivery.
|
||||
|
||||
When your requirement sessions are transparent, inclusive, and customer-centric, you'll gather better requirements. And when you use the [Open Decision Framework][4] for running those sessions, participants feel more involved and empowered, and they deliver more accurate and complete requirements. In other words:
|
||||
|
||||
**Transparent + Inclusive + Customer-Centric = Better Requirements = Successful Projects**
|
||||
|
||||
--------------------------------------------------------------------------------
|
||||
|
||||
via: https://opensource.com/open-organization/18/2/constructing-project-requirements
|
||||
|
||||
作者:[Tracy Buckner][a]
|
||||
译者:[译者ID](https://github.com/译者ID)
|
||||
校对:[校对者ID](https://github.com/校对者ID)
|
||||
|
||||
本文由 [LCTT](https://github.com/LCTT/TranslateProject) 原创编译,[Linux中国](https://linux.cn/) 荣誉推出
|
||||
|
||||
[a]:https://opensource.com/users/tracyb
|
||||
[1]:http://calleam.com/WTPF/?page_id=1445
|
||||
[2]:https://opensource.com/open-organization/resources/open-decision-framework
|
||||
[3]:https://opensource.com/open-organization/resources/open-org-definition
|
||||
[4]:https://opensource.com/open-organization/16/6/introducing-open-decision-framework
|
@ -1,3 +1,12 @@
|
||||
[#]: collector: (lujun9972)
|
||||
[#]: translator: (geekpi)
|
||||
[#]: reviewer: ( )
|
||||
[#]: publisher: ( )
|
||||
[#]: subject: (How a university network assistant used Linux in the 90s)
|
||||
[#]: via: (https://opensource.com/article/18/5/my-linux-story-student)
|
||||
[#]: author: ([Alan Formy-Duva](https://opensource.com/users/alanfdoss)
|
||||
[#]: url: ( )
|
||||
|
||||
How a university network assistant used Linux in the 90s
|
||||
======
|
||||
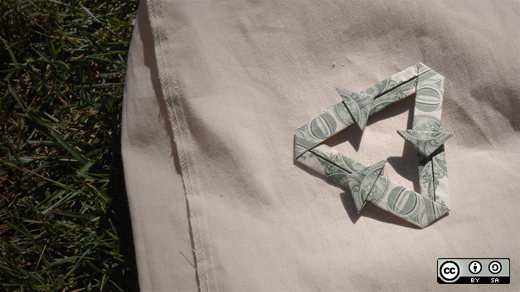
|
||||
|
@ -1,4 +1,3 @@
|
||||
Translating by DavidChenLiang
|
||||
Python
|
||||
============================================================
|
||||
|
||||
|
@ -1,5 +1,3 @@
|
||||
translating by cyleft
|
||||
|
||||
Taking notes with Laverna, a web-based information organizer
|
||||
======
|
||||
|
||||
|
@ -1,153 +0,0 @@
|
||||
How to write your favorite R functions in Python
|
||||
======
|
||||
R or Python? This Python script mimics convenient R-style functions for doing statistics nice and easy.
|
||||
|
||||
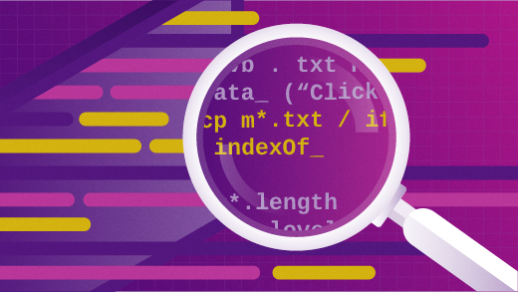
|
||||
|
||||
One of the great modern battles of data science and machine learning is "Python vs. R." There is no doubt that both have gained enormous ground in recent years to become top programming languages for data science, predictive analytics, and machine learning. In fact, according to a recent IEEE article, Python overtook C++ as the [top programming language][1] and R firmly secured its spot in the top 10.
|
||||
|
||||
However, there are some fundamental differences between these two. [R was developed primarily][2] as a tool for statistical analysis and quick prototyping of a data analysis problem. Python, on the other hand, was developed as a general purpose, modern object-oriented language in the same vein as C++ or Java but with a simpler learning curve and more flexible demeanor. Consequently, R continues to be extremely popular among statisticians, quantitative biologists, physicists, and economists, whereas Python has slowly emerged as the top language for day-to-day scripting, automation, backend web development, analytics, and general machine learning frameworks and has an extensive support base and open source development community work.
|
||||
|
||||
### Mimicking functional programming in a Python environment
|
||||
|
||||
[R's nature as a functional programming language][3] provides users with an extremely simple and compact interface for quick calculations of probabilities and essential descriptive/inferential statistics for a data analysis problem. For example, wouldn't it be great to be able to solve the following problems with just a single, compact function call?
|
||||
|
||||
* How to calculate the mean/median/mode of a data vector.
|
||||
* How to calculate the cumulative probability of some event following a normal distribution. What if the distribution is Poisson?
|
||||
* How to calculate the inter-quartile range of a series of data points.
|
||||
* How to generate a few random numbers following a Student's t-distribution.
|
||||
|
||||
|
||||
|
||||
The R programming environment can do all of these.
|
||||
|
||||
On the other hand, Python's scripting ability allows analysts to use those statistics in a wide variety of analytics pipelines with limitless sophistication and creativity.
|
||||
|
||||
To combine the advantages of both worlds, you just need a simple Python-based wrapper library that contains the most commonly used functions pertaining to probability distributions and descriptive statistics defined in R-style. This enables you to call those functions really fast without having to go to the proper Python statistical libraries and figure out the whole list of methods and arguments.
|
||||
|
||||
### Python wrapper script for most convenient R-functions
|
||||
|
||||
[I wrote a Python script][4] to define the most convenient and widely used R-functions in simple, statistical analysis—in Python. After importing this script, you will be able to use those R-functions naturally, just like in an R programming environment.
|
||||
|
||||
The goal of this script is to provide simple Python subroutines mimicking R-style statistical functions for quickly calculating density/point estimates, cumulative distributions, and quantiles and generating random variates for important probability distributions.
|
||||
|
||||
To maintain the spirit of R styling, the script uses no class hierarchy and only raw functions are defined in the file. Therefore, a user can import this one Python script and use all the functions whenever they're needed with a single name call.
|
||||
|
||||
Note that I use the word mimic. Under no circumstance am I claiming to emulate R's true functional programming paradigm, which consists of a deep environmental setup and complex relationships between those environments and objects. This script allows me (and I hope countless other Python users) to quickly fire up a Python program or Jupyter notebook, import the script, and start doing simple descriptive statistics in no time. That's the goal, nothing more, nothing less.
|
||||
|
||||
If you've coded in R (maybe in grad school) and are just starting to learn and use Python for data analysis, you will be happy to see and use some of the same well-known functions in your Jupyter notebook in a manner similar to how you use them in your R environment.
|
||||
|
||||
Whatever your reason, using this script is fun.
|
||||
|
||||
### Simple examples
|
||||
|
||||
To start, just import the script and start working with lists of numbers as if they were data vectors in R.
|
||||
|
||||
```
|
||||
from R_functions import *
|
||||
lst=[20,12,16,32,27,65,44,45,22,18]
|
||||
<more code, more statistics...>
|
||||
```
|
||||
|
||||
Say you want to calculate the [Tuckey five-number][5] summary from a vector of data points. You just call one simple function, **fivenum** , and pass on the vector. It will return the five-number summary in a NumPy array.
|
||||
|
||||
```
|
||||
lst=[20,12,16,32,27,65,44,45,22,18]
|
||||
fivenum(lst)
|
||||
> array([12. , 18.5, 24.5, 41. , 65. ])
|
||||
```
|
||||
|
||||
Maybe you want to know the answer to the following question:
|
||||
|
||||
Suppose a machine outputs 10 finished goods per hour on average with a standard deviation of 2. The output pattern follows a near normal distribution. What is the probability that the machine will output at least 7 but no more than 12 units in the next hour?
|
||||
|
||||
The answer is essentially this:
|
||||
|
||||
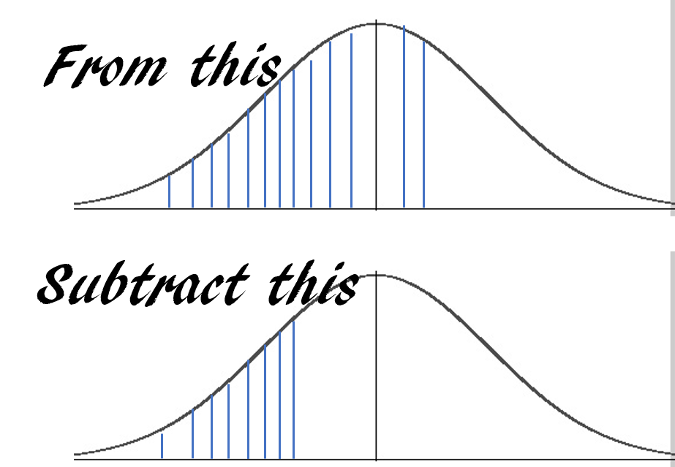
|
||||
|
||||
You can obtain the answer with just one line of code using **pnorm** :
|
||||
|
||||
```
|
||||
pnorm(12,10,2)-pnorm(7,10,2)
|
||||
> 0.7745375447996848
|
||||
```
|
||||
|
||||
Or maybe you need to answer the following:
|
||||
|
||||
Suppose you have a loaded coin with the probability of turning heads up 60% every time you toss it. You are playing a game of 10 tosses. How do you plot and map out the chances of all the possible number of wins (from 0 to 10) with this coin?
|
||||
|
||||
You can obtain a nice bar chart with just a few lines of code using just one function, **dbinom** :
|
||||
|
||||
```
|
||||
probs=[]
|
||||
import matplotlib.pyplot as plt
|
||||
for i in range(11):
|
||||
probs.append(dbinom(i,10,0.6))
|
||||
plt.bar(range(11),height=probs)
|
||||
plt.grid(True)
|
||||
plt.show()
|
||||
```
|
||||
|
||||
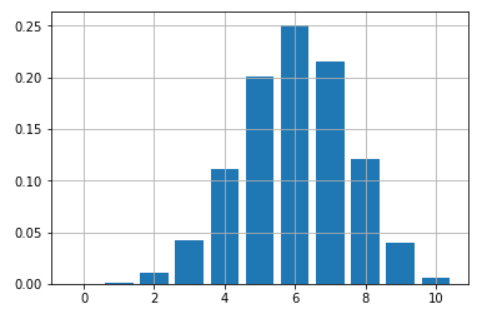
|
||||
|
||||
### Simple interface for probability calculations
|
||||
|
||||
R offers an extremely simple and intuitive interface for quick calculations from essential probability distributions. The interface goes like this:
|
||||
|
||||
* **d** {distribution} gives the density function value at a point **x**
|
||||
* **p** {distribution} gives the cumulative value at a point **x**
|
||||
* **q** {distribution} gives the quantile function value at a probability **p**
|
||||
* **r** {distribution} generates one or multiple random variates
|
||||
|
||||
|
||||
|
||||
In our implementation, we stick to this interface and its associated argument list so you can execute these functions exactly like you would in an R environment.
|
||||
|
||||
### Currently implemented functions
|
||||
|
||||
The following R-style functions are implemented in the script for fast calling.
|
||||
|
||||
* Mean, median, variance, standard deviation
|
||||
* Tuckey five-number summary, IQR
|
||||
* Covariance of a matrix or between two vectors
|
||||
* Density, cumulative probability, quantile function, and random variate generation for the following distributions: normal, uniform, binomial, Poisson, F, Student's t, Chi-square, beta, and gamma.
|
||||
|
||||
|
||||
|
||||
### Work in progress
|
||||
|
||||
Obviously, this is a work in progress, and I plan to add some other convenient R-functions to this script. For example, in R, a single line of command **lm** can get you an ordinary least-square fitted model to a numerical dataset with all the necessary inferential statistics (P-values, standard error, etc.). This is powerfully brief and compact! On the other hand, standard linear regression problems in Python are often tackled using [Scikit-learn][6], which needs a bit more scripting for this use, so I plan to incorporate this single function linear model fitting feature using Python's [statsmodels][7] backend.
|
||||
|
||||
If you like and use this script in your work, please help others find it by starring or forking its [GitHub repository][8]. Also, you can check my other [GitHub repos][9] for fun code snippets in Python, R, or MATLAB and some machine learning resources.
|
||||
|
||||
If you have any questions or ideas to share, please contact me at [tirthajyoti[AT]gmail.com][10]. If you are, like me, passionate about machine learning and data science, please [add me on LinkedIn][11] or [follow me on Twitter. ][12]
|
||||
|
||||
Originally published on [Towards Data Science][13]. Reposted under [CC BY-SA 4.0][14].
|
||||
|
||||
--------------------------------------------------------------------------------
|
||||
|
||||
via: https://opensource.com/article/18/10/write-favorite-r-functions-python
|
||||
|
||||
作者:[Tirthajyoti Sarkar][a]
|
||||
选题:[lujun9972][b]
|
||||
译者:[译者ID](https://github.com/译者ID)
|
||||
校对:[校对者ID](https://github.com/校对者ID)
|
||||
|
||||
本文由 [LCTT](https://github.com/LCTT/TranslateProject) 原创编译,[Linux中国](https://linux.cn/) 荣誉推出
|
||||
|
||||
[a]: https://opensource.com/users/tirthajyoti
|
||||
[b]: https://github.com/lujun9972
|
||||
[1]: https://spectrum.ieee.org/at-work/innovation/the-2018-top-programming-languages
|
||||
[2]: https://www.coursera.org/lecture/r-programming/overview-and-history-of-r-pAbaE
|
||||
[3]: http://adv-r.had.co.nz/Functional-programming.html
|
||||
[4]: https://github.com/tirthajyoti/StatsUsingPython/blob/master/R_Functions.py
|
||||
[5]: https://en.wikipedia.org/wiki/Five-number_summary
|
||||
[6]: http://scikit-learn.org/stable/
|
||||
[7]: https://www.statsmodels.org/stable/index.html
|
||||
[8]: https://github.com/tirthajyoti/StatsUsingPython
|
||||
[9]: https://github.com/tirthajyoti?tab=repositories
|
||||
[10]: mailto:tirthajyoti@gmail.com
|
||||
[11]: https://www.linkedin.com/in/tirthajyoti-sarkar-2127aa7/
|
||||
[12]: https://twitter.com/tirthajyotiS
|
||||
[13]: https://towardsdatascience.com/how-to-write-your-favorite-r-functions-in-python-11e1e9c29089
|
||||
[14]: https://creativecommons.org/licenses/by-sa/4.0/
|
@ -1,144 +0,0 @@
|
||||
[#]: collector: (lujun9972)
|
||||
[#]: translator: (chenxinlong)
|
||||
[#]: reviewer: ( )
|
||||
[#]: publisher: ( )
|
||||
[#]: subject: (How To Configure IP Address In Ubuntu 18.04 LTS)
|
||||
[#]: via: (https://www.ostechnix.com/how-to-configure-ip-address-in-ubuntu-18-04-lts/)
|
||||
[#]: author: (SK https://www.ostechnix.com/author/sk/)
|
||||
[#]: url: ( )
|
||||
|
||||
翻译中 ...
|
||||
|
||||
How To Configure IP Address In Ubuntu 18.04 LTS
|
||||
======
|
||||
|
||||
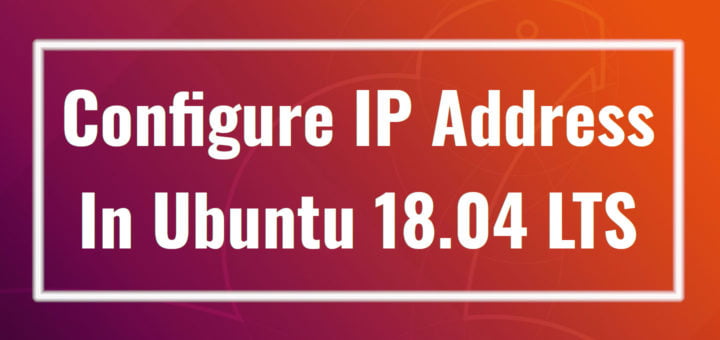
|
||||
|
||||
The method of configuring IP address on Ubuntu 18.04 LTS is significantly different than the older methods. Unlike the previous versions, the Ubuntu 18.04 uses **Netplan** , a new command line network configuration utility, to configure IP address. Netplan has been introduced by Ubuntu developers in Ubuntu 17.10. In this new approach, we no longer use **/etc/network/interfaces** file to configure IP address rather we use a YAML file. The default configuration files of Netplan are found under **/etc/netplan/** directory. In this brief tutorial, we are going to learn to configure static and dynamic IP address in **Ubuntu 18.04 LTS** minimal server.
|
||||
|
||||
### Configure Static IP Address In Ubuntu 18.04 LTS
|
||||
|
||||
Let us find out the default network configuration file:
|
||||
|
||||
```
|
||||
$ ls /etc/netplan/
|
||||
50-cloud-init.yaml
|
||||
```
|
||||
|
||||
As you can see, the default network configuration file is **50-cloud-init.yaml** and it is obviously a YAML file.
|
||||
|
||||
Now, let check the contents of this file:
|
||||
|
||||
```
|
||||
$ cat /etc/netplan/50-cloud-init.yaml
|
||||
```
|
||||
|
||||
I have configured my network card to obtain IP address from the DHCP server when I am installing Ubuntu 18.04, so here is my network configuration details:
|
||||
|
||||
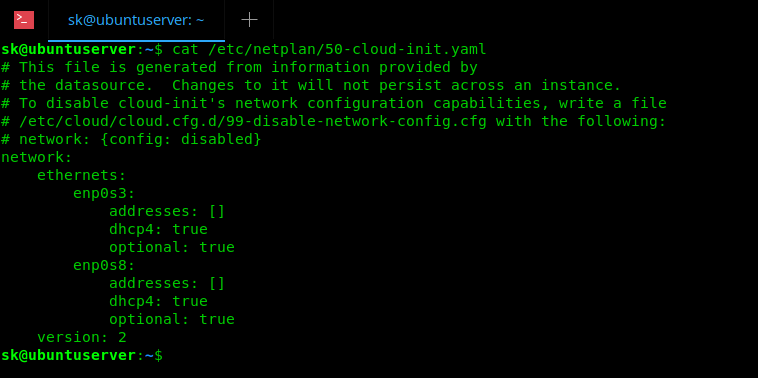
|
||||
|
||||
As you can see, I have two network cards, namely **enp0s3** and **enp0s8** , and both are configured to accept IPs from the DHCP server.
|
||||
|
||||
Let us now configure static IP addresses to both network cards.
|
||||
|
||||
To do so, open the default network configuration file in any editor of your choice.
|
||||
|
||||
```
|
||||
$ sudo nano /etc/netplan/50-cloud-init.yaml
|
||||
```
|
||||
|
||||
Now, update the file by adding the IP address, netmask, gateway and DNS server. For the purpose of this file, I have used **192.168.225.50** as my IP for **enp0s3** and **192.168.225.51** for **enp0s8** , **192.168.225.1** as gateway, **255.255.255.0** as netwmask and **8.8.8.8** , **8.8.4.4** as DNS servers.
|
||||
|
||||
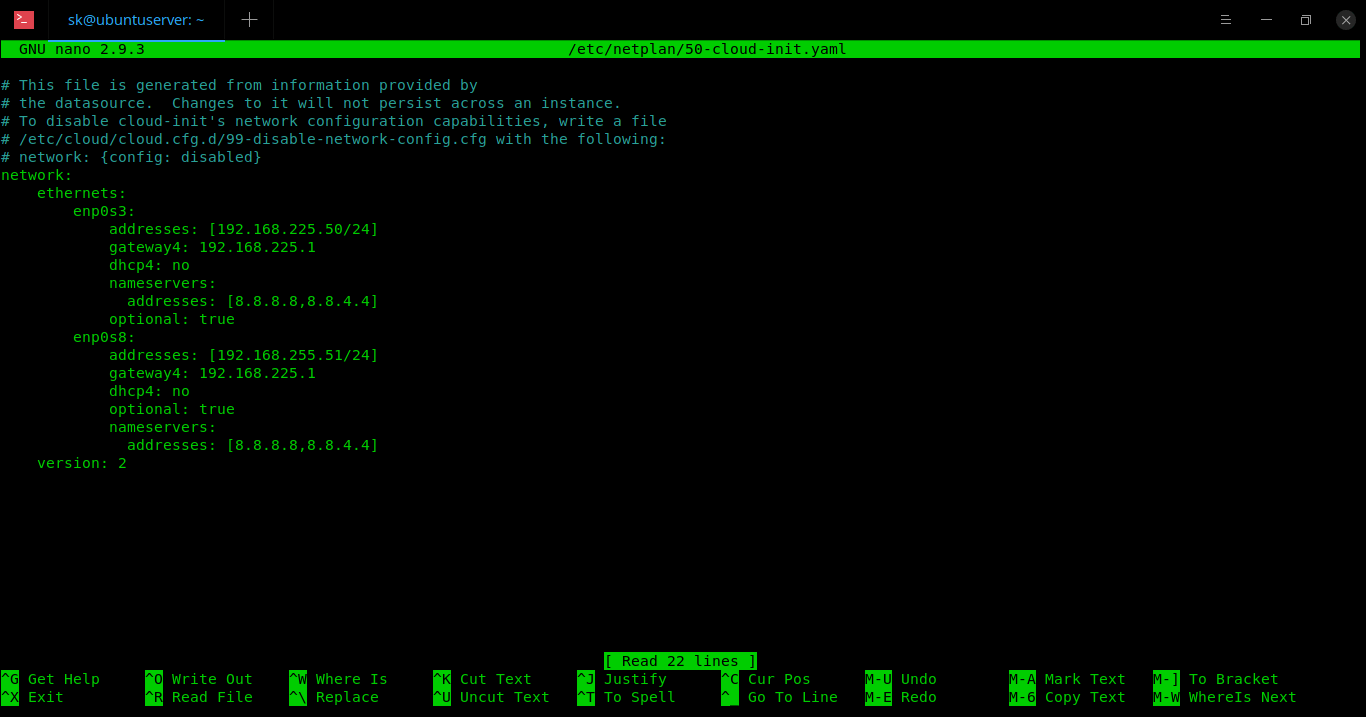
|
||||
|
||||
Please mind the space between the lines. Don’t use **TAB** to align the lines as it will not work in Ubuntu 18.04. Instead, just use SPACEBAR key to make them in a consistent order as shown in the above picture.
|
||||
|
||||
Also, we don’t use a separate line to define netmask (255.255.255.0) in Ubuntu 18.04. For instance, in older Ubuntu versions, we configure IP and netmask like below:
|
||||
|
||||
```
|
||||
address = 192.168.225.50
|
||||
netmask = 255.255.255.0
|
||||
```
|
||||
|
||||
However, with netplan, we combine those two lines with a single line as shown below:
|
||||
|
||||
```
|
||||
addresses : [192.168.225.50/24]
|
||||
```
|
||||
|
||||
Once you’re done, Save and close the file.
|
||||
|
||||
Apply the network configuration using command:
|
||||
|
||||
```
|
||||
$ sudo netplan apply
|
||||
```
|
||||
|
||||
If there are any issues, run the following command to investigate and check what is the problem in the configuration.
|
||||
|
||||
```
|
||||
$ sudo netplan --debug apply
|
||||
```
|
||||
|
||||
Output:
|
||||
|
||||
```
|
||||
** (generate:1556): DEBUG: 09:14:47.220: Processing input file //etc/netplan/50-cloud-init.yaml..
|
||||
** (generate:1556): DEBUG: 09:14:47.221: starting new processing pass
|
||||
** (generate:1556): DEBUG: 09:14:47.221: enp0s8: setting default backend to 1
|
||||
** (generate:1556): DEBUG: 09:14:47.222: enp0s3: setting default backend to 1
|
||||
** (generate:1556): DEBUG: 09:14:47.222: Generating output files..
|
||||
** (generate:1556): DEBUG: 09:14:47.223: NetworkManager: definition enp0s8 is not for us (backend 1)
|
||||
** (generate:1556): DEBUG: 09:14:47.223: NetworkManager: definition enp0s3 is not for us (backend 1)
|
||||
DEBUG:netplan generated networkd configuration exists, restarting networkd
|
||||
DEBUG:no netplan generated NM configuration exists
|
||||
DEBUG:device enp0s3 operstate is up, not replugging
|
||||
DEBUG:netplan triggering .link rules for enp0s3
|
||||
DEBUG:device lo operstate is unknown, not replugging
|
||||
DEBUG:netplan triggering .link rules for lo
|
||||
DEBUG:device enp0s8 operstate is up, not replugging
|
||||
DEBUG:netplan triggering .link rules for enp0s8
|
||||
```
|
||||
|
||||
Now, let us check the Ip address using command:
|
||||
|
||||
```
|
||||
$ ip addr
|
||||
```
|
||||
|
||||
Sample output from my Ubuntu 18.04 LTS:
|
||||
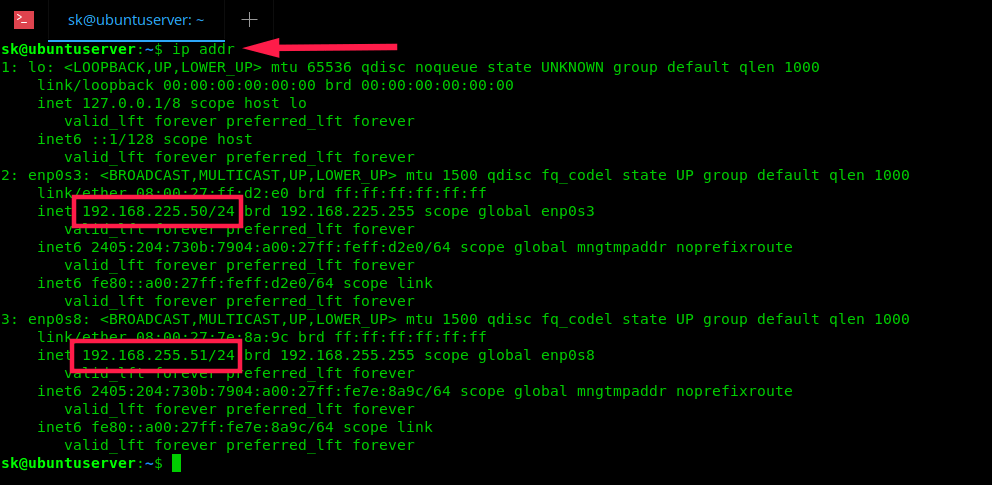
|
||||
|
||||
Congratulations! We have successfully configured static IP address in Ubuntu 18.04 LTS with Netplan configuration tool.
|
||||
|
||||
For more details, refer the Netplan man pages.
|
||||
|
||||
```
|
||||
$ man netplan
|
||||
```
|
||||
|
||||
### Configure Dynamic IP Address In Ubuntu 18.04 LTS
|
||||
|
||||
To configure dynamic address, just leave the default configuration file as the way it is. If you already have configured static IP address, just remove the newly added lines and make the YAML file look like exactly as shown in the **figure 1** in the previous section.
|
||||
|
||||
That’s all. You know now how to configure static and dynamic IP in Ubuntu 18.04 LTS server. Personally, I don’t like this new method. The old method is much easier and better. How about you? Did you find it easy or hard? Let me know in the comment section below.
|
||||
|
||||
More good stuffs to come. Stay tuned!
|
||||
|
||||
Cheers!
|
||||
|
||||
|
||||
|
||||
--------------------------------------------------------------------------------
|
||||
|
||||
via: https://www.ostechnix.com/how-to-configure-ip-address-in-ubuntu-18-04-lts/
|
||||
|
||||
作者:[SK][a]
|
||||
选题:[lujun9972][b]
|
||||
译者:[译者ID](https://github.com/译者ID)
|
||||
校对:[校对者ID](https://github.com/校对者ID)
|
||||
|
||||
本文由 [LCTT](https://github.com/LCTT/TranslateProject) 原创编译,[Linux中国](https://linux.cn/) 荣誉推出
|
||||
|
||||
[a]: https://www.ostechnix.com/author/sk/
|
||||
[b]: https://github.com/lujun9972
|
@ -0,0 +1,40 @@
|
||||
使用开放决策框架收集项目需求
|
||||
======
|
||||
|
||||
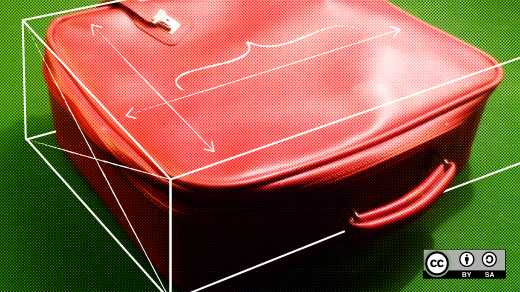
|
||||
|
||||
众所周知,明确、简洁和可衡量的需求会带来更多成功的项目。一项关于[麦肯锡与牛津大学][1]的大型项目的研究表明:“平均而言,大型 IT 项目超出预算 45%,时间每推移 7%,价值就比预期低 56% “。该研究还表明,造成这种失败的一些原因是“模糊的业务目标,不同步的利益相关者以及过度的返工”。
|
||||
|
||||
业务分析师经常发现自己通过持续对话来构建这些需求。为此,他们必须吸引多个利益相关方,并确保参与者提供明确的业务目标。这样可以减少返工,提高更多项目的成功率。
|
||||
|
||||
他们可以用开放和包容的方式做到这一点。
|
||||
|
||||
### 成功的框架
|
||||
|
||||
提高项目成功率的一个工具是[开放决策框架][2]。开放决策框架是一种资源,可以帮助用户在拥抱[开放原则][3]的组织中做出更有效的决策。该框架强调三个主要原则:透明、包容、以客户为中心。
|
||||
|
||||
**透明**。很多时候,开发人员和产品设计人员都认为他们知道利益相关者如何使用特定工具或软件。但这些假设往往是不正确的,并导致对利益相关者实际需求的误解。开发人员和企业主讨论时实行透明势在必行。开发团队不仅需要了解一些好的情景,还需要了解利益相关方面对某些工具或流程所面临的挑战。提出诸如以下问题:“必须手动完成哪些步骤?”以及“这个工具是否按预期运行?”这提供了对问题的共同理解和讨论的基准。
|
||||
|
||||
**包容**。对于业务分析师来说,在收集需求时观察肢体语言和视觉暗示非常重要。如果有人双臂交叉或睁着眼睛坐着,那么这清楚地表明他们感觉没被聆听。业务分析师必须鼓励那些不被聆听的人公开交流,并给他们机会被聆听。在开始会议之前,制定基本规则,使所有人都能发表意见并分享他们的想法。聆听提供的反馈,并在收到反馈时礼貌地回复。多样化的意见和协作解决问题将为会议带来令人兴奋的想法。
|
||||
|
||||
**以顾客为中心**。以客户为中心的第一步是识别客户。谁从这种变化、更新或开发中受益?在项目早期,进行利益相关者映射,以帮助确定关键利益相关者,他们在项目中的角色以及他们适应大局的方式。让合适的客户参与并确保满足他们的需求将会确定更成功的要求,进行更现实(现实)的测试,并最终成功交付。
|
||||
|
||||
当你的需求会议透明、包容和以客户为中心时,你将收集更好的需求。当你使用[开放决策框架][4]来进行会议时,参与者会感觉有更多参与与授权,他们会提供更准确和完整的需求。换一种说法:
|
||||
|
||||
**透明+包容+以客户为中心=更好的需求=成功的项目**
|
||||
|
||||
--------------------------------------------------------------------------------
|
||||
|
||||
via: https://opensource.com/open-organization/18/2/constructing-project-requirements
|
||||
|
||||
作者:[Tracy Buckner][a]
|
||||
译者:[geekpi](https://github.com/geekpi)
|
||||
校对:[校对者ID](https://github.com/校对者ID)
|
||||
|
||||
本文由 [LCTT](https://github.com/LCTT/TranslateProject) 原创编译,[Linux中国](https://linux.cn/) 荣誉推出
|
||||
|
||||
[a]:https://opensource.com/users/tracyb
|
||||
[1]:http://calleam.com/WTPF/?page_id=1445
|
||||
[2]:https://opensource.com/open-organization/resources/open-decision-framework
|
||||
[3]:https://opensource.com/open-organization/resources/open-org-definition
|
||||
[4]:https://opensource.com/open-organization/16/6/introducing-open-decision-framework
|
@ -0,0 +1,147 @@
|
||||
如何在 Python 中写你喜爱的 R 函数
|
||||
======
|
||||
R 还是 Python ? 这个 Python 脚本模仿方便的 R 风格函数,使统计数据变得简单易行。
|
||||
|
||||
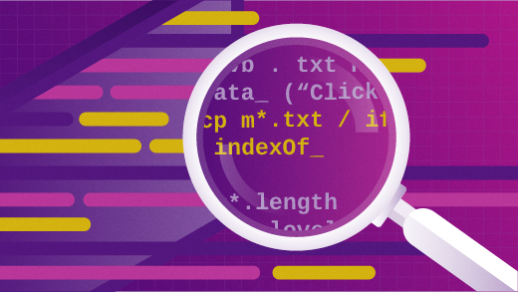
|
||||
|
||||
“Python vs. R” 是数据科学和机器学习的现代战争之一。毫无疑问,近年来两者发展迅猛并成为数据科学,预测分析和机器学习领域的顶级编程语言。事实上,根据 IEEE 最近一篇文章,Python 已在 [最受欢迎编程语言排行榜][1] 中超越 C++ 并且 R 语言也稳居前 10 位。
|
||||
|
||||
但是,这两者之间存在一些根本区别。[R] 主要作为统计分析和数据分析问题的快速原型设计的工具而开发的。另一方面,Python 开发为一种通用的,现代的面向对象语言,类似 C++ 或 Java,但具有更简单的学习曲线和更灵活的语言风格。因此,R 仍在统计学家,定量生物学家,物理学家和经济学家中备受青睐,而 Python 已逐渐成为日常脚本,自动化,后端Web开发,分析和通用机器学习框架的顶级语言并拥有广泛的支持基础和开源开发社区。
|
||||
|
||||
###在 Python 环境中模仿函数式编程
|
||||
|
||||
[R] 作为函数式编程语言的天性为用户提供了一个极其简单和紧凑的借口,用于快速计算概率和数据分析问题的基本描述/推论统计。例如,只用一个紧凑的函数调用来解决以下问题难道不是很好吗?
|
||||
|
||||
* 如何计算数据向量的均值 / 中值 / 众数。
|
||||
* 如何计算某些服从正态分布事件的累积概率。如果服从 Poisson 分布会怎样?
|
||||
* 如何计算一系列数据点的四分差。
|
||||
* 如何生成服从学生 t 分布的一些随机数。
|
||||
|
||||
R编程环境可以完成所有这些工作。
|
||||
|
||||
另一方面,Python的脚本编写能力使分析师能够在各种分析流程中使用这些统计数据,具有无限的复杂性和创造力。
|
||||
|
||||
要结合二者的优势,您只需要一个简单的基于 Python 的包装器库,其中包含与 R 风格定义的概率分布和描述性统计相关的最常用函数。 这使您可以非常快速地调用这些函数,而无需转到正确的 Python 统计库并理解整个方法和参数列表。
|
||||
|
||||
|
||||
### 便于调用 R 函数的 Python 包装脚本
|
||||
|
||||
[我编写了一个Python脚本] [4] 在 Python 中定义了简单统计分析中方便广泛使用的 R 函数。
|
||||
|
||||
此脚本的目标是提供简单的 Python 子例程,模仿 R 风格的统计函数,以快速计算密度/点估计,累积分布和分位数,并生成重要概率分布的随机变量。
|
||||
|
||||
为了保持 R 风格的精髓,脚本不使用类层次结构,并且只在文件中定义原始函数。 因此,用户可以导入这个 Python 脚本,并在需要单个名称调用时使用所有功能。
|
||||
|
||||
请注意,我使用 mimic 这个词。 在任何情况下,我都声称要模仿 R 的真正的函数式编程范例,该范式包括深层环境设置以及这些环境和对象之间的复杂关系。 这个脚本允许我(我希望无数其他 Python 用户)快速启动P ython 程序或 Jupyter 笔记本,导入脚本,并立即开始进行简单的描述性统计。 这就是目标,仅此而已。
|
||||
|
||||
如果您已经写过 R 代码(可能在研究生院)并且刚刚开始学习并使用 Python 进行数据分析,那么您将很高兴看到并在 Jupyter 笔记本中以类似在 R 环境中一样使用一些相同的知名函数。
|
||||
|
||||
无论出于何种原因,使用这个脚本很有趣。
|
||||
|
||||
### 简单的例子
|
||||
|
||||
首先,只需导入脚本并开始处理数字列表,就好像它们是 R 中的数据向量一样。
|
||||
|
||||
```
|
||||
from R_functions import *
|
||||
lst=[20,12,16,32,27,65,44,45,22,18]
|
||||
<more code, more statistics...>
|
||||
```
|
||||
|
||||
假设您想从数据点向量计算[Tuckey五数] [5]摘要。 你只需要调用一个简单的函数 ** fivenum **,然后传递向量。 它将返回 NumPy 数组中的五数摘要。
|
||||
|
||||
```
|
||||
lst=[20,12,16,32,27,65,44,45,22,18]
|
||||
fivenum(lst)
|
||||
> array([12. , 18.5, 24.5, 41. , 65. ])
|
||||
```
|
||||
|
||||
或许你想要知道下面问题的解答
|
||||
|
||||
假设一台机器平均每小时输出 10 件成品,标准偏差为 2。输出模式遵循接近正态的分布。 机器在下一个小时内输出至少 7 个但不超过 12 个单位的概率是多少?
|
||||
|
||||
答案基本上是这样的:
|
||||
|
||||
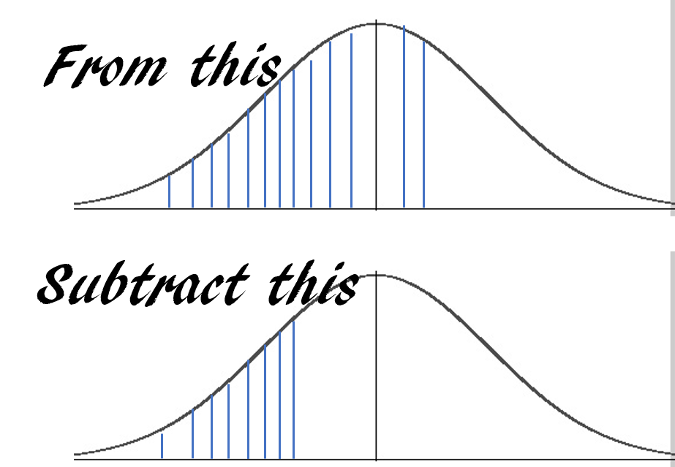
|
||||
|
||||
您可以使用 **pnorm** 只用一行代码获得答案:
|
||||
|
||||
```
|
||||
pnorm(12,10,2)-pnorm(7,10,2)
|
||||
> 0.7745375447996848
|
||||
```
|
||||
或者您可能需要回答以下问题:
|
||||
|
||||
假设你有一个不正的硬币,每次投它时有 60% 可能正面朝上。 你正在玩10次投掷游戏。 你如何绘制并给出这枚硬币所有可能的胜利数(从0到10)?
|
||||
|
||||
只需使用一个函数 **dbinom** 就可以获得一个只有几行代码的漂亮条形图:
|
||||
|
||||
```
|
||||
probs=[]
|
||||
import matplotlib.pyplot as plt
|
||||
for i in range(11):
|
||||
probs.append(dbinom(i,10,0.6))
|
||||
plt.bar(range(11),height=probs)
|
||||
plt.grid(True)
|
||||
plt.show()
|
||||
```
|
||||
|
||||
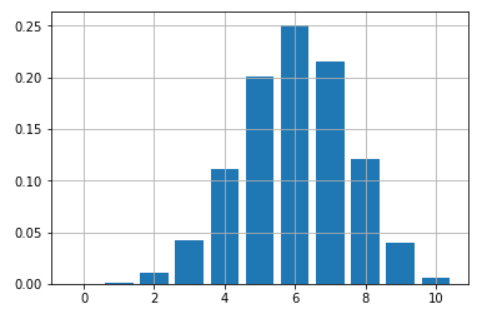
|
||||
|
||||
### 简单概率计算接口
|
||||
|
||||
R 提供了一个非常简单直观的界面,可以从基本概率分布中快速计算。 接口如下:
|
||||
|
||||
* **d** {distribution} 给出点 **x** 处的密度函数值
|
||||
* **p** {distribution} 给出 **x**点的累积值
|
||||
* **q** {distribution} 以概率 **p**给出分位数函数值
|
||||
* **r** {distribution} 生成一个或多个随机变量
|
||||
|
||||
在我们的实现中,我们坚持使用此接口及其关联的参数列表,以便您可以像在 R 环境中一样执行这些函数。
|
||||
|
||||
### 目前实现的函数
|
||||
|
||||
脚本中实现了以下R风格函数,以便快速调用。
|
||||
|
||||
* 均值,中位数,方差,标准差
|
||||
* Tuckey 五数总结,IQR
|
||||
* 矩阵的协方差或两个向量之间的协方差
|
||||
* 以下分布的密度,累积概率,分位数函数和随机变量生成:正态,均匀,二项式,泊松,F,学生t,卡方,β和伽马
|
||||
|
||||
### 进行中的工作
|
||||
|
||||
显然,这是一项正在进行的工作,我计划在此脚本中添加一些其他方便的R函数。 例如,在 R 中,单行命令 ** lm ** 可以为数字数据集提供一个普通的最小二乘拟合模型,其中包含所有必要的推理统计量(P 值,标准误差等)。 这是有力的简洁和紧凑! 另一方面,Python 中的标准线性回归问题经常使用 [Scikit-learn] [6] 来处理,这需要更多的脚本用于此用途,所以我打算使用 Python 的 [statsmodels] 合并这个单函数线性模型拟合特征 [7]后端。
|
||||
|
||||
如果您喜欢并在工作中使用此脚本,请通过主演或分析其 [GitHub存储库] [8]帮助其他人找到它。 另外,您可以查看我的其他 [GitHub repos] [9],了解 Python,R 或 MATLAB 中的有趣代码片段以及一些机器学习资源。
|
||||
|
||||
如果您有任何问题或想法要分享,请通过 [tirthajyoti [AT] gmail.com] [10]与我联系。 如果你像我一样热衷于机器学习和数据科学,请 [加我在LinkedIn] [11]或[在Twitter上关注我。] [12]
|
||||
|
||||
最初发表于[走向数据科学] [13]。 转载于[CC BY-SA 4.0] [14]。
|
||||
|
||||
--------------------------------------------------------------------------------
|
||||
|
||||
via: https://opensource.com/article/18/10/write-favorite-r-functions-python
|
||||
|
||||
作者:[Tirthajyoti Sarkar][a]
|
||||
选题:[lujun9972][b]
|
||||
译者:[yongshouzhang](https://github.com/yongshouzhang)
|
||||
校对:[校对者ID](https://github.com/校对者ID)
|
||||
|
||||
本文由 [LCTT](https://github.com/LCTT/TranslateProject) 原创编译,[Linux中国](https://linux.cn/) 荣誉推出
|
||||
|
||||
[a]: https://opensource.com/users/tirthajyoti
|
||||
[b]: https://github.com/lujun9972
|
||||
[1]: https://spectrum.ieee.org/at-work/innovation/the-2018-top-programming-languages
|
||||
[2]: https://www.coursera.org/lecture/r-programming/overview-and-history-of-r-pAbaE
|
||||
[3]: http://adv-r.had.co.nz/Functional-programming.html
|
||||
[4]: https://github.com/tirthajyoti/StatsUsingPython/blob/master/R_Functions.py
|
||||
[5]: https://en.wikipedia.org/wiki/Five-number_summary
|
||||
[6]: http://scikit-learn.org/stable/
|
||||
[7]: https://www.statsmodels.org/stable/index.html
|
||||
[8]: https://github.com/tirthajyoti/StatsUsingPython
|
||||
[9]: https://github.com/tirthajyoti?tab=repositories
|
||||
[10]: mailto:tirthajyoti@gmail.com
|
||||
[11]: https://www.linkedin.com/in/tirthajyoti-sarkar-2127aa7/
|
||||
[12]: https://twitter.com/tirthajyotiS
|
||||
[13]: https://towardsdatascience.com/how-to-write-your-favorite-r-functions-in-python-11e1e9c29089
|
||||
[14]: https://creativecommons.org/licenses/by-sa/4.0/
|
@ -0,0 +1,137 @@
|
||||
[#]: collector: (lujun9972)
|
||||
[#]: translator: (chenxinlong)
|
||||
[#]: reviewer: ( )
|
||||
[#]: publisher: ( )
|
||||
[#]: subject: (How To Configure IP Address In Ubuntu 18.04 LTS)
|
||||
[#]: via: (https://www.ostechnix.com/how-to-configure-ip-address-in-ubuntu-18-04-lts/)
|
||||
[#]: author: (SK https://www.ostechnix.com/author/sk/)
|
||||
[#]: url: ( )
|
||||
|
||||
如何在 Ubuntu 18.04 LTS 中配置 IP 地址
|
||||
======
|
||||
|
||||
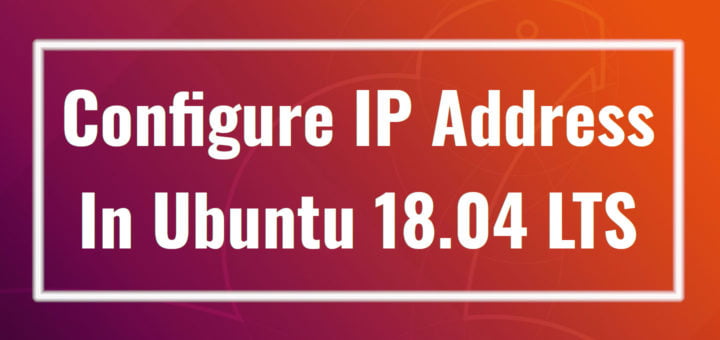
|
||||
|
||||
|
||||
在 Ubuntu 18.04 LTS 中配置 IP 地址的方法和以往使用的配置方法有很大的不同。和旧版本的不同之处在于,Ubuntu 18.04 使用 **Netplan** 来配置 IP 地址,**Netplan** 是一个新的命令行网络配置工具。其实在 Ubuntu 17.10 的时候 Ubuntu 开发者就已经介绍过 Netplan 了。接下来要介绍的新的 IP 配置方法不会再用到 **/etc/network/interfaces** 这个文件,取而代之的是一个 YAML 文件。默认的 Netplan 配置文件一般在 **/etc/netplan** 目录下。 在这篇教程中,我们会去学习在 **Ubuntu 18.04 LTS** 的最小版本中配置静态 IP 和动态 IP 。
|
||||
|
||||
### 在 Ubuntu 18.04 LTS 中配置静态 IP 地址
|
||||
|
||||
首先先找到 Netplan 默认的网络配置文件所在之处:
|
||||
|
||||
```
|
||||
$ ls /etc/netplan/
|
||||
50-cloud-init.yaml
|
||||
```
|
||||
|
||||
我们可以看到,默认的网络配置文件是 **50-cloud-init.yaml** ,这是一个 YAML 文件。
|
||||
|
||||
然后我们再看一下这个文件的内容是什么:
|
||||
|
||||
```
|
||||
$ cat /etc/netplan/50-cloud-init.yaml
|
||||
```
|
||||
|
||||
我在之前安装 Ubuntu 18.04 的时候为了从 DHCP 服务器获取 IP 地址已经已经做过了网卡的相关配置,所以详细配置直接看下图:
|
||||
|
||||
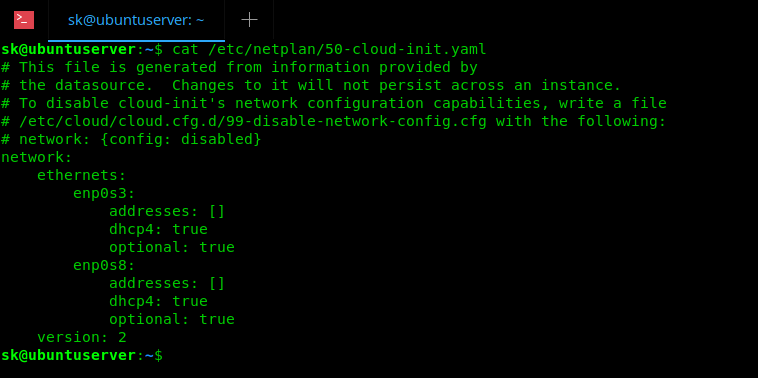
|
||||
|
||||
可以看到这边有两个网卡,分别是 **enp0s3** 和 **enp0s8** ,并且这两个网卡都配置为从 DHCP 服务器中获取 IP。
|
||||
|
||||
现在我们给这两个网卡都配置为静态 IP 地址,先用任意一种编辑器来编辑配置文件。
|
||||
|
||||
```
|
||||
$ sudo nano /etc/netplan/50-cloud-init.yaml
|
||||
```
|
||||
|
||||
接下来我们分别添加 IP 地址、子网掩码、网关、DNS 服务器等配置。分别用 **192.168.225.50** 作为网卡 **enp0s3** 的 IP 地址, **192.168.225.51** 作为网卡 **enp0s8** 的 IP 地址, **192.168.225.1** 作为网关地址, **255.255.255.0** 作为子网掩码。然后用 **8.8.8.8** 、 **8.8.4.4** 这两个 DNS 服务器 IP。
|
||||
|
||||
|
||||
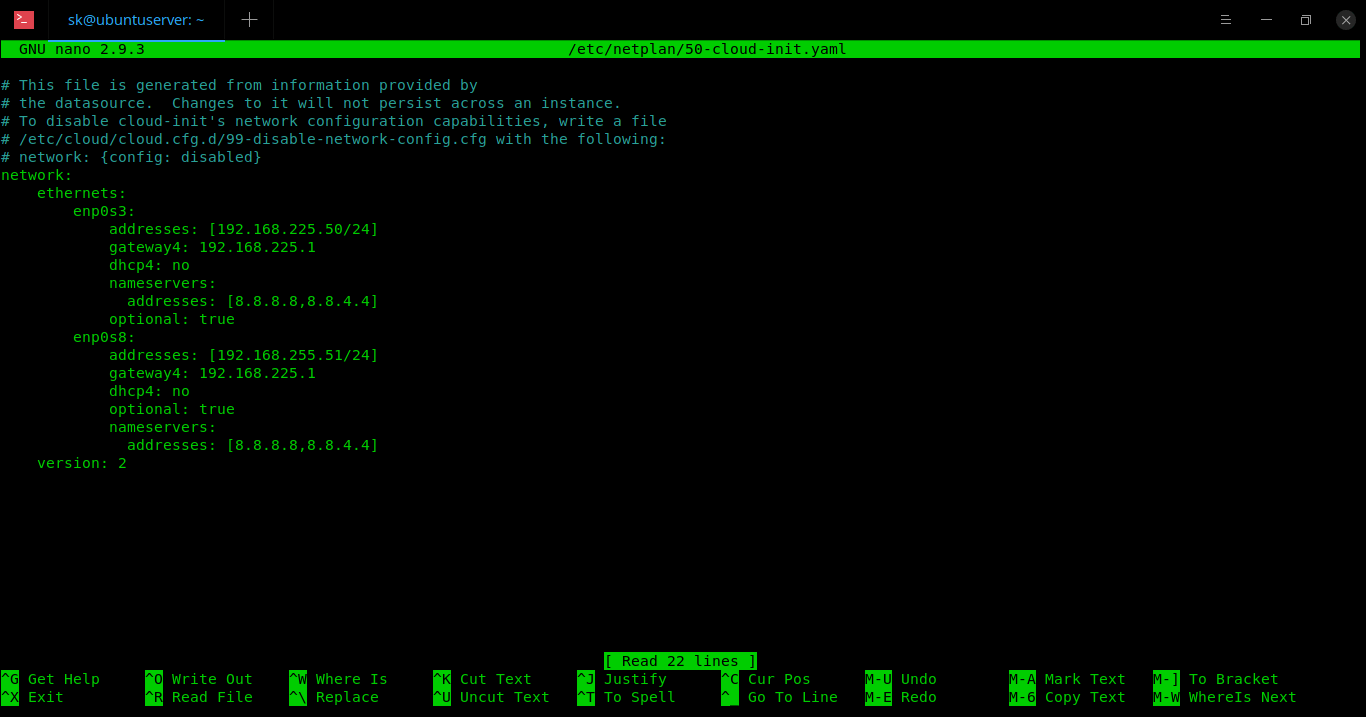
|
||||
|
||||
要注意的一点是,在 Ubuntu 18.04 里,这个配置文件的每一行都必须靠空格来缩进,不能用 **TAB** 来代替,否则配置会不起作用。如上图所示的配置文件中每行的缩进都是靠空格键实现的。
|
||||
|
||||
同时,在 Ubuntu 18.04 中,我们定义子网掩码的时候不是像旧版本的那样把 IP 和子网掩码分成两项配置。在旧版本的 Ubuntu 里,我们一般配置的 IP 和子网掩码是这样的:
|
||||
|
||||
```
|
||||
address = 192.168.225.50
|
||||
netmask = 255.255.255.0
|
||||
```
|
||||
|
||||
而在 netplan 中,我们把这两项合并成一项,就像这样:
|
||||
|
||||
```
|
||||
addresses : [192.168.225.50/24]
|
||||
```
|
||||
|
||||
配置完成之后保存并关闭配置文件。然后用下面这行命令来应用刚才的配置:
|
||||
|
||||
```
|
||||
$ sudo netplan apply
|
||||
```
|
||||
|
||||
如果在应用配置的时候有出现问题的话,可以通过如下的命令来查看刚才配置的内容出了什么问题。
|
||||
|
||||
```
|
||||
$ sudo netplan --debug apply
|
||||
```
|
||||
|
||||
这行命令会输出这些 debug 信息:
|
||||
|
||||
```
|
||||
** (generate:1556): DEBUG: 09:14:47.220: Processing input file //etc/netplan/50-cloud-init.yaml..
|
||||
** (generate:1556): DEBUG: 09:14:47.221: starting new processing pass
|
||||
** (generate:1556): DEBUG: 09:14:47.221: enp0s8: setting default backend to 1
|
||||
** (generate:1556): DEBUG: 09:14:47.222: enp0s3: setting default backend to 1
|
||||
** (generate:1556): DEBUG: 09:14:47.222: Generating output files..
|
||||
** (generate:1556): DEBUG: 09:14:47.223: NetworkManager: definition enp0s8 is not for us (backend 1)
|
||||
** (generate:1556): DEBUG: 09:14:47.223: NetworkManager: definition enp0s3 is not for us (backend 1)
|
||||
DEBUG:netplan generated networkd configuration exists, restarting networkd
|
||||
DEBUG:no netplan generated NM configuration exists
|
||||
DEBUG:device enp0s3 operstate is up, not replugging
|
||||
DEBUG:netplan triggering .link rules for enp0s3
|
||||
DEBUG:device lo operstate is unknown, not replugging
|
||||
DEBUG:netplan triggering .link rules for lo
|
||||
DEBUG:device enp0s8 operstate is up, not replugging
|
||||
DEBUG:netplan triggering .link rules for enp0s8
|
||||
```
|
||||
|
||||
如果配置正常且生效的话,我们可以用下面这个命令来查看一下 ip:
|
||||
|
||||
```
|
||||
$ ip addr
|
||||
```
|
||||
|
||||
在我的 Ubuntu 18.04 中配置完之后执行命令输出的信息如下:
|
||||
|
||||
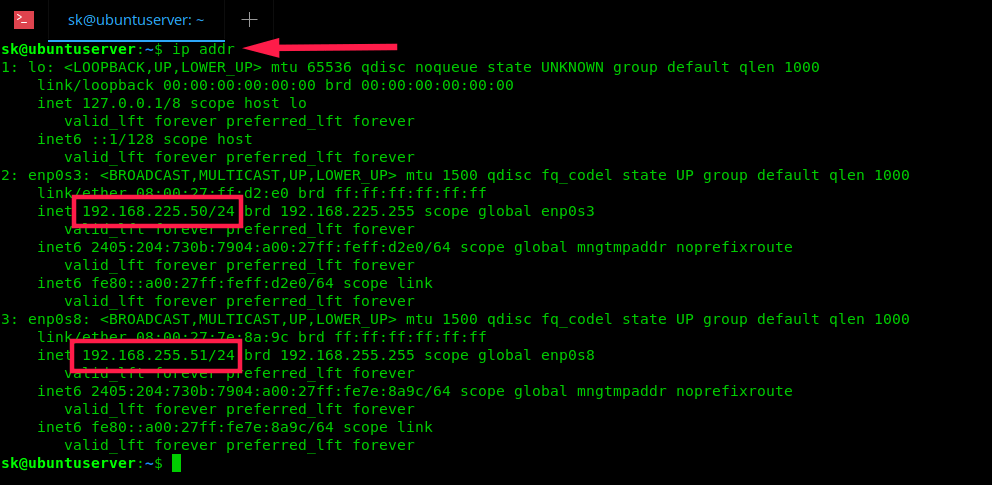
|
||||
|
||||
到此为止,我们已经成功地在 Ubuntu 18.04 LTS 中用 Netplan 完成了静态 IP 的配置。
|
||||
|
||||
更多关于 Netplan 的信息,可以在用 man 命令在手册中查看:
|
||||
|
||||
```
|
||||
$ man netplan
|
||||
```
|
||||
|
||||
### 在 Ubuntu 18.04 LTS 中配置动态 IP 地址
|
||||
|
||||
其实配置文件中的初始配置就是动态 IP 的配置,所以你想要使用动态 IP 的话不需要再去做任何的配置操作。如果你已经配置了静态 IP 地址,想要恢复之前动态 IP 的配置,就把在上面静态 IP 配置中所添加的相关配置项删除,把整个配置文件恢复成上面的图 1 所示的样子就行了。
|
||||
|
||||
现在你已经学会在 Ubuntu 18.04 中配置静态和动态 IP 地址了。个人而言,我 (原作者) 其实不太喜欢这种方式,旧的配置方式反而来得简单。你们觉得呢 ?
|
||||
|
||||
|
||||
|
||||
--------------------------------------------------------------------------------
|
||||
|
||||
via: https://www.ostechnix.com/how-to-configure-ip-address-in-ubuntu-18-04-lts/
|
||||
|
||||
作者:[SK][a]
|
||||
选题:[lujun9972][b]
|
||||
译者:[chenxinlong](https://github.com/chenxinlong)
|
||||
校对:[校对者ID](https://github.com/校对者ID)
|
||||
|
||||
本文由 [LCTT](https://github.com/LCTT/TranslateProject) 原创编译,[Linux中国](https://linux.cn/) 荣誉推出
|
||||
|
||||
[a]: https://www.ostechnix.com/author/sk/
|
||||
[b]: https://github.com/lujun9972
|
Loading…
Reference in New Issue
Block a user